- 1Department of Microbiology and Molecular Genetics, Michigan State University, East Lansing, MI, United States
- 2Clinical Microbiology Laboratory, University of Michigan, Ann Arbor, MI, United States
- 3Microbiology Division, Detroit Medical Center University Laboratories, Wayne State University, Detroit, MI, United States
- 4Microbiology, Immunology & Molecular Laboratories, Sparrow Hospital, Lansing, MI, United States
- 5Bureau of Laboratories, Michigan Department of Health and Human Services, Lansing, MI, United States
Non-typhoidal Salmonella (NTS) are important enteric pathogens causing over 1 million foodborne illnesses in the U.S. annually. The widespread emergence of antibiotic resistance in NTS isolates has limited the availability of antibiotics that can be used for therapy. Since Michigan is not part of the FoodNet surveillance system, few studies have quantified antibiotic resistance frequencies and identified risk factors for NTS infections in the state. We obtained 198 clinical NTS isolates via active surveillance at four Michigan hospitals from 2011 to 2014 for classification of serovars and susceptibility to 24 antibiotics using broth microdilution. The 198 isolates belonged to 35 different serovars with Enteritidis (36.9%) predominating followed by Typhimurium (19.5%) and Newport (9.7%), though the proportion of each varied by year, residence, and season. The number of Enteritidis and Typhimurium cases was higher in the summer, while Enteritidis cases were significantly more common among urban vs. rural residents. A total of 30 (15.2%) NTS isolates were resistant to ≥1 antibiotic and 15 (7.5%) were resistant to ≥3 antimicrobial classes; a significantly greater proportion of Typhimurium isolates were resistant compared to Enteritidis isolates and an increasing trend in the frequency of tetracycline resistance and multidrug resistance was observed over the 4-year period. Resistant infections were associated with longer hospital stays as the mean stay was 5.9 days for patients with resistant isolates relative to 4.0 days for patients infected with susceptible isolates. Multinomial logistic regression indicated that infection with serovars other than Enteritidis [Odds ratio (OR): 3.8, 95% confidence interval (CI): 1.23–11.82] as well as infection during the fall (OR: 3.0; 95% CI: 1.22–7.60) were independently associated with resistance. Together, these findings demonstrate the importance of surveillance, monitoring resistance frequencies, and identifying risk factors that can aid in the development of new prevention strategies.
Introduction
The gram-negative pathogen, Salmonella enterica, is an important public health concern resulting in 93.8 million cases of foodborne infections globally each year (1). In 2015, infection with non-typhoidal S. enterica (NTS) serovars was a leading cause of death, with 90,300 deaths reported (2). Infections with NTS can cause nausea, vomiting, abdominal pain, myalgia (muscle pain) and arthralgia (joint pain), while hepatomegaly (liver enlargement), and splenomegaly (spleen enlargement) can develop in some cases (3). Systemic infections can also occur in immunocompromised patients (4). Because of these complications, NTS infections were estimated to contribute to 70 disability-adjusted life years (DALY) lost/100,000 persons worldwide in 2010 (5). In the U.S., the number of NTS infections was estimated to be 1.2 million per year with 23,000 hospitalizations and 450 deaths (6). Salmonella infections were also reported to have the highest mean cost of illness among all foodborne infections (7). Geographical differences in serovar prevalence have been documented as well (8). In Europe and Asia, for instance, S. Enteritidis was the leading cause of clinical infections in 2002, whereas S. Typhimurium was the highest in North America followed by S. Enteritidis, S. Newport and S. Heidelberg. The Typhimurium and Enteritidis serovars, however, have been reported to cause the greatest number of enterocolitis and bacteremia cases (3).
NTS has been frequently isolated from commercially raised chickens and other poultry, and contact with cattle, pigs, horses, and other domestic animals are important risk factors for NTS infections (9). In addition, 74,000 Salmonella infections have been attributed to reptile and amphibian exposures in the U.S. each year (10); contact with reptiles and cats was associated with salmonellosis in a prior Michigan study (11). Other studies have identified serovar-specific risk factors as well. A study in the Netherlands, for example, found consumption of raw eggs and products containing raw eggs to be linked to Salmonella Enteritidis infections, while exposure to raw meat and playing in a sandbox were risk factors for S. Typhimurium infections (12). Prior history of antibiotic use, living on a livestock farm, and international travel were also identified as risk factors for S. Typhimurium infections in Canada (13). NTS isolates have been recovered from environmental sources including water and soil and can often survive in these environments for extended periods of time (14, 15). Taken together, these studies indicate the importance of the environment as a reservoir for Salmonella.
Antibiotic-resistant NTS infections have also emerged and are increasing in frequency in the U.S. resulting in high hospitalization rates and ~$365,000,000 in medical costs every year (16). Fluoroquinolones, third generation cephalosporins, penicillins, macrolides, and trimethoprim-sulfamethoxazole are commonly prescribed for the treatment of salmonellosis, particularly in patients with immunocompromising conditions, young children and the elderly (17). Importantly, antibiotic resistant Salmonella infections have been linked to more severe disease outcomes including bloodstream infections as well as hospitalization (18) and multidrug resistance has emerged. Resistance to ampicillin, chloramphenicol, streptomycin, sulfonamides and tetracycline, previously defined as ACSSuT isolates, for instance, has been reported in multiple NTS serovars in different geographical regions (19–22). In addition to ACSSuT, resistance to ampicillin, streptomycin, sulfonamides, and tetracycline (ASSuT) has been observed in S. Typhimurium from humans, food animals and retail meats in the U.S. (23). The emergence of these multi-drug phenotypes in Salmonella are of great concern since alternative antibiotics that can be used to treat Salmonella infections are limited.
Emergence of widespread resistance in Salmonella is attributed to the overuse of antibiotics and limits the effectiveness of these antibiotics for the treatment of human infections. Michigan is not included in the CDC FoodNet surveillance network, which monitors the incidence of foodborne illnesses and collects case information from 10 states in the U.S., covering 15% of the U.S. population. Consequently, we sought to examine the distribution of NTS serovars causing disease in Michigan and evaluate risk factors for infection using an active surveillance system at four hospitals. We also calculated the frequency of antibiotic resistance in NTS isolates and identified factors associated with resistance and NTS infections. This study highlights the importance of enhanced surveillance for resistant pathogens to ensure that the most appropriate drug targets are used, and to identify risk factors for infection and patients with an increased risk of more debilitating conditions.
Materials and Methods
Strain Source and Collection
From 2011 to 2014, 198 NTS isolates were collected as part of the Enterics Research Investigational Network (ERIN), which was set up in collaboration with the Michigan Department of Health and Human Services (MDHHS) and four major hospitals in southern Michigan. Serovar classification was conducted at the MDHHS using traditional serotyping protocols as recommended by the Association of Public Health Laboratories (24); serovar data was extracted from the Michigan Disease Surveillance System (MDSS) for each case. To ensure that the ERIN surveillance network was representative of enteric infections occurring in Michigan, the frequency of ERIN cases was shown to be similar to those identified throughout the state of Michigan during the same time period (25). Isolates were stored in Luria-Bertani broth with 10% glycerol at −80°C until further testing.
Antimicrobial Susceptibility Profiling
For NTS, susceptibilities to 24 antibiotics were determined by broth microdilution using Sensititre GN4F Trek plates (Trek Diagnostic Systems, Cleveland, OH, USA) according to the manufacturer's instructions. Nine antibiotic classes were tested and included: aminoglycosides (amikacin, gentamicin, tobramycin); β-lactam antibiotics [penicillins (ampicillin, piperacillin); β-lactam/β-lactamase inhibitor combinations (ampicillin/sulbactam 2:1 ratio, piperacillin/ tazobactam constant 4, ticarcillin/clavulanic acid constant 2); cephalosporins (cefazolin, ceftazidime, ceftriaxone, cefipime)]; carbapenems (imipenem, doripenem, ertapenem, meropenem); tetracyclines (tetracycline, minocycline); fluoroquinolones (ciprofloxacin, levofloxacin); glycylcyclines (tigecycline); nitrofurans (nitrofurantoin); monobactams (aztreonam); and anti-folates (trimethoprim/sulfamethoxazole). The minimum inhibitory concentration (MIC) was determined by identifying the lowest concentration of antibiotic that prevented visible bacterial growth. Escherichia coli ATCC 25922, which is susceptible to all antibiotics evaluated, was used as the quality control strain. The results of the susceptibility tests were interpreted as resistant or susceptible in accordance with published guidelines (26) and isolates were classified as multidrug resistant if they were resistant to three or more antimicrobial classes.
