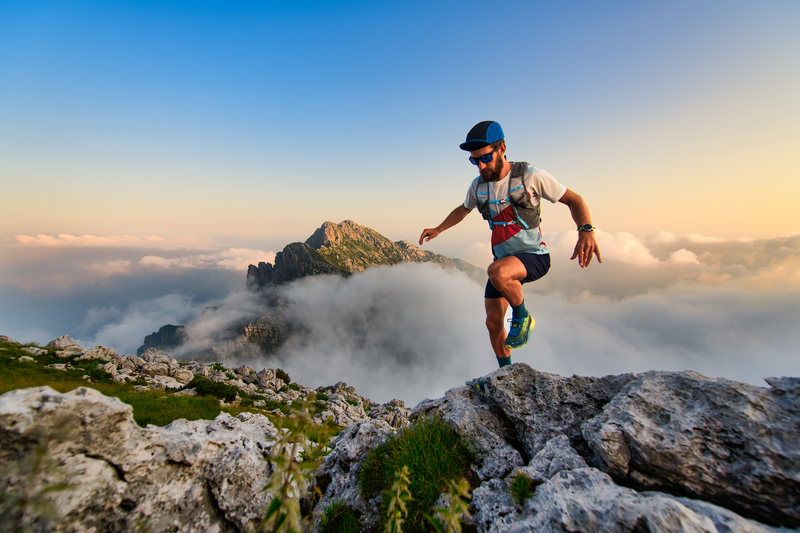
95% of researchers rate our articles as excellent or good
Learn more about the work of our research integrity team to safeguard the quality of each article we publish.
Find out more
ORIGINAL RESEARCH article
Front. Med. Eng. , 16 December 2024
Sec. Computational Medicine
Volume 2 - 2024 | https://doi.org/10.3389/fmede.2024.1455116
We here present a deep-learning approach for computing depth of anesthesia (DoA) for pigs undergoing general anesthesia with propofol, integrated into a novel general anesthesia specialized MatLab-based graphical user interface (GAM-GUI) toolbox. This toolbox permits the collection of EEG signals from a BIOPAC MP160 device in real-time. They are analyzed using classical signal processing algorithms combined with pharmacokinetic and pharmacodynamic (PK/PD) predictions of anesthetic concentrations and their effects on DoA and the prediction of DoA using a novel deep learning-based algorithm. Integrating the DoA estimation algorithm into a supporting toolbox allows for the clinical validation of the prediction and its immediate application in veterinary practice. This novel, artificial-intelligence-driven, user-defined, open-access software tool offers a valuable resource for both researchers and clinicians in conducting EEG analysis in real-time and offline settings in pigs and, potentially, other animal species. Its open-source nature differentiates it from proprietary platforms like Sedline and BIS, providing greater flexibility and accessibility.
The customization of general anesthetic dosages requires a precise assessment of their effect. Electroencephalographic (EEG) activity has been extensively explored for this purpose in human patients, giving rise to EEG-based metrics that serve as objective indicators of depth of anesthesia (DoA). Noteworthy examples include the bispectral index (BIS) Johansen (2006), Narcotrend Kreuer and Wilhelm (2006), and patient state index (PSI) Drover and Ortega (2006), all of which could also be harnessed as feedback parameters for closed-loop technology in automated drug delivery systems. It is hypothesized that they combine well-identified EEG signal features such as burst suppression ratio (BSR), spectral edge (or median) frequency (SEF or MSF), spectral power ratio (SPR) of different frequency bands, and/or entropy (Purdon et al., 2015); (Connor, 2022); (Lee et al., 2019); (Hwang et al., 2023), distilling them into a single value ranging from 0 (representative of deep anesthetic state) to 100 (full wakefulness).
Despite also being often applied to veterinary species, they have been based and validated only on human data; thus, their validity in animals must first be assessed. The algorithms responsible for computing those DoA indices developed so far are proprietary and impervious to modification. Moreover, their applicability in human settings is marred by several reported limitations: failure to account for drug-specific alterations in EEG patterns caused by different anesthetic agents (Purdon et al., 2015; Mahajan et al., 2017), delayed return to baseline value when contrasted with the recovery of consciousness, leading to the potential risk of recall or awareness issues (Russell, 2006) and the failure to account for neural inertia (Eleveld et al., 2021). The correlation between EEG-derived metrics and the degree of cerebral depression might diverge during the induction and recovery phases of anesthesia. The differences between humans and pigs should be viewed as an example, as similar variations are likely to be found among other species.
Conventional EEG indices might be inadequate for accurately gauging DoA. The principal problem here is their closed nature, which makes impossible to incorporate experience and suggestions from the scientific community. The creation of an open index would greatly improve the current situation, and its algorithm could be easily adapted to the different protocol used and/or species involved. Even if the index used is convenient, especially when a close-loop infusion is performed, the real-time interpretation of the unprocessed EEG and its density spectral array (DSA) would provide more detailed information, assisting anesthetists even further in their decisions (Purdon et al., 2015). Due to the complementary nature of these assessment strategies, it is vital to develop a tool capable of displaying them together, making it possible to modify the algorithm on which the DoA index is based.
This study presents a general anesthesia specialized MatLab-based graphical user interface (GAM-GUI) (Caillet et al., 2023), a novel artificial-intelligence-driven, user-defined, open-access software tool. It permits the combined interpretation of both classic EEG features (e.g., BSR, SEF, SPR, entropy, and DSA) and a new DoA index based on a deep learning (DL) algorithm both offline and in real-time. This would provide a valuable resource for both researchers and clinicians in conducting EEG analysis in pigs and potentially other animal species. The real-time interface of the GAM-GUI tool running on a user’s PC also allows connection with the EEG device (BIOPAC, 2023) for real-time EEG data acquisition. Moreover, GAM-GUI permits the use of pharmacokinetic/pharmacodynamic (PK/PD) models to administer injectable anesthetics via a target control infusion in a closed loop fashion. The use of this new tool would help anesthesiologists perform patient-based DoA evaluation through a comprehensive assessment of the EEG signal.
