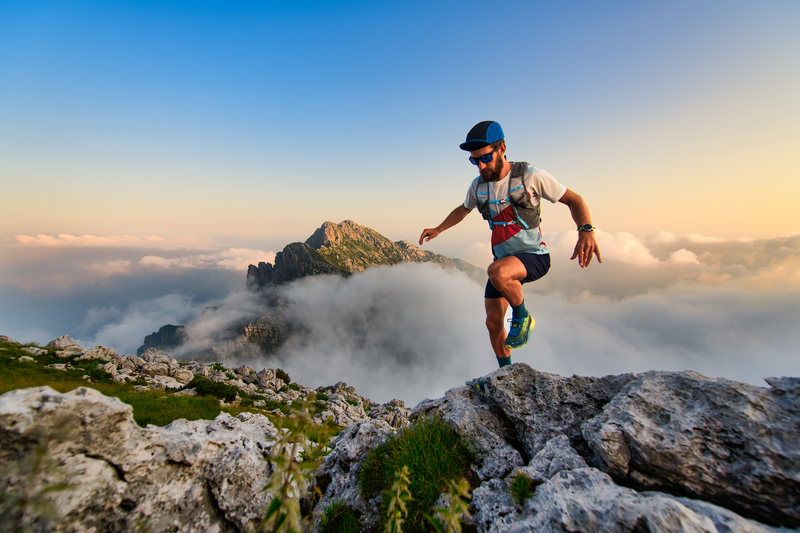
94% of researchers rate our articles as excellent or good
Learn more about the work of our research integrity team to safeguard the quality of each article we publish.
Find out more
ORIGINAL RESEARCH article
Front. Med. Eng. , 07 May 2024
Sec. Advanced Technologies for Medicine
Volume 2 - 2024 | https://doi.org/10.3389/fmede.2024.1374272
This article is part of the Research Topic Bridging the Gap: Understanding Adoption and Acceptance of Emerging Healthcare Technologies View all 3 articles
Introduction: This study examines how online technologies can support patients and their families address specific medical needs by creating an integrated theoretical framework based on the Health Belief Model, Social Exchange Theory, and Technology Acceptance Model.
Methods: We obtained a total of 264 responses from an online survey posted in several COPD Facebook groups. After data preprocessing, we were left with 155 responses that met our inclusion criteria. Using the Partial Least Squares-Structural Equation Model (PLS-SEM), we evaluate how individuals in Chronic Obstructive Pulmonary Disease (COPD) Facebook groups seek information.
Results: This study examines the relationship between demographic variables, psychological attributes (including emotional support and self-worth), perceived disease severity, benefits and barriers, and the perceived utility and ease of internet use in the information-seeking behavior of COPD patients on Facebook.
Discussion: The findings underscore the importance of ease of internet use and intuitive platform design as a critical determinant that positively affects user engagement and fosters active participation among online participants. These findings offer critical insights into how online communities help patients and families obtain broad support and enhance the adoption and acceptance of emerging healthcare technologies. This also shows the advantages that online platforms offer individuals managing chronic diseases. Given these insights, we urge healthcare providers to recognize the beneficial effects of online health communities on patient well-being and health outcomes. This understanding is essential for healthcare professionals seeking to enhance patient care, aiming to improve the quality of life for individuals with chronic diseases in an increasingly digital landscape.
This study looks into how individuals are becoming increasingly reliant on online social media platforms to obtain health information in dealing with chronic diseases, such as COPD. Using the theoretical frameworks of the Health Belief Model (Rosenstock, 1974), Social Exchange Theory (Homans, 1958), and the Technology Acceptance Model (Davis, 1989), we look at different constructs included in these frameworks to understand what impacts the acceptance and effectiveness of these online platforms. The purpose of the Health Belief Model, a psychological and behavior theory, was developed to explain and predict health behaviors by looking at individuals’ attitudes and beliefs toward health-related issues. The HBM focuses on the failure of people to consent to disease prevention programs. The Social Exchange Theory suggests that human relationships are created through a course of cost-benefit analysis, meaning the relationship is worth the effort. Lastly, looking through the lens of perceived ease of use and perceived usefulness, the Technology Acceptance Model examines human’s intention to use new technology. Through these frameworks, this research aims to comprehend and explain the role of digital health communities in disease management and, in the broader sense, the adoption of healthcare technology.