Data Analysis
Epidemiological, demographic, and clinical data were obtained from the MDSS and managed using Microsoft Access and Excel. Season was classified as spring (March, April, and May), summer (June, July, and August), fall (September, October, and November) and winter (December, January, and February) based on the sample collection date; for those cases with a missing collection date, the stool arrival date and/or onset dates were used. Counties in Michigan were classified as urban or rural based on the classification scheme devised by the National Center for Health Statistics; only 10 Michigan counties were designated as urban (27). Based on the published rates of antibiotic prescription use in adults and children in Michigan (28), counties were classified as having high or low prescribing rates. High rates were defined as those counties where hospital service areas had >30% higher use rates relative to the state average. A dichotomous variable was created for length of hospital stay by defining a long hospital stay as one that was greater than 4 days.
SAS version 9.4 (SAS Institute, Cary, NC, USA) and Epi Info™ version 7.0 were used for all statistical analyses. χ2 test and Fisher's exact test were used for dichotomous variables to identify significant associations between the dependent and independent variables; a p-value ≤ 0.05 was considered significant. A univariate analysis was first conducted, and those variables found to have strong associations with the outcome (p-value ≤ 0.20) were included in the multivariate analysis unless the variable contained >15 missing values. Multivariate analysis using forward logistic regression was performed to build a model containing significant variables (p-value ≤ 0.05) along with potentially confounding factors such as age and sex. The Mantel-Haenszel χ2 test was used to test for trends and the student's t-test was used for testing statistical significance between means. Finally, the χ2 test for equality of proportions was used for comparing sample proportions.
Results
Characteristics of Cases With Non-typhoidal Salmonella (NTS) Infections
A total of 198 NTS cases were identified and roughly half were male (53.1%; n = 104) and between 18 and 52 years of age (n = 81; 40.9%). Forty-four (22.2%) cases were younger than 10 years of age and 14 were younger than 2 years. More cases self-reported as Caucasians (n = 125; 74.0%) than as African Americans (n = 33; 19.5%) or other races (n = 11; 6.5%); race was not known for 29 cases. Diarrhea (n = 172, 97.2%), abdominal pain (n = 135, 80.4%), and fever (n = 106, 69.3%) were commonly reported symptoms, though 70 (41.9%) patients also reported bloody diarrhea. Sixty-five patients (34.6%) were hospitalized between 1 day and 17 days; the average duration of hospitalization was 4.4 days.
No significant difference in the proportion of cases was observed by year (p-value = 0.075), though a higher number was reported in the summer and fall (n = 139; 70.2%) compared to the winter and spring months (p-value < 0.0001). Differences were also observed among the four participating hospitals, which represented 10.6, 23.2, 29.3, and 36.9% of the 198 cases. When stratified by county, 56.2% (n = 108) of the cases lived in rural counties and 43.7% (n = 84) resided in urban counties; the residence was not known for one case. A subset of five cases resided in other states (Colorado, Georgia, Illinois, Ohio, and South Dakota), though each patient developed symptoms and were diagnosed with salmonellosis while visiting Michigan. Of the 166 cases with travel information available, a significantly higher proportion of patients had not traveled in the past month (n = 103, 62.0%) when compared to those who reported travel (n = 63; 37.9%) (p-value = 0.0019).
Patients reporting contact with animals (n = 95; 61.3%) were also more likely to be affected when compared to those reporting no animal contact (n = 60; 38.7%) (p-value = 0.0049). Among these 95 cases, 12 (12.6%) had contact with reptiles such as turtles and lizards, and nine (9.5%) reported contact with livestock including cattle, horses, goats, or pigs. Contact with domestic animals (e.g., cats, dogs, and small mammals) was also reported in 83 of the 95 (87.4%) cases.
Because more cases resided in rural counties, we conducted a case-case analysis between the rural and urban cases to further detect differences in disease frequencies (Table S1); the five cases from other states were excluded from this analysis. Notably, any animal contact was more common in patients living in rural vs. urban counties (OR: 1.8; 95% CI: 0.95–3.57), however, the association was not statistically significant. Contact with “other” animals including small mammals, fish and/or amphibians was also more common in rural cases (Fisher's exact p-value = 0.0095), though only one case from the urban counties reported “other” contact. No significant difference was observed by season or for well water consumption in rural (n = 18, 19.8%) vs. urban (n = 7, 11.9%) cases; however, pork consumption was significantly lower (OR: 0.1; 95% CI: 0.02–1.12) and peanut butter consumption was significantly higher (OR: 2.1, 95% CI: 1.06–4.35) in rural vs. urban cases.
Risk Factors for More Severe NTS Infections
To identify predictors of hospitalization, a marker for more severe infections, we used hospitalization as the dependent variable among the 165 cases with data available. In the univariate analysis, patients self-reporting nausea (OR: 2.0; 95% CI: 1.05–3.91) and vomiting (OR: 1.9; 95% CI: 0.99–3.64) were significantly more likely to be hospitalized as were patients over the age of 59 years (n = 16; 51.6%). Patients from urban counties were also more likely to be hospitalized (n = 34; 41.9%) than cases from rural counties (n = 29; 27.9%). No associations were identified with specific serovars.
Multivariate logistic regression using forward selection identified urban residence (OR: 2.1; 95% CI: 1.06–4.04) and vomiting (OR: 1.9; 95% CI: 0.99–3.78) to be the strongest predictors of hospitalization while controlling for age and sex. Because vomiting and nausea were associated with each other (p-value < 0.0001), only vomiting was included in the model. Age over 59 years met the p > 0.25 significance level for entry into the model but despite the positive association (OR: 2.0; 95% CI: 0.87–4.78), it was not statistically significant.
Distribution of Salmonella enterica Serovars in Michigan Cases
The 198 NTS isolates were classified into 35 different S. enterica serovars; the serovar could not be determined for three isolates (Table S2). Among the 195 typed isolates, the predominant serovar was Enteritidis (n = 72; 36.9%) followed by Typhimurium (n = 38; 19.5%), Newport (n = 19; 9.7%), Hartford (n = 6; 3.1%), Saintpaul (n = 5; 2.6%), and Heidelberg (n = 4; 2.1%). The remaining 51 isolates represented 28 different serovars with fewer than three isolates per type. Nine isolates were classified as I 4, [5], 12:i:- /I 4, 5,12:i- (n = 3), I 4, 12:b- (n = 3), I 4, 12:i:- (n = 3), and III 50:Kz (n = 1), which likely represent variants of known serovars.
A significant difference in the proportion of Enteritidis cases was observed by hospital (p-value = 0.02). The highest proportion was observed at two sites with 24 (33.3%) and 25 (34.7%) Enteritidis cases, though 12 (16.7%) and 11 (15.3%) additional cases were identified at the remaining two sites. The proportion of Typhimurium cases also differed at the four hospitals (p-value = 0.003) with frequencies of 44.7% (n = 17), 34.2% (n = 13), 13.2% (n = 5), and 7.9% (n = 3) per site. S. Heidelberg was recovered from only one case at each hospital, but significant differences were observed in the proportion of Newport cases across hospitals (p-value = 0.009) as well as cases with all other serovars combined (p-value = 0.0003).
Differences were also observed in the proportion of specific serovars by year. In 2011 and 2012, for instance, 21.1% (n = 12) of the 57 cases and 35.2% (n = 18) of the 51 cases, respectively, were classified as Enteritidis, whereas higher frequencies were observed in 2013 (n = 20 of 34; 58.8%) and 2014 (n = 22 of 53; 41.5%). The proportion of Typhimurium cases was similar in years 2011, 2012, and 2014 (average = 16.2%), though a greater proportion of cases (n = 12; 35.3%) were recovered in 2013. Of the 19 Newport cases, the proportion of Newport cases decreased in 2013 (n = 2; 10.5%) and 2014 (n = 3; 15.8%) relative to 2011(n = 7; 36.8%) and 2012 (n = 7; 36.8%), however, the difference between each period was not significant (p-value = 0.22). Only four Heidelberg cases were identified and three of these cases were from 2014. Among all other serovars reported, it is notable that the highest frequencies were recovered in 2011 (n = 28; 49.1%) followed by 2014 (n = 17; 32.1%) and 2012 (n = 17; 33.3%). A more diverse serovar population was reported in 2011 as well given that infections were caused by 26 different serovars, while 15 and 17 serovars were reported in 2012 and 2014, respectively. Only Enteritidis, Typhimurium and Newport were recovered in 2013.
