- 1NIH Undiagnosed Diseases Program, Common Fund, Office of the Director, National Institutes of Health, Bethesda, MD, United States
- 2National Human Genome Research Institute, National Institutes of Health, Bethesda, MD, United States
- 3Department of Molecular and Cellular Pharmacology, University of Miami School of Medicine, Miami, FL, United States
- 4Appistry, Inc., St. Louis, MO, United States
- 5MicroSoft Research, Redmond, WA, United States
- 6William Harvey Research Institute, Barts and The London School of Medicine and Dentistry, Queen Mary University of London, London, United Kingdom
- 7Environmental Genomics and Systems Biology, Lawrence Berkeley National Laboratory, Berkeley, CA, United States
- 8Palmieri Metabolic Disease Laboratory, Children’s Hospital of Philadelphia, Philadelphia, PA, United States
- 9Department of Pathology and Laboratory of Medicine, University of Pennsylvania, Philadelphia, PA, United States
- 10The Jackson Laboratory for Genomic Medicine, Farmington, CT, United States
- 11Department of Medical Informatics and Clinical Epidemiology, Oregon Health & Science University, Portland, OR, United States
Traditionally, the use of genomic information for personalized medical decisions relies on prior discovery and validation of genotype–phenotype associations. This approach constrains care for patients presenting with undescribed problems. The National Institutes of Health (NIH) Undiagnosed Diseases Program (UDP) hypothesized that defining disease as maladaptation to an ecological niche allows delineation of a logical framework to diagnose and evaluate such patients. Herein, we present the philosophical bases, methodologies, and processes implemented by the NIH UDP. The NIH UDP incorporated use of the Human Phenotype Ontology, developed a genomic alignment strategy cognizant of parental genotypes, pursued agnostic biochemical analyses, implemented functional validation, and established virtual villages of global experts. This systematic approach provided a foundation for the diagnostic or non-diagnostic answers provided to patients and serves as a paradigm for scalable translational research.
Introduction
As established in 2008, the purpose of the National Institutes of Health (NIH) Undiagnosed Diseases Program (UDP) is to provide answers to patients with conditions that have eluded diagnosis and to advance medical knowledge about rare and common diseases (1). At its fundamental core, the NIH UDP is, therefore, an implementation of both personalized and genomic medicine.
Personalized medicine, which is the customization of healthcare to the individual patient, conceptually flows from the dawn of medicine. Medical practice has had a long tradition of being inherently “personal” to each patient. Current usage of “personalized medicine” denotes, however, the use of technology to enable a personalization not previously feasible and is generally applied in the context of using genetic information to guide medical care. The use of genetic information in this manner arose from the Human Genome Project and technological advances that apply genomic information to medical practice (2).
Within genomic medicine, DNA sequence variations are mined for predictors of susceptibility and resistance to diseases, as well as for medication safety and efficacy. The former use has proven its utility in the diagnosis of many inherited disorders (3), the management of several cancers, and disease stratification (4). The latter has proven its usefulness for delineating appropriate anticancer therapies, anticoagulant therapy, and cholesterol reduction treatments among others (5, 6).
Genomic and precision medicine decisions generally rely on prior discovery and validation of genotype–phenotype associations across many patients. While this approach can be effective for patients with a previously identified disease correlation, it is inadequate for NIH UDP patients who present with undescribed problems. Herein, we describe the philosophical bases, methodologies, and processes that the NIH UDP developed to provide answers to patients with conditions that have eluded diagnosis and to advance biomedical knowledge about disease mechanisms.
Defining Disease: The Philosophical Basis of the NIH UDP
As implied by the title UDP, definition of a diagnosis is crucial to understanding the Program’s purpose and approach. Given that a diagnosis is a culturally appropriate explanation for a problem (7), then, within Occidental medical culture, a diagnosis is a material and rational explanation testable by the scientific method (8). Within this perspective, diseases arise from malfunctioning biological processes causing harm and are not inclusive of illness caused by loss of mental or social well-being (9, 10). Adhering to this objectivist occidental medical definition, the NIH UDP has generally chosen to exclude diseases with sociocultural etiologies.
Biological or physiological malfunction is the product of gene–environment interactions over time (11). Thus, disease can be considered maladaptation to an ecological niche (12). Such maladaptations are characterized by disturbances of genetic, developmental, and physiological homeostases (12). The NIH UDP has defined genetic homeostasis as the sum of human evolutionary history encoded within DNA sequence, developmental homeostasis as the lifetime response of an organism to an ecological niche, and physiological homeostasis as the biochemical and molecular balance detectable at the moment of inquiry. In this construct, the developmental and physiological homeostatic responses to the environment are constrained by an organism’s genetic composition.
For most of human evolutionary history, natural selection molded humans to be hunter-gatherers. They walked many miles each day and ate a diverse, relatively unprocessed diet (11). Among many adaptations for survival, the development of culture sets humans apart from other organisms and allows them to change their environment to buffer against selective pressure. Through cultural evolution, humans colonize environments and develop lifestyles that they are not primarily adapted to by natural selection. Within the current urban lifestyle, for example, industrialization has exposed humans to novel toxins and processed food and enabled them to avoid most physical activity. Unable to alter millennia of natural selection, the mismatch of human bodies to this modern ecological niche causes most human disease in wealthy societies (11). These mismatch diseases, which include osteoporosis, cardiovascular disease, some cancers, type 2 diabetes, and metabolic syndrome, rarely arise from recent strong single-gene mutations but instead from multiple adaptations selected over the millennia of human existence. This perspective on gene–environment interactions consequently divides disease into rare monogenic or oligogenic disorders and common cultural mismatch disorders.
The NIH UDP has chosen for two reasons to focus its efforts on undescribed diseases likely to have a monogenic or oligogenic etiology. First, many cultural mismatch disorders are diagnosed and have defined etiologies and treatments (11). Second, monogenic or oligogenic disorders are more tractable for causal genetic discovery, and consequently, a material and rational explanation testable by the scientific method, i.e., a molecular diagnosis, is more achievable.
To provide answers for patients judged to have monogenic or oligogenic disorders that have eluded diagnosis, the NIH UDP screened for disturbances of the genetic, developmental, and physiologic homeostases. In addition, the NIH UDP implemented a management and communication system to facilitate collaborations and solutions (5). As represented in Figure 1, the NIH UDP process can be broken into the following steps: (1) patient selection, (2) patient phenotyping, (3) integrated analysis, (4) causal confirmation, and (5) disposition. The methodology and processes developed are described in the following sections.