The widespread adoption of digital platforms for health information reflects a significant shift toward technology acceptance in healthcare. A substantial portion of U.S. adults, approximately twenty-four percent of adults report utilizing social media platforms on a weekly basis to seek health-related information or advice (Lopes, et al., 2023), with platforms like Facebook serving as a primary resource (Jeyaraman, et al., 2023). For individuals with severe health conditions, specialized online communities have become crucial support communities, with studies indicating that a majority engage with condition-specific content, and many participate in sharing this information (Ashtari and Taylor, 2023). Google’s health-related searches, which represent a sizeable fraction of its daily queries, further underscore the public’s reliance on technology for health information (Prasad, 2023). Facebook, with its 2.09 billion daily users in the first month of 2024 (McLachlan, 2024), illustrates the platform’s significant role in the digital health revolution. The increase is indicative of the growing acceptance of Web 2.0 and Web 3.0 technologies for health information-seeking (Pew Research Center, 2021). These platforms, particularly Facebook groups, provide a supportive environment for individuals with chronic conditions like diabetes, cancer, and COPD, facilitating not just information exchange but also emotional support (Raylan and Andrews, 2023).
This study examines the effectiveness of online information-seeking, with a focus on understanding the adoption and acceptance of emerging healthcare technologies. By employing Partial Least Squares-Structural Equation Modeling (PLS-SEM), the research is framed within the context of the Health Belief Model (Rosenstock, 1974), Social Exchange Theory (Homans, 1958), and Technology Acceptance Model (Davis, 1989).
The prevalence of Chronic Obstructive Pulmonary Disease (COPD) and its significant health burden worldwide highlight the necessity for and reliance on online health communities (OHCs) for disease management and support (Global Initiative for Chronic Obstructive Lung Disease Incorporated, 2024). According to the Centers for Disease Control and Prevention, (2023), COPD is prevalent in approximately 6.5% or 14.2 million US adults in 2021. COPD is a debilitating disease where those who are afflicted with the disease often experience shortness of breath, which triggers anxiety, which causes more shortness of breath. This vicious circle makes many patients homebound, which makes patients less active, and causes weaker lungs, adding to this vicious cycle. Take, for instance, a post from one of the Facebook participants. “I’m going to use what little energy I have today to breathe and maybe blink. That’s about it.” Another aspect of the disease is that many patients have questions that arise and need answers in their time of need. Take, for instance, a Facebook post at two in the morning. “Do you take Dialresp? Are you experiencing heart palpitations? Blurred vision? Diarrhea? Yea, me too.” Luckily, this person was told to go to the Emergency Room. In light of this and many others just like it in these Facebook groups, our research delves into the specific attributes of online technologies that support effective information-seeking behavior around COPD patients.
These OHCs are increasingly researched for their role in fostering an environment conducive to the exchange of valuable health information, leading to improved outcomes such as better disease management, self-efficacy, and overall quality of life (Mo and Coulson, 2014; Atanasova et al., 2018; Feng et al., 2021; Sun et al., 2023). The adoption of these platforms is a testament to the growing acceptance of emerging technologies in healthcare, as they empower individuals to make informed health decisions (Li et al., 2019). This acceptance is becoming increasingly crucial as healthcare systems continue to integrate more technology-driven solutions.
Understanding the nuances of demographic influences on health technology acceptance is critical. This study examines the interplay of age, marital status, education level, and income in relation to emotional support, reputation, and self-worth within COPD Facebook groups (Bijak et al., 2018). Our hypotheses posit correlations between these demographics and the psychosocial constructs:
H1:. There is a correlation between user demographics and emotional support.
H2:. There is a correlation between user demographics and reputation.
H3:. There is a correlation between user demographics and self-worth.