Because the number of cases differed by season with the highest proportion of cases occurring in summer than in other seasons (p-value < 0.0001), we also examined seasonal variation by serovar (Figure 1). The number of Enteritidis cases was significantly higher in the summer months (n = 34; 47.2%) when compared to the fall (n = 11; 15.3%), winter (n = 10; 13.9%), and spring (n = 17; 23.6%) months (p-value = 0.0001). Similar trends were observed for Typhimurium in the summer (n = 16, 42.1%) vs. fall (n = 10; 26.3%), winter (n = 6; 15.8%), and spring (n = 6; 15.8%) months. The majority (n = 14; 73.7%) of the 19 Newport cases were reported in the summer as were most cases infected with other serovars (n = 31; 46.9%). During the summer months, the greatest proportion of cases were caused by Enteritidis (n = 34, 35.8%), Typhimurium (n = 16, 16.8%), and Newport (n = 14, 14.7%).
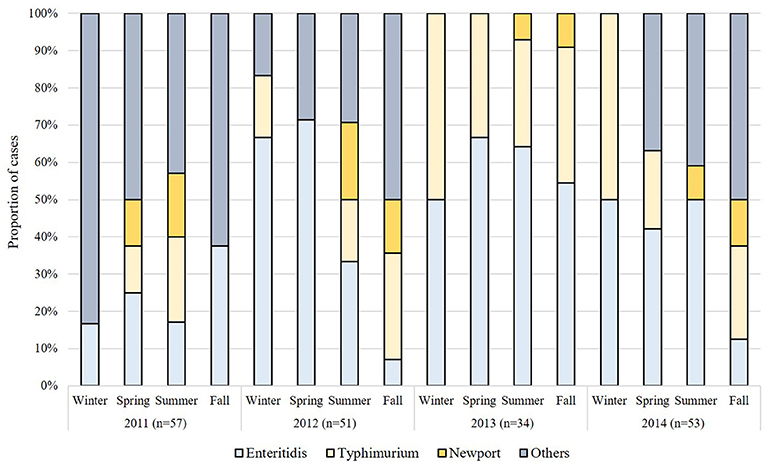
Figure 1. Seasonal variation in the distribution of non-typhoidal Salmonella (NTS) serovars in Michigan by year.
Risk Factors for Infection With Specific Salmonella Serovars
Several serovar-specific factors were identified. In the univariate analysis, significantly more Enteritidis cases were reported in urban (n = 40; 57.9%) vs. rural counties (n = 31; 37.3%) (p-value = 0.011). Enteritidis was also associated with consumption of bottled water at home (n = 11; 64.7%) relative to municipal (n = 30; 37.0%) (p-value = 0.03) or well water (n = 10, 52.6%) (p-value = 0.21) as well as pork consumption (OR: 7.2; 95% CI: 0.85–61.5). The small sample sizes and missing data records for these variables, however, may have skewed the associations and prevented their inclusion into the multinomial regression analysis.
By contrast, Typhimurium infections were more common in the 95 patients reporting animal contact (n = 24; 25.3%) than those without animal contact (n = 7; 12.1%) (p-value = 0.078). Contact with livestock (Fisher's exact p-value = 0.0002) and other animals such as amphibians, small mammals, and fish (OR: 3.8; 95% CI: 1.41–9.97) were more common in Typhimurium cases relative to cases infected with other serovars.
A multinomial logit model using forward selection was used containing the following three outcomes: (1) Enteritidis infection; (2) Typhimurium infection; and (3) infection by all other serovars. When controlling for age and sex, residence was found to be a significant predictor of Enteritidis but not Typhimurium infections. Specifically, cases living in rural areas were significantly less likely to have infections caused by Enteritidis (OR: 0.4; 95% CI: 0.23–0.85; p-value = 0.01) but not Typhimurium (OR: 1.2; 95% CI: 0.52–2.71; p-value = 0.69); only the former association was significant. Because of missing data and too few cases per outcome, however, no additional variables could be examined in the multivariate analysis.
Antibiotic Resistance Profiles in 198 Non-typhoidal Salmonella (NTS) Isolates
Resistance to at least one antibiotic was observed among 30 of the 198 (15.1%) NTS isolates (Figure 2). Resistance to the β-lactam, ampicillin (11.6%), and tetracycline (11.1%) predominated followed by resistance to trimethoprim-sulfamethoxazole (2.5%), gentamicin (0.5%), and other β-lactams including cephalosporins like cefazolin (2.0%), ceftazidime (2.0%), and ceftriaxone (1.0%). No resistance was observed to 13 of the 24 antibiotics tested. Overall, 19 distinct antibiotic resistance patterns were observed among the 30 resistant NTS isolates (Table S3). Multidrug resistance (MDR) to ≥ 3 antimicrobial classes was observed in 15 (7.5%) isolates while four (2.0%) isolates were resistant to ≥ 4 antimicrobial classes; nine (4.5%) isolates were resistant to only one antimicrobial class. When stratified by serovar (Figure 3), only 10 of the 35 NTS serovars contained isolates that were resistant to at least one antibiotic. Among these 10 serovars, Enteritidis was significantly less likely to comprise resistant isolates than Typhimurium (Fisher's exact p-value = 0.022) as well as the other eight serovars combined (Fisher's exact p-value < 0.0001). In all, four S. Enteritidis (n = 72; 5.6%) and eight S. Typhimurium (n = 38; 21.0%) isolates were resistant to at least one antibiotic.
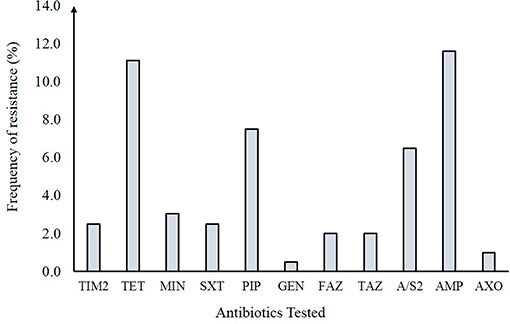
Figure 2. Antibiotic resistance frequencies in 198 non-typhoidal Salmonella (NTS) isolates from Michigan. TIM2, Ticarcillin / clavulanic acid constant 2; TET, Tetracycline; MIN, Minocycline; SXT, Trimethoprim / sulfamethoxazole; PIP, Piperacillin; \ GEN, Gentamicin; FAZ, Cefazolin; TAZ, Ceftazidime; A/S2, Ampicillin / sulbactam 2:1 ratio; AMP, Ampicillin; AXO, Ceftriaxone.
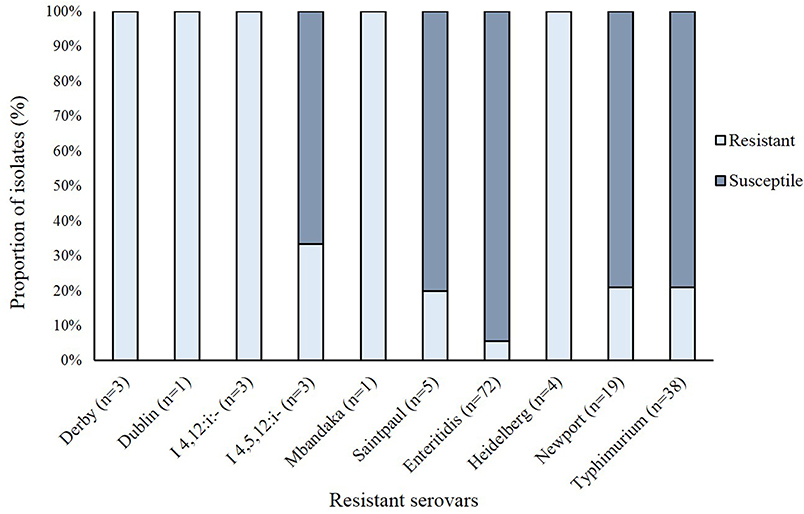
Figure 3. Frequency of antibiotic resistance in 149 non-typhoidal Salmonella (NTS) isolates representing the 10 serovars with at least one resistant isolate.
