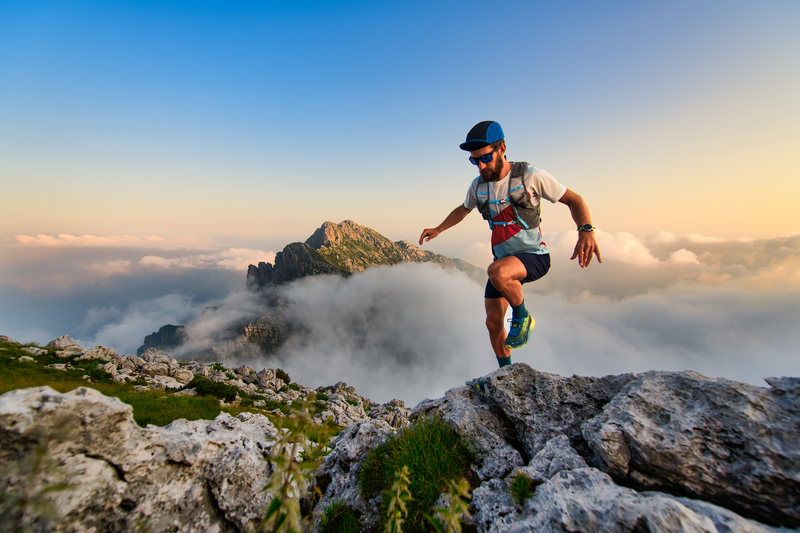
95% of researchers rate our articles as excellent or good
Learn more about the work of our research integrity team to safeguard the quality of each article we publish.
Find out more
PERSPECTIVE article
Front. Med. Technol. , 27 April 2023
Sec. Regenerative Technologies
Volume 5 - 2023 | https://doi.org/10.3389/fmedt.2023.1154653
Any Regenerative Medicine (RM) business requires reliably predictable cell and tissue products. Regulatory agencies expect control and documentation. However, laboratory tissue production is currently not predictable or well-controlled. Before conditions can be controlled to meet the needs of cells and tissues in culture for RM, we have to know what those needs are and be able to quantify them. Therefore, identification and measurement of critical cell quality attributes at a cellular or pericellular level is essential to generating reproducible cell and tissue products. Here, we identify some of the critical cell and process parameters for cell and tissue products as well as technologies available for sensing them. We also discuss available and needed technologies for monitoring both 2D and 3D cultures to manufacture reliable cell and tissue products for clinical and non-clinical use. As any industry matures, it improves and standardizes the quality of its products. Cytocentric measurement of cell and tissue quality attributes are needed for RM.
We previously published the cytocentric principles for regeneration of cell and tissue products (1) which outlines the basic needs of cells in culture. Cells need protection from contamination, physiologic simulation, and full-time conditions for cultures that are optimal, individualized, and dynamic. Growing cells into tissues requires sensing those needs, then meeting them at all times during production.
In forming a cultured tissue, cells have a tough job. They must overcome all the stresses of a violent primary isolation process to expand in number, connect with other cells, heal, and function as a tissue again. It is our challenge to provide them all they need to recover, grow, and form the most reliable and functional tissue product.
Given the diversity in structure and function of mature organs, there is no such thing as a typical RM tissue production process. However, whether it is a decellularization/recellularization process to generate intervertebral discs (2) or a 3D-printed scaffold seeded with iPSC-derived retinal progenitor cells (3), most in vitro tissue-forming processing has similar basic stages as shown in Figure 1. Cells are isolated from tissue, expanded in vitro, and there may or may not be manipulation such as derivation of induced pluripotent stem cells or the correction of a genetic problem. Cells are evaluated for the desired phenotype based on cell markers after this manipulation, and go into in a master cell bank (MCB) for an allogeneic treatment or patient-specific cryogenic storage. The cells are then loaded into a construct and further matured in 3D. The construct may undergo further manipulation to form the replacement organ, like folding into higher-order 4D microstructures (4) or assembly into larger organ structures.