Drawing on Social Exchange Theory (SET), we explore the reciprocal exchange of support and information in online health communities (Homans, 1958; Yan et al., 2016). These exchanges include both tangible and intangible resources, with knowledge sharing being a pivotal activity where costs and benefits are weighed (Yan, et al., 2016). Benefits are the positive component of the interaction. Benefits include the meeting of informational needs, increased personal reputation, provision of social support, gratifying the sense of self-worth, and increased personal reputation. Information need is a question that needs an answer. Participating in an online health community is a tool that patients use to get questions answered in a timely and efficient manner and has become an important source for searching and exchanging information, advice, support, and experiences (Atanasova et al., 2018). Social support is also a positive exchange that enhances the participant’s welfare and allows participants to interact with others with similar diseases. Social support includes but is not limited to socialization, emotional support, and companionship (Wang et al., 2017). A sense of self-worth captures how individuals see themselves as providing value through knowledge sharing (Bock et al., 2005). Lastly, reputation refers to a person’s perception of earning the respect of other participants in the group (Yan et al., 2016). The barriers or costs are the negatives in the interaction and can include cognitive or executional costs of acting. These negatives diminish the social exchange within the OHC. In SET, as it relates to online health communities, costs present themselves in both executional and cognitive forms. Executional costs include resources like time, materials, and money. Cognitive costs are the process of the participant retrieving information from memory. By retrieving information from the past, feelings such as irritation, panic, or depression could be reimagined by the participant (Yan et al., 2016). The constructs being implemented from this theory are emotional support, reputation, and sense of self-worth. Emotional support is obtained in online health communities by helping participants reduce feelings of anxiety, receive social support, and receive comfort and care (Yan et al., 2016). Emotional support is showing (either verbally or nonverbally) another human being’s care and compassion (Villines, 2022). Thus, we propose the following hypotheses:
H4:. There is a correlation between user emotional support and perceived disease severity.
H5:. There is a correlation between user emotional support and perceived benefits.
H6:. There is a correlation between user emotional support and perceived barriers.
H7:. There is a correlation between user emotional support and usefulness.
H8:. There is a correlation between user emotional support and perceived ease of use.
Reputation refers to a participant’s perception of the potential to earn respect or elevate one’s social status by participating in a Facebook group. Participants build their reputation by sharing their expertise in disease-specific online communities. We, therefore, propose that if a participant believes they have a good reputation within the group, there will be enhanced knowledge sharing within the Facebook group (Yan et al., 2016).
H9:. There is a correlation between user reputation and perceived disease severity.
H10:. There is a correlation between user reputation and perceived benefits.
H11:. There is a correlation between user reputation and perceived barriers.
H12:. There is a correlation between user reputation and perceived usefulness.
H13:. There is a correlation between user reputation and perceived ease of use.
Sense of self-worth describes the extent to which participants see themselves providing value to the online community by sharing their knowledge with the community. Participants realize that sharing information makes them feel more confident in their standing within the Facebook group; therefore, their involvement in the online community improves (Yan et al., 2016). Thus, we propose the following:
H14:. There is a correlation between user self-worth and perceived disease severity.
H15:. There is a correlation between user self-worth and perceived benefits.
H16:. There is a correlation between user self-worth and perceived barriers.
H17:. There is a correlation between user self-worth and perceived usefulness.
H18:. There is a correlation between user self-worth and perceived ease of use.
The Health Belief Model (HBM) provides a lens through which to understand individual actions to prevent disease. Rosenstock (1974) based his HBM on Lewin’s (1951) idea of ‘valence,’ which refers to forces that pull an individual toward a desirable object, not an undesirable one. Rosenstock later expanded the model to understand why people did not participate in public health programs provided by the Public Health Service between the 1950s and 1960 (Rosenstock, 1974). At the time, it was evident that the public was unwilling to accept disease preventatives and screening tests for early detection of diseases like Tuberculosis (TB), cervical cancer, polio, and dental disease (Rosenstock, 1974). Rosenstock posits that for an individual to take action to avoid an undesirable object (disease), he would first need to believe that he was susceptible to the disease and that some section of his life would suffer from some severity. Furthermore, the individual would trust that taking disease prevention actions or screening would create a positive outcome and that this action would not have any perceived barriers like embarrassment, inconvenience, pain, or cost (Rosenstock, 1974). According to Rosenstock, an individual’s emotional weight balances the perceived costs and benefits of the recommended action (Abraham and Sheeran, 2015). The constructs being implemented from this theory are perceived severity, perceived benefits, and perceived barriers. We hypothesize that these perceptions significantly correlate with the effectiveness of health information-seeking behavior. Perceived severity is a participant’s beliefs about the seriousness and magnitude of the threat brought on by their disease. The participants may also be concerned with the effects of the disease, like physical deterioration (Rosenstock, 1974). We propose:
H19:. There is a correlation between perceived disease severity and health information-seeking effectiveness.