The paper is organized as follows. In Section 2, we present the general functionality of the GAM-GUI toolbox and reveal all the details of the novel DL algorithms for DoA index estimation. The results of the DoA estimation algorithm are presented in Section 3. In Section 4, we present the related work composed of a discussion of the real-time requirements for the system, existing machine learning approaches for DoA index estimation, and EEG signal processing tools. Conclusions are drawn in Section 5.
In this section, we will first introduce the essential algorithms for EEG signal processing, enabling the extraction of the observational information about DoA. We then briefly present our GAM-GUI tool, revealing additional details of a PK/PD mathematical model of propofol implemented in the dedicated panel of our tool to allow the evaluation of propofol dynamics.
In the search for accurate DoA measures, several aspects of the EEG signal are known to correlate with the patient’s state during general anesthesia.
Density specral array (DSA) or spectrogram is a powerful signal visualization tool, especially for DoA analysis where most of the relevant features are computed in the frequency domain (Kim et al., 2020). DSA allows the visualization of a representation of the re-partition of spectral power (SP). This is displayed as a heat map where the high power is shown in a warmer color (red) and low power in colder colors (blue). While using DSA in EEG-base anesthetic state monitoring is not standard practice, numerous studies have reported the relationship between EEG oscillations and DoA. An example of the DSA derived from the EEG signal collected from a pig undergoing anesthesia with propofol with indications of anesthesia processes mapped to it is used as background for depicting other EEG features (Figure 1).
Figure 1. BSR (red) and SEF90 (blue) features of EEG signal of a pig undergoing propofol anesthesia. A vertical bar “Start propofol” indicates the beginning of propofol delivery following one of the protocols described in Section 2.3.1. The pig was intubated during the whole period between the “Intubation” and “Extubation” vertical bars.
Burst suppression ratio (BSR) is a measure of the amount of time that a patient’s brain is in a state of burst suppression, which is characterized by alternating periods of high-amplitude bursts and isoelectric (low amplitude) activity. The proportion of time spent in suppression increases with anesthetic dose. The BSR provides values between
To compute BSR, the EEG signal is divided into epochs of fixed length, such as
In order to produce a BSR signal, such computations are performed with a certain step, such as
Spectral edge frequency (SEF) allows the computing of a frequency below which a defined percentage of the signal’s power is located. The computation of spectral edge frequency 90% (SEF90) or 95% (SEF95) has often been used to guide DoA estimation. In our GUI, we allow a user to parametrize the SEF percentage, therefore also allowing the computation of the spectral median edge frequency—for example, 50%. In general, as anesthesia deepens, the SEF also decreases, which is not the case on Figure 1. This is probably due to the anatomical and morphological peculiarities of pigs, causing the signal of deep anesthesia state to be contaminated with the ECG signal, raising the values of SEF90. This indicates that the DoA index developed for humans will not work the same on pigs.
Peak frequency (PF) is the location of the signal peak frequency—the frequency value at the maximal power of the spectrum. Since a frequency shift of oscillatory power peaks may not be captured by SEF90 or SEF95, the location of the signal PF may bring additional information. Since the largest part of the EEG signal power is located in a very low-frequency band, below
Spectral power ratio (SPR) is a feature that allows comparison of the spectral power of different frequency bands within the EEG signal. Classically, the EEG signal is divided into the following set of frequency bands: delta –
Entropy provides an estimation of the complexity of EEG signals. It has been observed that during the deeper phases of anesthesia, entropy will be lower than in the awake or waking phase. In human anesthesia, it is known that different parts of the brain may provide distinct EEG signatures, thus carrying different information in response to general anesthesia (Yeom et al., 2017). Therefore, in recent years special attention has also been given to phase lag entropy (Shin et al., 2020; Jun et al., 2019; Kim et al., 2021), a measurement of temporal pattern diversity in the phase relationship between two EEG signals from prefrontal and frontal montages. We do not differentiate here between signals collected from different scalp locations, and therefore we have evaluated the entropy of each collected EEG signal separately. We computed entropy over temporal domain EEG signals, even though some have investigated spectral entropy’s (SE) correlation with BIS (Ra et al., 2021). Our main reason for choosing the entropy of the temporal domain over the SE is our goal of implementing DoA estimation in real-time where one entropy value would be expected every
Thus, we implemented the Shannon method to calculate entropy in the time domain. First, we computed a histogram of the amplitudes of the EEG signal for a moving window. Then, we used the formula below on all bins of the histogram containing at least one value.
The probability of
The novel general anesthesia Matlab-based graphical user interface (GAM-GUI) tool provides an analysis of EEG signals to support the signal features described above. Generally, we can split the analysis into offline and real-time. During offline analysis, EEG signals collected previously during the experiment can be analyzed channel by channel as well as multiple EEG channels in parallel. The view of the offline analysis tab is presented in Figure 2. There, GAM-GUI allows selection of any EEG signal from a database and makes a first quick analysis of EEG signal quality based on the DSA previously displayed right after the signal load. In addition, it is possible to set the essential parameters for signal processing algorithms and display the results in a superposed manner (as demonstrated in Figure 2), where each result can be turned off and on by clicking the dedicated buttons.
However, the real-time version of GAM-GUI allows the same signal processing performed within defined time constraints that we set to be
Additional details of GAM-GUI functionality, including a description of the communication algorithm and some user guiding suggestions, can be found in our technical report (Caillet et al., 2023). The demo version of the offline data analysis tool can be found at https://gitlab.hevs.ch/alena.simalats/gam-gui. Fully functional software providing real-time data acquisition, processing, and DoA index evaluation adapted for use with a BIOPAC MP160 EEG acquisition device can be found at https://gitlab.hevs.ch/alena.simalats/RT-DoAi-Vet.