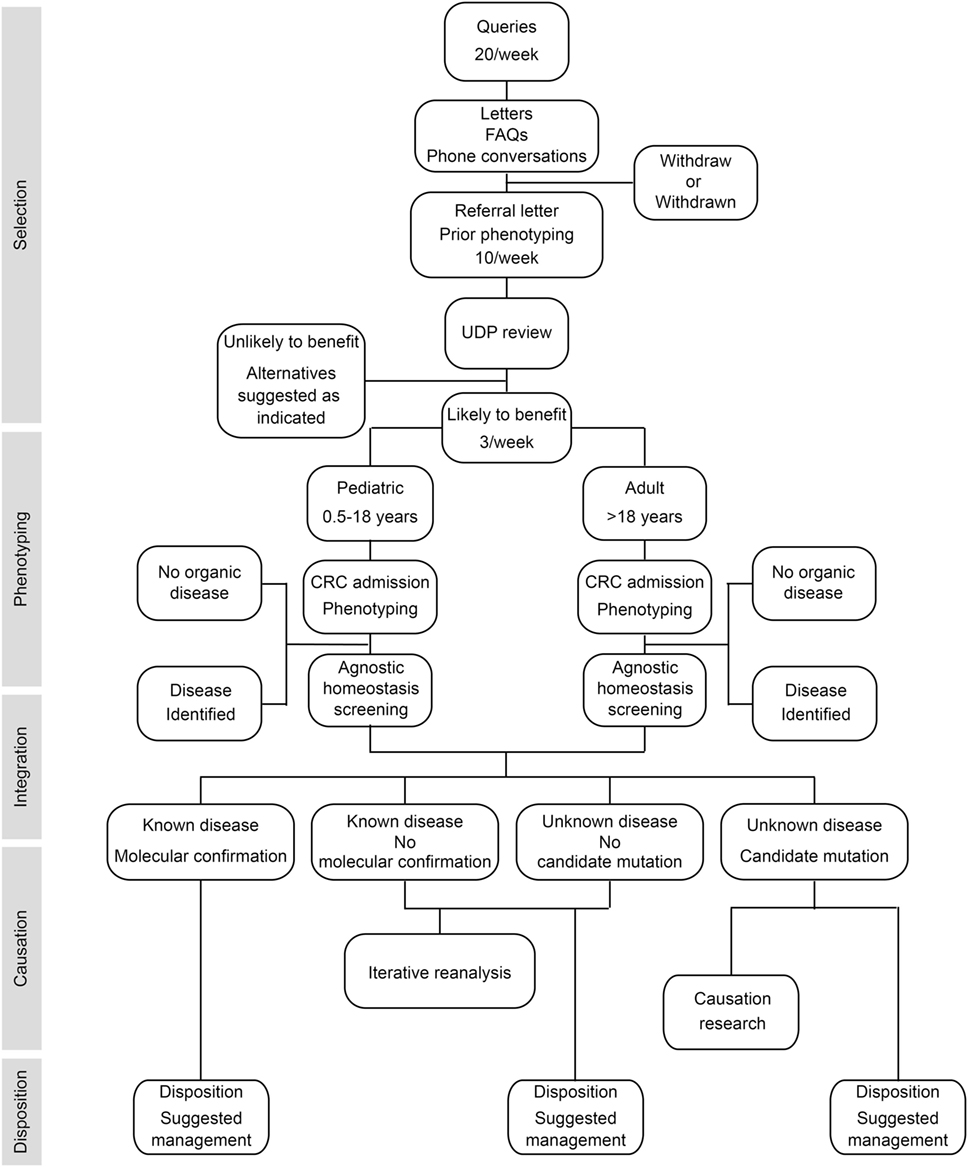
Figure 1. Flow diagram showing the process by which patients are accepted into and evaluated for a diagnosis within the National Institutes of Health (NIH) Undiagnosed Diseases Program (UDP). The process is divided into five major components listed along the left side. The initial component is patient selection. This is followed by patient admission to the NIH clinical research center (CRC) for phenotyping and, when appropriate, agnostic screening for disturbances of evolutionary, developmental, and biochemical homeostases. These data are then integrated computationally and through discussion to determine if there is a known medical diagnosis. Patients with a diagnosis are given disposition recommendations based on that diagnosis. For those without a diagnosis and without a candidate cause, their data are queued for iterative reanalysis, and they and their referring physician are given disposition recommendations based on what was learned. For those without a diagnosis and with a candidate cause, their data are subjected, as resources allow, to research studies to evaluate the potential causality, and they and their referring physician are given disposition recommendations based on what was learned.
Methodologies and Results of the NIH UDP
Patient Selection
Individuals with a broad spectrum of disorders apply to the NIH UDP (1, 13, 14). The experimental paradigm of the NIH UDP is predicated on an identifiable biological dysfunction arising from a monogenic or oligogenic etiology; thus, the ascertainment of the appropriate families is critical for an interpretable outcome and ethical experimentation (15–17). To satisfy these requirements, the NIH UDP selection criteria for admitting individuals to the clinical research center (CRC) included (1) a physician referral providing a clear picture of the patient’s illness and promising follow-up care after the UDP evaluation, (2) records of previous care and evaluations showing elimination of known disorders, (3) medical records and findings supporting a genetic etiology, (4) willingness of family members to participate for segregation of putative genetic causes, and (5) a problem within the expertise of the care available at the NIH CRC. All patients or their guardians and participating family members gave informed consent to clinical protocol 76-HG-0238, which was approved by the NHGRI Institutional Review Board.
Patient Phenotyping
Having selected patients appropriate to the experimental paradigm, the next step for the NIH UDP was delineation of the disease phenotype. Given that disease is the loss of evolutionary, developmental, or physiological homeostasis and that a phenotype is the expression of that loss, characterization of the disease requires a thorough and unbiased assessment of each homeostatic disturbance. To this end, the NIH UDP implemented the following methodologies for assessment of these homeostases.
Assessment of Genetic Homeostasis
Nothing in biology makes sense except in the light of evolution.
Theodosius Dobzhansky
American Biology Teacher (1973) 35:125–129
Classically, genetic characterization has been performed by collecting a family history and carefully examining and testing family members to determine affected and unaffected status. This is usually presented as a pedigree and family history within the medical record. The NIH UDP continues this practice for immediate family members and occasionally for additional generations.
Given that the family and medical history meet criteria supporting a genetic etiology (see supplemental methods), identifying the point in the meiotic history of the family when the disturbance in evolutionary or genetic homeostasis occurred enables the generation of inheritance hypotheses and comparison of the affected individual’s genome to meiotically close reference genomes. Assessing evolutionary homeostasis through genome or exome sequencing, the NIH UDP developed and implemented DiploidAlign, an alignment strategy that imputes information from population and both parental genomes and then aligns the proband’s sequence data to those imputed genomes (see supplemental methods) (18–20).