Perceived benefits are the users’ perception that a particular course of action will effectively reduce disease threats. Rosenstock (1974) states that perceived benefits can also be influenced by the pressures and norms of their social group. We therefore propose:
H20:. There is a correlation between perceived benefits and health information-seeking effectiveness.
Perceived Barriers are ideas that a course of action is inconvenient, difficult to implement, or not helpful in managing their disease. Rosenstock (1974) asserts that for an action to be followed through, the barriers (negative) must be far less than the benefit (positive). If the negative has a higher value than the positive outcome, the participant will believe the action to be highly unpleasant and will not find participation beneficial. (Ha19-Ha21). Thus, we propose:
H21:. There is a correlation between perceived barriers and health information-seeking effectiveness.
Furthermore, the Technology Acceptance Model (TAM) informs our understanding of the acceptance and use of online health communities as technological systems (Davis, 1989; Ahadzadeh et al., 2015). To understand these relationships, TAM calculates participants’ behavior through perceptions of usefulness, ease of use, and subsequent system adoption (Lazard et al., 2016). An online community is a peer-to-peer technology that connects patients, caregivers, and healthcare providers through the Internet (Hodgkin et al., 2018). Using the TAM framework, research showed that perceived usefulness, perceived ease of use, and attitude positively influence behavioral intention to use the technology (Wong et al., 2014). A positive attitude toward technology, good technological infrastructure, and a user-friendly interface will enhance the likelihood of adoption and usage of the online resource. TAM is being used to view user behavior related to exchanges within online health communities from the technology perspective, which in this study is driven by the OHC. (Ahadzadeh et al., 2015). The constructs implemented from this model are perceived usefulness and perceived ease of Internet use. Perceived usefulness (PU) is the degree to which a person believes that a particular system (online health community) will enhance their quality of life (Davis, 1989). A system (OHC) must enhance or benefit the users. In turn, this would affect the degree to which users participate in the Facebook group. Lastly, perceived ease of Internet (PEIU) use is the degree to which a person believes that using a particular system (online health community) would be free of effort (Ahadzadeh et al., 2015). We propose that perceived usefulness and ease of use are pivotal determinants of participants’ engagement with these communities. Keeping with this theory, we propose:
Ha22:. There is a correlation between perceived usefulness and health information-seeking effectiveness.
Ha23:. There is a correlation between perceived ease of use and health information-seeking effectiveness.
The dependent variable of our study is information-seeking effectiveness, which encompasses the value of the information exchange in terms of social support, accuracy, and relevance to patient care (Gustafson and Shelkhm, 1999; Nambisan, 2011). More and more health-related websites are available to provide patients with answers to medical questions and help them manage their health. This is a shift from sole reliance on physicians to greater personal access to medical information and improved personal health management. Today, there is greater acceptance of the patient as no longer a passive information recipient (Gerber and Eiser, 2001). Patients are now encouraged to be active participants in their healthcare. The Internet offers patients new access to medical information, and the most significant impact on medical decision-making may come from increased access to medical information prior to the physician-patient encounter. As patients increasingly turn to online platforms for health information, it is essential, as researchers, to gain more knowledge in the area of health information exchange, particularly in peer-to-peer health exchanges (Gerber and Eiser, 2001). Information obtained by the patient must be accurate, timely, and medically appropriate to positively affect patient health outcomes, which is key to quality healthcare (Ciaglia, 2017).
In sum, this study’s integrated model, guided by SET, HBM, and TAM, elucidates the correlations between demographic constructs, psychosocial constructs, and technological acceptance, thereby contributing to a comprehensive understanding of the adoption and acceptance of emerging online healthcare technologies. Based on the above hypotheses and following the Health Belief Model, Social Exchange Theory, and TAM, Figure 1 represents the proposed model for this study.