The GAM-GUI tool offers multiple computational panels, among which we have the panel for evaluating the dynamics of the anesthetic used—propofol. This permits a description of the relationship between the delivered dose of the drug, the resulting concentration in the body (i.e., PK model), and the effect of the drug on the body (i.e., PD model for a chosen timeline).
In brief, the PK model of propofol for humans (Eleveld et al., 2018) and for pigs (Egan et al., 2003b) is usually described by a three-compartment model extended with a fourth virtual compartment representing the effect site of the brain. The system of compartments can be seen as four communicating vessels, the drug exchange rate being regulated by
where
The PD part of the model for pigs was described as a double sigmoid function in Mirra et al. (2022a):
Here,
The PKPD panel depicted in Figure 3 allows insertion of the parameters of the PK and PD models and display of the change of drug concentration in the central and affected compartments, as well as the PD effect of the drug concentration, following the injection rates that can also be set in this panel. As the default PK parameters, we set the values of the propofol population PK model developed for pigs (Egan et al., 2003b). The PD part of the model is implemented as a double sigmoid function as described in Mirra et al. (2022a) and detailed above. However, to implement a model of another drug, the exchange rate parameters must be updated. Figure 3 depicts the plasma concentration and PD effect level computed by the PKPD model following the actual injection rate of the experiment during which the EEG signal was recorded.
Figure 3. Tab 1: PKPD panel depicting the plasma concentration and PD effect level following the actual injection rate of the experiment on a pig under propofol anesthesia.
The core element of the real-time GAM-GUI is the deep-learning-based DoA index computation. To describe the DL algorithm and discuss its performance, we first present the data set that was used for model training and validation in Section 2.3.1. The implementation of a supervised method for DoA estimation requires defining a reference ground-truth signal indicating the genuine DoA values. Therefore, in Section 2.3.2 we describe how the ideal DoA reference signal was created. Furthermore, we provide the results of the analysis of EEG features’ relevance to the DoA estimation in Section 2.3.3, while the DL algorithm is presented in Section 2.4.
The EEG signals were collected in Mirra et al. (2022b) and Mirra et al. (2024) and re-analyzed retrospectively for the present investigation. Details of data collection and anesthetic protocols can be found at these references. The dataset used for training and validation was composed of EEG signals collected during 31 experimental events on pigs undergoing general anesthesia. The EEG signals were collected by eight channels of a BIOPAC MP160 EEG acquisition device (BIOPAC, 2023) and 4 four of a Sedline device (MASIMO, 2023) monitoring pigs anesthetized with propofol. During each experiment, 12 EEG electrodes were placed on each pig’s head, each collecting a separate EEG signal from different areas of the brain, providing time-series with
In human anesthesia, it is known that different parts of the brain may provide distinct EEG signatures, thus carrying different information in response to general anesthesia (Yeom et al., 2017). The PSI index, for example, accounts for changes in symmetry and synchronization between brain regions and the inhibition of frontal cortex regions (Drover and Ortega, 2006). For the present investigation, the signals recorded at different cortex regions from the same individual were integrated, as well as bilateral recordings. Each EEG signal was considered unique and as potentially contributing supplementary information to the system. Therefore, the analysis is global at this stage and not specific for one electrode placement. Our final dataset thus contains a total of 358 EEG time series each associated with one reference DoA index.
In order to use supervised methods like the long short-term memory (LSTM) model presented in Section 2.4, it is necessary to define a reference ground-truth DoA signal. This may create a paradox to base the reference signal on EEG-derived features while exact EEG features’ correlation to DoA is not known. Therefore, we combined three existing metrics for DoA estimation. First, a personalized PKPD model was developed for each subject of the study. Variability in both PK and PD parameters is known to be large for humans (Eleveld et al., 2018) and is expected to also be large for animals. Therefore, initial PKPD parameters were aligned to previously published PK (Egan et al., 2003a) and PD models (Mirra et al., 2022a). Furthermore, those parameters were personalized based on an individual output variable for DoA oriented by the individual PSI values and clinical observations. Finally, for each subject, an individualized DoA effect curve based on the history of propofol delivery, PSI, and clinical observations was used as ground-truth DoA.
The next step in developing the best performing DL model for DoA estimation was to select the best predictors. In Caillet et al. (2024), we had evaluated the prediction capability of EEG features for DoA estimation, with three algorithms having core computations of variance such as Spearman correlation (CORRs), principle component analysis (PCA), and ReliefF algorithms. The choice of algorithm was driven by the idea of linking upcoming results with the old practice of DoA estimation using BIS and PSI, where formulae for DoA estimation are the arithmetic combination of features having a higher correlation with DoA. We also proposed two strategies for pruning the large features set ranked by these three algorithms.
Figure 4 presents the final features ranking, the details of which are presented in Caillet et al. (2024), as well as two strategies for selecting the best features for DoA estimation. The features are sorted in descending rank order from top to bottom. The mnemonics of each feature name on the figure follow the general rule such as first naming the feature type (e.g. SP, SPR, and entropy) and then the frequency band over which it was computed. The PF feature was computed only over the full frequency band.
Figure 4. Features pruning following the two presented strategies. To help the reader, we have used similar colors to group SP features based on their frequency. SP features representing frequency ranges greater than 1 Hz are additionally highlighted in bold. Some other unique features are regrouped with similar color codes, such as BSR, SEF90, and SEF95.