Important differences in resistance frequencies were also identified by year of isolation (Figure 4). Significant increases in resistance to tetracycline and cephalosporins, for example, were observed between 2011 and 2014 as were increases in multidrug resistance (p-value < 0.05). No significant difference in the frequency of resistance to trimethoprim-sulfamethoxazole or gentamicin were noted. A comparison between all NTS isolates from Michigan to those tested by the National Antimicrobial Resistance Monitoring System (NARMS) (29) during the same time period also revealed slight differences in resistance frequencies by antibiotic (Figure 5A). None of these differences, however, were significant. For Enteritidis, tetracycline resistance was lower in Michigan (n = 1, 1.4%) than NARMS (n = 48, 3.0%) isolates (Figure 5B), while resistance to ampicillin and tetracycline was higher in Typhimurium isolates from NARMS than Michigan (Figure 5C). Resistance to trimethoprim-sulfamethoxazole was also higher in the Michigan Typhimurium isolates (n = 2, 5.3%) compared to the NARMS isolates (n = 21, 1.7%), yet none of the frequency differences observed among Enteritidis and Typhimurium isolates were statistically significant.
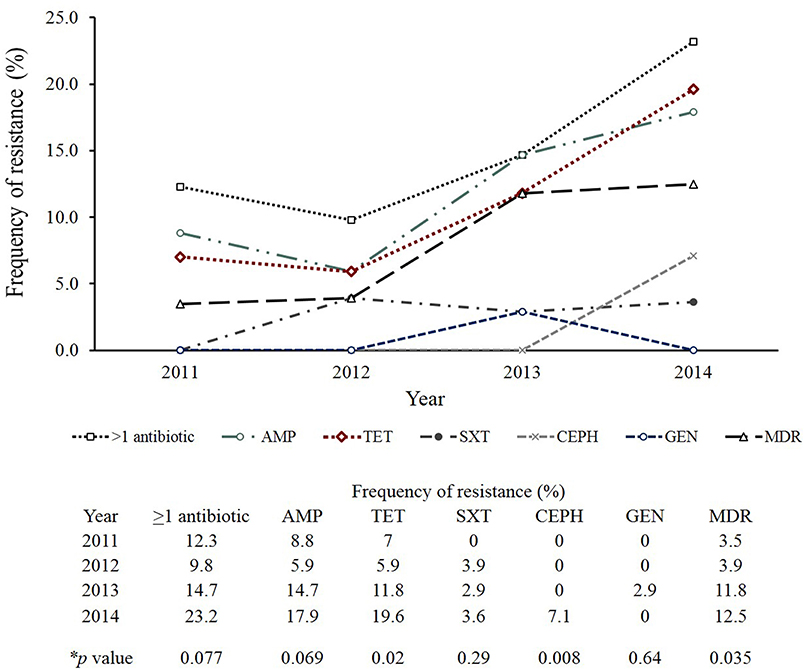
Figure 4. Trends in antibiotic resistance among clinical non-typhoidal Salmonella (NTS) isolates from Michigan over time. Mantel-Haenszel chi-square was used to identify trends over time and calculate p-values. AMP, Ampicillin; TET, Tetracycline; SXT, Trimethoprim/sulfamethoxazole; CEPH, Cephalosporin; GEN, Gentamicin; MDR, Multidrug resistance (resistance to ≥3 antimicrobial classes).
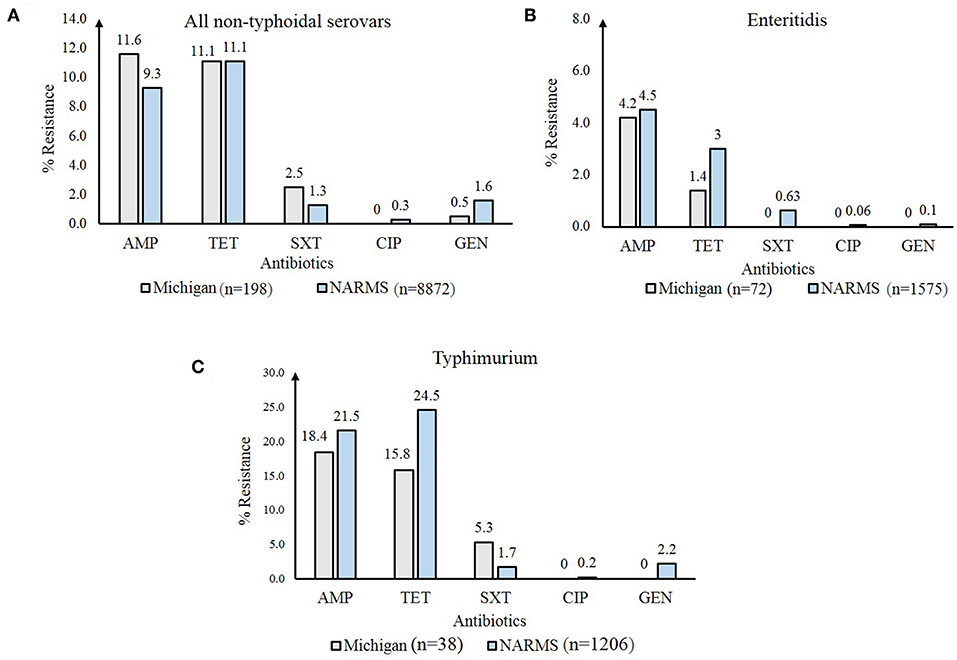
Figure 5. Frequency of antibiotic resistance among Non-Typhoidal Salmonella (NTS) isolates from Michigan compared to those from the National Antimicrobial Resistance Monitoring System (NARMS) (29), 2011-2014. Resistance frequencies in: (A) all NTS serovars; (B) Enteritidis; and (C) Typhimurium. AMP, ampicillin; TET, tetracycline; SXT, trimethoprim-sulfamethoxazole; CIP, ciprofloxacin; GEN, gentamicin.
Epidemiological Associations With Antibiotic Resistant NTS Infections
To identify factors associated with resistant NTS infections, we conducted univariate and multivariate analyses using resistance to at least one (≥ 1) antibiotic as the dependent variable. The univariate analysis demonstrated that the odds of resistance was significantly higher in Typhimurium isolates (OR: 4.5; 95% CI: 1.27-16.22) and other NTS serovars (OR: 4.6; 95% CI: 1.47-14.20) compared to Enteritidis isolates (Table 1). Higher resistance frequencies were also observed in counties with low antibiotic prescribing rates (n = 26, 16.9%) compared to those with high rates (n = 4, 10.3%), yet this difference was not statistically significant. Furthermore, frequencies of antibiotic resistant infections were higher in urban (n = 14, 16.7%) than rural (n = 16, 14.8%) areas and were lowest in the summer (n = 10, 10.3%) compared to winter, spring and fall (n = 20, 19.8%) (OR: 2.1; 95% CI: 0.95-4.86).
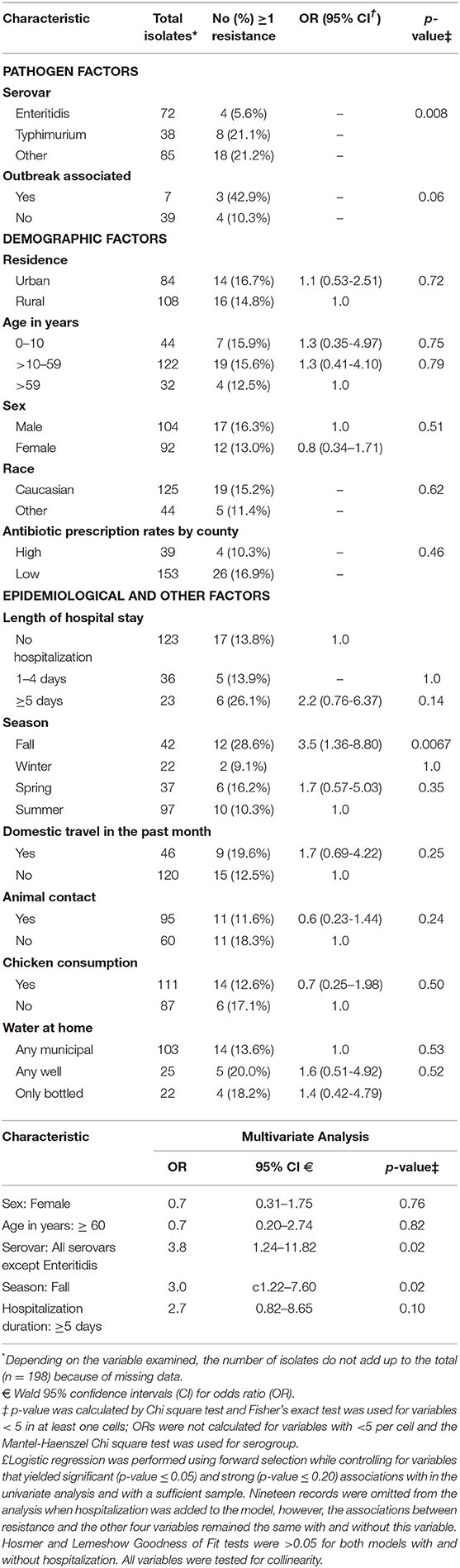
Table 1. Univariate and multivariate analysis to identify factors associated with antibiotic resistance in 198 clinical non-typhoidal Salmonella in Michigan, 2011–2014.