Figure 1 outlines the foundational conceptual framework for health information-seeking behavior, with constructs such as Demographics, Emotional Support (ES), Self-worth (SW), Reputation, and how they potentially influence other factors like Perceived Benefits (PBene), Perceived Barriers (PBarr), Perceived Usefulness (PU) and Information-seeking Effectiveness (ISE).
This section describes the methodology and research design for the study. The survey was based on the theoretical models and disseminated through Qualtrics. The sample size was determined in accordance with Hatcher’s recommendations, and all responses were on a voluntary basis with no compensation. Preprocessing entailed cleaning and classifying replies for analysis. SmartPLS was selected for its suitability for small samples and non-normally distributed data. The data-gathering method was comprehensive, guaranteeing privacy and anonymity.
The purpose of this research is to examine how members of COPD Facebook groups seek and utilize disease-specific information for personal health management. To achieve this, we designed a survey to assess the effectiveness of the online health community model for individuals with COPD to obtain accurate and reliable health information. This model is anchored in the principles of Social Exchange Theory, Health Belief Model, and the Technology Acceptance Model (TAM), forming a modified framework for evaluating technology acceptance among COPD patients.
In line with the conceptual constructs, a 30-item questionnaire was crafted to gather data on the proposed model’s effectiveness among COPD Facebook groups. Employing a five-point Likert scale, recognized for its robustness in gauging participant attitudes since 1932 (Likert, 1932), we assessed all constructs except demographic data, which were measured using categorical scales. The survey was operationalized through Qualtrics, an intuitive online platform facilitating global survey distribution and data collection (Schmidt, 1997).
A pilot test was conducted with Ph.D. candidates specializing in online communities and faculty experts in health communication to validate the survey’s face validity. Based on their feedback, adjustments were made to refine the survey’s clarity and comprehensiveness. The estimated completion time was set at 5–10 min to ensure thorough engagement without causing respondent fatigue. IRB approval was secured from the University of North Texas Institutional Review Board. Tables 1, 2 give information on each construct, code, and questions used in the survey.
In the assessment of participant demographics, Table 1 illustrates the four key questions used in the Facebook survey, capturing the essential demographic data including age, gender, marital status, and income.
Table 2 lists the constructs used in the survey along with their respective sources and the number of questions pertaining to each construct.
Following Hatcher’s (1994) guideline, the sample size was determined to require at least 150 responses to ensure adequate statistical power for the 30 survey items. Recruitment was executed through announcements in COPD Facebook groups, directing participants to the Qualtrics survey. Participation was voluntary with no compensation for participation in the Qualtrics survey. Privacy and anonymity were strictly maintained; no personal health identifiers were collected.
Data collection was initiated in October 2019 across several COPD Facebook groups, with subsequent rounds following due to initial low response rates. By April 2020, a total of 264 responses were received.
In the preprocessing stage, data cleansing included a systematic process where duplicated entries were identified and removed using Excel’s “Remove Duplicates” function. Spelling errors in the data were corrected using Excel’s “Find and Replace” function. Lastly, the data de-identification was performed, complying with the university’s ethical standards. This de-identification removed all personal identifiers without compromising data integrity.
Of the 264 responses, 155 met our inclusion criteria. Demographic data was sorted and categorized based on predetermined classifications, which assisted subgroup analysis. Responses in the survey utilized a Likert scale of one to five to reflect the respondent’s responses. Data validation procedures were addressed, including range checks, consistency assessments, and checking for anomalies.
SmartPLS (v.3.2.9) was used for data analysis to conduct structural equation modeling that uses the partial least squares (PLS) modeling method. SmartPLS is an ideal tool for exploratory research and theory validation (Hair et al., 2017). It is designed to optimize computational efficiency, which allows for shorter run times and faster convergence compared to covariance-based SEM software. Other alternative SEM software, such as AMOS of Mplus, also offers similar functionalities. However, SmartPLS was preferred over other software since it is well-suited for research with small sample sizes (Hair et al., 2017).