Among the SPR features, we selected the ratio of SP density of the above-mentioned frequency bands vs. full SP density such that SPR 0.5–4 Hz would mean the ration of SP 0.5–4 Hz and 0.5–100 Hz. We also included the classical SPRs often mentioned in the literature, such as
In Figure 4, the columns “All features” show that SP features with higher frequencies within the 1 Hz range (e.g., SP 29–30 Hz or SP 31–32 Hz) are ranked higher than SP features covering larger frequency ranges (e.g., SP 25–35 Hz or SP 15–35 Hz). However, there is a change of ranking for lower frequencies. For instance, SP 12–25 Hz is ranked higher than SP 14–15 Hz, SP 13–14 Hz, and so forth. To reduce information redundancy, different strategies can be followed as follows.
For frequencies above 15 Hz, SP features can be chosen with a 1 Hz range and SP and SPR features with larger ranges excluded. Conversely, for lower frequencies, it may be preferable to retain SP and SPR features with larger ranges and exclude those with a 1 Hz range. An exception among lower-frequency SP features might be made for SP 0–1 Hz, SP 1–2 Hz, SP 2–3 Hz, and SP 3–4 Hz, since three of these are ranked higher than SP and SPR 0.5–4 Hz. The results of feature pruning following this strategy are presented in Strategy 1, Figure 4.
In Caillet et al. (2024), we made the crucial observation that data resolution may significantly impact prediction stability, especially when dealing with very small numbers such as the SP of high ranges (e.g., SP 29–30 Hz or SP 31–32 Hz). Therefore, when selecting SP features, they might be prioritized over spectral bands larger than 1 Hz, especially for frequencies above 14 Hz. SP features over narrow frequency bands can be included only for frequencies between 0 and 4 Hz, as they exhibit maximum spectral power. As illustrated in Strategy 2, Figure 4, we first pruned the SP features for frequencies above 12 Hz. It is notable that SP 15–35 Hz encompasses the information of both SP 25–35 Hz and SP 12–25 Hz features. Additionally, SP and SPR features over the same frequency band are likely to convey similar information, as SPR can be considered a normalized SP signal. Following this rationale, we further selected the best 26 and 10 features.
However, it is also clear that the choice of the best set of features also depends on the ML/DL methods that are to be used for further DoA estimation. Therefore, since we used the long short-term memory algorithm (Section 2.4), we also performed ablation study of features in order to rank each specifically for this algorithm.
When choosing the model, we aimed to provide anesthesiologists with a DoA index represented by a curve similar to BIS or PSI—that is, data with temporal quality, where each subsequent value depends on those previous. This naturally led to our choice of a recurrent neural network (RNN) and its particular class of LSTM models designed to learn long-term dependencies in sequential data and “retain” information from prior time steps. Different layers with a corresponding number of neurons of our deep learning model are presented in Figure 5.
The first layer contains carefully selected EEG features (Section 2.3.3) given to the Autoencoder layer. Autoencoders, such as the stacked denoising autoencoder (SDAE), have shown significant promise in tasks requiring dimensional and noise reduction, and meaningful and lower dimension feature space extraction. Therefore, we opted to enhance the LSTM model with an SDAE layer. Finally, lower-dimensional feature space is given to the LSTM layer followed by regression and output layers providing a single output. Adaptive moment estimation (ADAM) was chosen as the optimization algorithm for the LSTM model due to its higher time efficiency and ability to adjust the learning rate (LR) for each weight in the neural network. The initial LR value was set to 0.0001, which in our case strikes a balance between fast convergence and maintaining model accuracy. A mini-batch size of five was chosen. Using smaller mini-batches allowed us to speed up training and reduce memory consumption, making the model learning process both efficient and more manageable. We divided our dataset of 358 EEG recordings into a training and validation set, with 298 and 60 recordings respectively.
In order to find the best mode configuration, we ran multiple model training, changing the number of LSTM and SDAE layers. We considered the best performing models as having the minimal root-mean-square error (RMSE) for the validation set.
To cover as much space of possible configurations as possible for model training in terms of number of features and LSTM/SDAE nodes, we first considered different numbers of features, such as the 5-, 10-, 20-, and 26-best features selected based on tree features ranking algorithms, such as CORRs, PCA and ReliefF following the strategies presented in Section 2.3.3. Strategy 2 for feature selection gave generally better performance results than Strategy 1. Moreover, thanks to regrouping the high frequency SP features of 1 Hz bandwidth into SP features of larger frequency bands with Strategy 2, DoA estimation is expected to be more resilient to electrical noise in real clinical settings. Such a strategy also reduces the computational load thanks to fewer high-order band-path filters that otherwise would be required by Strategy 1. Therefore, we here present the results of model configuration for features selected based only on strategies 2 and 3. We also ran experiments for all 65 features—without performing feature selection—thus providing us with nine different configurations based only on a number of selected features ranked with two different methods.
The EEG features in our study form a set of predictors that are the results of DoA and not its cause. It is known that variables that directly cause the estimated value are generally much better predictors. In our study, the history of the propofol delivery rate (“delivery_rate”) is the only predictor that directly causes changes in DoA. The feature causality graph is as follows:
However, in clinical veterinary settings, it can be a challenge to know the delivery rate of an anesthetic drug in real-time. This requires an infusion pump capable of performing autonomous drug injection and providing the delivery rate values synchronously with an EEG device. Using only the delivery rate in the absence of EEG for DoA estimation would result in a DL model that merely repeats the population PK/PD model. Therefore, EEG signals are important for personalizing the DoA estimation. In this study, we evaluated two approaches. In one, we aimed to build a DoA estimation model based solely on EEG signals. In the other, we assessed how the knowledge of the propofol delivery rate can improve DoA estimation.