Assessment of Developmental Homeostasis
Developmental homeostasis and its disturbances reflect the manifestation and evolution of disease during the lifetime of an individual. Classically, this information has been collected through medical history and serial physical examination. Although the temporal manifestation and evolution of disease are predicted to manifest in the transcriptome and epigenome profiles (21), the NIH UDP has not routinely assessed the transcriptome and epigenome because the disease-related changes were thought often specific to minimally accessible affected tissues.
Systematic collection of medical history and physical examination information require the use of a standardized vocabulary. Because traditional clinical vocabularies had been shown to be insufficient (22), the NIH UDP uses the Human Phenotype Ontology (HPO) (23), a standardized vocabulary of phenotypic abnormalities encountered in human disease, and the PhenoTips graphical user interface and search engine (24, 25). This allows comparison to other human disorders and model organisms as well as identification of relationships between human phenotypic abnormalities and cellular and biochemical networks (26).
Assessment of Physiologic Homeostasis
Physiologic or biochemical homeostasis reflects the equilibrium of the body at a moment in time, i.e., the moment at which the fluid or tissue is collected. Measurement of this homeostasis is the sine qua non of clinical pathology laboratories and is usually directed by a differential diagnosis. Given that the individuals presenting within the NIH UDP have, by definition, undescribed disorders, the differential diagnosis is absent to minimal, and thus, screens agnostic to diagnosis were used to detect disturbances of physiological or biochemical homeostasis.
Exemplifying the utility of this agnostic approach, approximately 50% of UDP patients screened for perturbation of protein glycosylation or free glycans in the plasma or urine differed from healthy controls (data not shown). These qualitative and quantitative changes in glycosylation, whether primary or secondary, have diagnostic, mechanistic, or therapeutic value as illustrated by detection of glycosylation abnormalities in DNA repair disorders (27, 28), ciliopathies (29, 30), mitochondriopathies (31, 32), and Golgi disorders (33). In contrast, detailed metabolomics studies uncovered very few anomalies, suggesting that the current medical testing technology already detects most disorders of metabolism prior to referral to the NIH UDP (data not shown). The NIH UDP did not pursue lipidome analysis; however, we hypothesize that, like the glycome analyses, these will define previously undetected primary and secondary changes having diagnostic, mechanistic, or therapeutic value and will be the subject of future investigation.
Integration of Measures of Homeostatic Disturbance
Having characterized these homeostases, the observations were integrated to minimize investigator bias and to generate testable hypotheses for disease causation. To accomplish this, the NIH UDP used the HPO terms to implement bioinformatic tools such as Exomiser1 and PhenIX (34–36). These software programs compare HPO terms to similar phenotypic profiles in humans and model organisms, improving prioritization of candidate disease variants. Illustrating the utility of this approach, reanalysis of UDP patient sequence data with Exomiser identified about 10–20% additional molecular diagnoses compared to those identified by manual curation alone (19).
This strategy also facilitated prioritizing of sequence variants within gene networks seeded by genes giving similar phenotypes when mutated in humans or model organisms and was effective for identifying atypical presentations (18, 37). A tool enabling such analysis is Exome Walker (38), which is incorporated into Exomiser for exome sequence analysis (19). This method prioritized mutations in MED23 and UNC80 as likely causes of neurodevelopmental disorders prior to mutations being reported in other families (19, 39, 40).
Delineation of a Sequence Variant As Causal or Not
In what circumstances can we pass from this observed association to a verdict of causation? Upon what basis should we proceed to do so?
Sir Austin Bradford Hill
Proceedings of the Royal Society of Medicine (1965) 58:295-300
Classically, a genetic cause for a trait is accepted if (1) variants segregate with disease, (2) multiple independent alleles of the gene give the same phenotype, and (3) expression of the wild-type gene rescues the phenotype. Accomplishment of these three in medical genetics is seldom possible. Consequently, medical genetics relies on associations that meet minimum evidence (41–43). In other words, causality in medical genetics is probabilistic and rarely deterministic (44).
Large pedigrees are generally used to acquire statistical evidence for segregation of a genetic locus with disease (43), and cohorts of independent families define independent alleles (45). When a disease occurs in a small family and is unrecognized or undescribed, characterization of segregation and identification of independent alleles is difficult. A proposed redress for both problems is to establish large databases of phenotypes and genotypes and use them to identify other families with the same disease and a shared potential genetic basis. To this end, the NIH UDP participates in the Matchmaker Exchange by depositing data in PhenomeCentral2 (46, 47). Data are also deposited in dbGaP.3 For some cases, we make a minimal amount of phenotypic and genotypic information available publically on the Monarch Initiative website4 to aid patient matching against known diseases and model organisms and to promote collaboration.5
In the absence of identifying another family, two methods can provide causative evidence: (1) amelioration of disease in the patient by pharmacologic targeting of the mutation or (2) recapitulation of the disease in a model system by introducing the precise mutation observed in the human. Exemplifying substantiation of causation through pharmacological targeting, a novel de novo GRIN2A mutation identified in a boy with early-onset epileptic encephalopathy was deemed as causative of his seizures, because the inhibitor identified in vitro for this mutant N-methyl-d-aspartate receptor effectively treated his seizures (48, 49). Illustrating delineation of causality through recapitulation of a disease in a model system, introduction of heterozygous ATP6V1H loss-of-function mutations in zebrafish and mice recapitulated the dominant osteoporosis segregating in the human family (50, 51).
In the absence of the above, the NIH UDP grades sequence variants to reflect the level of support. The first or lowest level is a bioinformatically derived likelihood of the variant being associated with disease. The second or intermediate level adds experimental evidence showing that the mutation alters properties of a gene product with a function consistent with the observed clinical phenotype. The third level adds in vivo studies to show overlap with the human phenotype and failure of the mutant but not of the wild-type human cDNA to rescue. Illustrating this third level of evidence, we used Drosophila model systems to carry out functional screens of 11 candidate genes to establish a causal link between rare genetic variants deemed potentially disease-causing and the nervous system phenotypes of UDP patients (Table 1). In the first phase of the screen, we used the Drosophila GAL4/UAS system to perform RNAi-mediated knockdown of candidate genes ubiquitously and specifically within the nervous system of Drosophila (52). We carefully analyzed effects on survival, behavior, and lifespan. Ubiquitous knockdown of each of the 11 genes resulted in early developmental lethality (Supplementary Table 1), suggesting that these genes are essential for viability. Also, nervous system-specific knockdown of each gene shortened adult life span and caused a degree of reduced developmental survival: mild (DARS, SPRPK3, UBE2V2, MED23, GEMIN5, and NID2), moderate (CHD4 and ATP1A3), or severe (AARS, GARS, and SMC3). Analysis of the neural-motor circuit using negative geotaxis (climbing) behavior (53) detected moderate dysfunction with knockdown of DARS, MED23, and NID2 and more severe impairments with knockdown of UBE2V2 and SPRK3. Furthermore, for all knockdown groups, climbing behavior declined further at 20 days after eclosion, indicating a possible age-dependent impairment of CNS function.