Figure 1. Types of measurements for regenerative medicine tissue production quality attributes. Processes for RM tissue production are as diverse as the tissues they produce; however many of them have these general steps. Cells are isolated, expanded in vitro, and some processes have manipulation steps, i.e. generation of iPSC and re-differentiation. There there is a selection step and cells may go into a Master Cell Bank (MCB) for storage, whether the process is allogeneic or patient-specific. For producing the organ, the cells may undergo a secondary expansion before being loaded into a scaffold to make a construct and placed in a bioreactor. There may be more construct maturation steps as well as construct folding or assembly into higher-order structures. At each step away from simple 2D culture, measurement of the quality attributes, and the needs of cells, gets more difficult. There are static measures like cell number, live/dead, phenotype, and morphology that are well established for 2D cultures, but need more development for higher order structures. In addition, there are static quality attributes like cell aggregation, appearance of functional maturity, and mechanical integrity that could be applied to cells integrated into 3D and higher-order structures. Dynamic measurements add the element of time. Static measurements in 2D cultures become doubling time, maturation rates, migration rates, and change in morphology. Dynamic measures may better predict later product QAs. In addition to these attributes, cells integrated into constructs may have self-assembly rates, cell signaling changes, and functional changes like acquiring a heartbeat, and responses to stimulus that reflect proper whole-organ function. Each of these attributes need to be measured as a reflection of cell quality. Environmental QAs like oxygen and glucose consumption rate, lactate production rate, change in pH, and reactive oxygen species (ROS), can be critical process parameters (CPPs). Also production of soluble factors that indicate organ function, like urine or insulin could be measured in the liquid phase of the culture. These quality attributes are reflective of the overall function of the organ, but are indirect measures of cell health. They are also rarely measured in process steps before introduction to the bioreactor, but perhaps if they were used throughout the production process, they could help predict product quality earlier.
At each of these stages, the needs of cells may change. Detecting the characteristics of cells in 3D constructs becomes difficult or impossible using the technologies that we traditionally use on dissociated cells in 2D culture. Environmental measures of cell/tissue health like glucose consumption and change in secretion profile can be monitored in bioreactors, but these are indirect. Assessing the healing organ for cellular activities like migration into functional layers and phenotypic maturation also has to change to organ-level functional assessments like urine production for kidney, and beating rhythm for heart.
Traditional manual cell culture equipment and techniques used in 2D culture are the most familiar to researchers and the least expensive, but they expose cells and tissues to non-physiologic room air (25°C/20% O2/0.1% CO2) conditions and other stresses. They introduce variability and subjective decision making into the production process. The cells experience environments which are stressful, unseen, undocumented, and largely uncontrolled.
What cytocentric technologies can we use to measure cell quality so that we not only produce, but reliably reproduce RM tissue products?
What defines a Critical Quality Attribute (CQA)?
A physical, chemical, biological, or microbiological property or characteristic that should be within an appropriate limit, range, or distribution to ensure the desired product quality. (5)
As per US Code of Federal Regulations (21CFR610) (6), standard criteria for product quality must be met to allow for the release of a cell therapy product. These include sterility, purity, identity, and potency. Sterility criteria are defined in 21CFR610.12, and are required to ensure viable contaminating microorganisms are not present. Identity criteria are defined in 21CFR610.14, and are critical not only for proper product labeling, but also to distinguish the product from others manufactured in the same facility. This usually means identification of cell-specific marker panels of expressed cell proteins, mRNAs, or secreted molecules. Purity criteria are defined in 21CFR610.13, and testing must be performed to ensure that the product is free of extraneous material, process residuals, or any contaminating cells. These might compromise the efficacy or safety of the final product. Potency criteria are defined in 21CFR610.3, and measure the biological activity of the product. Sterility, purity, identity, and potency are often defined as critical quality attributes (CQAs) because these product characteristics have great impact on product quality. Figure 1 shows several other important cytocentric measurements that could be CQAs, and that are often used for characterizing cell-based products and intermediates, including appearance (morphology), viability, cell count, and doubling time. In some cell products, passage number is also critical.