Notable differences in resistance frequencies were observed among the 59 patients who were hospitalized for one or more days. Among these 59 cases, the mean hospital stay was 5.9 days for the 39 patients hospitalized with resistant NTS isolates compared to 4.0 days for the 48 patients infected with pansusceptible isolates (Table S4). Six of the 23 (26.1%) patients with hospital stays of ≥5 days had resistant infections compared to five of the 36 (13.9%) patients with stays between 1 and 4 days (Student's t-test p-value < 0.05). Cases with tetracycline resistant and susceptible infections had mean hospital stays of 6.0 days and 4.2 days, respectively (Student's t-test p-value = 0.068), whereas the mean hospital stay was 6.2 days for patients infected with ampicillin-resistant NTS compared to 4.0 days for patients with ampicillin-susceptible infections (Student's t-test p-value < 0.05). No association was observed between hospitalization and infection with either Enteritidis (n = 21, 30.9%) or Typhimurium (n = 11, 31.4%) when compared to all other serovars (n = 30, 36.6%).
Multivariate analysis using forward regression was performed to identify predictors of resistant infections. Because the frequency of resistance to ≥1 antibiotic was similar in isolates belonging to Typhimurium and the other NTS serovars except Enteritidis, they were grouped together for the multivariate analysis. After adjusting for sex and age, the model indicated that compared to Enteritidis, the remaining serovars were 3.8 times more likely to be resistant to at least one antibiotic (Table 1). Moreover, resistant NTS infections were significantly more likely to occur in fall (OR: 3.1; 95% CI: 1.22–7.60) than in the other three seasons. A strong association (OR: 2.7) was also observed between resistance and hospitalization stays longer than 5 days, however, it was not statistically significant while controlling for the other four variables.
Discussion
This study, which was conducted between 2011 and 2014 using data and isolates from an active surveillance system at four large, metropolitan hospital systems, indicated that most (64.1%) of the 198 cases were over the age of 19 and 16.2% were over 60 years of age. These data differ from those reported in a prior Michigan study of Enteritidis infections occurring between 1995 and 2001, which found children <4 years of age to have the greatest risk of infection (30). Although age was not significantly associated with Enteritidis infections or infections caused by any other serovars, these data suggest that the demographics of salmonellosis cases may have changed over time. Additional studies in different Michigan hospitals are therefore required.
Significantly more NTS infections occurred in Michigan during the summer and fall months consistent with a CDC report showing that most Salmonella infections occur between June and October in the U.S. (31). An increased frequency of infections caused by S. Enteritidis specifically (30) and other enteric pathogens has also been reported during the summer. Shiga toxin-producing E. coli (STEC) infections, for example, were highest in Michigan during the summer (37.3%) and fall (29.5%) over a 12-year period (2001–2012) (32). Such seasonal variation has been explained by recreational activities, inadequate cooking and suboptimal food storage temperatures during outdoor picnics and barbeques (33) as well as visiting petting zoos and farms (34). Variation in the distribution of serovars by season was also observed and is consistent with data from a study of 690,479 Salmonella infections reported to the CDC. Specifically, this study found that the number of different Salmonella serotypes causing human infection, or the “serotype richness,” was greatest in the winter even though the greatest number of infections occurred during the summer (35).
Although salmonellosis frequencies were not significantly higher in patients residing in rural vs. urban areas (p-value = 0.083), urban residence was found to be a predictor of infection with S. Enteritidis using logistic regression. Intriguingly, urban residence was also associated with hospitalization due to any NTS infection, a finding that may be related to proximity of the health care facilities included in the study. The association between residence location and infection with specific serovars is supported by data from prior studies showing a lower prevalence of Enteritidis in the farm environment relative to other serovars. A Canadian study of urban and rural streams, for example, detected low frequencies of Enteritidis in the rural streams (36), while Enteritidis was rarely recovered from livestock and poultry in Alberta (37) or in the environment of commercial poultry farms in California (38). It is therefore possible that Salmonella serovars other than Enteritidis may be more widespread in the environment. An association was also observed between Typhimurium infections and history of animal contact, which has been reported in many prior studies. Indeed, one study indicated that living on a livestock farm was an independent risk factor for acquiring S. Typhimurium DT104 infections (13), while another study recovered indistinguishable S. Typhimurium DT104 isolates from a child and animals living on the same farm (39). The identification of animal contact as a risk factor for NTS infections in Michigan may be important as it highlights the need for additional studies to investigate this epidemiological association and develop more targeted prevention strategies. Indeed, we were only able to evaluate whether or not any animal contact was reported, which fails to adequately describe the level or duration of contact or even the well-being of the animals.
Importantly, a wide range of antibiotic resistance profiles in the 198 NTS isolates recovered from patients at four Michigan hospitals was observed. High frequencies of resistance to ampicillin and tetracycline were identified with an increasing trend in tetracycline resistance over the 4-year period; tetracycline resistance also contributed to the high frequency of MDR in the NTS isolates examined. It is notable that an increase in tetracycline resistance was not observed by NARMS for the same time period (p-value = 0.66) and hence, it is possible that Michigan harbors a unique population of resistant NTS. NARMS also reported an increased frequency of MDR in 2015 (12%) relative to 2008 (9.5%) (29). While tetracycline is not widely recommended for clinical use, antibiotics such as amoxicillin, penicillin, and sulfamethoxazole-trimethoprim are commonly prescribed to adults and children in Michigan (28). The high prescription rates of these antibiotics, among others, is likely to have an impact on resistance frequencies in the state. Unfortunately, actual antibiotic usage data was not available for NTS cases in our study as these data are not collected by the MDHHS during case interviews. We also observed an increasing trend in the frequency of MDR in NTS over the 2011–2014 time period, however, the difference was not significant by year and could be due to our focused surveillance of only four hospitals. It is possible that increases in MDR frequencies over time could be linked to international travel or food imports as suggested previously (40, 41); however, travel was not associated with MDR infections in our study and import risk was not evaluated.
Although tetracyclines are not widely used in human medicine, they are among the most commonly used antibiotics in livestock and poultry worldwide (42). The FDA has estimated that 3,535,701 (kg)2 of tetracyclines are used in food-producing animals in the U.S. each year representing 64% of all antibiotics used (43). While the use of tetracyclines in these animals decreased by 40% in 2016–2017 relative to the period between 2009 and 2017 (43), antibiotic residues and resistance determinants can persist in the environment (44) and animal reservoir, be transmitted to humans, and contribute to MDR in both gram-positive and -negative bacteria (45). Indeed, the emergence and spread of resistance between food animals and people has been documented. One historical study, for instance, found identical resistance patterns in E. coli isolates from livestock and farming families (46), while others have documented spread through food products and water (47–49). Since we have only examined resistance in clinical NTS isolates, additional studies are needed to quantify resistance frequencies in isolates from other sources to identify those strain types, virulence gene profiles, and resistance phenotypes that pose the highest risk of transmission to and infection in humans. A recent study, for example, documented different antibiotic resistance profiles among isolates from different sources and suggested that multiple sources in the food-chain are responsible for resistant Salmonella infections in humans (23).