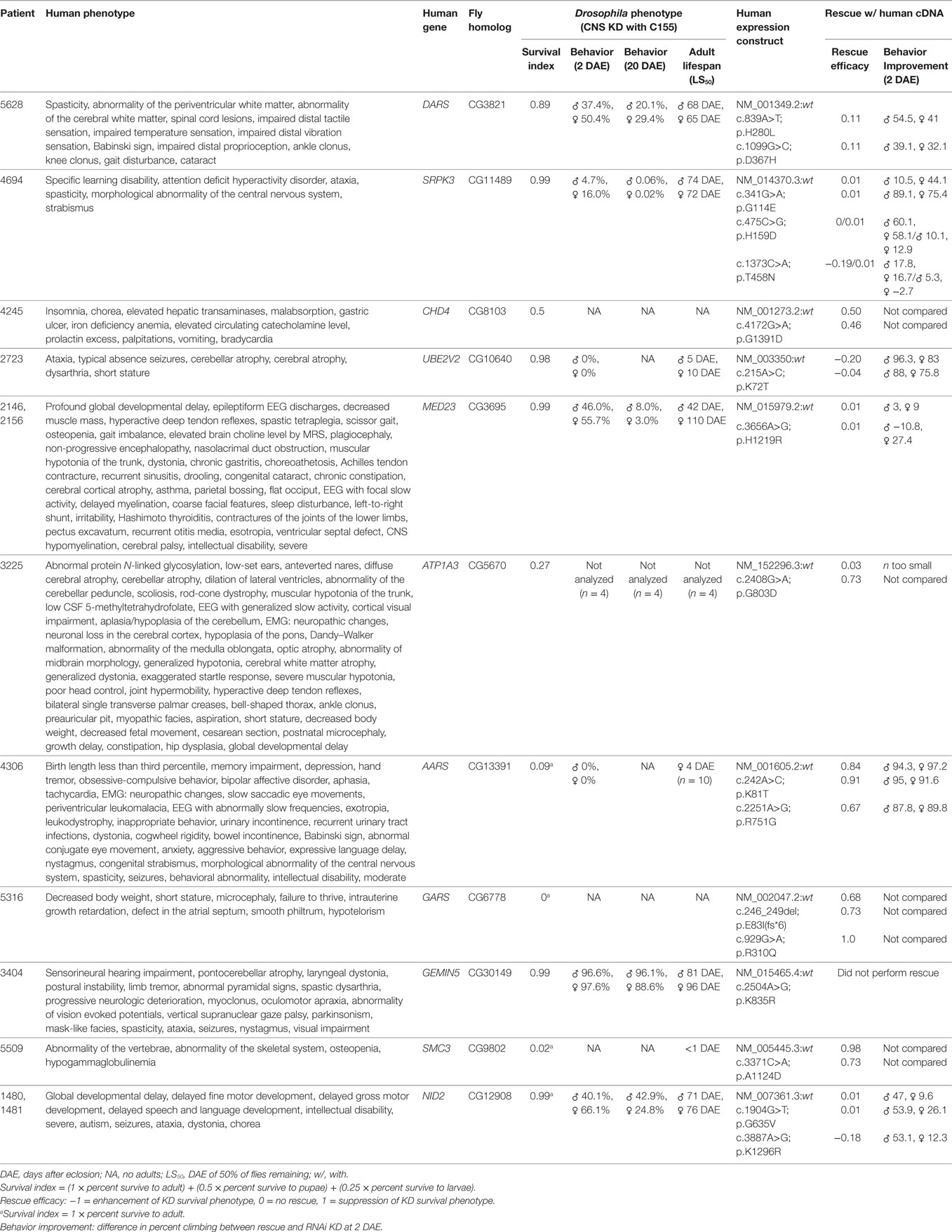
Table 1. Results of Drosophila central nervous system knockdown and rescue for 11 mutations in differing candidate genes.
In the second phase of this screen, we analyzed the consequence of overexpressing the human gene (wild-type or mutant variant) in flies with loss of the Drosophila ortholog. By using data from two independent experiments, we recorded enhancement or suppression of phenotypes associated with the loss of function in Drosophila (Table 1). Of the 11 genes, overexpression of six human wild-type genes (ATP1A3, AARS, GARS, SMC3, NID2, and CHD4) significantly suppressed loss-of-function phenotypes observed with CNS knockdown, suggesting the functional conservation between human and Drosophila orthologs. Comparing the rescue capability of the human wild-type versus the mutant constructs, expression of mutant constructs for three genes (AARS, SMC3, and NID2) had reduced rescue efficacy, expression of mutant constructs for two genes (GARS and ATP1A3) had greater rescue efficacy, and expression of mutant constructs for one gene (CHD4) showed no significant difference.
The reduced rescue efficacy of the human mutant versus wild type supported the pathological causality of the mutation, whereas neither a lack of difference between the mutant and wild type nor increased rescue efficacy of the mutant negated or supported causality. Possible explanations for a lack of difference between the mutant and wild type in the last two classes were that (1) the mutation is not deleterious; (2) the mutation is mildly deleterious and overexpression in Drosophila was sufficient to restore normal function; or (3) the mutation is deleterious, but the phenotype was below detection of the assay.
Patient Disposition
Having completed these evaluations, a patient admitted to the NIH UDP might be diagnosed with a known disorder unrecognized during prior evaluations, an atypical presentation of a known disorder, a combination of several disorders, or a previously unreported disorder. Alternatively, in the absence of proof of causation or association, the patient’s problems might continue to elude explanation and remain undiagnosed. Disposition summaries, which are collaboratively decided on by the clinical and research staff, are communicated to all patients and their referring clinicians by letter and discussed by phone.
Systems Management of the NIH UDP through Scalable Translational Research
The NIH UDP provides translational research for approximately 100–120 families per annum. Typically, individual physician scientists focus on a limited number of diseases; therefore, the NIH UDP defined a need for a scalable means of translational research.