Since the drug delivery rate causes DoA, it clearly has higher potential for DoA prediction. In order to analyze how knowledge of the delivery rate can improve DL-based DoA prediction, we ran experiments for nine selected configurations of feature settings with and without delivery rate, thus providing features_set = 18 different configurations. For each feature set decided, we selected the number of LSTM layers of the model as a factor of
We considered the most accurate models as those having the smallest RMSE computed for the validation set. For each feature set we thus selected the two best performing models, resulting in 36 models for DoA training only with LSTM neurons without the SDAE layer. Finally, we augmented each of the 36 models with three configurations of the SDAE layer, composed of a number of SDAE neurons twice smaller, equal, and twice greater than the number of features of each model out the 36 models, resulting in 108 configurations of SDAE- LSTM models.
The results of two sets of the best ten models are presented in Figure 6.
Figure 6. Ten best models for selected sets of EEG features, including the propofol delivery rate, and ten best models based only on EEG features.
We present the RMSE values for both training and validation sets since the big difference in these values may indicate that the trained model is overfitted. We also include the RMSE values for identical models based only on the LSTM layer “LSTM only” augmented with the SDAE layer “LSTM + SDAE”. The corresponding number of neurons in the LSTM and SDAE layers are indicated in the columns “LSTM layer” and “SDAE layer”, respectively. The mnemonics of the model names are as follows: Selected5, Selected10, Selected26, etc., meaning the list of 5, 10 or 26 best features ranked in Simalatsar et al. (2024), while LSTM5, LSTM10 etc., mean the best 5, 10, etc. features selected with the ablation study of LSTM model. The presence of “delivery_rate” in the model’s name indicates that this rate was included as a predictor.
It is clear that the inclusion of the delivery rate in the model prediction greatly improves the model’s accuracy. Moreover, the presence of the delivery rate as a feature of the number of EEG features can be small as well as the number of LSTM and SDAE layers. The best model for DoA predication based only on EEG features requires a large number of features as well as LSTM layers. Note also that inclusion of all features does not necessarily produce the best results since the best two performing models are based on Selected 26 and LSTM 20 feature sets. The model’s delivery rate and additional SDAE layer do not much improve the model’s performance. However, for the models without the delivery rate, the additional SDAE layer helps improve the model’s accuracy.
Figure 7 presents the performance of the two most accurate models without the delivery rate augmented by SDAE layer and without it, such as 975 LSTM neurons and no SDAE layer (solid green line) with 390 LSTM neurons augmented with an SDAE layer of 130 neurons (solid blue line). We selected two models that use an identical set of features—ALL —to create comparable conditions for model comparison with the goal of assessing the impact of the presence or absence of the SDAE layer. We randomly selected three experiments to compare the models’ performance. In Figure 7, two of our models are compared to the PSI signal acquired during those experiments with the Sedline monitor, as well as to a ground-truth signal computed using a personalized PKPD model for each experiment. Since the propofol delivery rate was increased in a stepwise manner, we observe the ground-truth DoA decreasing in a similar stepwise pattern. All models performed comparably when the DoA was above 40%. However, below 40%, the PSI signal became very unstable and unreliable, particularly in the second and third experiments. Both our models remained stable, even though they struggled to accurately reflect the deeper stages of anesthesia. The accuracy of these two models, measured by RMSE, is quite similar. However, the DoA curve estimated by the model augmented with the SDAE layer is more stable, likely due to the SDAE’s ability to reduce signal noise. This result supports the inclusion of the SDAE layer, even though it does not directly improve RMSE-based model accuracy.
Figure 7. Comparison of DoA effect curve computed with two models without delivery rate: 975 LSTM neurons no SDAE layer (solid green line) with 390 LSTM neurons augmented with SDAE layer of 130 neurons (solid blue line).
On Figures 8, 9, we did a similar comparison of two other models with the goal of assessing the impact of the presence or absence of delivery rate as a feature. Figure 8 depicts the visual comparison of DoA estimation with the best trained model number 1, including delivery rate, while Figure 9 depicts the visual comparison of DoA estimation with model number 11—the best model without delivery rate. It is apparent that the model with the delivery rate performs much better than the model without. The model without the delivery rate is most likely to be used in clinical settings. Even though it performs worse than the model with the delivery rate, it still performed much better than the PSI index.
Figure 8. Comparison of DoA effect curve estimated with our best model with the delivery rate (Model 1: selected top five features based on strategy 2 with a delivery rate, with 75 LSTM layers and two SDAE layers), represented by the solid blue line, with the ground-truth DoA curve (solid red line) and Sedline PSI index (unstable fine line).
Figure 9. Comparison of DoA effect curve estimated with our best model without delivery rate (Model 11: selected top 26 features based on strategy 2 without delivery rate, with 520 LSTM layers and 52 SDAE layers), represented by solid blue line, with ground-truth DoA curve (solid red line) and Sedline PSI index (unstable fine line).
Both models exhibited remarkable stability and accuracy during the initial stages of the falling-asleep and awakening phases. However, we observed a notable discrepancy during the deep anesthesia phase, indicating a greater prediction error. Our hypothesis is that this inaccuracy is due to contamination of the EEG signal with the electrocardiogram (ECG). In a deep state of anesthesia, EEG signal power decreases markedly, and therefore the ECG signal is otherwise masked by the EEG. Multiple harmonics at various frequencies (Figure 10) corrupted our prediction, since features like SP represent an essential set of training features.
This discrepancy in performance across different phases has led us to contemplate the potential benefits of a more specialized approach. Specifically, we are considering training separate models for each phase of the anesthesia process: one for the period before deep phase of anesthesia, one for the duration of the deep phase, and one for the recovery phase. This strategy could allow each model to more effectively learn the unique characteristics and patterns specific to its respective phase, thereby potentially enhancing overall prediction accuracy.
This study presents the results of interdisciplinary work that brings together EEG signal processing in a Matlab environment and a novel approach DoA prediction using a DL model. We here discuss these three research domains. First, we present the basic reasoning behind the choice of real-time constraints. We then present research which applies machine learning algorithms for DoA estimation. Finally, we discuss how our GUI is different from the other tools that enable EEG signal processing.