As such, it is important to consider that the genetic and phenotypic diversity of NTS isolates in different geographical locations may play a role in human infections as specific NTS lineages circulating in Michigan may be more likely to carry resistance determinants. Support for this possibility comes from our finding that NTS serovars had varying resistance profiles. Notably, multivariate logistic regression identified serovar Enteritidis to be significantly less likely to be resistant to at least one antibiotic compared to all other NTS serovars. Serovar-dependent differences in resistance have been observed in NTS isolates in different geographic locations including Spain (50) and Brazil (51). The reason for serovar-specific differences is not clear, however, one study found that ciprofloxacin-resistant S. Typhimurium isolates were more competitive during growth in vitro with increasing concentrations of ciprofloxacin than the ciprofloxacin-resistant S. Enteritidis isolates (52). Other studies have shown that certain NTS serovars such as Kentucky, Typhimurium, and Heidelberg, were more likely to have MDR (53–55), whereas some serovars (e.g., Enteritidis, Montevideo, Infantis, and Mbandaka) were more commonly pansusceptible or were resistant to fewer antibiotics (56, 57). Such differences could be due to the specificity of or ability to take up certain plasmids carrying resistance genes as some plasmids may be more commonly acquired across serovars and other bacterial species (58). Serovars such as Kentucky and Heidelberg have also been shown to have a mutation in the methyl mismatch repair (MMR) system, which may contribute to genetic heterogeneity (59). This genome plasticity has been offered as an explanation for higher frequencies of antibiotic resistance within these strain backgrounds (60). Differences in fitness between serovars, genetic plasticity and dissimilar resistance mechanisms could partly explain the varying frequencies of resistance in NTS serovars worldwide. Although serogrouping has been useful for differentiating NTS isolates for surveillance studies (61), future studies are needed to characterize the isolates using whole genome sequencing to identify bacterial features commonly associated with specific resistance phenotypes and carriage of specific resistance determinants. Having these data could help identify sources of resistant infections and identify whether some strains are epidemiologically linked.
Our study also identified season to be a predictor of resistant NTS infections, with fewer resistant infections occurring in the summer and more in the fall as is consistent with seasonal variation observed in prior studies. A study of fluoroquinolone resistance in Campylobacter, for example, observed higher frequencies in the winter and spring compared to the summer. This difference was attributed to higher consumption of poultry products containing resistant bacteria in the winter and more frequent exposure to susceptible Campylobacter through other sources in the summer (62). Our prior studies have also observed higher resistance frequencies in C. jejuni (63) and STEC (64) recovered from Michigan patients in the winter and/or spring and could be due, in part, to seasonal variation in antibiotic prescription rates (65, 66). Because prescribing rates may vary by the type of infection and geographic location given climate and other factors, additional studies are required to understand the most important factors that impact antibiotic resistance development and trends in Michigan.
While we did not identify antibiotic resistant NTS infections to be a significant predictor of hospitalization, we did observe a strong association between resistance in NTS and a longer duration (≥5 days) of hospitalization. This finding is not surprising given that resistant infections take longer to clear than susceptible infections during antibiotic treatment and is consistent with other studies. Importantly, studies in other pathogens have identified associations between resistance and mortality, hospitalization, hospital stay duration, and the need for surgery (18, 64, 67, 68).
Because NTS pathogens are a leading cause of enteric infections worldwide and have been shown to frequently resist clinically important antibiotics, continuous surveillance, and monitoring is critical. NTS isolation and routine testing for resistance is imperative in order to help medical personnel and public health officials determine and modify the course of treatment for NTS infections. Furthermore, identifying risk factors for NTS and resistant NTS infections may help in the development of disease management policies and antibiotic use standards aimed at curbing the spread of NTS and resistance determinants.
Data Availability Statement
All datasets generated for this study are included in the article/Supplementary Material.
Ethics Statement
The studies involving human participants were reviewed and approved by Institutional Review Boards at Michigan State University (MSU; Lansing, MI, USA; IRB #10-736SM), the MDHHS (842-PHALAB), and the four participating hospitals. Written informed consent from the participants' legal guardian/next of kin was not required to participate in this study in accordance with the national legislation and the institutional requirements.
Author Contributions
SM performed the experiments with CA and RM organized samples and extracted the epidemiological data. SM and SDM managed the data, conducted the analyses, and drafted the manuscript. SM, SDM, JR, DN, HS, PL, and WK designed the study and organized sample collection at each site. All authors contributed and approved the manuscript content.
Funding
This work was supported by the National Institutes of Health Enterics Research Investigational Network Cooperative Research Center (U19AI090872 to SDM), the Michigan State University (MSU) Foundation, and the United States Department of Agriculture (MICL02475). The Department of Microbiology and Molecular Genetics at MSU, the Ronald and Sharon Rogowski Fellowship and the MSU College of Natural Science provided student support to SM. A portion of these findings were included in SM dissertation from MSU (69).
Conflict of Interest
The authors declare that the research was conducted in the absence of any commercial or financial relationships that could be construed as a potential conflict of interest.
Acknowledgments
We thank Ben Hutton and Jason Wholehan at the MDHHS and the laboratory staff at each participating hospital for help with specimen processing and culture as well as James Collins and Tiffany Henderson at the MDHHS Bureau of Epidemiology for help with MDSS.
Supplementary Material
The Supplementary Material for this article can be found online at: https://www.frontiersin.org/articles/10.3389/fmed.2019.00250/full#supplementary-material
References
1. Majowicz SE, Musto J, Scallan E, Angulo FJ, Kirk M, O'brien SJ, et al. The global burden of nontyphoidal Salmonella gastroenteritis. Clin Infect Dis. (2010) 50:882–9. doi: 10.1086/650733
2. Global Burden of Diseases Diarrhoeal Diseases Collaborators. Estimates of global, regional, and national morbidity, mortality, and aetiologies of diarrhoeal diseases: a systematic analysis for the Global Burden of Disease Study 2015. Lancet Infect Dis. (2017) 17:909–48. doi: 10.1016/S1473-3099(17)30276-1
3. Crim SM, Iwamoto M, Huang JY, Griffin PM, Gilliss D, Cronquist AB, et al. Incidence and trends of infection with pathogens transmitted commonly through food–Foodborne Diseases Active Surveillance Network, 10 U.S. sites, 2006-2013. Morb Mortal Wkly Rep. (2014) 63:328–32.
4. Gal-Mor O, Boyle EC, Grassl GA. Same species, different diseases: how and why typhoidal and non-typhoidal Salmonella enterica serovars differ. Front Microbiol. (2014) 5:391. doi: 10.3389/fmicb.2014.00391
5. Murray CJ, Vos T, Lozano R, Naghavi M, Flaxman AD, Michaud C, et al. Disability-adjusted life years (DALYs) for 291 diseases and injuries in 21 regions, 1990-2010: a systematic analysis for the Global Burden of Disease Study 2010. Lancet. (2012) 380:2197–223. doi: 10.1016/S0140-6736(12)61689-4
6. Scallan E, Hoekstra RM, Angulo FJ, Tauxe RV, Widdowson MA, Roy SL, et al. Foodborne illness acquired in the United States–major pathogens. Emerg Infect Dis. (2011) 17:7–15. doi: 10.3201/eid1701.P11101
7. Hoffmann S, Maculloch B, Batz M. Economic Burden of Major Foodborne Illnesses Acquired in the United States. United States Department of Agriculture (2015). Available online at: https://www.ers.usda.gov/webdocs/publications/43984/52807_eib140.pdf
8. Galanis E, Lo Fo Wong DM, Patrick ME, Binsztein N, Cieslik A, Chalermchikit T, et al. Web-based surveillance and global Salmonella distribution, 2000-2002. Emerg Infect Dis. (2006) 12:381–8. doi: 10.3201/eid1205.050854
9. Hoelzer K, Switt AIM, Wiedmann M. Animal contact as a source of human non-typhoidal salmonellosis. Vet Res. (2011) 42:34. doi: 10.1186/1297-9716-42-34
10. Mermin J, Hutwagner L, Vugia D, Shallow S, Daily P, Bender J, et al. Reptiles, amphibians, and human Salmonella infection: a population-based, case-control study. Clin Infect Dis. (2004) 38 (Suppl. 3):S253–61. doi: 10.1086/381594
11. Younus M, Wilkins MJ, Davies HD, Rahbar MH, Funk J, Nguyen C, et al. The role of exposures to animals and other risk factors in sporadic, non-typhoidal Salmonella infections in Michigan children. Zoonoses Public Health. (2010) 57:e170–6. doi: 10.1111/j.1863-2378.2010.01324.x
12. Doorduyn Y, Van Den Brandhof WE, Van Duynhoven YT, Wannet WJ, Van Pelt W. Risk factors for Salmonella enteritidis and typhimurium (DT104 and non-DT104) infections in the Netherlands: predominant roles for raw eggs in Enteritidis and sandboxes in Typhimurium infections. Epidemiol Infect. (2006) 134:617–26. doi: 10.1017/S0950268805005406
13. Dore K, Buxton J, Henry B, Pollari F, Middleton D, Fyfe M, et al. Risk factors for Salmonella Typhimurium DT104 and non-DT104 infection: a Canadian multi-provincial case-control study. Epidemiol Infect. (2004) 132:485–93. doi: 10.1017/S0950268803001924
14. Cherry WB, Hanks JB, Thomason BM, Murlin AM, Biddle JW, Croom JM. Salmonellae as an index of pollution of surface waters. Appl Microbiol. (1972) 24:334–40.
