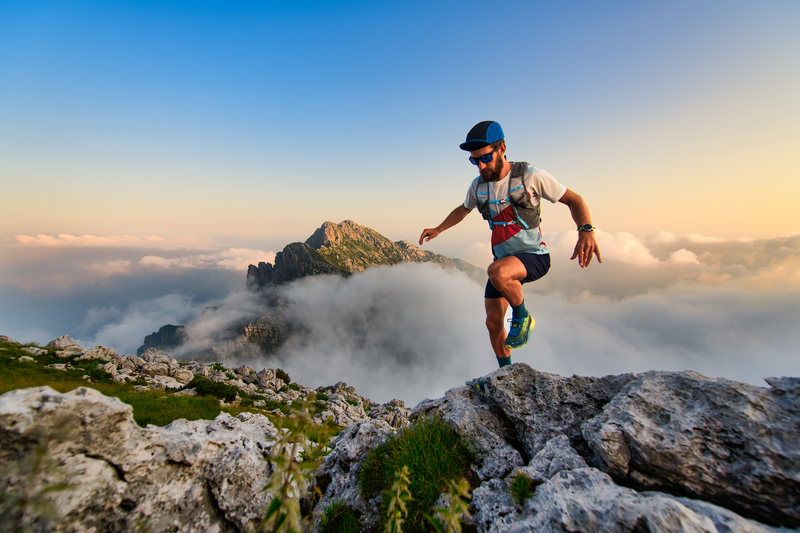
95% of researchers rate our articles as excellent or good
Learn more about the work of our research integrity team to safeguard the quality of each article we publish.
Find out more
REVIEW article
Front. Med. Technol. , 16 December 2022
Sec. Diagnostic and Therapeutic Devices
Volume 4 - 2022 | https://doi.org/10.3389/fmedt.2022.901331
This article is part of the Research Topic Insights in Diagnostic and Therapeutic Devices View all 5 articles
Background: Despite being available for more than three decades, quantitative gait analysis remains largely associated with research institutions and not well leveraged in clinical settings. This is mostly due to the high cost/cumbersome equipment and complex protocols and data management/analysis associated with traditional gait labs, as well as the diverse training/experience and preference of clinical teams. Observational gait and qualitative scales continue to be predominantly used in clinics despite evidence of less efficacy of quantifying gait.
Research objective: This study provides a scoping review of the status of clinical gait assessment, including shedding light on common gait pathologies, clinical parameters, indices, and scales. We also highlight novel state-of-the-art gait characterization and analysis approaches and the integration of commercially available wearable tools and technology and AI-driven computational platforms.
Methods: A comprehensive literature search was conducted within PubMed, Web of Science, Medline, and ScienceDirect for all articles published until December 2021 using a set of keywords, including normal and pathological gait, gait parameters, gait assessment, gait analysis, wearable systems, inertial measurement units, accelerometer, gyroscope, magnetometer, insole sensors, electromyography sensors. Original articles that met the selection criteria were included.
Results and significance: Clinical gait analysis remains highly observational and is hence subjective and largely influenced by the observer's background and experience. Quantitative Instrumented gait analysis (IGA) has the capability of providing clinicians with accurate and reliable gait data for diagnosis and monitoring but is limited in clinical applicability mainly due to logistics. Rapidly emerging smart wearable technology, multi-modality, and sensor fusion approaches, as well as AI-driven computational platforms are increasingly commanding greater attention in gait assessment. These tools promise a paradigm shift in the quantification of gait in the clinic and beyond. On the other hand, standardization of clinical protocols and ensuring their feasibility to map the complex features of human gait and represent them meaningfully remain critical challenges.
Changes in the signature of gait, or the unique sequential walking pattern in humans, reveal key information about the status and progression of numerous underlying health challenges, from neurological and musculoskeletal conditions to cardiovascular and metabolic disease, and to ageing-associated ambulatory dysfunction and trauma. Accurate reliable identification of gait patterns and characteristics in clinical settings, as well as monitoring and evaluating them over time, enable effective tailored treatment, inform predictive outcome assessment, and an allow for an overall better practice of precision medicine.
In clinical gait assessment, both a person's “ability” to walk and “how” the individual walks are highly relevant. The walking ability of a person is typically based on two main aspects: how far can an individual walk and what is his/her tolerance level (1). For example, for post stroke gait assessment, 3-, 6-, or 10 min walk tests are used, in addition to Functional Ambulation Category (FAC), Short Physical Performance Battery (SPPB), and/or Motor Assessment Scale (MAS). Other clinical subjective assessment scales include the Unified Parkinson Disease Rating Scale (UPDRS) the Scale for the Rating and Assessment of Ataxia (SARA)], the Alzheimer's Disease Assessment Scale (ADAS), the Expanded Disability Status Scale (EDSS) the High-level MobilitARTIy Assessment Tool (HiMAT), and the Dynamic Gait Index (2). The quality of gait or “how” the person walks, on the other hand, highly depends on the quantification of gait patterns and accurate identification of specific gait characteristics. Despite evidence of the advantages of quantitative instrumented gait analysis (IGA) in clinical practice and recommendations by the National Institute for Health and Clinical Excellence (NICE) (3) identifying IGA is the preferrable choice for “gait-improving surgery”, it remains not well leveraged in clinical settings due to the high cost/cumbersome equipment and complex protocols/data analysis associated with traditional gait labs, as well as diverse training, experience and preference of clinical teams (3–5). Moreover, the use of IGA by allied health professionals, such as physiotherapists, occupational therapists and orthotists, and training also remain non standardized and limited (5–7).
Observational gait analysis continues to be popular among clinicians due to its inherent simplicity, availability, and low cost (8). On the other hand, the validity, reliability, specificity, and responsiveness (9, 10) of these qualitative methods are controversial and increasingly being questioned (6). Furthermore, there is evidence to suggest that subjective clinical assessment scales may not be sensitive to disease severity and specific characteristics and may limit understanding of underlying disease mechanisms, hence adversely impacting optimal treatment (11). Examples of such scales include Multiple Sclerosis (MS), where subjective measures, such as the Expanded Disability Status Scale (EDSS), the Multiple Sclerosis Severity Scale (MSSS), Multiple Sclerosis Walking Scale (MSWS), and Multiple Sclerosis Functional Composite (MSFC), continue to be widely used in clinical practice. These scales have been criticized for lack of sensitivity (12), high interrater variability (13), as well as being prone to practice effects and variability (14, 15). Similarly, clinical assessment of Parkinson's disease (PD) using the Unified Parkinson's Disease Rating Scale (UPDRS) is subjective and largely dependent on the expertise and experience of the clinicians, as well as the severity of the disease (16). In Stroke patients, assessment tests such as Functional Ambulation Category (FAC), Short Physical Performance Battery (SPPB), and/or Motor Assessment Scale (MAS) are typically employed, along with qualitative observational/visual gait analysis (using naked eye or video images). Nevertheless, the validity, reliability, specificity, and responsiveness of these qualitative methods are questioned (9), and although they may be useful for the rudimentary evaluation of some gait parameters, they are not adequate for analyzing the multifaceted aspects of gait variability and complexity (17).
Instrumented gait analysis (IGA), which can provide accurate and precise quantitative measurement of gait patterns and characteristics, has long been the gold standard for gait assessment in research practice (18). IGA generally refers to the use of instrumentation to capture and analyze a variety of human gait parameters (spatiotemporal, kinematic, and kinetic measures). Traditional IGA systems include motion capture systems, and force plates, instrumented walkways, and treadmills, while more recent systems comprise of miniaturized wearable sensing system, computational platforms and modalities (18). Literature on the clinical applicability and efficacy of IGA indicates that IGA-based quantitative assessment can improve the diagnosis, outcome prediction, and rehabilitation of various gait impairments as compared to conventional observational scales and techniques for gait dysfunction in a wide spectrum of diseases including MS, PD, Stroke, and Cerebral Palsy (9–13). A recent review on the clinical efficacy of IGA confirms that there is strong evidence that 3-D gait analysis, or 3DGA; has the potential to alter and reinforce treatment decisions; increases confidence in treatment planning and agreement among clinicians; can better identify diagnostic groups and expected treatment outcomes; and overall can improve patient outcomes if recommendations are followed (19).
Emerging at an unprecedented rate, wearable sensing systems and associated computational modalities are rapidly transforming the quality and accessibility of healthcare, spanning multiple applications from neurology and orthopedics to cardiovascular, metabolic, and mental health. Magnetic (e.g., magnetometers), inertial measurement (e.g., accelerometers and gyroscopes), and force sensors (e.g., insole foot pressure) nowadays offer unprecedented data capture opportunities that can overcome limitations of non-wearable devices due to their low-cost, less setup-time and complexity, lightweight, and portability, making them ideal for out-of-lab and continuous monitoring in the clinic and beyond (20). Magneto-inertial measurement units (MIMUs), in conjunction with force pressure sensors, have the capability of capturing spatiotemporal, kinematic, and kinetic gait data (2) rendering the concept of a mobile gait lab a reality. Such labs can inherently overcome the limitations of IGA traditional labs, providing less costly and cumbersome tools with potential for gait assessment in natural environments (clinics, homes, sports arenas, etc.), user friendly interfaces, and the opportunity to provide continuous real-time feedback to clinicians and patients, as well as tele rehabilitation capabilities. In addition, wearable systems allow for easy synchronization with other physiological measurement systems, including EMG, ECG, and EEG, towards the acquisition of invaluable multimodal continuous physiological data in various settings.
This scoping review aims to provide a summary of the current state of clinical gait assessment, including shedding light on gait pathologies and clinical indices and scales, as well as a roadmap for the development of future gait mobile labs- highlighting the clinical validity and reliability of the latest devices and data interpretation algorithms. The word novel in the title of this review reflects recent emergence/implementation of the technologies reviewed and/or recent commercialization. This includes wearable technologies, as well as AI-driven computational platforms. The remainder of the review is structured as follows: Section 2 describes the adopted methodology, including the approach, search strategy and selection criteria. Section 3 details clinical gait pathologies, relevant parameters, as well as current clinical gait assessment tools, scales, and indices, while Section 4 presents gait assessment technologies applicable to clinical settings, including state-of-the-art imaging techniques and wearable technologies, algorithms, and novel AI-driven computational platforms. Section 5 deliberates on the concept of a mobile gait lab for clinical applications. Section 6 highlights the limitations, while Section 7 presents the conclusive remarks and future work.