Humans use the distribution of cognitive processes among a group with a variety of skills, e.g., a village, to solve complex problems. The success of these groups or villages requires knowledge of available resources, delineation of social relationships, and effective communication (54, 55). With this as precedent (56), the NIH UDP developed a scalable solution for translational research to coordinate the translational research needs of each family (5).
Definition of a Common Knowledge Base
Performance of translational research requires knowledge and understanding of the problem: what has been tried to address the problem, what has been completed, and what reagents are available. To address these needs, the NIH UDP constructed an integrated system of inventory and data and process management, the Undiagnosed Diseases Program Integrated Collaboration System (UDPICS) (19). This system accumulates an inventory of all biospecimens and associated metadata at the time of collection. In addition, it collects and collates the information generated on each family during their clinical evaluation and research analysis. Finally, each temporal process is similarly documented and linked to antecedent and subsequent processes.
To facilitate coordination and communication, the NIH UDP implemented standard operating procedures, standardization of biospecimens and associated metadata, HPO description of patients (57), and standard genotypic and phenotypic description of model organisms.6 These provided a common foundation for solution generation and for data sharing.
Building of Villages for Scalable Translational Research and Patient-Based Solutions
With delineation of available resources, the next challenge was addressing the patient or family’s problem. Historically, human hunter-gatherer and early agrarian communities formed around shared needs for survival. Modern communities continue to coalesce around shared values although not necessarily principles of survival. Unlike traditional physical villages, many modern communities are virtual and form through the use of Internet and social media tools. For both physical and virtual villages, member identification, communication, and a delineation of responsibilities and relationships are critical for community (58, 59).
In this context, the NIH UDP created virtual communities of geographically distributed experts to enable scalable translational research. Because identification of experts for such communities is traditionally limited by personal awareness, the NIH UDP also facilitated the development of computational tools using disease phenotypes or associated genotypes to identify and rank potential collaborators (60–62). These experts can be then contacted about collaborating on a patient’s problem. Although still a work in progress, UDPICS transformed translational research for the NIH UDP (19).
Discussion
We report for the first time how the NIH UDP definition of disease within the rubric of evolutionary biology, i.e., maladaptation to an evolutionary niche, provided a logical construct for defining a systematic approach to diagnostic testing, interpretation, collaboration, and translational research. In this context, we also tested several theories including the alignment of next-generation sequence reads to deduced parental reference sequences, a systematic multistep approach to defining genetic causality for variants of uncertain significance, and distributed cognition as an efficient scalable model for translational research.
The embodiment of disease within the rubric of evolutionary biology and the delineation of the homeostatic components of adaptation allowed the NIH UDP, upon exhaustion of standard medical approaches, to take a systematic approach to agnostic measure of each of these homeostases. Chromosome microarray and exome sequencing, integrated with the phenome (via HPO) to measure both evolutionary and developmental homeostasis, detected diagnostic mutations in 20–30% of patients (1, 63). These measures, integrated with agnostic analyses of the glycome, seem poised to delineate disease mechanisms and causes in possibly half of the NIH UDP patients.
Postulating that mutations causing undiagnosed disorders are missed because haploblock-specific variants impede sequence alignment, we tested measurement of evolutionary homeostasis by aligning patient sequence to parental- and population-deduced reference sequences. This approach marginally improved alignment and genotyping to Hg37 but did not increased diagnostic rate. Therefore, although this approach is cognizant of the diploid nature of the human genome and haploblock-specific variants, it does not appear to improve detection of causal mutations sufficiently to justify the increased computational costs. In contrast, based on the preliminary studies of others, de novo assembly of long reads might enable detection of causal mutations undetected by short read next-generation sequencing (64).
Delineation of causative variants for traits investigated within individualized precision medicine remains problematic (65–69). The NIH UDP experience and the conclusions of others reiterate prior tenets of medical genetics regarding definition of causality. Specifically, the NIH UDP has observed that, for a single individual, defining a variant in a gene not previously associated with a trait as causative of that trait is not scientifically defensible. As stated by MacArthur et al. “strong evidence that a variant is deleterious (in an evolutionary sense) and/or damaging (to gene function) is not sufficient to implicate a variant as playing a causal role in disease” (65). In contrast, delineation of causality for a novel variant in a gene previously associated with a trait is possible as we demonstrated herein using pharmacologic suppression and Drosophila melanogaster as a model system.
Identifying pathogenicity for the many different novel variants identified in disease-associated genes requires collaboration to leverage global expertise. The underlying principles for this are those of distributed cognition (54, 55) enabled through various Internet and social media tools (19). By this means, the NIH UDP was able to systematically and methodically assemble virtual villages of collaborators to provide translational research appropriate to each problem and to provide medically and economically efficient translational research.
We conclude that the NIH UDP experience of systematically and methodically integrating concepts from multiple disciplines provides a guide for individualized or personalized medical practices. These principles are currently being refined and extended through the Undiagnosed Diseases Network launched by the NIH in September 2015 (63) and through the Undiagnosed Diseases Network International (70).
Ethics Statement
All patients or their guardians and participating family members gave informed consent to clinical protocol 76-HG-0238, which was approved by the NHGRI Institutional Review Board.
Author Contributions
WG, DA, TM, RZ, and CFB conceived the methodology. WG, DA, TM, JD, RG, CG, DD, GG, MN, CW, LW, CJT, and CT participated in patient selection and phenotyping. MAH, PR, D Smedley, and NW developed the HPO annotation guidelines, curated the patients’ phenotype data, and helped with the exome analysis. EV, TG, CA, WB, AB, LC, EF, JG, JE, PP, D Simeonov, EL, AL, D Smedley, MS, KX, DA, and TM participated in patient genotyping, the development of DiploidAlign, or implementation of DiploidAlign. CB, JB, MD, ZD-P, YH, MK, CL, VM, MM, FM, JP, KS, YZ, and RZ performed cell biology, biochemical, and model organism experiments to delineate causality. RF, YH, and VM participated in patient sample extraction, archiving, and distribution. EV, CA, WB, DD, EF, JG, EL, AL, VM, KS, MS, and ZV developed or implemented and tested software infrastructure. EV, CFB, CB, and RZ wrote the manuscript. All authors read and approved the final manuscript.
Conflict of Interest Statement
The authors declare that the research was conducted in the absence of any commercial or financial relationships that could be construed as a potential conflict of interest.