The goal of real-time EEG monitoring and DoA estimation is to provide immediate adequate evaluation of a patient state’s under anesthesia. Such information can either be used by anesthesiologists to adapt the delivery rate of anesthetic or can also be used to implement an automated propofol delivery pump performing regular readjustments of the delivery rate based on the DoA index. At present, validated target control infusion (TCI) systems for the automated delivery of intravenous (IV) propofol to assist general anesthesia are only available for use in human medicine (Burton et al., 2020). This is achieved using open-loop systems based on population PK models but neglecting inter- and intra-patient variability to predict brain drug concentration—thus with an implicitly high level of imprecision (Schnider et al., 2021; Eleveld et al., 2018). While BIS-based propofol TCI has been reported in animals (e.g. pigs and dogs), its validity and wider applicability in veterinary medicine appear highly questionable because the BIS index is potentially influenced by several unrelated external factors which are unstable and contaminated by artefacts. Therefore, in view of the potential implementation of such a TCI pump, a novel DoA index must be developed which is stable, clear of artifacts, and insensitive to external electric noise. These were the main qualities that we targeted when implementing a novel DL based index (Section 2.4). On the other hand, an automated TCI system must regularly update the drug delivery rate based on feedback received and analyzed as EEG signals. Current validated open-loop TCI systems perform the delivery rate readjustment every second. Therefore, we also chose the time-constrain as
ML/DL algorithms have already been applied to refine an EEG-based forecast of DoA. Previous research has attempted to predict a binary outcome between either awakeness or unconscious/anesthetized status (Ramaswamy et al., 2019; Nagaraj et al., 2018; Nicolaou et al., 2012; Mirsadeghi et al., 2015). In some of these studies, the classification was extended to levels of sedation corresponding to the Modified Observer and Assessment of Alertness/Sedation Scale (MOAAS) (Ramaswamy et al., 2019; Nagaraj et al., 2018). This scale is validated to discriminate different DoAs in humans via response to stimulation at increasing intensity, which is not possible in anesthetized states nor in animals. While typical EEG features are provided to these approaches as potential candidates for the final algorithm, Ramaswamy et al. (2019) incorporated a larger number of DoA-related features (up to 44 variables) to improve classification of a patient’s DoA.
Other studies have considered the explicit time-dependent nature of unconsciousness in a statistically principled fashion with hidden Markov models (HMMs), which allow the combining of ML/DL EEG features from individual epochs with information on how the EEG temporally evolves between epochs and thus improves ML classification of unconsciousness (Abel et al., 2021). However, this solution provides a mix of binary and probability information and does not deliver a continuous DoA index to orient an anesthetist’s decision.
We assume that classification algorithms are used in the above studies due to the absence of an ideal reference DoA index trend curve, providing DoA values with high resolution for each prediction step. We have developed an approach for computing such a trend curve and therefore used a DL model to estimate DoA similar to the BIS or PSI.
There are several Matlab based frameworks for EEG signal processing. Among these, EEGLAB (Delorme and Makeig, 2004) is the best known and most powerful toolbox oriented toward the neurosciences, such as processing and analyzing high density EEG (hdEEG) like an EEG system collecting 24 or even 256 EEG signals in parallel placed on different areas of the head of an individual. EEGLAB provides a range of tools and functions tailored for the processing and analysis of hdEEG, which are essential for analyzing event-related potential (ERP) data. It includes tools for importing and pre-processing data, filtering, artifact removal, feature extraction, and advanced analysis techniques such as independent component analysis (ICA), time-frequency analysis, and source localization. EEGLAB also provides a range of visualizations, such as scalp maps, topographic plots (topoplots), and scalp heatmaps of values of individual components. There are also FreeSurfer Dale et al. (1999) and FieldTrip Oostenveld et al. (2011) MATLAB toolboxes, which specialize in processing chain for sleep studies with hdEEG.
In contrast to EEGLAB, FreeSurfer, and FieldTrip, our GAM-GUI toolbox is a specialized tool for anesthesia with a much lower density of EEG signal, such that they are placed only on the frontal and prefrontal areas of the head with great in-depth analysis of each individual signal. GAM-GUI tool aggregates are all necessary algorithms for EEG feature extraction essential for DoA prediction; they are therefore much lighter in computation. This thus allowed us to create a real-time version of the tool performing data acquisition, analysis, and DoA estimation within the time constraints of
We tested our model in a real setting on pigs undergoing general anesthesia. Our model proved to be more stable than PSI and more satisfactory for veterinary staff. Remarkably, it performs very well during both the induction and recovery phases of anesthesia, capturing the particularities of both phases that usually pose a problem to existing DoA indexes. Extension to other species and other anesthetics would require a dataset containing EEG signals for those species anesthetized with the medication of interest and a reliable personalized reference DoA signal. However, this does not mean that the same model without any modification can work similarly on other species, since at present it is the PSI index that is used most in veterinary practice.
The datasets presented in this study can be found in online repositories. The names of the repository/repositories and accession number(s) can be found below: https://gitlab.hevs.ch/alena.simalats/gam-gui.
BC: software, visualization, and writing–review and editing. GM: software, validation, and writing–review and editing. SD: software and writing–review and editing. DH: software and writing–review and editing. AM: conceptualization, data curation, validation, and writing–review and editing. OL: conceptualization, data curation, investigation, supervision, validation, and writing–review and editing. AS: conceptualization, data curation, formal analysis, funding acquisition, investigation, methodology, project administration, resources, software, supervision, validation, visualization, writing–original draft, and writing–review and editing.
The author(s) declare that financial support was received for the research, authorship, and/or publication of this article. The authors would like to thank the University of Applied Sciences and Arts Western Switzerland for funding the research, the results of which are presented in this article.