This review is aimed at summarizing various clinical gait pathologies and associated parameters, applicable gait analysis techniques and gait indices, and the latest trends in wearables systems and algorithms. To address this broader research objective, the authors adopted a scoping review approach rather than a systematic review approach. As reported in (21), scoping reviews are ideal for addressing a broader scope with a more expansive inclusion criterion.
A keyword search was performed in PubMed, Web of Science, Medline, and ScienceDirect databases, using a combination of search terms from the following groups: 1. (normal gait OR pathological gait OR gait parameters OR gait indices), 2. (gait assessment OR gait analysis), 3. (wearable systems OR wearable algorithms), 4. (inertial measurement units OR accelerometer OR gyroscope OR magnetometer OR insole sensors OR electromyography sensors). No limit for the year of publication was set, however, the search was last updated in December 2021. Only articles written in the English language were considered in this review. In addition, the reference list of the included articles was checked to identify additional relevant publications meeting the inclusion criteria. The literature search and data extraction were carried out independently by two authors (AAH, DMM) and any inconsistencies and disagreements discrepancies were resolved through following discussions with the other authors (NA, MER, KK).
This scoping review included original published works and review articles which met the following inclusion/exclusion criteria: (i) studies addressing various gait disorders and associated gait parameters, (ii) studies focusing on instrumented gait analysis techniques and gait indices, (iii) studies evaluating the use, validity, and reliability of wearable-based gait measurement devices/systems for measuring gait events, and evaluating and assessing gait dysfunction, (iv) studies concerning the applicability of sensor fusion techniques and algorithms applicable for wearable-based systems with application to gait analysis. The title and/or abstract of the studies were initially screened for suitability. The full-text articles meeting the inclusion criteria were obtained for data extraction and synthesis. A flowchart explaining the same is shown in Figure 1.
Normal gait can be defined as a series of rhythmic, systematic, and coordinated movements of the limbs and trunk that results in the forward advancement of the body's center of mass (22). A result of intricate dynamic interactions between the central nervous system and feedback mechanisms (23), walking is characterized by individual gait cycles and functional phases (Figure 2). A gait cycle consists of two main phases, stance, and swing, which are further divided into five and three functional phases, respectively. The stance phase corresponds to the duration between heel strike and toe-off of the same foot, constituting approximately 60% of the gait cycle. The swing phase begins with toe-off and ends with heel contact of the same foot and occupies 40% of the cycle. As each functional phase contributes to successfully accomplishing the goal of walking, healthy gait involves cyclic and complementary movements of the limbs under control. It is characterized by stance stability; toe clearance during the swing; pre-positioning at swing; sufficient step length; as well as mechanical and metabolic efficiency (24). Table 1 provides gait parameter ranges based on studies on healthy adults. Determining an appropriate normal range for many of the features is highly challenging as individuals exhibit a wide range of gait patterns across different age groups and gender (17).
Figure 2. Normal gait cycle (adapted from 1).
Table 1. Gait parameters for healthy individuals (1).
Gait disorders are typically associated with deficits in the brain, spinal cord, peripheral nerves, muscles, joints, or bones. Some medical conditions leading to pathological gait include but not limited to muscular dystrophy, myelodysplasia, cerebral palsy, arthritis, osteoarthritis, head injury, lower limb amputation, multiple sclerosis, rheumatoid, spinal cord injury, parkinsonism, and stroke (25).
In neuromuscular conditions, the loss of central control affects the motion. In general, patients walk slower than healthy individuals and with compromised spatiotemporal, kinematic, and kinetic parameters. In older adults, a walking speed decline of 0.7% per year is observed, along with significant changes in cadence and step length. The aging population also exhibits lower knee extension at heel-strike and knee flexion during the swing phase (23, 26). The following subsections describe some of the most common gait disorders and associated pathological parameters. The associated impacted parameters are summarized in Table 2.
Gait ailments associated with aging lead to reduction in the quality of life and increased morbidity and mortality. Elderly patients exhibit complex gait disorders, and their dual task ability deteriorates due to a decline in their central resources (23, 26).
Specific gait dysfunction noted in the elderly population are summarized as follows:
Shuffling with a reduced step height and stride length characterizes hypokinetic gait disorder (27). Reduced arm swing with slow turning movements is also present in isolation. Festination, when patients use rapid small steps to maintain the feet beneath the forward moving trunk, is also observed. Ataxic elements include broad stance width and an increased variability in timing and amplitude of steps (27). Gait associated with underlying diseases, such as Parkinson's disease, cerebrovascular disease, and ventricular widening, is classified within hypokinetic-rigid gait disorders (27, 28).
Defined as gait during which people move slowly with a wider base, and shorter stride, with minimal trunk movement, while the knees and elbows are bent. Whereas careless gait is when patients appear overly confident and walk insensitively fast. Careless gait is due to confusion and delirium associated with old age (27).
Patients with post-anoxic encephalopathy exhibit bouncing gait and stance. This is also observed in patients with Parkinson's disease-causing excessive trunk movements contributing to falls. Several dystonic patients are reported to walk on their toes (27, 28).
Gait dysfunction is common in elderly people due to adverse effects of drugs leading to extrapyramidal side-effects, sedation, orthostatic hypotension, behavioral abnormalities, or ataxia (27, 28).
Elderly people often exhibit fluctuating or episodic gait disorder after exercise due to fatigue, and it might be an indication of underlying vascular or neurogenic limping. Freezing gait is part of hypokinetic-rigid syndrome (27, 28).
PD is a neurological disorder which leads to cognition, where gait impairment deteriorates with disease progression, increasing reliance on cognition to control gait. Due to cognitive impairment with PD, the ability to compensate for gait disorders diminishes, leading to further gait impairment. PD is characterized by deficit in amplitude and gait speed, along with increased gait variability (29).
Neuropathy of motor, sensory, and autonomic components of the nervous system are one of the many complications of Type II Diabetes (T2D). An intact central and peripheral nervous system are essential to initiate and control healthy gait, along with sufficient muscle strength, bone, and joint movements in complete range for normal locomotion. Patients diagnosed with T2D take extra steps when walking in straight paths and during turns, along with an overall reduction in walking speed, step length, cadence, and fewer acceleration patterns as compared to age-matched healthy controls. Joint range of motion is also altered in T2D, where patients with diabetic peripheral neuropathy exhibit a reduced range of motion at the ankle joint in dorsi and plantar flexion and a reduced flexion and extension range of motion at the knee joint in both, as compared to non-diabetic people (24).
Hemiplegia after stroke contributes to significant reduction in gait performance. In stroke survivors, function of the cerebral cortex is usually impaired, whilst that of spinal cord is preserved. Dysfunction is typically demonstrated by a marked asymmetrical deficit. Decreased walking speed and cadence, in addition to longer gait cycle and double limb support as compared to healthy individuals. For hemiplegic stroke survivors, a reduced peak extension of the hip joint in late stance, varying peak lateral pelvis displacement, knee flexion and decreased plantarflexion of ankle at toe off are reported. The GRF (Ground Reaction Force) pattern is characterized as asymmetric, along with decreased amplitude of joint moments, at the lower limb joints on the paretic side (30).
Large deficits in gait speed (31), stride length (32, 33), sagittal hip range of motion (32, 33), hip abduction moment-coronal plane (31), and negligible changes in transverse plane hip range of motion (31), deficiency in single limb support time (31), are reported in patients post THA as compared to healthy controls. Peak hip extension is typically reduced, whereas peak hip flexion remains similar as compared to controls. In addition, peak hip abduction moment is reduced along with peak hip external rotation moment (34).
The use of observational gait analysis and subjective rating sales continues to be widespread in clinical settings, both as a diagnostic tool and as a prognostic measure, as previously mentioned. Although these techniques can be useful for the initial rudimentary evaluation of some gait parameters, the validity, reliability, specificity, and responsiveness of these qualitative methods are highly questionable. Researchers have therefore proposed various pathology-specific gait indices and summary measures (35) based on commercially available technologies with accepted levels of accuracy Table 3. summarizes the current clinical gait summary measures, discrete and continuous gait indices, and non-linear approaches reported in literature, along with advantages and disadvantages.
In the past couple of decades, remarkable technological advancement has been witnessed in the field of gait assessment and analysis, particularly in gait assessment technology. Instrumented walkways, both portable and non-portable, became a good alternative to complicated, bulky and non-portable traditional gait labs. These systems (for example the Walkway and StrideWay from Tekscan Inc., Boston, United States) are now widely used in research and to a limited extent in clinical practice. They typically include low-profile floor walkway systems equipped with grids of embedded sensors below the surface, which record foot-strike patterns as a function of time and space as an individual walks across the platform, and dedicated software which computes the various spatiotemporal gait measures Although these instrumented mats involve less setup time and are generally simple to operate as compared to traditional IGA labs, they are expensive, restrictive to specific operational environment to over-ground trials (36, 37).
Marker-based optical motion capture (Mocap) is another rapidly emerging technology effective for obtaining 3D kinematic movement data. Passive Mocap systems [e.g., Vicon (Vicon Motion Systems Ltd, Oxford, United Kingdom) and ELITE optoelectronic system (BTS S.p.A., Milano, Italy)], include retro-reflective markers (that reflect the light emitted by high-resolution infrared cameras) attached to specific anatomic landmarks. The location of the marker is identified by decoding the camera images. Here, the markers must be calibrated for identification before the recording session commences. Active Mocap systems (e.g., Optotrak motion capture system; Northern Digital Inc., Waterloo, Canada), on the other hand, use light-emitting diode (LED) markers (reflect their own light powered by a battery), which are automatically identified (38, 39). In the context of clinical relevance, although such systems yield extremely accurate reliable data, operational factors including infrastructure, non-portability, high cost, additional time required for initial set-up and calibration, operational complexity, and restrictions to indoor setup impose hurdles to their functional deployment in clinics and rehabilitation centers (84). Recently, more portable cost-effective alternatives, such as Microsoft Kinect (based on a depth sensor-based markerless motion capture solution) became the application of choice (40).
Optoelectronic systems (e.g., Optogait®, Microgate, Italy) have also been used to capture spatiotemporal gait parameters. These mainly consist of a transmitting and a receiving bar containing an infrared light. Interruptions of the communication between the emitter and receiver are detected by the system to calculate the various gait parameters (41).