15. Baudart J, Lemarchand K, Brisabois A, Lebaron P. Diversity of Salmonella strains isolated from the aquatic environment as determined by serotyping and amplification of the ribosomal DNA spacer regions. Appl Environ Microbiol. (2000) 66:1544–52. doi: 10.1128/AEM.66.4.1544-1552.2000
16. Centers for Disease Control and Prevention (2013). Antibiotic Resistance Threats in the United States, 2013. Available online at: https://www.cdc.gov/drugresistance/threat-report-2013/pdf/ar-threats-2013-508.pdf
17. Sanchez-Vargas FM, Abu-El-Haija MA, Gomez-Duarte OG. Salmonella infections: an update on epidemiology, management, and prevention. Travel Med Infect Dis. (2011) 9:263–77. doi: 10.1016/j.tmaid.2011.11.001
18. Varma JK, Molbak K, Barrett TJ, Beebe JL, Jones TF, Rabatsky-Ehr T, et al. Antimicrobial-resistant nontyphoidal Salmonella is associated with excess bloodstream infections and hospitalizations. J Infect Dis. (2005) 191:554–61. doi: 10.1086/427263
19. Helms M, Ethelberg S, Molbak K, Group DTS. International Salmonella Typhimurium DT104 infections, 1992-2001. Emerg Infect Dis. (2005) 11:859–67. doi: 10.3201/eid1106.041017
20. Yu CY, Chou SJ, Yeh CM, Chao MR, Huang KC, Chang YF, et al. Prevalence and characterization of multidrug-resistant (type ACSSuT) Salmonella enterica serovar Typhimurium strains in isolates from four gosling farms and a hatchery farm. J Clin Microbiol. (2008) 46:522–6. doi: 10.1128/JCM.00709-07
21. Dos Reis EM, Rodrigues Ddos P, De Freitas-Almeida AC, Hofer E. Prevalence of R-type ACSSuT in strains of Salmonella serovar Typhimurium DT193 isolated from human infections in Brazil. Rev Panam Salud Publica. (2011) 29:387–92.
22. Afema JA, Mather AE, Sischo WM. Antimicrobial resistance profiles and diversity in Salmonella from humans and cattle, 2004-2011. Zoonoses Public Health. (2015) 62:506–17. doi: 10.1111/zph.12172
23. Wang X, Biswas S, Paudyal N, Pan H, Li X, Fang W, et al. Antibiotic resistance in Salmonella typhimurium isolates recovered from the food chain through national antimicrobial resistance monitoring system between 1996 and 2016. Front Microbiol. (2019) 10:985. doi: 10.3389/fmicb.2019.00985
24. Association of Public Health Laboratories. Salmonella Serotyping in US Public Health Laboratories. (Silver Spring, MD) (2014). Available online at: https://www.aphl.org/aboutAPHL/publications/Documents/FS_SalmonellaSustainabilityWhitePaper_Nov2014.pdf
25. Singh P, Teal TK, Marsh TL, Tiedje JM, Mosci R, Jernigan K, et al. Intestinal microbial communities associated with acute enteric infections and disease recovery. Microbiome. (2015) 3:45. doi: 10.1186/s40168-015-0109-2
26. Clinical Laboratory Standards Institute. Performance Standards for Antimicrobial Susceptibility Testing; Twenty-Fourth Information Supplement. (2014). Available online at: https://clsi.org/standards/products/microbiology/documents/m100/
27. Ingram DD, Franco SJ. 2013 NCHS urban-rural classification scheme for counties. Vital Health Stat. (2014) 2:1–73.
28. Kofke-Egger H, Udow-Phillips M. Antibiotic Prescribing and Use. University of Michigan, Center for Healthcare Research and Transformation, Ann Arbor, MI (2011). Available online at: https://www.chrt.org/publication/antibiotic-prescribing-use/
29. Centers for Disease Control and Prevention (2018). National Antimicrobial Resistance Monitoring System (NARMS) Now: Human Data. Atlanta, GA. Available online at: https://www.cdc.gov/narmsnow
30. Younus M, Wilkins MJ, Arshad MM, Rahbar MH, Saeed AM. Demographic risk factors and incidence of Salmonella enteritidis infection in Michigan. Foodborne Pathog Dis. (2006) 3:266–73. doi: 10.1089/fpd.2006.3.266
31. Centers for Disease Control and Prevention (2013). National Enteric Disease Surveillance: Salmonella Annual Report, 2011. Atlanta, GA. Available onlne at: https://www.cdc.gov/ncezid/dfwed/PDFs/salmonella-annual-report-2011-508c.pdf
32. Tseng M, Sha Q, Rudrik JT, Collins J, Henderson T, Funk JA, et al. Increasing incidence of non-O157 Shiga toxin-producing Escherichia coli (STEC) in Michigan and association with clinical illness. Epidemiol Infect. (2016) 144:1394–405. doi: 10.1017/S0950268815002836
33. Akil L, Ahmad HA, Reddy RS. Effects of climate change on Salmonella infections. Foodborne Pathog Dis. (2014) 11:974–80. doi: 10.1089/fpd.2014.1802
34. Conrad CC, Stanford K, Narvaez-Bravo C, Callaway T, Mcallister T. Farm fairs and petting zoos: a review of animal contact as a source of zoonotic enteric disease. Foodborne Pathog Dis. (2017) 14:59–73. doi: 10.1089/fpd.2016.2185
35. Judd MC, Hoekstra RM, Mahon BE, Fields PI, Wong KK. Epidemiologic patterns of human Salmonella serotype diversity in the USA, 1996-2016. Epidemiol Infect. (2019) 147:e187. doi: 10.1017/S0950268819000724
36. Thomas JL, Slawson RM, Taylor WD. Salmonella serotype diversity and seasonality in urban and rural streams. J Appl Microbiol. (2013) 114:907–22. doi: 10.1111/jam.12079
37. Guerin MT, Martin SW, Darlington GA, Rajic A. A temporal study of Salmonella serovars in animals in Alberta between 1990 and 2001. Can J Vet Res. (2005) 69:88–99. doi: 10.1007/BF03404039
38. Dailey N, Niemeier D, Elkhoraibi C, Senties-Cue CG, Pitesky M. Descriptive survey and Salmonella surveillance of pastured poultry layer farms in California. Poult Sci. (2017) 96:957–65. doi: 10.3382/ps/pew360
39. Hendriksen SW, Orsel K, Wagenaar JA, Miko A, Van Duijkeren E. Animal-to-human transmission of Salmonella Typhimurium DT104A variant. Emerg Infect Dis. (2004) 10:2225–7. doi: 10.3201/eid1012.040286
40. Aarestrup FM, Hendriksen RS, Lockett J, Gay K, Teates K, Mcdermott PF, et al. International spread of multidrug-resistant Salmonella Schwarzengrund in food products. Emerg Infect Dis. (2007) 13:726–31. doi: 10.3201/eid1305.061489
41. Williamson D, Lane C, Easton M, Valcanis M, Strachan J, Veitch M, et al. Increasing antimicrobial resistance in non-typhoidal Salmonella in Australia, 1979 - 2015 Antimicrob. Agents Chemother. (2017) 62:e02012–17. doi: 10.1128/AAC.02012-17
42. Chopra I, Roberts M. Tetracycline antibiotics: mode of action, applications, molecular biology, and epidemiology of bacterial resistance. Microbiol Mol Biol Rev. (2001) 65:232–60. doi: 10.1128/MMBR.65.2.232-260.2001
43. Food and Drug Administration. Summary Report on Antimicrobials Sold or Distributed for Use in Food-Producing Animals. (2011). Available online at: https://www.fda.gov/media/119332/download
44. Hamscher G, Sczesny S, Hoper H, Nau H. Determination of persistent tetracycline residues in soil fertilized with liquid manure by high-performance liquid chromatography with electrospray ionization tandem mass spectrometry. Anal Chem. (2002) 74:1509–18. doi: 10.1021/ac015588m
45. Levy SB. The Antibiotic Paradox: How Miracle Drugs are Destroying the Miracle. New York, NY: Plenum Press (1992). doi: 10.1007/978-1-4899-6042-9