In the vast majority of laboratories, cell number, morphology, density, and viability are all static CQAs that are assessed through subjective visual inspection, despite clear and long-standing problems with reproducibility and reference standards (7, 8). Chemical methods used to assay cell number and viability measure membrane integrity, proliferation, and mitochondrial activity. AlamarBlue (resazurin), unlike tetrazolium salts, is a nontoxic redox indicator that has been used to assess metabolic activity over time in 2D and 3D cultures (9), However, the dye is pH, temperature, and light sensitive (10), and the uptake of AlamarBlue by cells can be altered by the surrounding construct materials (11). Intracellular ATP can be measured in organoids using assays like Cell Titre Glo and ATPlite, and the nucleic acid content of a construct can be measured with dyes like Pico Green. However, the effect of dye residues on patients present risks to their use in clinical RM products and could limit their use to sentinel organ cultures in a batch. Cytocentric technologies for in-line, continuous monitoring during cell and tissue production need to be label-free and non-destructive, as well as rigorous.
The first Cytocentric Principle is to protect cell cultures from microbial contamination (1). Imaging and optical technologies may help reduce contamination risks by reducing the intrusion of physical probes. Advances in imaging and image processing increasingly transform subjective attributes into quantitative metrics that can be assessed, documented, and monitored. Moreover, non-invasive imaging and monitoring can be automated (3, 12, 13). Automation of process documentation and decision-making algorithms based on imaging parameters is not trivial. It requires rigor and process integration across several domains: image capture, image processing, image analysis, metric reporting, and data archiving. Consensus in precise and stable definitions of metrics, process and reporting standards, and systems of data capture and integration will increasingly enable process documentation and monitoring.
Following established standards (14), large field of view (LFOV) imaging strategies enable assembly of images over large areas of cell populated surfaces at pixel sizes of ∼1 micron or less. When captured with appropriate focus, processed into a seamless montage and corrected for lighting, diverse image-based tools can measure individual cells (size, area, shape, circularity) and group metrics (cell clustering, cell density, confluence, connectivity, alignment, heterogeneity). Texture metrics can assess tightly adherent cell sheets, where individual cells are not identifiable. In addition to static variables, dynamic variables (e.g., migration, proliferation, change in morphology, detachment, lysis) can also be assessed over time with quantitative imaging (Figure 1).
Phase contrast and bright-field microscopy performed with high resolution imaging, at multiple focal planes in time series, can provide extensive data sets with minimal effects on the cells. This is especially important for many cell culture experiments that require or benefit from continuous monitoring of cell growth. Image analysis software can measure changes in confluence and doubling rates, as well as track morphological changes, providing valuable insights about the health of the cells and rich data sets for additional insights. Phase contrast can be used to track morphological changes in cells following growth factor stimulation meant to enhance particular biological activities. For example, following IFNγ-treatment of MSCs, morphological subsets associated with enhanced immunosuppressive properties can be observed emerging after 24 h of IFNγ-stimulation (15).
Quantitative imaging also can be used effectively for quality/reproducibility control for cell sourcing. Performance based clone selection, i.e., starting a fabrication process by selecting individual cell clones based on defined quantitative metrics will reduce variability in outcome. Quantitative imaging metrics can also feed machine learning algorithms that define CQAs for starting materials and clones that are linked to critical downstream product CQAs.
Digital Holographic Microscopy (DHM) is the most common form of quantitative phase imaging and allows for non-invasive imaging of living cells over time. DHM allows for real-time detection and quantification of both single cells and cell populations without the need to disrupt routine cell culture. DHM has been used on a wide range of cells, ranging from bacteria and protozoa to mammalian cells (16). In addition, DHM can be used to study a range of dynamic cellular parameters from movement, proliferation, and cell death to morphological, phenotypical, and behavioral changes (17). Moreover, as DHM is non-invasive and introduces minimal external stress to the cells, the technology is suitable for long term live-cell imaging. This is especially important for cells that are difficult to obtain or grow. DHM is a uniquely cytocentric measurement.