An evolution in the measurement of gait kinetic parameters can also be witnessed in the last two decades. These parameters include ground reaction forces, and intersegmental joint reaction forces, moments, and powers. Instrumented walkways offer dynamic plantar pressure mapping but are expensive and do not provide joint kinetic data. Force plates are also used in various gait analysis studies (38, 39, 42). These are able to provide intersegmental joint reaction forces by using the ground reaction forces measured along with inverse dynamics models (Winters book) Chen et al. (93) developed a novel remote sensing technology called “Electrostatic Field Sensing (EFS)” for measuring human gait including stepping, walking, and running, and further extended the work to post-stroke gait. This technology is credited with several advantages, such as being non-contact, affordable, and allows long-time monitoring (43). Shoe insole systems represent another category of gait quantification tools and techniques. These systems are designed to allow for the recording of both dynamic plantar pressure and spatiotemporal data. F-scan (Tekscan Inc., Boston, United States) is an ultra-thin in-shoe pressure measurement system utilizing Force-Sensitive Resistive films (FSR) technology (44).
The characteristics of different measurement systems applicable to clinical settings are summarized in Table 4, and the pros and cons of these systems are listed in Table 5.
Computational pipeline using computer vision techniques has been proposed as an ecological and precise method to quantify gait in children with neurodevelopmental disorders, along with the pose estimation software to obtain whole-body gait synchrony and balance (45). Speed, arm swing, postural control, and smoothness (or roughness) of movement features of gait for Parkinson's patients were extracted using videos processed by ordinal random forest classification model. Significant correlation between clinician labels and model estimates was reported, which provides gait impairment severity assessment in Parkinson's disease using single patient video, thereby reducing the need for sophisticated gait equipment (46). Computer vision-based gait assessment tools promise frequent gait monitoring using minimal resources (46). Deep learning to detect human subject in 2D images and then combining 3D sensing data to measure gait features has proven to be more robust than depth cameras in gait parameter acquisition (47).
As previously mentioned, marker-based optoelectronic systems are currently the most widely used systems in IGA among both research and clinical communities. On the other hand, one of the main sources of error inherent to these systems is the degree of movement of the skin, muscle, and other soft tissues, or the so- called soft tissue artifacts (STA), under the markers in relation to bony landmarks, hence violating the rigid body assumption underlying these methods (48, 49). Moreover, STA varies by marker location in a unique and unpredictable manner, particularly during dynamic activities, which can make it unreliable for clinical applications (50).
Although not yet widespread in biomechanics, computer vision based markerless gait assessment methods offer a promising tool for gait assessment in research, as well clinical and sports biomechanics applications. By leveraging modern technologies, such as improved solvers, advanced image features and modern machine learning, markerless vision-based systems can reduce the required number of cameras, incorporating moving cameras, increasing the number of tracked individuals, and offering robust detection and fitting in diverse environments. On the other hand, issues such as accuracy and field-based feasibility remain to be addressed (51).
Three-dimensional imaging techniques have been successfully used to directly determine bone movements during walking as a gold reference standard to validate/improve current motion capture techniques (54). For example, researchers have resorted to quantifying STA by comparing with reference 3D kinematics of bone reconstructed from fluoroscopy-based tracking (53). Fluoroscopy has also emerged as a means for tracking position and orientation of underlying skeletal anatomy of the foot/ankle (54). Although single plane fluoroscopy yielded large errors when used to evaluate the accuracy of multi-segment foot models (49), dual fluoroscopy (DF) was found reliable and is considered as the current reference standard to compare joint angles (55). Combined with 2D/3D registration, video-fluoroscopy allows for accurate quantification of 3D joint motion free of STA (56). High-speed dual fluoroscopy (DF) has been reported to measure in-vivo bone motion of the foot and ankle with sub-millimeter and sub-degree errors (57). DF has also been used to evaluate multi-segment foot models and reported good agreement between DF and skin-marker data for the first metatarsal and sagittal plane measurements of the longitudinal arch (48).
Various researchers investigated the use of DF for clinical applications. In-vivo dual fluoroscopy was used to quantify the hip joint kinematics of patients with Femoroactabular impingement syndrome (FAIS) relative to asymptomatic, morphologically normal control participants during standing, level walking, incline walking and an unweighted functional activity. The kinematic position of the hip joint was obtained by registering projections of 3D computed Tomography models with DF images (58). Knee kinematic profiles were also obtained using 3D video-fluoroscopy and compared to actual and nominal flexion-extension, internal-external rotations, and antero-posterior translations profiles with optical mocap during stair climbing (59). Joint function for total talonavicular replacement after a complex articular fracture was evaluated using a full body gait analysis and 3D joint kinematics based on single-plane fluoroscopy (60). The 3D video fluoroscopic analysis was performed to assess joint motion of the replaced ankle (60). DF and CT imaging techniques were both employed to calculate in-vivo hip kinematics, along with model-based tracking, to compare the effect of different coordinate systems (61). Since marker-based systems are unable to accurately analyze talocrural or subtalar motion because the talus lacks palpable landmarks to place external markers (54), digitized video fluoroscopy was reportedly used to determine the sagittal plane motion of the medial longitudinal arch during dynamic gait (62). Characteristics of knee joint motion were also analyzed in 6DOF during treadmill walking using a dual fluoroscopy imaging system at different speeds (63).
DF uses anatomical landmarks visible on 3D CT reconstructions which substantially reduces errors due to STA (58). Computed tomography (CT) scans of participants are usually needed in DF to determine bone position from the DF images. Single plane fluoroscopy is restricted to 2D motion capture, while using a second FS allows for a full 3D analysis although a single gantry system has lower radiation than the biplane system with reported ionizing radiation levels of 10 µSv per trial (54). Stationary image intensifiers and static systems have a restricted field of view limiting their application to highly restricted movements (56). Moving fluoroscopes, consisting of a fluoroscopic unit mounted on a moving trolley which moves with the subject and is controlled by wire sensors to ensure that it remains in the field of view of the image intensifier (56), provide an enhanced field of view ideal for dynamic scenarios and moving joints.
Fluoroscopic systems designed for precise capture of bone movement and joint kinematics, unlike optical or inertial systems, are not yet commercially available, generally requiring in-house instrumentation and further performance evaluation. The evaluation would typically include determining the resolution of the hardware imaging chain, assessing how the hardware and software reduce or eliminate various distortions, and measuring static and dynamic accuracies and precisions based on precisely known motions and positions (64). Image quality is a major determinant of error in fluoroscopic applications (62). Pulse imaging of fluoroscopes, such as pulse width, limits image quality at a given frame rate. Increasing the pulse rate, which is function of pulse width, may add to radiation exposure, leading to an important tradeoff consideration between image quality and radiation exposure (63). Moving video-fluoroscopes reported lower gait velocity, step length, and cadence as compared to control conditions, indicating altered time distance parameters towards those of slow walking (56). So far, dynamic MRI used to define in-vivo talocrural and subtalar kinematics (65) does not allow data collection during normal gait.
Continued multidisciplinary collaborative efforts among biomechanists, imaging and computer vision experts, and clinicians are essential for fully leveraging these highly promising techniques in clinical applications.
Wearable technology – the use of body-worn sensors to measure the characteristics of human locomotion, has recently emerged as an efficient, convenient, and most importantly, inexpensive option to quantitative gait analysis for both clinical and research-based applications (Figure 3). In general, it uses individual sensor elements, such as accelerometers, gyroscopes, magneto resistive sensors, force/pressure sensors, goniometers, inclinometers, and electromyographic (EMG) sensors, or combined as an inertial measurement unit (IMU) (66). In comparison to conventional counterparts (e.g., walkway and camera based Mocap), wearable sensing enables continuous gait monitoring (> 2 h) outside the lab or clinic, allowing for replication of natural patterns of walking. Moreover, gait patterns over an ample distance could be measured as opposed to limited walking distance in a lab-based setting.
Accelerometers are often used in gait analysis for assessing stability and risk of fall. In a study which used a single tri-axial accelerometer mounted on the sacrum to analyze the risk of fall among 80 participants, accelerometry-based techniques were found to be able to detect subjects with increased risk of fall by employing appropriate machine learning techniques (66). In (67), a 3D accelerometer attached to the lower back was used for stability assessment of older adults. The applicability of a single accelerometer, worn on the back was further examined in (68), which highlights promising results for implementation in routine clinical practices. Considerable work has also been carried out to assess the consistency of gait characteristics obtained from accelerometers, where discrepancies in sensors positioning yield to critical errors (69). Furthermore, in (70), the authors have provided a comprehensive review on the use of accelerometry-based gait analysis techniques and their application to clinical settings.
Gyroscopes are also increasingly employed for gait studies. These devices measure angular velocity and are often combined with accelerometers and other micro-electromechanical systems (MEMS) devices to enhance performance through sensor fusion techniques. They have found applications in step detection, gait event detection, segmental kinematics, and more. For instance, a single gyroscope placed in the instep of the foot was successfully used to detect gait events, including heel strike, foot flat, heel off, and toe-off (71). Another study involved two gyroscopes, mounted on the lower left and right side of the waist to calculate walking steps and step length (72).
Magnetometers measure the magnetic field direction and intensity at a specific point. In combination with other inertial sensors (accelerometers and gyroscopes), they form a so-called inertial measurement unit (IMU), which can produce a drift-free estimation of gait parameters (73). Sophisticated commercialized IMUs (Physiolog 5 IMU, Gait Up, Switzerland, MTw Awinda, Xsens Technologies B.V., Netherlands), as well as in-house developed systems, were equally used for gait studies (74). In the context of human motion analysis, IMUs are employed for several possible goals, for example, to estimate the joint angles (74), to detect the risk of fall in an elderly population, long term monitoring of activities and symptoms (75), measurement of gait events, spatiotemporal parameters (76, 77, 78), ground reaction forces and moments (79), and estimation of gait symmetry (80). Mariani et al. (2010) used IMUs to measure foot kinematics in a study involving both young and elderly and reported the suitability of the system to clinical practice (81). Parisi et al. developed a low-cost system with a single IMU attached to the lower trunk to examine the gait characteristics of both hemiparetic and normal control subjects through measurement of spatiotemporal parameters, which showed excellent correlation with the parameters obtained from a standard reference system (78).