Acknowledgments
We thank the National Human Genome Research Institute Information Technology staff for their untiring collaboration and advice. We also thank Peter Chines of NHGRI for providing SNP probe mapping assistance and software. We thank Sean Leighton, Heath Moylan, and Jamie Osman for support in operationalizing DiploidAlign. This work was supported in part by the National Human Genome Research Institute (HG000215-07) and by the Common Fund, Office of the Director and the Intramural Research Program of the National Human Genome Research Institute (NIH, Bethesda, MD, USA). The Drosophila project is supported by 1R21GM119018, and by NIH UDP: HHSN268201300038C, HHSN268201400033C, HHSN268201600043P. The Monarch Initiative is supported by an NIH Office of the Director Grant #5R24OD011883, as well as by NIH UDP: HHSN268201300036C, HHSN268201400093P.
Supplementary Material
The Supplementary Material for this article can be found online at: http://journal.frontiersin.org/article/10.3389/fmed.2017.00062/full#supplementary-material.
Footnotes
References
1. Gahl WA, Markello TC, Toro C, Fajardo KF, Sincan M, Gill F, et al. The National Institutes of Health undiagnosed diseases program: insights into rare diseases. Genet Med (2012) 14:51–9. doi: 10.1038/gim.0b013e318232a005
2. Collins FS, Varmus H. A new initiative on precision medicine. N Engl J Med (2015) 372:793–5. doi:10.1056/NEJMp1500523
3. Posey JE, Harel T, Liu P, Rosenfeld JA, James RA, Coban Akdemir ZH, et al. Resolution of disease phenotypes resulting from multilocus genomic variation. N Engl J Med (2017) 376:21–31. doi:10.1056/NEJMoa1516767
4. Chakravarthi BV, Nepal S, Varambally S. Genomic and epigenomic alterations in cancer. Am J Pathol (2016) 186:1724–35. doi:10.1016/j.ajpath.2016.02.023
5. Caudle KE, Klein TE, Hoffman JM, Muller DJ, Whirl-Carrillo M, Gong L, et al. Incorporation of pharmacogenomics into routine clinical practice: the clinical pharmacogenetics implementation consortium (CPIC) guideline development process. Curr Drug Metab (2014) 15:209–17. doi:10.2174/1389200215666140130124910
6. Kapoor R, Tan-Koi WC, Teo YY. Role of pharmacogenetics in public health and clinical health care: a SWOT analysis. Eur J Hum Genet (2016) 24(12):1651–7. doi:10.1038/ejhg.2016.114
7. Hanson M. Speaking of Epidemics in Chinese Medicine: Disease and the Geographic Imagination in Late Imperial China. Abingdon-on-Thames: Routledge (2013).
8. Duan X, Markello T, Adams D, Toro C, Tifft C, Gahl WA, et al. Cultural differences define diagnosis and genomic medicine practice: implications for undiagnosed diseases program in China. Front Med (2013) 7:389–94. doi:10.1007/s11684-013-0281-3
9. Murphy D. Concepts of disease and health. In: Zalta EN, editor. The Stanford Encyclopedia of Philosophy. Stanford: The Metaphysics Research Lab, Center for the Study of Language and Information, Stanford University (2008). Available from: https://plato.stanford.edu/entries/health-disease/
11. Lieberman D. The Story of the Human Body: Evolution, Health, and Disease. New York: Pantheon (2013).
12. Child B. Genetic Medicine: A Logic of Disease. Baltimore: Johns Hopkins University Press (2003).
13. Adams DR, Sincan M, Fuentes Fajardo K, Mullikin JC, Pierson TM, Toro C, et al. Analysis of DNA sequence variants detected by high-throughput sequencing. Hum Mutat (2012) 33:599–608. doi:10.1002/humu.22035
14. Gahl WA, Tifft CJ. The NIH undiagnosed diseases program: lessons learned. JAMA (2011) 305:1904–5. doi:10.1001/jama.2011.613
15. Department of Health E, and Welfare. The Belmont Report: Ethical Principles and Guidelines for the Protection of Human Subjects of Research, Report of the National Commission for the Protection of Human Subjects of Biomedical and Behavioral Research. Washington, DC: OPRR Reports (1979).
16. Emanuel EJ, Wendler D, Grady C. What makes clinical research ethical? JAMA (2000) 283:2701–11. doi:10.1001/jama.283.20.2701
17. Tribunals NM. Trials of War Criminals before the Nuremberg Military Tribunals under Control Council Law No. 10 (Vol. 2). Washington, DC: U.S. Government Printing Office (1949).
18. Albert JS, Bhattacharyya N, Wolfe LA, Bone WP, Maduro V, Accardi J, et al. Impaired osteoblast and osteoclast function characterize the osteoporosis of Snyder-Robinson syndrome. Orphanet J Rare Dis (2015) 10:27. doi:10.1186/s13023-015-0235-8
19. Links AE, Draper D, Lee E, Guzman J, Valivullah Z, Maduro V, et al. Distributed cognition and process management enabling individualized translational research: the NIH undiagnosed diseases program experience. Front Med (2016) 3:39. doi:10.3389/fmed.2016.00039
20. Bone WP, Washington NL, Buske OJ, Adams DR, Davis J, Draper D, et al. Computational evaluation of exome sequence data using human and model organism phenotypes improves diagnostic efficiency. Genet Med (2016) 18:608–17. doi:10.1038/gim.2015.137
21. Gibson G. Wellness and health omics linked to the environment: the WHOLE approach to personalized medicine. Adv Exp Med Biol (2014) 799:1–14. doi:10.1007/978-1-4614-8778-4_1
22. Robinson PN. Deep phenotyping for precision medicine. Hum Mutat (2012) 33:777–80. doi:10.1002/humu.22080
23. Köhler S, Vasilevsky NA, Engelstad M, Foster E, McMurry J, Aymé S, et al. The human phenotype ontology in 2017. Nucleic Acids Res (2017) 45:D865–76. doi:10.1093/nar/gkw1039
24. Girdea M, Dumitriu S, Fiume M, Bowdin S, Boycott KM, Chénier S, et al. PhenoTips: patient phenotyping software for clinical and research use. Hum Mutat (2013) 34:1057–65. doi:10.1002/humu.22347
25. Köhler S, Doelken SC, Mungall CJ, Bauer S, Firth HV, Bailleul-Forestier I, et al. The human phenotype ontology project: linking molecular biology and disease through phenotype data. Nucleic Acids Res (2014) 42:D966–74. doi:10.1093/nar/gkt1026
26. Mungall CJ, McMurry JA, Köhler S, Balhoff JP, Borromeo C, Brush M, et al. The monarch initiative: an integrative data and analytic platform connecting phenotypes to genotypes across species. Nucleic Acids Res (2017) 45:D712–22. doi:10.1093/nar/gkw1128
27. Vanhooren V, Dewaele S, Libert C, Engelborghs S, De Deyn PP, Toussaint O, et al. Serum N-glycan profile shift during human ageing. Exp Gerontol (2010) 45:738–43. doi:10.1016/j.exger.2010.08.009
28. Shehata L, Simeonov DR, Raams A, Wolfe L, Vanderver A, Li X, et al. ERCC6 dysfunction presenting as progressive neurological decline with brain hypomyelination. Am J Med Genet A (2014) 164A(11):2892–900. doi:10.1002/ajmg.a.36709
29. Boskovski MT, Yuan S, Pedersen NB, Goth CK, Makova S, Clausen H, et al. The heterotaxy gene GALNT11 glycosylates Notch to orchestrate cilia type and laterality. Nature (2013) 504:456–9. doi:10.1038/nature12723
30. Kane MS, Davids M, Bond M, Adams CJ, Grout ME, Phelps IG, et al. Association of abnormal glycosylation with Joubert syndrome type 10. Cilia (2016) 6:2. doi:10.1186/s13630-017-0048-6
31. Burnham-Marusich AR, Berninsone PM. Multiple proteins with essential mitochondrial functions have glycosylated isoforms. Mitochondrion (2012) 12:423–7. doi:10.1016/j.mito.2012.04.004
32. Spiro RG, Spiro MJ, Bhoyroo VD. Studies on the regulation of the biosynthesis of glucose-containing oligosaccharide-lipids. Effect of energy deprivation. J Biol Chem (1983) 258:9469–76.