Over the past decade, a broad spectrum of 3D culture models employing scaffolds, spheroids, organoids, organs-on-chips (OOC), and bioprinted scaffolds have emerged to better recapitulate the complexity of in vivo tissues. In contrast to 2D traditional cell culture, these models may be more physiologically relevant in vitro systems for drug development and screening, disease modeling, regenerative medicine, and fundamental biomedical research (18–22).
OOC technologies enable the precise control of the micro-environmental factors that can modulate cell conditions, 3D tissue development, and tissue responses to stimuli. This allows OOC systems to provide more in vivo-like environmental, biological, biomechanical, and biochemical cues to the cells for creating progressively more complex tissue and organ models (23). Co-culture, 3D tissue-tissue interfaces, cyclic shear, stretch, electrical biomechanical signals, and controlled oxygen microenvironments all provide cells in culture a more biologically relevant environment (19, 24, 25).
Microfluidic devices also provide a platform where the cell and tissue culture conditions and metabolic activities can be monitored non-destructively and continuously. This capability of the OOC technology is still in its infancy, but has tremendous potential for regenerative medicine and tissue engineering applications (26, 27). Miniaturized electrodes integrated into the microfluidic devices have been used for obtaining spatiotemporal information on cell attachment, growth, morphology, function, differentiation, transepithelial-endothelial electrical resistance (TEER), electrophysiological function, biomechanical contractility, and motility in microfluidic and OOC devices (26–29). Many of these measurements could be important early indicators of product quality and could become CQAs for relevant cell manufacturing processes.
This illustrates the need for more development of technologies such as sensors and integrated electrodes that can collect and communicate cell information to the culture controlling system automatically for documentation, analysis, and adjustments in environment for higher order tissue structures.
The perfusing media in OOC models can simultaneously be sampled to monitor critical process parameters (CPP) in the cell environment that also not only reflect culture health, but also control it. Allowing the cells to grow undisturbed in optimal, reproducible conditions is fundamental to cytocentric measurements.
A Critical Process Parameter (CPP) is defined as a process parameter whose variability has an impact on a critical quality attribute and therefore should be monitored or controlled to ensure the process produces the desired quality (5). The use of non-destructive, non-intrusive sensors is in accord with cytocentric manufacturing approaches. Electrochemical sensors can measure glucose, lactate, L-glutamate and other analytes that can indicate cell culture conditions (Figure 1) (30–33). Optical sensors employ a range of analyte sensitive indicator dyes that emit a signal upon illumination and interaction with the target analyte (34, 35). Luminescence optical sensors have most successfully been used in sensing oxygen in microfluidic devices (19, 26, 30, 34). Temperature and pH have also been measured in microfluidic devices using optical sensors (27, 35). However, they have been integrated into OOC devices only in a limited way.
In-line process analytics of cytocentric CPPs such as O2, CO2, lactate, temperature, cell density, viability and cell morphometrics are key to ensuring the quality of growing cell and tissue cultures. Dissolved oxygen (DO) and pH are two critical parameters that are known to affect cell and tissue metabolism, morphology, gene expression, and cell health, and protein production (36). For analysis of 3D cultures during production, some promising innovations employ the functionalization of ECM materials with luminescent optical sensor nanoparticles for live oxygen monitoring (37), as well as the use of dielectric impedance spectroscopy to characterize cell viability (38).
As oxygen does not dissolve easily in aqueous media, local DO around the cell is a function of local cell density, metabolic rate, fluid convection and the path of O2 diffusion. Studies have incorporated electrochemical sensors to monitor oxygen and lactate in liver spheroids cultured in 96 well plates (39). These technologies may be able to more finely control tissue microenvironments for the development of physiologically relevant healthy and diseased tissue models.
However, monitoring of DO and pH does not often begin until the cells enter the bioreactor as constructs. Cell culture incubators are usually at 5% CO2 and 18%–20% O2. The CO2, in conjunction with a carbonate buffer system in many cell culture media, will stabilize the pericellular pH between 7.2–7.4. If the medium contains phenol red, researchers open the incubator and take cultures out to visually estimate the color of the media for pH. This is a highly subjective and irreproducible assessment.