Insole systems for gait measurement and analysis represent a major category, which is cost-effective, portable, and applicable for both indoor and outdoor settings. Over the years, various technologies were developed (82), tested, and commercialized. These include capacitive sensors (Pedar system, Novel GmbH, Germany) (83), force-sensing resistors (FSR) (F-Scan, Tekscan Inc., United States) (84), and piezoresistive sensors (FlexiForce system, Tekscan, United States and ParoTec system, Paromed, Germany) (82). Researchers have adopted different approaches about the design, fabrication, and applications of insole systems. Both prefabricated and in-house fabricated insole systems have been tested for healthy and pathological gait (85, 86). Some studies have also integrated inertial measurement units (IMU) with shoe insoles to enhance their capabilities. Despite the fact that these shoe-based systems have successfully been used for various gait analysis applications, they suffer from some drawbacks, such as (i) distortion of the flexible contact surface due to repeated loading, which leads to changes in the sensor response, (ii) drift in the output due to prolonged load application that causes heat inside the shoe, and (iii) need for subject-specific calibration that may alter accuracy (87). Mancinelli et al. (2012) presented ActiveGait – a novel sensorized shoe system for real-time monitoring of gait deviations associated with Cerebral Palsy in children. They reported that the severity of gait deviations can be estimated with an accuracy greater than 80% using the features derived from the center of pressure trajectories gathered from the shoe system (88). In (87), the authors designed a novel flexible foot insole system using an optoelectronic sensing technology for monitoring plantar pressure deviations in real-time. The system consists of an array of 64 sensing elements and onboard electronics for signal processing and transmission. Experimental validation was conducted on healthy subjects while walking at self-selected slow and normal speed. A commercial force plate (AMTI, Watertown, United States) was used as a reference system for benchmarking. Jagos et al. (2017), on the other hand, developed the eSHOE, which consists of four FSR sensors, a three-axis accelerometer, and a three-axis gyroscope, and reported good agreement with the gait parameters obtained from the GAITRite mat (89). Various other studies have also examined the applicability of shoe-based systems for gait analysis (85, 90–92).
Another class of sensors that found major applications in gait studies is electromyography (EMG) sensors. Surface EMG is a non-invasive technique used to measure muscle activity. In (93), Lee et al. proposed a method using EMG signals to obtain biometrics from gait for personal identification methods. Another study adopted EMG techniques to understand the co-contraction patterns of thigh muscle during free walking using surface EMG (94). These research efforts emphasize the importance of wearable sensors in the study of human gait. The wearable systems discussed in this section are summarized in Table 6.
Although emerging new wearable technologies promise to enhance gait assessment and rehabilitation, there is limited research on the use of wearable technology to assess gait and mobility and its efficacy in clinical settings. According to a recently published review by Peters et. al. on the use of wearable technology to assess gait and mobility in stroke patients (95), most of the available studies are intervention studies conducted in laboratory settings that have used sensors to investigate change in cadence, step time variability, and gait speed. As wearable technologies continue to progress in affordability and accessibility, it is expected that such technologies would enable the gathering of movement-related data in “real-world” and various clinical settings. Importantly, these researchers indicated that so far only a limited number of studies examined reliability and validity of existing wearable devices, highlighting the need for more studies to examine psychometric and other properties when collecting gait and mobility information to determine which wearable technologies are most effective. Another recent review on the evaluation of the use of wearables in PD also indicates that novel technologies and wearables have the potential to enable early or differential diagnosis of PD, monitoring of motion state, prevention, or reduction of off-stage status, and assessing of movement complications. On the other hand, more research is required for the validation and the identification of more accurate markers of PD progression (96). Importantly, these authors warn that wearable devices may not be appropriate in cases of severe motor impairment, off-stage state, cognitive impairment, and for elderly patients and that further research is required for clinical validation.
Besides sensor technology, sensor fusion algorithms play a critical role in predicting the accuracy/precision of these wearable-based systems. Most of the research has focused mainly on gait feature detection, daily physical activity monitoring, and gait data classification targeting disease diagnosis and user recognition. These algorithms are based on different data mining and AI technology, including machine learning, fuzzy computing, wavelet transforms, genetic algorithms, and data fusions. Alaqtash et al. (97) developed an intelligent fuzzy computational algorithm for characterizing gait in healthy, as well as impaired subjects. McCamley et al. established a method to calculate initial and final contact of gait using continuous wavelet transforms, employing waist-mounted inertial sensors (98). Another study cited the use of a single accelerometer mounted at the lower trunk and a corresponding algorithm to identify gait spatiotemporal parameters (68). A real-time gait event detection algorithm was proposed in (99) making use of adaptive decision rules. Further in (100), an original signal processing algorithm is developed to extract heel strike, toe strike, heel-off, and toe-off from an accelerometer positioned on the feet.
A novel gyroscope only (GO) algorithm was proposed in (101) to calculate knee angle through the integration of gyroscope-derived knee angular velocity. A zero-angle update algorithm was implemented to eliminate drift in the integral value. In addition, published work on noise-zero crossing (NZC) gait phase algorithm was also adapted. This method is applicable for continuous monitoring of gait data. Nukala et al. used support vector machines (SVM), KNN, binary decision trees (BDT), and backpropagation artificial neural network (BP-ANN) to classify the gait of patients from normal subjects, where features extracted from raw signals from gyroscopes and accelerometers were used as inputs. This study reported the highest overall classification accuracy of 100% with BP-ANN, 98% with SVM, 96% with KNN, and 94% with BDT (102).
Li et al. proposed DTW algorithm, sample entropy method, and empirical mode decomposition to calculate 3 main gait features of post-stroke subjects: symmetry, complexity character, and stepping stability. A k-nearest neighbor (KNN) classifier trained on the acquired features showed a promising result (area under the curve (AUC) of 0.94), which suggests the feasibility of such techniques to automatic gait analysis systems (43). Rastegari et al. employed a feature selection technique called maximum information gain minimum correlation (MIGMC) to extract gait data of subjects with Parkinson's Disease (103). The performance of several machine learning classifiers, including Support Vector Machines, Random Forest, AdaBoost, Bagging, and Naïve Bayes were also assessed to test the power of the feature set obtained.
The use of novel computational platforms, including Machine Learning, Support Vector Machine, and Neural Network approaches, are increasingly commanding greater attention in gait and rehabilitation research. Although their use in clinical settings are not yet well leveraged, these tools promise a paradigm shift in stroke gait quantification and rehabilitation, as they provide means for acquiring, storing and analyzing multifactorial complex gait data, while capturing its non-linear dynamic variability and offering the invaluable benefits of predictive analytics (1). A recent review article discussed the potential value of ML in gait analysis towards quantification and rehabilitation (104). The authors concluded that further evidence is required although preliminary data demonstrates that the control strategies for gait rehabilitation benefit from reinforcement learning and (deep) neural-networks due to their ability to capture participants' variability. This review paper demonstrated the success of ML techniques in detecting gait disorders, predicting rehabilitation length, and control of rehabilitation devices. Further work is needed for verification in clinical settings.
Quantitative gait assessment is invaluable towards disease-specific and patient specific rehabilitation/therapeutic interventions. Spatiotemporal, kinematic, and kinetic parameters obtained during instrumented gait assessment can help clinicians benchmark, devise strategies, and evaluate the effect of various rehabilitation interventions. Gait disorders not only affect these parameters, and patterns and time spent in the various gait phases, but can also highly impact gait symmetry, and regularity, depending on the disease and severity (105). Increasing evidence supports a data-driven physical rehabilitation approach to the treatment of functional gait disturbance (106). There are multiple examples in literature on the effective use of quantitative gait measures towards more effective data-driven rehabilitation. A recent review by Biase et al. (107) studied the most relevant technologies used to evaluate gait features and the associated algorithms that have shown promise to aid diagnosis and symptom monitoring towards rehabilitation in Parkinson's disease (PD) patients. They reported physical kinematic features of pitch, roll and yaw rotations of the foot during walking, based on which feature extraction and classification techniques, such as principal component analysis (PCA) and support vector machines (SVM) method were used to classify the PD patients. They also used gait features, including step duration, rise and fall gradients of the swing phase, as well as standard deviation of the minima as quantitative measures, for benchmarking and monitoring PD motor status during rehabilitation. Interestingly, this review sheds light on need to change the evaluated gait features as a function of disease progression. Another study was Pistacchi et al. (108) suggested spatiotemporal gait parameters, such as speed and step length, where reduced step length seems to be a specific feature of Parkinson's disease gait particularly in early disease stages. On the other hand, asymmetry, step shuffling, double-limb support and increased cadence are more common in mild to moderate stages, while advanced stages are more frequent freezing of gait (FOG) and motor blocks, reduced balance and postural control, motor fluctuations and dyskinesia (109). Researchers have also investigated the evaluation of ambulatory systems for gait analysis post hip replacement (110). They found gait characteristics such as stride length and velocity, as well as thigh and shank rotations different from healthy individuals and recommended their use to monitor post-surgical rehabilitation efficacy. Spatiotemporal gait parameters, such as step length, width and cadence have been used (111) to assess the effect of swing resistance and assistance rehabilitation on gait symmetry in hemiplegic patients. Investigators have also studied whether specific variables measured routinely at a rehabilitation center were predictors of gait performance of hemiparetic stroke patients (112). They found that motor control and balance were the best predictors of gait performance. A recent review article on assessment methods of post stroke gait suggests that multiple spatiotemporal, kinematic, and kinetic parameters can be useful in diagnosing post-stroke gait dysfunction and as quantitative measures to evaluate rehabilitation outcomes (1). Spatiotemporal characteristics of post-stroke gait include reduced step or stride length, increased step length on the hemiparetic side, wider base of support, greater toe-out angle, reduced walking speed and cadence. Stride time, stance period on both lower limb, and double support time are also increased, in addition to less time in stance and more time in swing phase for the paretic side, as well as asymmetries in spatial and temporal factors. Kinematic parameters associated with hemiplegic gait (reduced mean peak extension of the hip joint in late stance, alterations in the lateral displacement of the pelvis and flexion of the knee, and decreased plantarflexion of the ankle at toe-off, in addition to a significant decrease in peak hip and knee flexion during the swing phase, reduced knee extension prior to initial contact, as well as decreased ankle dorsiflexion during swing), and kinetic parameters (asymmetric patterns, as well as decreased amplitudes of the joint moments and joint powers at the hip, knee, and ankle joints on the paretic side) can be used as quantitative means to design and evaluate effective rehabilitation (113–115). IGA has also been successfully used to quantify and improve gait dysfunction associated with ageing and assess the risk of falling (116). Spatiotemporal gait parameters such as velocity, swing time, stride length, stride time- and double support time variability, as well as heel strike and toe off angles, and foot clearance, have been suggested as plausible indicative quantitative measures (116) to assess the risk of falling in elderly subjects. Inertial sensor-equipped shoes additionally provided heel strike and toe off angles, and foot clearance (116). The study (117) summarizes that multi-component exercise therapy which consisted of strength, ROM exercise, balance, flexibility and stretching exercises, circuit exercise training, and gait training was found to enhance gait function for individuals suffering with diabetic peripheral neuropathy compared to control groups using spatiotemporal gait parameters like velocity, cadence, step length, step time, double support time, stride length, stride time, ankle ROM. Gait assessment has potential to develop patient training paradigms for overcoming gait disorders (111).
