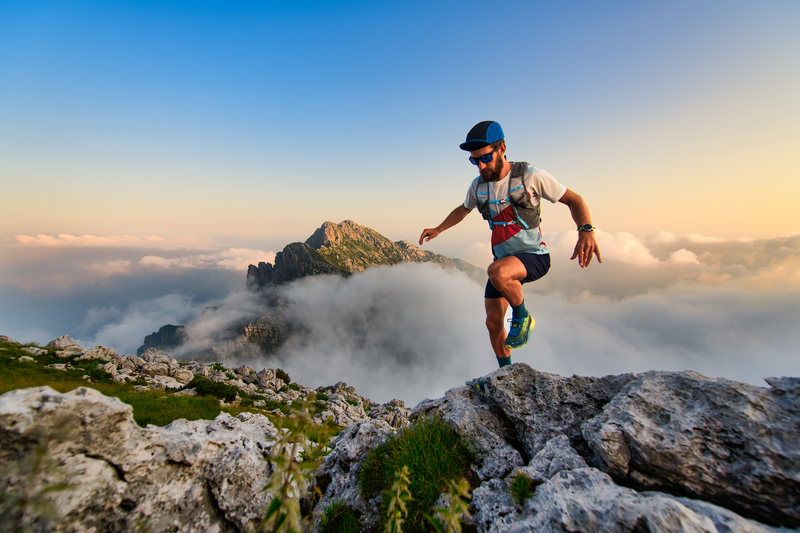
94% of researchers rate our articles as excellent or good
Learn more about the work of our research integrity team to safeguard the quality of each article we publish.
Find out more
TECHNOLOGY AND CODE article
Front. Med. Eng.
Sec. Advanced Technologies for Medicine
Volume 3 - 2025 | doi: 10.3389/fmede.2025.1547895
The final, formatted version of the article will be published soon.
You have multiple emails registered with Frontiers:
Please enter your email address:
If you already have an account, please login
You don't have a Frontiers account ? You can register here
Bone is constantly adapting each of its microstructural compartments by modeling and remodeling. These adaptations are delineated by whether bone formation and resorption are coupled in space and time. Time-lapse microCT imaging has become a valuable technique for characterizing bone dynamics in 3D. Our previous study used longitudinal microCT imaging to quantify modeling and remodeling across the bone microstructure in response to PTH treatment and mechanical loading. Here, we detail our technique of voxel-tracking to specifically identify time-dependent modeling and remodeling by examining the sequence of formation and resorption events in trabecular and cortical bone. We apply this technique to WT and SOST KO littermate mice under long-term mechanical loading and quantify site-specific bone volume changes. Loading particularly affected WT trabecular and periosteal bone by increasing anabolic modeling and remodeling while decreasing catabolic modeling. Under load-controlled loading, these effects were reduced in SOST KO mice. Endosteal bone was less responsive to loading for both genotypes, with subtler and more time-dependent responses resulting in a load-dependent increase in WT catabolic modeling. Thus, we present a technique that directly assesses longitudinal 3D bone modeling and remodeling across the bone microstructure.
Keywords: In vivo microCT, Bone Remodeling, Bone modeling, Trabecular bone, Cortical bone, mechanical loading
Received: 18 Dec 2024; Accepted: 28 Feb 2025.
Copyright: © 2025 Shyu, Robinson and Guo. This is an open-access article distributed under the terms of the Creative Commons Attribution License (CC BY). The use, distribution or reproduction in other forums is permitted, provided the original author(s) or licensor are credited and that the original publication in this journal is cited, in accordance with accepted academic practice. No use, distribution or reproduction is permitted which does not comply with these terms.
* Correspondence:
X. Edward Guo, Columbia University, New York City, United States
Disclaimer: All claims expressed in this article are solely those of the authors and do not necessarily represent those of their affiliated organizations, or those of the publisher, the editors and the reviewers. Any product that may be evaluated in this article or claim that may be made by its manufacturer is not guaranteed or endorsed by the publisher.
Research integrity at Frontiers
Learn more about the work of our research integrity team to safeguard the quality of each article we publish.