There are few readily available sensing technologies made specifically for measuring DO and pH levels in small 2D culture vessels. Fill volumes are far too low to cover these sensors. Optical sensing technologies, which consist of fluorescent sensor stickers and a separate reading device, have the potential to provide DO and pH monitoring in small 2D culture vessels because they can be smaller. However, outfitting each cell culture vessel with optical sensors currently proves cumbersome in terms of price, incubator space, and workflow. Most researchers forgo monitoring of DO and pH altogether in the 2D phase of RM processes. However, without these fundamental CPPs, it may be impossible to insure sufficient control of the cellular environment to produce a consistent RM product.
To achieve truly manufacturable RM products, environmental control, modeling, or sensing technologies must be implemented from the very beginning of cell and tissue culture processes (Figure 1). In a perfect scenario, ex vivo cells and tissues would have a perfectly simulated in vivo experience. Ideally, every important analyte, metabolite, and variable should be understood, recorded, and controlled through a broad array of sensors and automatic-control feedback loops. Cytocentric measurements would be universally performed in all of the diverse vessel types that cells encounter in every step throughout the tissue production process. Presently, this is not realistic, but it is time to lay the foundation and take the first steps. The National Institute of Standards and Technologies is active in the area of establishing new standards for cell measurements (link).
Potential CQAs and CPPs may vary between cell sources, tissue types, and product applications. For example, a metabolic shift may signal tissue maturation in liver, but not in skin. Defining the optimal conditions for different RM products will require knowledge and control of process parameters and their direct association with outcome metrics.
As we develop this knowledge, the ultimate goal is to use these data for active, real-time in-process adaptation. Detection of a product deviation, identified using imaging, can enable flagging of product variation and a diversity of in-process intervention strategies, such as oxygen tension modulation, targeted picking, or thinning routines (13). Cytocentric analytics and Smart Manufacturing algorithms are needed to identify CQAs and CPPs that reliably result in reproducible RM tissue products.
Cytocentric measurements are improving, particularly with development of imaging-based metrics. However, we need to continue the evolution from manual, static measurements to automated, dynamic measurements that allow for real-time measurement and control of process parameters. Technical challenges remain in getting the information we need about the constantly changing tissue cultures in our care. The use of continuous measurement technologies like the electrochemical and optical sensors in bioreactors, microfluidic devices, and tissue culture systems need to be more widespread. The knowledge obtained from such sophisticated in vitro systems is essential to developing functional and truly reproducible in vivo-like tissues for Regenerative Medicine.
The original contributions presented in the study are included in the article/Supplementary Material, further inquiries can be directed to the corresponding author.
Ethical review and approval was not required for this study in accordance with the local legislation and institutional requirements.
All authors discussed the contents, wrote the manuscript, and approved of the final manuscript. TP edited portions. ADH conceived and designed the paper, and edited the final manuscript.
We thank Ray Gould, Stassa P.D. Henn and Shannon Darou for their critical reading of the manuscript.
Authors ADH, and RY are employed by BioSpherix, Ltd. Author TP is employed by 3DSystems. Author SS is employed by Vigene Biosciences. Author LL is employed by PHI AB. Author TFFH is employed by Thrive Bioscience. Author JB was employed by SBI, Inc. Author GFM is employed by CellX Technologies.
The remaining authors declare that the research was conducted in the absence of any commercial or financial relationships that could be construed as a potential conflict of interest.
All claims expressed in this article are solely those of the authors and do not necessarily represent those of their affiliated organizations, or those of the publisher, the editors and the reviewers. Any product that may be evaluated in this article, or claim that may be made by its manufacturer, is not guaranteed or endorsed by the publisher.
2D, 2-dimensional; 3D, 3-dimensional; AI, artificial intelligence; BSC, biological safety cabinet; CO2, carbon dioxide; CPP, critical process parameter; CQA, critical quality attribute; DO, dissolved oxygen; DHM, digital holographic microscopy; LFOV, large field–of-view; OOC, organ-on-a-chip; RM, regenerative medicine; RH, relative humidity; ROS, reactive oxygen species; MTT, methyl thiazol tetrazolium, MPS, microphysiological systems; QBD, quality by design; TEER, transepithelial-endothelial electrical resistance.