In recent decades, the healthcare field has witnessed a tremendous interest in the use of wearable sensing modalities and AI-driven data management/analysis techniques for patient diagnosis, monitoring, and rehabilitation. The portability, lightweight, ease of use, and high-power efficiency are some of the factors that promote applicability to a clinical platform.
There are few examples in literature demonstrating the potential success of using wearable-based systems for gait assessment in clinical settings. Prajapati et al. assessed the walking activity of inpatients with subacute stroke using commercial accelerometers attached above the ankle. They found that the walking bouts were shorter in duration and gait was more asymmetric (118). Studies have established test-retest reliability and accuracy of different sensor technologies; however, further validation trials are recommended prior to any clinical use. Hsu et al. assessed the test-retest reliability of an accelerometer-based system with infrared assist for measuring spatiotemporal parameters, including walking speed, step length, and cadence, as well as trunk control parameters, including gait symmetry, gait regularity, acceleration root mean square, and acceleration root mean square ratio of healthy subjects in hospital (119). This study showed excellent test-retest reliability of the parameters considered, and thus highlighting the reliability of an infrared assisted, trunk accelerometer-based device for clinical gait analysis. Another study investigated the concurrent validity and test-retest reliability of gait parameters (cadence, gait velocity, step time, step length, step time variability, and step time asymmetry) acquired from elderly subjects, using a tri-axial accelerometer attached to the center of body mass (120). In comparison to a reference GAITRite system, the acquired parameters showed good validity and reliability. Poitras et al. performed a systematic review of 42 studies assessing the reliability and validity of wearable sensors, specifically, IMUs, for quantifying the joint motion (121). Evidence suggests that IMU could be an alternative solution to an expensive motion capture system, as it shows good validity for lower-limb analysis involving fewer complex tasks. However, more work is needed to draw a better conclusion with regards to its reliability, as well as to standardize the protocol to get more accurate data in a clinical setting. Importantly, additional research efforts are needed to examine the responsiveness of wearables in free-living conditions in hospital settings.
This review aimed to summarize available published work on the present and future of gait analysis in clinical settings. The focus was to highlight current systems, scales, and indices, as well as recent technology-driven gait characterization and analysis approaches and their applicability to clinical settings. Within this context, pathological gait associated with different disease, as well as ageing was briefly discussed. As such, this article may have not covered the complete spectrum of gait pathologies and associated parameters. A scoping (non-systematic) search methodology was selected to broaden the scope and integration of the three main aspects of focus (gait pathology, clinical assessment, recent tools, and technologies). In addition, we do not recommend any specific protocol over the other, as most of the papers incorporate different inclusion/exclusion criteria for subject selection, as well as different sampling sizes, which may render comparisons unrealistic.
This scoping review aimed to shed light on the status of gait assessment in clinical settings, as well as the state-of-the-art emerging tools and technologies and their potential clinical applicability. Clinical gait analysis continues to rely mainly on observational gait and quantitative scales and is hence subjective and suffers from variability and the lack of sensitivity influenced by the observer's background and experience. Based on the reviewed literature, quantitative IGA-based gait analysis, commonly used in research labs, has the capability of providing clinicians with accurate and reliable gait data for informed diagnosis and continuous monitoring. On the other hand, several factors, including high cost and infrastructure challenges; data variability, complexity, and multidimensionality; lack of sufficient knowledge and standardized training in clinical environments; and time constraints, continue to limit its wide-spread deployment. Rapidly emerging smart wearable technology and AI, including Machine Learning, Support Vector Machine, and Neural Network approaches, are increasingly playing a bigger role in gait assessment. Although their use in clinical settings is not yet well leveraged, these tools promise an unprecedented paradigm shift in the quantification of gait in the clinic and beyond, as they provide means for acquiring, storing, and analyzing multifactorial complex gait data, while capturing its non-linear dynamic variability and offering the invaluable benefits of predictive analytics.
Researchers are also paying increased attention to multisource and multi-modality sensor fusion approaches, which can further add value by integrating the output of multiple sensors to capture the complexity and variability of gait. Multimodality sensor fusion also allows for simultaneous monitoring of various physiological signals during locomotion, such as EMG, ECG, and EEG, where fusing these with various gait measures (spatiotemporal, kinematic, and kinetic) can shed light on underlying health conditions and disease etiology towards better informed outcome prediction and clinical decisions. As the volume of data from the variety of sensors, including electroencephalography, electro-oculography, electro-cardiography, and electromyography, motion capture and force sensors data, substantially increases, more AI-driven sophisticated data management and modeling are needed to quantify and interpret complex network AI/NN models. Models which include static and dynamic features, combined with sophisticated data reduction and individualized feature selection of the most relevant gait characteristics are needed to close the loop for this paradigm shift. Future work is warranted on a multidisciplinary level: to validate the clinical applicability and integration of the various sensing modalities, to ensure proper synchronization of the various systems for accurate continuous real-time monitoring, to develop and validate fast and reliable computational platforms, and to implement modular user-friendly interfaces easy to use in any environment.
In summary, instrumented gait analysis is a well-established tool for the quantitative assessment of gait dysfunction which could be effectively used for functional diagnosis, treatment/surgery/rehabilitation/planning, and progression monitoring for a wide spectrum of disease. The literature indicates that recent advancement in wearable technology and computationally advanced data analytics, including AI, can overcome the challenges of traditional gait labs, allowing for less costly, portable, and relatively simple gait testing protocols in clinical settings, as well as user-friendly data management, analysis, and interpretation computational platforms. On the other hand, the development of clinically driven standardized methodology and procedures is of paramount significance and remains largely unaddressed. These standardized practices should not only focus on quantitative gait diagnosis but should also incorporate sophisticated objective measures and 3-D dynamic gait profiles and markers for monitoring progress and outcome prediction and evaluation. Proper gait protocols should be devised and leveraged towards identifying gait characteristics that could be effectively used as early disease diagnostic markers. Importantly, training clinical teams at various levels, from doctors and surgeons to physiotherapists and other allied health professionals, on properly using these novel assessment and computational tools is equally important and warrants an equally rapid paradigm shift in training and practice in clinical settings towards patient-specific precise medicine.
AAH, MR and KK conceived the idea. AAH, DMM, KK and MR formulated the objective for this review. AAH designed the search strategy, conducted abstract screening and full text review, extracted the data, and drafted the manuscript. KK, NA, DMM, and AAH contributed to writing the manuscript. DMM and NA performed a part of the literature survey, including abstract screening, full text review, and data extraction. MR, and KK provided significant guidance on the content of the manuscript, overall supervision, and critical feedback. All authors contributed to the article and approved the submitted version.
This publication is based upon work supported by the HEIC at Khalifa University of Science and Technology.
The authors declare that the research was conducted in the absence of any commercial or financial relationships that could be construed as a potential conflict of interest.
All claims expressed in this article are solely those of the authors and do not necessarily represent those of their affiliated organizations, or those of the publisher, the editors and the reviewers. Any product that may be evaluated in this article, or claim that may be made by its manufacturer, is not guaranteed or endorsed by the publisher.