The authors declare that the research was conducted in the absence of any commercial or financial relationships that could be construed as a potential conflict of interest.
All claims expressed in this article are solely those of the authors and do not necessarily represent those of their affiliated organizations, or those of the publisher, the editors, and the reviewers. Any product that may be evaluated in this article, or claim that may be made by its manufacturer, is not guaranteed or endorsed by the publisher.
Abel, J., Badgeley, M., Meschede-Krasa, B., Schamberg, G., Garwood, I., Lecamwasam, K., et al. (2021). Machine learning of EEG spectra classifies unconsciousness during gabaergic anesthesia. PLOS ONE 16, e0246165. doi:10.1371/journal.pone.0246165
BIOPAC (2023). BIOPAC MP160 EEG acquisition device. Available at: https://www.biopac.com/.
Burton, F. M., Lowe, D. J., Millar, J. E., Corfield, A. R., Watson, M. J., Shaw, M., et al. (2020). Effect of target-controlled propofol infusion to reduce the incidence of adverse events for procedural sedation in the emergency department: a systematic review. Eur. J. Emerg. Med. 27, 253–259. doi:10.1097/MEJ.0000000000000655
Bustomi, A., and Wijaya, S. K.Prawito (2017). Analyzing power spectral of electroencephalogram (eeg) signal to identify motoric arm movement using emotiv epoc+. AIP. doi:10.1063/1.4991175
Caillet, B., Devènes, S., Mâitre, G., Hight, D., Mirra, A., Levionnois, O., et al. (2023). General Anaesthesia Matlab-based Graphical User Interface: a tool for EEG signal acquisition, processing and visualisation offline and in real-time. Tech. Rep. HES-SO.
Caillet, B., Maître, G., Mirra, A., Levionnois, O. L., and Simalatsar, A. (2024). Measure of the prediction capability of EEG features for depth of anesthesia in pigs. Front. Med. Eng. 2. doi:10.3389/fmede.2024.1393224
Connor, C. (2022). Open reimplementation of the bis algorithms for depth of anesthesia. Anesth. analgesia 135, 855–864. doi:10.1213/ane.0000000000006119
Dale, A. M., Fischl, B., and Sereno, M. I. (1999). Cortical surface-based analysis: I. segmentation and surface reconstruction. Neuroimage 9, 179–194. doi:10.1006/nimg.1998.0395
Delorme, A., and Makeig, S. (2004). Eeglab: an open source toolbox for analysis of single-trial eeg dynamics including independent component analysis. J. Neurosci. Methods 134, 9–21. doi:10.1016/j.jneumeth.2003.10.009
Drover, D., and Ortega, H. R. (2006). Patient state index. Best Pract. and Res. Clin. Anaesthesiol. 20, 121–128. doi:10.1016/j.bpa.2005.07.008
Egan, T., Kern, S., Johnson, K., and Pace, N. (2003a). The pharmacokinetics and pharmacodynamics of propofol in a modified cyclodextrin formulation (captisol) versus propofol in a lipid formulation (diprivan): an electroencephalographic and hemodynamic study in a porcine model. Anesth. analgesia 97, 72–79. doi:10.1213/01.ane.0000066019.42467.7a
Egan, T., Kern, S., Johnson, K., and Pace, N. (2003b). The pharmacokinetics and pharmacodynamics of propofol in a modified cyclodextrin formulation (captisol) versus propofol in a lipid formulation (diprivan): an electroencephalographic and hemodynamic study in a porcine model. Anesth. analgesia 97, 72–79. doi:10.1213/01.ANE.0000066019.42467.7A
Eleveld, D., Colin, P., Absalom, A., and Struys, M. (2018). Pharmacokinetic–pharmacodynamic model for propofol for broad application in anaesthesia and sedation. Br. J. Anaesth. 120, 942–959. doi:10.1016/j.bja.2018.01.018
Eleveld, D., Colin, P., Absalom, A., and Struys, M. (2021). Resisting neural inertia: an exercise in floccinaucinihilipilification? Br. J. Anaesth. 126, 31–34. doi:10.1016/j.bja.2020.09.025
Hwang, E., Park, H.-S., Kim, H., Kim, J.-Y., Jeong, H., Kim, J., et al. (2023). Development of a bispectral index score prediction model based on an interpretable deep learning algorithm. Artif. Intell. Med. 143, 102569. doi:10.1016/j.artmed.2023.102569
Johansen, J. W. (2006). Update on bispectral index monitoring. Best Pract. and Res. Clin. Anaesthesiol. 20, 81–99. doi:10.1016/j.bpa.2005.08.004
Jun, M., Yoo, J., Park, S., Na, S., Kwon, H., Nho, J., et al. (2019). Assessment of phase-lag entropy, a new measure of electroencephalographic signals, for propofol-induced sedation. Korean J. Anesthesiol. 72, 351–356. doi:10.4097/kja.d.19.00019
Kim, K.-M., Lee, K.-H., and Park, J.-H. (2021). Phase lag entropy as a surrogate measurement of hypnotic depth during sevoflurane anesthesia. Medicina 57, 1034. doi:10.3390/medicina57101034
Kim, M., Fricchione, G., Brown, E., and Akeju, O. (2020). Role of electroencephalogram oscillations and the spectrogram in monitoring anaesthesia. BJA Educ. 20, 166–172. doi:10.1016/j.bjae.2020.01.004
Kreuer, S., and Wilhelm, W. (2006). The narcotrend monitor. Best Pract. and Res. Clin. Anaesthesiol. 20, 111–119. doi:10.1016/j.bpa.2005.08.010
Lee, H., Ryu, H., Park, Y., Yoon, S., Yang, S., Oh, H., et al. (2019). Data driven investigation of bispectral index algorithm. Sci. Rep. 9, 13769. doi:10.1038/s41598-019-50391-x