1. Henn AD, Mitra K, Hunsberger J, Sun XS, Nardone M, Montero R, et al. Applying the cytocentric principles to regenerative medicine for reproducibility. Curr Stem Cell Rep. (2022) 8(4). doi: 10.1038/srep02327
2. Penolazzi L, Lambertini E, D’Agostino S, Pozzobon M, Notarangelo MP, Greco P, et al. Decellularized extracellular matrix-based scaffold and hypoxic priming: a promising combination to improve the phenotype of degenerate intervertebral disc cells. Life Sci. (2022) 301:120623. doi: 10.1016/j.lfs.2022.120623
3. Bohrer LR, Stone NE, Mullin NK, Voigt AP, Anfinson KR, Fick JL, et al. Automating iPSC generation to enable autologous photoreceptor cell replacement therapy. J Transl Med. (2023) 21(1):161. doi: 10.1186/s12967-023-03966-2
4. Wang Y, Cui H, Esworthy T, Mei D, Wang Y, Zhang LG. Emerging 4D printing strategies for next-generation tissue regeneration and medical devices. Adv Mater. (2022) 34(20):2109198. doi: 10.1002/adma.202109198
5. Center for Drug Evaluation and Research (U.S.) cfBEaRUS, & International Conference on Harmonisation. Guidance for Industry: Q8(R2) pharmaceutical development. In: Services USDoHaH, editor. Rockville, MD2009.
7. Berkson J, Magath TB, Hurn M. The error of estimate of the blood cell count as made with the hemocytometer. Am J Physiol-Legacy Content. (1939) 128(2):309–23. doi: 10.1152/ajplegacy.1939.128.2.309
8. Piccinini F, Tesei A, Arienti C, Bevilacqua A. Cell counting and viability assessment of 2D and 3D cell cultures: expected reliability of the trypan blue assay. Biol Proced Online. (2017) 19(1):8. doi: 10.1186/s12575-017-0056-3
9. Bikmulina P, Kosheleva N, Efremov Y, Antoshin A, Heydari Z, Kapustina V, et al. 3D or not 3D: a guide to assess cell viability in 3D cell systems. Soft Matter. (2022) 18(11):2222–33. doi: 10.1039/D2SM00018K
10. Rampersad SN. Multiple applications of alamar blue as an indicator of metabolic function and cellular health in cell viability bioassays. Sensors. (2012) 12(9):12347–60. doi: 10.3390/s120912347
11. Bonnier F, Keating ME, Wróbel TP, Majzner K, Baranska M, Garcia-Munoz A, et al. Cell viability assessment using the alamar blue assay: a comparison of 2D and 3D cell culture models. Toxicol in Vitro. (2015) 29(1):124–31. doi: 10.1016/j.tiv.2014.09.014
12. Sartorius.com Ao. Live-cell analysis instruments (2022) Available at: https://www.sartorius.com/en/products/live-cell-imaging-analysis/live-cell-analysis-instruments
13. cellxtechnologies.com Ao. Cell X technologies (2022) Available at: https://cellxtechnologies.com/cell-x-technology
14. Standard Practice for Automated Colony Forming Unit (CFU) Assays - Image Acquisition and Analysis Method for enumerating and Characterizing Cells and Colonies in Culture (2020).
15. Marklein RA, Klinker MW, Drake KA, Polikowsky HG, Lessey-Morillon EC, Bauer SR. Morphological profiling using machine learning reveals emergent subpopulations of interferon-γ–stimulated mesenchymal stromal cells that predict immunosuppression. Cytotherapy. (2019) 21(1):17–31. doi: 10.1016/j.jcyt.2018.10.008
16. Alm K, El-Schich Z, Miniotis MF, Wingren AG, Janicke B, Oredsson S. Cells and holograms—holograms and digital holographic microscopy as a tool to study the morphology of living cells. Holography—Basic Princ Contem App. (2013). p. 335–51.