1. Mohan DM, Khandoker AH, Wasti SA, Ismail Ibrahim Ismail Alali S, Jelinek HF, Khalaf K. Assessment methods of post-stroke gait: a scoping review of technology-driven approaches to gait characterization and analysis. Front Neurol. (2021) 12:650024. doi: 10.3389/fneur.2021.650024
2. Celik Y, Stuart S, Woo WL, Godfrey A. Gait analysis in neurological populations: progression in the use of wearables. Med Eng Phys. (2021) 87:9–29. doi: 10.1016/j.medengphy.2020.11.005
3. Rathinam C, Bateman A, Peirson J, Skinner J. Observational gait assessment tools in paediatrics–a systematic review. Gait Posture. (2014) 40:279–85. S0966-6362(14)00478-0 24798609
4. Eastlack ME, Arvidson J, Snyder-Mackler L, Danoff JV, McGarvey CL. Interrater reliability of videotaped observational gait-analysis assessments. Phys Ther. (1991) 71:465–72. doi: 10.1093/ptj/71.6.465
5. Hebda-Boon A, Zhang B, Amankwah A, Shortland AP, Morrissey D. Clinicians’ experiences of instrumented gait analysis in management of patients with cerebral palsy: a qualitative study. Phys Occup Ther Pediatr. (2022) 42(4):403–15. doi: 10.1080/01942638.2022.2037808
6. Toro B, Nester C, Farren P. A review of observational gait assessment in clinical practice. null. (2003) 19:137–49. doi: 10.1080/09593980307964
7. Franki I, Desloovere K, De Cat J, Feys H, Molenaers G, Calders P, et al. The evidence-base for basic physical therapy techniques targeting lower limb function in children with cerebral palsy: a systematic review using the international classification of functioning, disability and health as a conceptual framework. J Rehabil Med. (2012) 44:385–95. doi: 10.2340/16501977-0983
8. Wallmann HW. Introduction to observational gait analysis. Home Health Care Manag Pract. (2009) 22:66–8. doi: 10.1177/1084822309343277
9. Ferrarello F, Bianchi VA, Baccini M, Rubbieri G, Mossello E, Cavallini MC, et al. Tools for observational gait analysis in patients with stroke: a systematic review. Phys Ther. (2013) 93:1673–85. doi: 10.2522/ptj.20120344
10. Turani N, Kemiksizoğlu A, Karataş M, Ozker R. Assessment of hemiplegic gait using the Wisconsin gait scale. Scand J Caring Sci. (2004) 18:103–8. doi: 10.1111/j.1471-6712.2004.00262.x
11. Dasgupta P, VanSwearingen J, Godfrey A, Redfern M, Montero-Odasso M, Sejdic E. Acceleration gait measures as proxies for motor skill of walking: a narrative review. IEEE Trans Neural Syst Rehabil Eng. (2021) 29:249–61. doi: 10.1109/TNSRE.2020.3044260
12. Noseworthy JH. Clinical scoring methods for multiple sclerosis. Ann Neurol. (1994) 36:S80–5. doi: 10.1002/ana.410360718
13. Noseworthy JH, Vandervoort MK, Wong CJ, Ebers GC. Interrater variability with the expanded disability Status scale (EDSS) and functional systems (FS) in a multiple sclerosis clinical trial. The Canadian cooperation MS study group. Neurol. (1990) 40:971–5. doi: 10.1212/wnl.40.6.971
14. Roxburgh RH, Seaman SR, Masterman T, Hensiek AE, Sawcer SJ, Vukusic S, et al. Multiple sclerosis severity score: using disability and disease duration to rate disease severity. Neurol. (2005) 64:1144–51. doi: 10.1212/01.WNL.0000156155.19270.F8
15. Vienne-Jumeau A, Quijoux F, Vidal PP, Ricard D. Value of gait analysis for measuring disease severity using inertial sensors in patients with multiple sclerosis: protocol for a systematic review and meta-analysis. Syst Rev. (2019) 8:15. doi: 10.1186/s13643-018-0918-z
16. Balaji E, Brindha D, Elumalai VK, Umesh K. Data-driven gait analysis for diagnosis and severity rating of Parkinson's Disease. Med Eng Phys. (2021) 91:54–64. S1350-4533(21)00028-X 34074466
17. Mohan DM, Khandoker AH, Wasti SA, Ismail Ibrahim Ismail Alali S, Jelinek HF, Khalaf K. Assessment methods of post-stroke gait: a scoping review of technology-driven approaches to gait characterization and Analysis. Front Neurol. (2021) 12:650024. doi: 10.3389/fneur.2021.650024
18. Cappozzo A. Gait analysis methodology. Hum Mov Sci. (1984) 3:27–50. doi: 10.1016/0167-9457(84)90004-6
19. Wren TAL, Tucker CA, Rethlefsen SA, Gorton GE 3rd, Õunpuu S. Clinical efficacy of instrumented gait analysis: systematic review 2020 update. Gait Posture. (2020) 80:274–9. S0966-6362(20)30181-8 32563727
20. Saboor A, Kask T, Kuusik A, Alam MM, Le Moullec Y, Niazi IK, et al. Latest research trends in gait analysis using wearable sensors and machine learning: a systematic review. IEEE Access. (2020) 8:167830–64. doi: 10.1109/ACCESS.2020.3022818
21. Munn Z, Peters MDJ, Stern C, Tufanaru C, McArthur A, Aromataris E. Systematic review or scoping review? Guidance for authors when choosing between a systematic or scoping review approach. BMC Med Res Methodol. (2018) 18:143. doi: 10.1186/s12874-018-0611-x
22. Winter DA. The biomechanics and motor control of humna gait: Normal, elderly and pathological. Waterloo: Ont.: Waterloo Biomechanics (1991).
23. Ali A, Sundaraj K, Ahmad B, Ahamed N, Islam A. Gait disorder rehabilitation using vision and non-vision based sensors: a systematic review. Bosnian Journal of Basic Medical Sciences. (2012) 12:193–202. doi: 10.17305/bjbms.2012.2484
24. Alam U, Riley DR, Jugdey RS, Azmi SS, Rajbhandari SS, D’août K, et al. Diabetic neuropathy and gait: a review. Diabetes Ther. (2017) 8:1253. doi: 10.1007/s13300-017-0295-y
25. Whittle MW. Clinical gait analysis: a review. Hum Mov Sci. (1996) 15:369–87. doi: 10.1016/0167-9457(96)00006-1
26. Fukuchi CA, Fukuchi RK, Duarte M. Effects of walking speed on gait biomechanics in healthy participants: a systematic review and meta-analysis. Syst Rev. (2019) 8:153. doi: 10.1186/s13643-019-1063-z
27. Snijders AH, van de Warrenburg BP, Giladi N, Bloem BR. Neurological gait disorders in elderly people: clinical approach and classification. Lancet Neurol. (2007) 6:63–74. S1474-4422(06)70678-0 [pii].17166803
28. Snijders AH, van de Warrenburg BP, Giladi N, Bloem BR. Neurological gait disorders in elderly people: clinical approach and classifi cation. Lancet Neurol. (2007) 6(1):63–74. doi: 10.1016/S1474-4422(06)70678-0
29. Intzandt B, Beck EN, Silveira CRA. The effects of exercise on cognition and gait in Parkinson's Disease: a scoping review. Neurosci Biobehav Rev. (2018) 95:136–69. doi: 10.1016/j.neubiorev.2018.09.018
30. Belda-Lois J, Mena-del Horno S, Bermejo-Bosch I, Moreno JC, Pons JL, Farina D, et al. Rehabilitation of gait after stroke: a review towards a top-down approach. J Neuroeng Rehabil. (2011) 8:66. doi: 10.1186/1743-0003-8-66
31. Bahl JS, Nelson MJ, Taylor M, Solomon LB, Arnold JB, Thewlis D. Biomechanical changes and recovery of gait function after total hip arthroplasty for osteoarthritis: a systematic review and meta-analysis. Osteoarthritis Cartilage. (2018) 26:847–63. S1063-4584(18)31013-6 [pii].29474993
32. Ewen AM, Stewart S, St Clair Gibson A, Kashyap SN, Caplan N. Post-operative gait analysis in total hip replacement patients—a review of current literature and meta-analysis. Gait Posture. (2012) 36:1–6. doi: 10.1016/j.gaitpost.2011.12.024
33. Kolk S, Minten MJ, van Bon GE, Rijnen WH, Geurts AC, Verdonschot N, et al. Gait and gait-related activities of daily living after total hip arthroplasty: a systematic review. Clin Biomech (Bristol, Avon). (2014) 29:705–18. S0268-0033(14)00127-2 [pii].24951319
34. Kolk S, Minten MJM, van Bon GEA, Rijnen WH, Geurts ACH, Verdonschot N, et al. Gait and gait-related activities of daily living after total hip arthroplasty: a systematic review. Clin Biomech. (2014) 29:705–18. doi: 10.1016/j.clinbiomech.2014.05.008
35. Ladha C, Din S, Nazarpour K, Hickey A, Morris R, Catt M, et al. Toward a low-cost gait analysis system for clinical and free-living assessment. Annu Int Conf IEEE Eng Med Biol Soc. (2016) 2016:1874–7. doi: 10.1109/EMBC.2016.7591086. PMID: 28268692
36. Patterson KK, Gage WH, Brooks D, Black SE, McIlroy WE. Evaluation of gait symmetry after stroke: a comparison of current methods and recommendations for standardization. Gait Posture. (2010) 31:241–6. doi: 10.1016/j.gaitpost.2009.10.014
37. Patterson KK, Gage WH, Brooks D, Black SE, McIlroy WE. Changes in gait symmetry and velocity after stroke: a cross-sectional study from weeks to years after stroke. Neurorehabil Neural Repair. (2010) 24:783–90. doi: 10.1177/1545968310372091
38. Laudanski A, Brouwer B, Li Q. Measurement of lower limb joint kinematics using inertial sensors during stair ascent and descent in healthy older adults and stroke survivors. J Healthc Eng. (2013) 4:555–76. doi: 10.1260/2040-2295.4.4.555
39. Rastegarpanah A, Scone T, Saadat M, Rastegarpanah M, Taylor SJ, Sadeghein N. Targeting effect on gait parameters in healthy individuals and post-stroke hemiparetic individuals. J Rehabil Assist Technol Eng. (2018) 5:2055668318766710. doi: 10.1177/2055668318766710
40. Latorre J, Colomer C, Alcañiz M, Llorens R. Gait analysis with the kinect v2: normative study with healthy individuals and comprehensive study of its sensitivity, validity, and reliability in individuals with stroke. J Neuroeng Rehabil. (2019) 16:97. doi: 10.1186/s12984-019-0568-y
41. Iosa M, Bini F, Marinozzi F, Fusco A, Morone G, Koch G, et al. Stability and harmony of gait in patients with subacute stroke. J Med Biol Eng. (2016) 36:635–43. doi: 10.1007/s40846-016-0178-0 [pii].27853414
42. Sousa ASP, Silva A, Santos R, Sousa F, Tavares JMRS. Interlimb coordination during the stance phase of gait in subjects with stroke. Arch Phys Med Rehabil. (2013) 94:2515–22. S0003-9993(13)00537-6 [pii].23871877