33. Davids M, Kane MS, He M, Wolfe LA, Li X, Raihan MA, et al. Disruption of Golgi morphology and altered protein glycosylation in PLA2G6-associated neurodegeneration. J Med Genet (2016) 53:180–9. doi:10.1136/jmedgenet-2015-103338
34. Washington NL, Haendel MA, Mungall CJ, Ashburner M, Westerfield M, Lewis SE. Linking human diseases to animal models using ontology-based phenotype annotation. PLoS Biol (2009) 7:e1000247. doi:10.1371/journal.pbio.1000247
35. Robinson PN, Köhler S, Oellrich A; Sanger Mouse Genetics Project, Wang K, Mungall CJ, et al. Improved exome prioritization of disease genes through cross-species phenotype comparison. Genome Res (2014) 24:340–8. doi:10.1101/gr.160325.113
36. Zemojtel T, Köhler S, Mackenroth L, Jäger M, Hecht J, Krawitz P, et al. Effective diagnosis of genetic disease by computational phenotype analysis of the disease-associated genome. Sci Transl Med (2014) 6:252ra123. doi:10.1126/scitranslmed.3009262
37. Markello T, Chen D, Kwan JY, Horkayne-Szakaly I, Morrison A, Simakova O, et al. York platelet syndrome is a CRAC channelopathy due to gain-of-function mutations in STIM1. Mol Genet Metab (2015) 114:474–82. doi:10.1016/j.ymgme.2014.12.307
38. Kohler S, Bauer S, Horn D, Robinson PN. Walking the interactome for prioritization of candidate disease genes. Am J Hum Genet (2008) 82:949–58. doi:10.1016/j.ajhg.2008.02.013
39. Trehan A, Brady JM, Maduro V, Bone WP, Huang Y, Golas GA, et al. MED23-associated intellectual disability in a non-consanguineous family. Am J Med Genet A (2015) 167:1374–80. doi:10.1002/ajmg.a.37047
40. Valkanas E, Schaffer K, Dunham C, Maduro V, du Souich C, Rupps R, et al. Phenotypic evolution of UNC80 loss of function. Am J Med Genet A (2016) 170(12):3106–14. doi:10.1002/ajmg.a.37929
41. NCI-NHGRI Working Group on Replication in Association Studies, Chanock SJ, Manolio T, Boehnke M, Boerwinkle E, Hunter DJ, et al. Replicating genotype-phenotype associations. Nature (2007) 447:655–60. doi:10.1038/447655a
42. Page GP, George V, Go RC, Page PZ, Allison DB. “Are we there yet?” Deciding when one has demonstrated specific genetic causation in complex diseases and quantitative traits. Am J Hum Genet (2003) 73:711–9. doi:10.1086/378900
44. Marian AJ. Causality in genetics: the gradient of genetic effects and back to Koch postulates of causality. Circ Res (2014) 114:e18–21. doi:10.1161/CIRCRESAHA.114.302904
45. St Hilaire C, Ziegler SG, Markello TC, Brusco A, Groden C, Gill F, et al. NT5E mutations and arterial calcifications. N Engl J Med (2011) 364:432–42. doi:10.1056/NEJMoa0912923
46. Philippakis AA, Azzariti DR, Beltran S, Brookes AJ, Brownstein CA, Brudno M, et al. The matchmaker exchange: a platform for rare disease gene discovery. Hum Mutat (2015) 36:915–21. doi:10.1002/humu.22858
47. Buske OJ, Girdea M, Dumitriu S, Gallinger B, Hartley T, Trang H, et al. PhenomeCentral: a portal for phenotypic and genotypic matchmaking of patients with rare genetic diseases. Hum Mutat (2015) 36:931–40. doi:10.1002/humu.22851
48. Yuan H, Hansen KB, Zhang J, Pierson TM, Markello TC, Fajardo KV, et al. Functional analysis of a de novo GRIN2A missense mutation associated with early-onset epileptic encephalopathy. Nat Commun (2014) 5:3251. doi:10.1038/ncomms4251
49. Pierson TM, Yuan H, Marsh ED, Fuentes-Fajardo K, Adams DR, Markello T, et al. GRIN2A mutation and early-onset epileptic encephalopathy: personalized therapy with memantine. Ann Clin Transl Neurol (2014) 1(3):190–8. doi:10.1002/acn3.39
50. Duan X, Liu J, Zheng X, Wang Z, Zhang Y, Hao Y, et al. Deficiency of ATP6V1H causes bone loss by inhibiting bone resorption and bone formation through the TGF-beta1 pathway. Theranostics (2016) 6:2183–95. doi:10.7150/thno.17140
51. Zhang Y, Huang H, Zhao G, Yokoyama T, Vega H, Huang Y, et al. ATP6V1H deficiency impairs bone development through activation of MMP9 and MMP13. PLoS Genet (2017) 13(2):e1006481. doi:10.1371/journal.pgen.1006481
52. Phelps CB, Brand AH. Ectopic gene expression in Drosophila using GAL4 system. Methods (1998) 14:367–79. doi:10.1006/meth.1998.0592
53. Hotta Y, Benzer S. Genetic dissection of the Drosophila nervous system by means of mosaics. Proc Natl Acad Sci U S A (1970) 67:1156–63. doi:10.1073/pnas.67.3.1156
54. Hollan J, Hutchins E, Kirsh D. Distributed cognition: toward a new foundation for human-computer interaction research. ACM Trans on Comput Hum Interact (2000) 7:174–96. doi:10.1145/353485.353487
56. Diviacco P, Fox P, Pshenichny C, Leadbetter A. Collaborative Knowledge in Scientific Research Networks. Hershey, PA: IGI Global (2014).