17. Wingren AG. Moving into a new dimension: tracking migrating cells with digital holographic cytometry in 3D. Cytometry Part A. (2019) 95(2):144–6. doi: 10.1002/cyto.a.23679
18. Yan X, Zhou L, Wu Z, Wang X, Chen X, Yang F, et al. High throughput scaffold-based 3D micro-tumor array for efficient drug screening and chemosensitivity testing. Biomaterials. (2019) 198:167–79. doi: 10.1016/j.biomaterials.2018.05.020
19. Jalili-Firoozinezhad S, Gazzaniga FS, Calamari EL, Camacho DM, Fadel CW, Bein A, et al. A complex human gut microbiome cultured in an anaerobic intestine-on-a-chip. Nat Biomed Eng. (2019) 3(7):520–31. doi: 10.1038/s41551-019-0397-0
20. Huh D, Matthews BD, Mammoto A, Montoya-Zavala M, Hsin HY, Ingber DE. Reconstituting organ-level lung functions on a chip. Science. (2010) 328(5986):1662–8. doi: 10.1126/science.1188302
21. Driehuis E, Kretzschmar K, Clevers H. Establishment of patient-derived cancer organoids for drug-screening applications. Nat Protoc. (2020) 15(10):3380–409. doi: 10.1038/s41596-020-0379-4
22. Mazzocchi A, Devarasetty M, Huntwork R, Soker S, Skardal A. Optimization of collagen type I-hyaluronan hybrid bioink for 3D bioprinted liver microenvironments. Biofabrication. (2018) 11(1):015003. doi: 10.1088/1758-5090/aae543
23. Ewart L, Apostolou A, Briggs SA, Carman CV, Chaff JT, Heng AR, et al. Performance assessment and economic analysis of a human liver-chip for predictive toxicology. Commun Med. (2022) 2(1):154. doi: 10.1038/s43856-022-00209-1
24. Kasendra M, Tovaglieri A, Sontheimer-Phelps A, Jalili-Firoozinezhad S, Bein A, Chalkiadaki A, et al. Development of a primary human small intestine-on-a-chip using biopsy-derived organoids. Sci Rep. (2018) 8(1):1–14. doi: 10.1038/s41598-018-21201-7
25. Benam KH, Villenave R, Lucchesi C, Varone A, Hubeau C, Lee H-H, et al. Small airway-on-a-chip enables analysis of human lung inflammation and drug responses in vitro. Nat Methods. (2016) 13(2):151–7. doi: 10.1038/nmeth.3697
26. Fuchs S, Johansson S, Tjell AØ, Werr G, Mayr T, Tenje M. In-line analysis of organ-on-chip systems with sensors: integration, fabrication, challenges, and potential. ACS Biomater Sci Eng. (2021) 7(7):2926–48. doi: 10.1021/acsbiomaterials.0c01110
27. Zhang YS, Aleman J, Shin SR, Kilic T, Kim D, Shaegh SAM, et al. Multisensor-integrated organs-on-chips platform for automated and continual in situ monitoring of organoid behaviors. Proc Natl Acad Sci USA. (2017) 114(12):E2293–302. doi: 10.1073/pnas.1612906114
28. Henry OY, Villenave R, Cronce MJ, Leineweber WD, Benz MA, Ingber DE. Organs-on-chips with integrated electrodes for trans-epithelial electrical resistance (TEER) measurements of human epithelial barrier function. Lab Chip. (2017) 17(13):2264–71. doi: 10.1039/C7LC00155J
29. Maoz BM, Herland A, Henry OY, Leineweber WD, Yadid M, Doyle J, et al. Organs-on-chips with combined multi-electrode array and transepithelial electrical resistance measurement capabilities. Lab Chip. (2017) 17(13):2294–302. doi: 10.1039/C7LC00412E
30. Bavli D, Prill S, Ezra E, Levy G, Cohen M, Vinken M, et al. Real-time monitoring of metabolic function in liver-on-chip microdevices tracks the dynamics of mitochondrial dysfunction. Proc Natl Acad Sci U S A. (2016) 113(16):E2231–40. doi: 10.1073/pnas.1522556113
31. Misun PM, Rothe J, Schmid YR, Hierlemann A, Frey O. Multi-analyte biosensor interface for real-time monitoring of 3D microtissue spheroids in hanging-drop networks. Microsyst Nanoeng. (2016) 2(1):1–9. doi: 10.1038/micronano.2016.22