Mahajan, C., Mishra, R. K., Prabhakar, H., Kapoor, I., and Bithal, P. (2017). Effect of nitrous oxide on bispectral index values at equi-minimum alveolar concentrations of sevoflurane and desflurane. Indian J. Anaesth. 61, 482–485. doi:10.4103/ija.IJA_363_16
Mirra, A., Gamez Maidanskaia, E., Levionnois, O., and Spadavecchia, C. (2024). How is the nociceptive withdrawal reflex influenced by increasing doses of propofol in pigs? Animals 14, 1081. doi:10.3390/ani14071081
Mirra, A., Spadavecchia, C., and Levionnois, O. (2022a). Correlation of sedline-generated variables and clinical signs with anaesthetic depth in experimental pigs receiving propofol. PLOS ONE 17, 02754844. doi:10.1371/journal.pone.0275484
Mirra, A., Spadavecchia, C., and Levionnois, O. (2022b). Correlation of sedline-generated variables and clinical signs with anaesthetic depth in experimental pigs receiving propofol. PLoS One 17, e0275484. doi:10.1371/journal.pone.0275484
Mirsadeghi, M., Behnam, H., Shalbaf, R., and Jelveh Moghadam, H. (2015). Characterizing awake and anesthetized states using a dimensionality reduction method. J. Med. Syst. 40, 13. doi:10.1007/s10916-015-0382-4
Nagaraj, S. B., McClain, L. M., Boyle, E. J., Zhou, D. W., Ramaswamy, S. M., Biswal, S., et al. (2018). Electroencephalogram based detection of deep sedation in icu patients using atomic decomposition. IEEE Trans. Biomed. Eng. 65, 2684–2691. doi:10.1109/TBME.2018.2813265
Nicolaou, N., Hourris, S., Alexandrou, P., and Georgiou, J. (2012). Eeg-based automatic classification of ‘awake’ versus ‘anesthetized’ state in general anesthesia using granger causality. PLOS ONE 7, e338699. doi:10.1371/journal.pone.0033869
Oostenveld, R., Fries, P., Maris, E., and Schoffelen, J. M. (2011). Fieldtrip: open source software for advanced analysis of meg, eeg, and invasive electrophysiological data. Comput. Intell. Neurosci. 2011, 1–9. doi:10.1155/2011/156869
Purdon, P., Sampson, A., Pavone, K., and Brown, E. (2015). Clinical electroencephalography for anesthesiologists. Anesthesiology 123, 937–960. doi:10.1097/aln.0000000000000841
Ra, J. S., Li, T., and Li, Y. (2021). A novel spectral entropy-based index for assessing the depth of anaesthesia. Brain Inf. 8, 10. doi:10.1186/s40708-021-00130-8
Ramaswamy, S. M., Kuizenga, M. H., Weerink, M. A., Vereecke, H. E., Struys, M. M., and Nagaraj, S. B. (2019). Novel drug-independent sedation level estimation based on machine learning of quantitative frontal electroencephalogram features in healthy volunteers. Br. J. Anaesth. 123, 479–487. doi:10.1016/j.bja.2019.06.004
Russell, I. (2006). The narcotrend ‘depth of anaesthesia’ monitor cannot reliably detect consciousness during general anaesthesia: an investigation using the isolated forearm technique. Br. J. Anaesth. 96, 346–352. doi:10.1093/bja/ael017
Saby, J. N., and Marshall, P. J. (2012). The utility of eeg band power analysis in the study of infancy and early childhood. Dev. Neuropsychol. 37, 253–273. doi:10.1080/87565641.2011.614663
Schnider, T. W., Minto, C. F., Egan, T. D., and Filipovic, M. (2021). Relationship between propofol target concentrations, bispectral index, and patient covariates during anesthesia. Anesth. and Analgesia 132, 735–742doi. doi:10.1213/ANE.0000000000005125
MASIMO (2023). Available at: https://www.masimo.com.
Shin, H., Kim, H., Jang, Y., You, H., Huh, H., Choi, Y., et al. (2020). Monitoring of anesthetic depth and eeg band power using phase lag entropy during propofol anesthesia. BMC Anesthesiol. 20, 49. doi:10.1186/s12871-020-00964-5
Keywords: EEG signal processing, depth of anesthesia, veterinary practice, long short-term memory model, pigs
Citation: Caillet B, Maître G, Devènes S, Hight D, Mirra A, Levionnois OL and Simalatsar A (2024) Long short-term-memory-based depth of anesthesia index computation for offline and real-time clinical application in pigs. Front. Med. Eng. 2:1455116. doi: 10.3389/fmede.2024.1455116
Received: 26 June 2024; Accepted: 11 November 2024;
Published: 16 December 2024.
Edited by:
Arvind Ramanathan, Argonne National Laboratory (DOE), United StatesReviewed by:
Caglar Uyulan, Izmir Kâtip Çelebi University, TürkiyeCopyright © 2024 Caillet, Maître, Devènes, Hight, Mirra, Levionnois and Simalatsar. This is an open-access article distributed under the terms of the Creative Commons Attribution License (CC BY). The use, distribution or reproduction in other forums is permitted, provided the original author(s) and the copyright owner(s) are credited and that the original publication in this journal is cited, in accordance with accepted academic practice. No use, distribution or reproduction is permitted which does not comply with these terms.
*Correspondence: Alena Simalatsar, YWxlbmEuc2ltYWxhdHNhckBoZXZzLmNo
Disclaimer: All claims expressed in this article are solely those of the authors and do not necessarily represent those of their affiliated organizations, or those of the publisher, the editors and the reviewers. Any product that may be evaluated in this article or claim that may be made by its manufacturer is not guaranteed or endorsed by the publisher.
Research integrity at Frontiers
Learn more about the work of our research integrity team to safeguard the quality of each article we publish.