43. Li M, Tian S, Sun L, Chen X. Gait analysis for post-stroke hemiparetic patient by multi-features fusion method. Sens (Basel). (2019) 19(7):1737. doi: 10.3390/s19071737
44. Krishnan V, Khoo I, Marayong P, DeMars K, Cormack J. Gait training in chronic stroke using walk-even feedback device: a pilot study. Neurosci J. (2016) 2016:1–8. doi: 10.1155/2016/6808319
45. Ardalan A, Yamane N, Rao AK, Montes J, Goldman S. Analysis of gait synchrony and balance in neurodevelopmental disorders using computer vision techniques. Health Informatics J. (2021) 27:14604582211055650. doi: 10.1177/14604582211055650
46. Rupprechter S, Morinan G, Peng Y, Foltynie T, Sibley K, Weil RS, et al. A clinically interpretable computer-vision based method for quantifying gait in Parkinson's Disease. Sens (Basel). (2021) 21:5437. doi: 10.3390/s21165437
47. Li Y, Zhang P, Zhang Y, Miyazaki K. 2019 41st annual international conference of the IEEE engineering in medicine and biology society (EMBC). Gait analysis using stereo camera in daily environment (2019). p. 1471–5
48. Kessler SE, Rainbow MJ, Lichtwark GA, Cresswell AG, D'Andrea SE, Konow N, et al. A direct comparison of biplanar videoradiography and optical motion capture for foot and ankle kinematics. Front Bioeng Biotechnol. (2019) 7:199. doi: 10.3389/fbioe.2019.00199
49. Tranberg R, Karlsson D. The relative skin movement of the foot: a 2-D roentgen photogrammetry study. Clin Biomech (Bristol, Avon). (1998) 13:71–6. S0268003397000521 [pii].11415773
50. Nester C, Jones RK, Liu A, Howard D, Lundberg A, Arndt A, et al. Foot kinematics during walking measured using bone and surface mounted markers. J Biomech. (2007) 40:3412–23. doi: 10.1016/j.jbiomech.2007.05.019
51. Colyer SL, Evans M, Cosker DP, Salo AIT. A review of the evolution of vision-based motion analysis and the integration of advanced computer vision methods towards developing a markerless system. Sports Med Open. (2018) 4:24. doi: 10.1186/s40798-018-0139-y
52. Baker R. Gait analysis methods in rehabilitation. J Neuroeng Rehabil. (2006) 3:4. doi: 10.1186/1743-0003-3-4
53. Stagni R, Fantozzi S, Cappello A, Leardini A. Quantification of soft tissue artefact in motion analysis by combining 3D fluoroscopy and stereophotogrammetry: a study on two subjects. Clin Biomech (Bristol, Avon). (2005) 20:320–9. doi: 10.1016/j.clinbiomech.2004.11.012
54. McHenry BD, Exten E, Long JT, Harris GF. Sagittal fluoroscopy for the assessment of hindfoot kinematics. J Biomech Eng. (2016) 138:4032445. doi: 10.1115/1.4032445
55. Roach KE, Foreman KB, MacWilliams BA, Karpos K, Nichols J, Anderson AE. The modified shriners hospitals for children Greenville (mSHCG) multi-segment foot model provides clinically acceptable measurements of ankle and midfoot angles: a dual fluoroscopy study. Gait Posture. (2021) 85:258–65. doi: 10.1016/j.gaitpost.2021.02.004
56. Hitz M, Schütz P, Angst M, Taylor WR, List R. Influence of the moving fluoroscope on gait patterns. PLoS One. (2018) 13:e0200608. doi: 10.1371/journal.pone.0200608
57. Roach KE, Wang B, Kapron AL, Fiorentino NM, Saltzman CL, Bo Foreman K, et al. In vivo kinematics of the tibiotalar and subtalar joints in asymptomatic subjects: a high-speed dual fluoroscopy study. J Biomech Eng. (2016) 138:910061. doi: 10.1115/1.4034263
58. Atkins PR, Fiorentino NM, Hartle JA, Aoki SK, Peters CL, Foreman KB, et al. In vivo pelvic and hip joint kinematics in patients with cam femoroacetabular impingement syndrome: a dual fluoroscopy study. J Orthop Res. (2020) 38:823–33. doi: 10.1002/jor.24509
59. Battaglia S, Belvedere C, Jaber SA, Affatato S, D'Angeli V, Leardini A. A new protocol from real joint motion data for wear simulation in total knee arthroplasty: stair climbing. Med Eng Phys. (2014) 36:1605–10. doi: 10.1016/j.medengphy.2014.08.010
60. Belvedere C, Cadossi M, Mazzotti A, Giannini S, Leardini A. Fluoroscopic and gait analyses for the functional performance of a custom-made total talonavicular replacement. J Foot Ankle Surg. (2017) 56:836–44. doi: 10.1053/j.jfas.2017.02.004
61. Uemura K, Atkins PR, Anderson AE. The effect of using different coordinate systems on in-vivo hip angles can be estimated from computed tomography images. J Biomech. (2019) 95:109318. doi: 10.1016/j.jbiomech.2019.109318
62. Wearing SC, Urry S, Perlman P, Smeathers J, Dubois P. Sagittal plane motion of the human arch during gait: a videofluoroscopic analysis. Foot Ankle Int. (1998) 19:738–42. doi: 10.1177/107110079801901105
63. Li G, Kozanek M, Hosseini A, Liu F, Van de Velde SK, Rubash HE. New fluoroscopic imaging technique for investigation of 6DOF knee kinematics during treadmill gait. J Orthop Surg Res. (2009) 4:6. doi: 10.1186/1749-799X-4-6
64. Iaquinto JM, Tsai R, Haynor DR, Fassbind MJ, Sangeorzan BJ, Ledoux WR. Marker-based validation of a biplane fluoroscopy system for quantifying foot kinematics. Med Eng Phys. (2014) 36:391–6. doi: 10.1016/j.medengphy.2013.08.013
65. Sheehan FT, Seisler AR, Siegel KL. In vivo talocrural and subtalar kinematics: a non-invasive 3D dynamic MRI study. Foot Ankle Int. (2007) 28:323–35. doi: 10.3113/FAI.2007.0323
66. Tao W, Liu T, Zheng R, Feng H. Gait analysis using wearable sensors. Sens (Basel, Switzerland). (2012) 12:2255–83. doi: 10.3390/s120202255
67. Ihlen EAF, Weiss A, Beck Y, Helbostad JL, Hausdorff JM. A comparison study of local dynamic stability measures of daily life walking in older adult community-dwelling fallers and non-fallers. J Biomech. (2016) 49:1498–503. doi: 10.1016/j.jbiomech.2016.03.019
68. Bugané F, Benedetti MG, Casadio G, Attala S, Biagi F, Manca M, et al. Estimation of spatial-temporal gait parameters in level walking based on a single accelerometer: validation on Normal subjects by standard gait analysis. Comput Methods Programs Biomed. (2012) 108:129–37. doi: 10.1016/j.cmpb.2012.02.003
69. Rispens SM, Pijnappels M, van Schooten KS, Beek PJ, Daffertshofer A, van Dieën JH. Consistency of gait characteristics as determined from acceleration data collected at different trunk locations. Gait Posture. (2014) 40:187–92. doi: 10.1016/j.gaitpost.2014.03.182
70. Jarchi D, Pope J, Lee TKM, Tamjidi L, Mirzaei A, Sanei S. A review on accelerometry-based gait analysis and emerging clinical applications. IEEE Rev Biomed Eng. (2018) 11:177–94. doi: 10.1109/RBME.2018.2807182
71. Felix P, Figueiredo J, Santos C, Moreno J. ADAPTIVE REAL-TIME TOOL FOR HUMAN GAIT EVENT DETECTION USING A WEARABLE GYROSCOPE. (2017). 653 p.
72. Nasr A, Nadeem T. A Novel Technique for Gait Analysis Using Two Waist Mounted Gyroscopes. In: Anonymous - 2019 IEEE Global Communications Conference (GLOBECOM); (2019). p. 1-6.
73. Kun L, Inoue Y, Shibata K, Enguo C. Ambulatory estimation of knee-joint kinematics in anatomical coordinate system using accelerometers and magnetometers. IEEE Trans on Biomed Eng. (2011) 58:435–42. doi: 10.1109/TBME.2010.2089454
74. Rodríguez-Martín D, Pérez-López C, Samà A, Cabestany J, Català A. A wearable inertial measurement unit for long-term monitoring in the dependency care area. Sens. (2013) 13(10):14079–104. doi: 10.3390/s131014079
75. Seel T, Raisch J, Schauer T. IMU-Based Joint angle measurement for gait analysis. Sens. (2014) 14(4):6891–909. doi: 10.3390/s140406891
76. Teufl W, Lorenz M, Miezal M, Taetz B, Fröhlich M, Bleser G. Towards inertial sensor based Mobile gait analysis: event-detection and spatio-temporal parameters. Sens. (2019) 19(1):38. doi: 10.3390/s19010038
77. Hwang T-H, Reh J, Effenberg AO, Blume H. Real-Time gait analysis using a single head-worn inertial measurement unit. IEEE Trans on Cons Elec. (2018) 64:240–8. doi: 10.1109/TCE.2018.2843289
78. Parisi F, Ferrari G, Baricich A, D'Innocenzo M, Cisari C, Mauro A. Accurate gait analysis in post-stroke patients using a single inertial measurement unit. In: Anonymous - 2016 IEEE 13th International Conference on Wearable and Implantable Body Sensor Networks (BSN); (2016). p. 335-340).
79. Karatsidis A, Bellusci G, Schepers HM, De Zee M, Andersen MS, Veltink PH. Estimation of ground reaction forces and moments during gait using only inertial motion capture. Senss. (2017) 17(1):75. doi: 10.3390/s17010075
80. Sant’ Anna A, Wickström N, Eklund H, Zügner R, Tranberg R. Assessment of gait symmetry and gait normality using inertial sensors: in-lab and in-situ evaluation. Berlin, Heidelberg: Springer (2013).
81. Mariani B, Hoskovec C, Rochat S, Büla C, Penders J, Aminian K. 3D Gait assessment in young and elderly subjects using foot-worn inertial sensors. J Biomech. (2010) 43:2999–3006. doi: 10.1016/j.jbiomech.2010.07.003
82. Razak AHA, Zayegh A, Begg RK, Wahab Y. Foot plantar pressure measurement system: a review. Sens (Basel, Switzerland). (2012) 12:9884–912. doi: 10.3390/s120709884
83. Novel® Pedar® System [Internet]. []. Available at: https://www.novel.de/products/pedar/
85. Leitch KM, Birmingham TB, Jones IC, Giffin JR, Jenkyn TR. In-shoe plantar pressure measurements for patients with knee osteoarthritis: reliability and effects of lateral heel wedges. Gait Posture. (2011) 34:391–6. doi: 10.1016/j.gaitpost.2011.06.008
86. Malvade PS, Joshi AK, Madhe SP. In-sole Shoe Foot Pressure Monitoring for Gait Analysis. In: Anonymous - 2017 International Conference on Computing, Communication, Control and Automation (ICCUBEA); (2017). p. 1-4.