57. Washington NL, Mungall CJ, Gibson M, Balhoff JP, Day-Richter J, Lewis SE. Phenote: A Biological Data Annotation Editor Using Ontologies. The 2nd International Biocuration Meeting. San Jose, CA (2007).
58. Larsen ON, Hill RJ. Social structure and interpersonal communication. Am J Sociol (1958) 63:497–505. doi:10.1086/222300
59. La Fond T, Roberts D, Neville J, Tyler J, Connaughton S. The impact of communication structure and interpersonal dependencies on distributed teams. Paper Presented at: 2012 ASE/IEEE International Conference on Privacy, Security, Risk and Trust (PASSAT) and 2012 International Conference on Social Computing (SocialCom). Amsterdam, Netherlands (2012).
60. Institute NHGR. Executive summary. Paper Presented at: NHGRI Genomic Medicine IX: NHGRI’s Genomic Medicine Portfolio – Bedside to Bench. Silver Spring, MD (2016).
61. Haendel M. Translating Human to Models and Back Again: Phenotype Ontologies for Data Integration and Discovery. NHGRI Genomic Medicine IX: NHGRI’s Genomic Medicine Portfolio – Bedside to Bench. Silver Spring, MD: National Human Genome Research Institute (NHGRI) (2016).
62. Haendel M. Envisioning a world where everyone helps solve disease. 8th International SWAT4LS Conference: Semantic Web Applications and Tools for Life Sciences. Cambridge, UK: SWAT4LS (2015).
63. Gahl WA, Mulvihill JJ, Toro C, Markello TC, Wise AL, Ramoni RB, et al. The NIH undiagnosed diseases program and network: applications to modern medicine. Mol Genet Metab (2016) 117:393–400. doi:10.1016/j.ymgme.2016.01.007
64. Brandler WM, Antaki D, Gujral M, Noor A, Rosanio G, Chapman TR, et al. Frequency and complexity of de novo structural mutation in autism. Am J Hum Genet (2016) 98:667–79. doi:10.1016/j.ajhg.2016.02.018
65. MacArthur DG, Manolio TA, Dimmock DP, Rehm HL, Shendure J, Abecasis GR, et al. Guidelines for investigating causality of sequence variants in human disease. Nature (2014) 508:469–76. doi:10.1038/nature13127
66. Yong E. Clinical genetics has a big problem that’s affecting people’s lives. Atlantic (2015). Available from: https://www.theatlantic.com/science/archive/2015/12/why-human-genetics-research-is-full-of-costly-mistakes/420693/ (accessed December 16, 2015).
67. Manrai AK, Funke BH, Rehm HL, Olesen MS, Maron BA, Szolovits P, et al. Genetic misdiagnoses and the potential for health disparities. N Engl J Med (2016) 375:655–65. doi:10.1056/NEJMsa1507092
68. Bell CJ, Dinwiddie DL, Miller NA, Hateley SL, Ganusova EE, Mudge J, et al. Carrier testing for severe childhood recessive diseases by next-generation sequencing. Sci Transl Med (2011) 3:65ra4. doi:10.1126/scitranslmed.3001756
69. Rehm HL, Berg JS, Brooks LD, Bustamante CD, Evans JP, Landrum MJ, et al. ClinGen – the clinical genome resource. N Engl J Med (2015) 372:2235–42. doi:10.1056/NEJMsr1406261
Keywords: rare disease, human phenotype ontology, distributed cognition, diploid alignment, glycome
Citation: Gall T, Valkanas E, Bello C, Markello T, Adams C, Bone WP, Brandt AJ, Brazill JM, Carmichael L, Davids M, Davis J, Diaz-Perez Z, Draper D, Elson J, Flynn ED, Godfrey R, Groden C, Hsieh C-K, Fischer R, Golas GA, Guzman J, Huang Y, Kane MS, Lee E, Li C, Links AE, Maduro V, Malicdan MCV, Malik FS, Nehrebecky M, Park J, Pemberton P, Schaffer K, Simeonov D, Sincan M, Smedley D, Valivullah Z, Wahl C, Washington N, Wolfe LA, Xu K, Zhu Y, Gahl WA, Tifft CJ, Toro C, Adams DR, He M, Robinson PN, Haendel MA, Zhai RG and Boerkoel CF (2017) Defining Disease, Diagnosis, and Translational Medicine within a Homeostatic Perturbation Paradigm: The National Institutes of Health Undiagnosed Diseases Program Experience. Front. Med. 4:62. doi: 10.3389/fmed.2017.00062
Received: 03 March 2017; Accepted: 03 May 2017;
Published: 26 May 2017
Edited by:
Weien Yuan, Shanghai Jiao Tong University, ChinaReviewed by:
Wei Li, Marshall University, United StatesWilliam Keith Gray, Northumbria Healthcare NHS Foundation Trust, United Kingdom
Copyright: © 2017 Gall, Valkanas, Bello, Markello, Adams, Bone, Brandt, Brazill, Carmichael, Davids, Davis, Diaz-Perez, Draper, Elson, Flynn, Godfrey, Groden, Hsieh, Fischer, Golas, Guzman, Huang, Kane, Lee, Li, Links, Maduro, Malicdan, Malik, Nehrebecky, Park, Pemberton, Schaffer, Simeonov, Sincan, Smedley, Valivullah, Wahl, Washington, Wolfe, Xu, Zhu, Gahl, Tifft, Toro, Adams, He, Robinson, Haendel, Zhai and Boerkoel. This is an open-access article distributed under the terms of the Creative Commons Attribution License (CC BY). The use, distribution or reproduction in other forums is permitted, provided the original author(s) or licensor are credited and that the original publication in this journal is cited, in accordance with accepted academic practice. No use, distribution or reproduction is permitted which does not comply with these terms.
*Correspondence: David R. Adams, ZGFkYW1zMSYjeDAwMDQwO21haWwubmloLmdvdg==
†These authors have contributed equally to this work.