46. Fein D, Burton G, Tsutakawa R, Blenden D. Matching of antibiotic resistance patterns of Escherichia coli of farm families and their animals. J Infect Dis. (1974) 130:274–9. doi: 10.1093/infdis/130.3.274
47. White DG, Zhao S, Sudler R, Ayers S, Friedman S, Chen S, et al. The isolation of antibiotic-resistant Salmonella from retail ground meats. N Engl J Med. (2001) 345:1147–54. doi: 10.1056/NEJMoa010315
48. Mackie RI, Koike S, Krapac I, Chee-Sanford J, Maxwell S, Aminov RI. Tetracycline residues and tetracycline resistance genes in groundwater impacted by swine production facilities. Anim Biotechnol. (2006) 17:157–76. doi: 10.1080/10495390600956953
49. Xi C, Zhang Y, Marrs CF, Ye W, Simon C, Foxman B, et al. Prevalence of antibiotic resistance in drinking water treatment and distribution systems. Appl Environ Microbiol. (2009) 75:5714–8. doi: 10.1128/AEM.00382-09
50. Soler P, Gonzalez-Sanz R, Bleda MJ, Hernandez G, Echeita A, Usera MA. Antimicrobial resistance in non-typhoidal Salmonella from human sources, Spain, 2001-2003. J Antimicrob Chemother. (2006) 58:310–4. doi: 10.1093/jac/dkl223
51. Voss-Rech D, Potter L, Vaz CS, Pereira DI, Sangioni LA, Vargas AC, et al. Antimicrobial resistance in nontyphoidal Salmonella isolated from human and poultry-related samples in Brazil: 20-year meta-analysis. Foodborne Pathog Dis. (2017) 14:116–24. doi: 10.1089/fpd.2016.2228
52. Zhang CZ, Ren SQ, Chang MX, Chen PX, Ding HZ, Jiang HX. Resistance mechanisms and fitness of Salmonella, Typhimurium and Salmonella Enteritidis mutants evolved under selection with ciprofloxacin in vitro. Sci Rep. (2017) 7:9113. doi: 10.1038/s41598-017-09151-y
53. Roy P, Dhillon AS, Lauerman LH, Schaberg DM, Bandli D, Johnson S. Results of Salmonella isolation from poultry products, poultry, poultry environment, and other characteristics. Avian Dis. (2002) 46:17–24. doi: 10.1637/0005-2086(2002)0460017:ROSIFP2.0.CO;2
54. Berrang ME, Bailey JS, Altekruse SF, Shaw WK Jr, Patel BL, Meinersmann RJ, et al. Prevalence, serotype, and antimicrobial resistance of Salmonella on broiler carcasses postpick and postchill in 20 U.S. processing plants. J Food Prot. (2009) 72:1610–5. doi: 10.4315/0362-028X-72.8.1610
55. Han J, David DE, Deck J, Lynne AM, Kaldhone P, Nayak R, et al. Comparison of Salmonella enterica serovar Heidelberg isolates from human patients with those from animal and food sources. J Clin Microbiol. (2011) 49:1130–3. doi: 10.1128/JCM.01931-10
56. Edrington TS, Schultz CL, Bischoff KM, Callaway TR, Looper ML, Genovese KJ, et al. Antimicrobial resistance and serotype prevalence of Salmonella isolated from dairy cattle in the southwestern United States. Microb Drug Resist. (2004) 10:51–6. doi: 10.1089/107662904323047808
57. M'ikanatha NM., Sandt CH, Localio AR, Tewari D, Rankin SC, et al. Multidrug-resistant Salmonella isolates from retail chicken meat compared with human clinical isolates. Foodborne Pathog Dis. (2010) 7:929–34. doi: 10.1089/fpd.2009.0499
58. Carattoli A. Resistance plasmid families in Enterobacteriaceae. Antimicrob Agents Chemother. (2009) 53:2227–38. doi: 10.1128/AAC.01707-08
59. Dhanani AS, Block G, Dewar K, Forgetta V, Topp E, Beiko RG, et al. Genomic comparison of non-typhoidal Salmonella enterica serovars Typhimurium, Enteritidis, Heidelberg, Hadar and Kentucky isolates from broiler chickens. PLoS ONE. (2015) 10:e0128773. doi: 10.1371/journal.pone.0128773
60. Shah DH, Paul NC, Sischo WC, Crespo R, Guard J. Population dynamics and antimicrobial resistance of the most prevalent poultry-associated Salmonella serotypes. Poult Sci. (2017) 96:687–702. doi: 10.3382/ps/pew342
61. Foley SL, Zhao S, Walker RD. Comparison of molecular typing methods for the differentiation of Salmonella foodborne pathogens. Foodborne Pathog Dis. (2007) 4:253–76. doi: 10.1089/fpd.2007.0085
62. Talsma E, Goettsch WG, Nieste HL, Schrijnemakers PM, Sprenger MJ. Resistance in Campylobacter species: increased resistance to fluoroquinolones and seasonal variation. Clin Infect Dis. (1999) 29:845–8. doi: 10.1086/520447
63. Cha W, Mosci R, Wengert SL, Singh P, Newton DW, Salimnia H, et al. Antimicrobial susceptibility profiles of human Campylobacter jejuni isolates and association with phylogenetic lineages. Front Microbiol. (2016) 7:589. doi: 10.3389/fmicb.2016.00589
64. Mukherjee S, Mosci RE, Anderson CM, Snyder BA, Collins J, Rudrik JT, et al. Antimicrobial drug-resistant Shiga toxin-producing Escherichia coli infections, Michigan, USA. Emerg Infect Dis. (2017) 23:1609–11. doi: 10.3201/eid2309.170523
65. Sun L, Klein EY, Laxminarayan R. Seasonality and temporal correlation between community antibiotic use and resistance in the United States. Clin Infect Dis. (2012) 55:687–94. doi: 10.1093/cid/cis509
66. Suda KJ, Hicks LA, Roberts RM, Hunkler RJ, Taylor TH. Trends and seasonal variation in outpatient antibiotic prescription rates in the United States, 2006 to 2010. Antimicrob Agents Chemother. (2014) 58:2763–6. doi: 10.1128/AAC.02239-13
67. Linden PK, Pasculle AW, Manez R, Kramer DJ, Fung JJ, Pinna AD, et al. Differences in outcomes for patients with bacteremia due to vancomycin-resistant Enterococcus faecium or vancomycin-susceptible E. faecium. Clin Infect Dis. (1996) 22:663–70. doi: 10.1093/clinids/22.4.663
68. Cosgrove S. The Relationship between antimicrobial resistance and patient outcomes: mortality, length of hospital stay, and health care costs. Clin Infect Dis. (2006) 42:S82–S89. doi: 10.1086/499406
Keywords: non-typhoidal Salmonella, antimicrobial resistance, epidemiology, risk factors, Michigan
Citation: Mukherjee S, Anderson CM, Mosci RE, Newton DW, Lephart P, Salimnia H, Khalife W, Rudrik JT and Manning SD (2019) Increasing Frequencies of Antibiotic Resistant Non-typhoidal Salmonella Infections in Michigan and Risk Factors for Disease. Front. Med. 6:250. doi: 10.3389/fmed.2019.00250
Received: 31 July 2019; Accepted: 17 October 2019;
Published: 08 November 2019.
Edited by:
Ilana L. B. C. Camargo, University of São Paulo, BrazilReviewed by:
Angelo Berchieri Junior, São Paulo State University, BrazilRafael Antonio Casarin Penha Filho, São Paulo State University, Brazil
Copyright © 2019 Mukherjee, Anderson, Mosci, Newton, Lephart, Salimnia, Khalife, Rudrik and Manning. This is an open-access article distributed under the terms of the Creative Commons Attribution License (CC BY). The use, distribution or reproduction in other forums is permitted, provided the original author(s) and the copyright owner(s) are credited and that the original publication in this journal is cited, in accordance with accepted academic practice. No use, distribution or reproduction is permitted which does not comply with these terms.
*Correspondence: Shannon D. Manning, mannin71@msu.edu