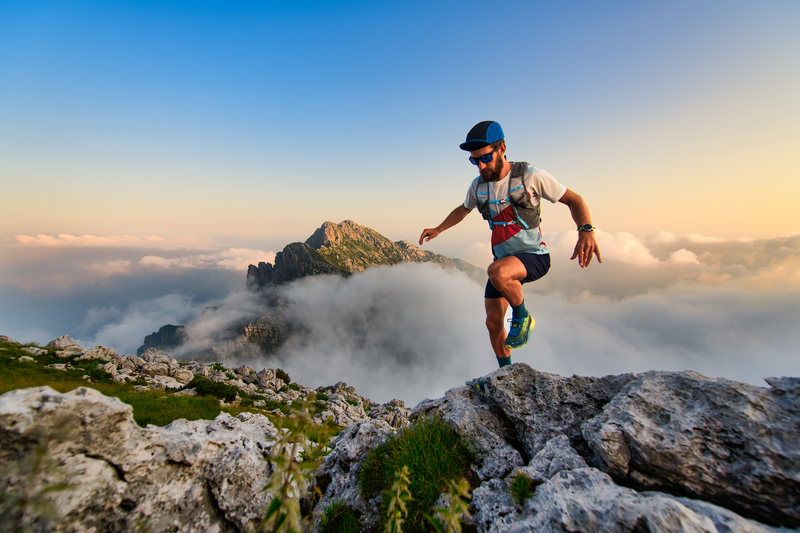
94% of researchers rate our articles as excellent or good
Learn more about the work of our research integrity team to safeguard the quality of each article we publish.
Find out more
REVIEW article
Front. Med. Technol.
Sec. Nano-Based Drug Delivery
Volume 6 - 2024 | doi: 10.3389/fmedt.2024.1434799
This article is part of the Research Topic Microbial Therapeutics: Harnessing the Human Microbiome for Disease Treatment and Prevention View all 3 articles
The final, formatted version of the article will be published soon.
You have multiple emails registered with Frontiers:
Please enter your email address:
If you already have an account, please login
You don't have a Frontiers account ? You can register here
The microbiome of the gut is a complex ecosystem that contains a wide variety of microbial species and functional capabilities. The microbiome has a significant impact on health and disease by affecting endocrinology, physiology, and neurology. It can change the progression of certain diseases and enhance treatment responses and tolerance. The gut microbiota plays a pivotal role in human health, influencing a wide range of physiological processes. Recent advances in computational tools and artificial intelligence (AI) have revolutionized the study of gut microbiota, enabling the identification of biomarkers that are critical for diagnosing and treating various diseases. This review hunts through the cutting-edge computational methodologies that integrate multi-omics data-such as metagenomics, metaproteomics, and metabolomics-providing a comprehensive understanding of the gut microbiome's composition and function. Additionally, machine learning (ML) approaches, including deep learning and network-based methods, are explored for their ability to uncover complex patterns within microbiome data, offering unprecedented insights into microbial interactions and their link to host health. By highlighting the synergy between traditional bioinformatics tools and advanced AI techniques, this review underscores the potential of these approaches in enhancing biomarker discovery and developing personalized therapeutic strategies. The convergence of computational advancements and microbiome research marks a significant step forward in precision medicine, paving the way for novel diagnostics and treatments tailored to individual microbiome profiles. Investigators have the ability to discover connections between the composition of microorganisms, the expression of genes, and the profiles of metabolites.Individual reactions to medicines that target gut microbes can be predicted by models driven by artificial intelligence. It is possible to obtain personalized and precision medicine by first gaining an understanding of the impact that the gut microbiota has on the development of disease. The application of machine learning allows for the customization of treatments to the specific microbial environment of an individual.
Keywords: gut microbiome, Gut Microbiota, Artificial intelligence (AI), Machine Learning (ML), Network-based methods
Received: 23 Jul 2024; Accepted: 16 Oct 2024.
Copyright: © 2024 Dakal, Xu and Kumar. This is an open-access article distributed under the terms of the Creative Commons Attribution License (CC BY). The use, distribution or reproduction in other forums is permitted, provided the original author(s) or licensor are credited and that the original publication in this journal is cited, in accordance with accepted academic practice. No use, distribution or reproduction is permitted which does not comply with these terms.
* Correspondence:
Tikam Chand Dakal, Department of Biotechnology, Faculty of Science, Mohanlal Sukhadia University, Udaipur, India
Disclaimer: All claims expressed in this article are solely those of the authors and do not necessarily represent those of their affiliated organizations, or those of the publisher, the editors and the reviewers. Any product that may be evaluated in this article or claim that may be made by its manufacturer is not guaranteed or endorsed by the publisher.
Research integrity at Frontiers
Learn more about the work of our research integrity team to safeguard the quality of each article we publish.