87. Crea S, Donati M, De Rossi S, Marco Maria Oddo CM, Vitiello N. A wireless flexible sensorized insole for gait analysis. Sens (Basel, Switzerland). (2014) 14:1073–93. doi: 10.3390/s140101073
88. Mancinelli C, Patel S, Deming LC, Nimec D, Chu JJ, Beckwith J, et al. A novel sensorized shoe system to classify gait severity in children with cerebral palsy. Annu Int Conf IEEE Eng Med Biol Soc. (2012) 2012:5010–3. doi: 10.1109/EMBC.2012.6347118
89. Jagos H, Pils K, Haller M, Wassermann C, Chhatwal C, Rafolt D, et al. Mobile gait analysis via eSHOEs instrumented shoe insoles: a pilot study for validation against the gold standard GAITRite(®). J Med Eng Technol. (2017) 41:375–86. doi: 10.1080/03091902.2017.1320434
90. Arafsha F, Hanna C, Aboualmagd A, Fraser S, El Saddik A. Instrumented wireless SmartInsole system for Mobile gait analysis: a validation pilot study with tekscan strideway. J Sens and Actuator Netw. (2018) 7(3):36. doi: 10.3390/jsan7030036
91. Nagano H, Begg RK. Shoe-Insole technology for injury prevention in walking. Sens (Basel). (2018) 18:1468. doi: 10.3390/s18051468
92. Lin F, Wang A, Zhuang Y, Tomita MR, Xu W. Smart insole: a wearable sensor device for unobtrusive gait monitoring in daily life. IEEE Trans on Indust Informat. (2016) 12:2281–91. doi: 10.1109/TII.2016.2585643
93. Lee M, Ryu J, Youn I. Biometric personal identification based on gait analysis using surface EMG signals. In: Anonymous - 2017 2nd IEEE International Conference on Computational Intelligence and Applications (ICCIA); (2017). p. 318-321).
94. Strazza A, Mengarelli A, Fioretti S, Burattini L, Agostini V, Knaflitz M, et al. Surface-EMG analysis for the quantification of thigh muscle dynamic co-contractions during Normal gait. Gait Posture. (2017) 51:228–33. doi: 10.1016/j.gaitpost.2016.11.003
95. Peters D, O’Brien E, Kamrud K, Roberts S, Rooney T, Thibodeau K, et al. Utilization of wearable technology to assess gait and mobility post-stroke: a systematic review. J Neuroeng Rehabil. (2021) 18(1):67. doi: 10.1186/s12984-021-00863-x
96. Lu R, Xu Y, Li X, Fan Y, Zeng W, Tan Y, et al. Evaluation of wearable sensor devices in Parkinson's Disease: a review of current Status and future prospects. Parkinson's Disease. (2020) 2020:4693019. doi: 10.1155/2020/4693019
97. Alaqtash M, Yu H, Brower R, Abdelgawad A, Sarkodie-Gyan T. Application of wearable sensors for human gait analysis using fuzzy computational algorithm. Eng Appl Artif Intell. (2011) 24:1018–25. doi: 10.1016/j.engappai.2011.04.010
98. McCamley J, Donati M, Grimpampi E, Mazzà C. An enhanced estimate of initial contact and final contact instants of time using lower trunk inertial sensor data. Gait Posture. (2012) 36:316–8. doi: 10.1016/j.gaitpost.2012.02.019
99. Felix P, Figueiredo J, Santos C, Moreno J. ADAPTIVE REAL-TIME TOOL FOR HUMAN GAIT EVENT DETECTION USING A WEARABLE GYROSCOPE. (2017). 653 p).
100. Boutaayamou M, Schwartz C, Stamatakis J, Denoël V, Maquet D, Forthomme B, et al. Development and validation of an accelerometer-based method for quantifying gait events. Med Eng Phys. (2015) 37:226–32. doi: 10.1016/j.medengphy.2015.01.001
101. Allseits E, Kim KJ, Bennett C, Gailey R, Gaunaurd I, Agrawal V. A novel method for estimating knee angle using two leg-mounted gyroscopes for continuous monitoring with Mobile health devices. Sens (Basel). (2018) 18:2759. doi: 10.3390/s18092759
102. Nukala BT, Nakano T, Rodriguez A, Tsay J, Lopez J, Nguyen TQ, et al. Real-Time classification of patients with balance disorders vs. Normal subjects using a low-cost small wireless wearable gait sensor. Biosens. (2016) 6(4):58. doi: 10.3390/bios6040058
103. Rastegari E, Azizian S, Ali H. Machine Learning and Similarity Network Approaches to Support Automatic Classification of Parkinson's Diseases Using Accelerometer-based Gait Analysis. (2019).).
104. Khera P, Kumar N. Role of machine learning in gait analysis: a review. J Med Eng Technol. (2020) 44:441–67. doi: 10.1080/03091902.2020.1822940
105. Zhao H, Wang Z, Qiu S, Shen Y, Wang J. IMU-based gait analysis for rehabilitation assessment of patients with gait disorders. In: Anonymous (2017). 622–6.
106. Butz C, Iske C, Truba N, Trott K. Treatment of functional gait abnormality in a rehabilitation setting: emphasizing the physical interventions for treating the whole child. Innov Clin Neurosci. (2019) 16:18–21.31832259
107. di Biase L, Di Santo A, Caminiti ML, De Liso A, Shah SA, Ricci L, et al. Gait analysis in Parkinson's Disease: an overview of the most accurate markers for diagnosis and symptoms monitoring. Sens (Basel). (2020) 20:3529. doi: 10.3390/s20123529
108. Pistacchi M, Gioulis M, Sanson F, De Giovannini E, Filippi G, Rossetto F, et al. Gait analysis and clinical correlations in early Parkinson's Disease. Funct Neurol. (2017) 32:28–34. doi: 10.11138/FNeur/2017.32.1.028
109. Mirelman A, Bonato P, Camicioli R, Ellis TD, Giladi N, Hamilton JL, et al. Gait impairments in Parkinson's Disease. Lancet Neurol. (2019) 18:697–708. doi: 10.1016/S1474-4422(19)30044-4
110. Aminian K, Trevisan C, Najafi B, Dejnabadi H, Frigo C, Pavan E, et al. Evaluation of an ambulatory system for gait analysis in hip osteoarthritis and after total hip replacement. Gait Posture. (2004) 20:102–7. doi: 10.1016/S0966-6362(03)00093-6
111. Yen SC, Schmit BD, Wu M. Using swing resistance and assistance to improve gait symmetry in individuals post-stroke. Hum Mov Sci. (2015) 42:212–24. doi: 10.1016/j.humov.2015.05.010
112. Bohannon RW. Gait performance of hemiparetic stroke patients: selected variables. Arch Phys Med Rehabil. (1987) 68:777–81.3675175
113. Nadeau S, Betschart M, Bethoux F. Gait analysis for poststroke rehabilitation: the relevance of biomechanical analysis and the impact of gait speed. Phys Med Rehabil Clin N Am. (2013) 24:265–76. doi: 10.1016/j.pmr.2012.11.007
114. Moseley A, Wales A, Herbert R, Schurr K, Moore S. Observation and analysis of hemiplegic gait: stance phase. Aust J Physiother. (1993) 39:259–67. doi: 10.1016/S0004-9514(14)60486-4
115. von Schroeder HP, Coutts RD, Lyden PD, Billings E Jr, Nickel VL. Gait parameters following stroke: a practical assessment. J Rehabil Res Dev. (1995) 32:25–31.7760264
116. Schülein S, Barth J, Rampp A, Rupprecht R, Eskofier BM, Winkler J, et al. Instrumented gait analysis: a measure of gait improvement by a wheeled walker in hospitalized geriatric patients. J Neuroeng Rehabil. (2017) 14:18. doi: 10.1186/s12984-017-0228-z
117. Melese H, Alamer A, Hailu Temesgen M, Kahsay G. Effectiveness of exercise therapy on gait function in diabetic peripheral neuropathy patients: a systematic review of randomized controlled trials. Diabetes Metab Syndr Obes. (2020) 13:2753–64. doi: 10.2147/DMSO.S261175
118. Prajapati SK, Gage WH, Brooks D, Black SE, McIlroy WE. A novel approach to ambulatory monitoring: investigation into the quantity and control of everyday walking in patients with subacute stroke. Neurorehabil Neural Repair. (2011) 25:6–14. doi: 10.1177/1545968310374189
119. Hsu C, Tsai Y, Yau C, Shie H, Wu C. Test-Retest reliability of an automated infrared-assisted trunk accelerometer-based gait analysis system. Sens. (2016) 16(8):1156. doi: 10.3390/s16081156
120. Byun S, Han JW, Kim TH, Kim KW. Test-Retest reliability and concurrent validity of a single tri-axial accelerometer-based gait analysis in older adults with Normal cognition. PLoS One. (2016) 11:e0158956. doi: 10.1371/journal.pone.0158956
121. Poitras I, Dupuis F, Bielmann M, Campeau-Lecours A, Mercier C, Bouyer LJ, et al. Validity and reliability of wearable sensors for joint angle estimation: a systematic review. Sens. (2019) 19(7):1555. doi: 10.3390/s19071555
122. Ro DH, Lee J, Lee J, Park JY, Han HS, Lee MC. Effects of knee osteoarthritis on hip and ankle gait mechanics. Adv Orthop. (2019) 2019:9757369. doi: 10.1155/2019/9757369
123. Viteckova S, Kutilek P, Svoboda Z, Krupicka R, Kauler J, Szabo Z. Gait symmetry measures: a review of current and prospective methods. Biomed Signal Process Control. (2018) 42:89–100. doi: 10.1016/j.bspc.2018.01.013
Keywords: clinical gait assessment, gait technologies, gait measures, mobile gait lab, gait pathologies
Citation: Hulleck AA, Menoth Mohan D, Abdallah N, El Rich M and Khalaf K (2022) Present and future of gait assessment in clinical practice: Towards the application of novel trends and technologies. Front. Med. Technol. 4:901331. doi: 10.3389/fmedt.2022.901331
Received: 21 March 2022; Accepted: 17 November 2022;
Published: 16 December 2022.
Edited by:
Gregg Suaning, The University of Sydney, AustraliaReviewed by:
Leo R. Quinlan, University of Galway, Ireland© 2022 Hulleck, Menoth Mohan, Abdallah, El Rich and Khalaf. This is an open-access article distributed under the terms of the Creative Commons Attribution License (CC BY). The use, distribution or reproduction in other forums is permitted, provided the original author(s) and the copyright owner(s) are credited and that the original publication in this journal is cited, in accordance with accepted academic practice. No use, distribution or reproduction is permitted which does not comply with these terms.
*Correspondence: Kinda Khalaf a2luZGEua2hhbGFmQGt1LmFjLmFl
Specialty Section: This article was submitted to Diagnostic and Therapeutic Devices, a section of the journal Frontiers in Medical Technology
Disclaimer: All claims expressed in this article are solely those of the authors and do not necessarily represent those of their affiliated organizations, or those of the publisher, the editors and the reviewers. Any product that may be evaluated in this article or claim that may be made by its manufacturer is not guaranteed or endorsed by the publisher.
Research integrity at Frontiers
Learn more about the work of our research integrity team to safeguard the quality of each article we publish.