32. Moser I, Jobst G, Urban GA. Biosensor arrays for simultaneous measurement of glucose, lactate, glutamate, and glutamine. Biosens Bioelectron. (2002) 17(4):297–302. doi: 10.1016/S0956-5663(01)00298-6
33. Rodrigues NP, Sakai Y, Fujii T. Cell-based microfluidic biochip for the electrochemical real-time monitoring of glucose and oxygen. Sens Actuators, B. (2008) 132(2):608–13. doi: 10.1016/j.snb.2007.12.025
34. Wang X-D, Wolfbeis OS. Optical methods for sensing and imaging oxygen: materials, spectroscopies and applications. Chem Soc Rev. (2014) 43(10):3666–761. doi: 10.1039/C4CS00039K
35. Wang X-D, Wolfbeis OS, Meier RJ. Luminescent probes and sensors for temperature. Chem Soc Rev. (2013) 42(19):7834–69. doi: 10.1039/c3cs60102a
36. Zakrzewski R, Lee K, Lye GJ. Development of a miniature bioreactor model to study the impact of pH and DOT fluctuations on CHO cell culture performance as a tool to understanding heterogeneity effects at large-scale. Biotechnol Prog. (2022) 38(4):e3264. doi: 10.1002/btpr.3264
37. Trampe E, Koren K, Akkineni AR, Senwitz C, Krujatz F, Lode A, et al. Functionalized bioink with optical sensor nanoparticles for O2 imaging in 3D-bioprinted constructs. Adv Funct Mater. (2018) 28(45):1804411. doi: 10.1002/adfm.201804411
38. Narayanan LK, Thompson TL, Shirwaiker RA, Starly B. Label free process monitoring of 3D bioprinted engineered constructs via dielectric impedance spectroscopy. Biofabrication. (2018) 10(3):035012. doi: 10.1088/1758-5090/aaccbf
Keywords: regenerative medicine, tissue engineering, cell culture techniques, organoids, stem cells, Sensors
Citation: Henn AD, Pereira T, Hunsberger J, Mitra K, Izadifar Z, Somara S, Lindström L, Forest Farb-Horch T, Boy J, Muschler GF, Bauer SR and Yerden R (2023) Cytocentric measurement for regenerative medicine. Front. Med. Technol. 5:1154653. doi: 10.3389/fmedt.2023.1154653
Received: 30 January 2023; Accepted: 11 April 2023;
Published: 27 April 2023.
Edited by:
Jonathan Ian Dawson, University of Southampton, United KingdomReviewed by:
Prasoon Kumar, National Institute of Technology, India© 2023 Henn, Pereira, Hunsberger, Mitra, Izadifar, Somara, Lindström, Forest Farb-Horch, Boy, Muschler, Bauer and Yerden. This is an open-access article distributed under the terms of the Creative Commons Attribution License (CC BY). The use, distribution or reproduction in other forums is permitted, provided the original author(s) and the copyright owner(s) are credited and that the original publication in this journal is cited, in accordance with accepted academic practice. No use, distribution or reproduction is permitted which does not comply with these terms.
*Correspondence: Alicia D. Henn YWhlbm5AYmlvc3BoZXJpeC5jb20=
†ORCID Alicia D. Henn orcid.org/0000-0001-8320-2369 Taci Pereira orcid.org/0000-0002-3739-5777 Kunal Mitra orcid.org/0000-0002-7277-4908 Lisa Lindström orcid.org/0009-0008-4510-4635 Steven R. Bauer orcid.org/0000-0003-2831-846X
Disclaimer: All claims expressed in this article are solely those of the authors and do not necessarily represent those of their affiliated organizations, or those of the publisher, the editors and the reviewers. Any product that may be evaluated in this article or claim that may be made by its manufacturer is not guaranteed or endorsed by the publisher.
Research integrity at Frontiers
Learn more about the work of our research integrity team to safeguard the quality of each article we publish.