Another justification for using SmartPLS is that Structural Equation Modeling (SEM) allows for modeling where data does not follow a normal (Gaussian) distribution. Alternative SEM methods assume that the underlying data are normally distributed. When data are normally distributed, they affect other estimation methods, such as Maximum Likelihood Estimation. However, most data deviate from normal distribution. Given the nature of our constructs, this feature was critical for our study.
In summary, SmartPLS provided a balance of user-friendliness, computational efficiency, and the ability to analyze data that does not follow a normal distribution required for our research. This justification aligns with our methodological choices and the objectives of our study.
In this section, it is noted that most participants are females between the ages of 55–74. Using SmartPLS, the results show that reputation was statistically insignificant and was eliminated from the model. Most constructs met Cronbach’s Alpha metric threshold, and the model fit well according to root mean square standards. The hypothesis testing showed significant results for the majority of constructs.
The analysis of the demographics of participants revealed that 76.8% of the respondents were female, 22.6% were males, and 0.6% noted “other” for their gender. Of the 155 participants, 0.6% were below 30 years of age, 11.0% were between 31 and 54, 44.5% were between 55 and 64, and another 34.8% were between 65 and 74. Lastly, 9% were over the age of 75. Most respondents were married (45.8%), 26.5% were divorced, 14.2% were single, and 13.5% were widowed. The last demographic to be recorded was income. The majority of people (71.6%) had less than $49,999 reported income, then $50,000 to $99,999, and then 5.2% reported over $100,000 income.
The following analysis was run using SmartPLS v. 3.2.9. Figure 1 illustrates the initial model with all constructs. The model is read from left to right, with each construct on the left being the independent construct(s) to the dependent construct(s) to the right. Thus, the independent construct(s) on the left predicts the dependent construct(s) to the right. We can see by the p-values that reputation was not significant, except in one construct (PS), and therefore was eliminated from the final model.
Figure 2 provides a quantitative analysis of the conceptual framework, showing the statistical relationships between the constructs. Each path in the model is annotated with a path coefficient representing the strength between variables and a p-value representing the significance of the relationship between the variables. Table 3 gives further details of the model illustrated in Figure 2, providing the adjusted R-squared values, path coefficients, and p-values for the relationships between the constructs in the proposed model.
The p-values for the construct reputation are not statistically significant, so we cannot reject the null hypothesis. Therefore, we will eliminate this construct from the new proposed model.
Figure 3 is the new proposed model. This model illustrates the path coefficients, p-values, and adjusted R2 values. Bootstrapping was applied to this ISE model to test the structural model. Once completed, the construct and reputation were removed. Bootstrapping is a nonparametric method that tests the significance of the model by creating randomly selected observations and comparing these random sets against the initial set of data. Bootstrapping was applied using 5,000 bootstrapped samples.
Figure 3 provides a quantitative analysis of the conceptual framework (without Reputation), showing the statistical relationships between the constructs. Each path in the model is annotated with a path coefficient representing the strength between variables and a p-value that represents the significance of the relationship between the variables.
The efficiency of a model is determined by evaluating the reliability and validity of each construct (Kerlinger and Lee, 2000). Table 4 shows all constructs from the model with the corresponding adjusted R2, average variance extracted (AVE), rho A, Cronbach’s Alpha, and Composite Reliability. The first value in this table is the Adjusted R2 (0.69.1). This value explains the variance between the latent variables; we see that this model explains 69.1% of the variance. The average variance extracted (AVE) value measures the convergent validity of the variance between constructs. An acceptable AVE should be higher than 0.50. Values with less than 0.50 have more variance in the error of the items than in the variance explained by the variable (Hair et al., 2017). Variables that are above 0.050 indicate there is strong convergent validity. Two measures in our model fall beneath this accepted value but fall within the less restrictive criteria from Cronbach’s Alpha and rho A values (Salkind, 2010).
Table 4. Proposed new model with convergent validity including the construct, adjusted R-squared, AVE, Cronbach’s Alpha, rho_A, and composite reliability.
Cronbach’s Alpha is another tool that measures the reliability of the scale (Salkind, 2010). An accepted Cronbach’s Alpha is 0.70, and 0.60 is the lowest acceptable threshold (Salkind, 2010). As we see in Table 4, all constructs fall within the range. Therefore, we can assume the constructs observed score variance is close to that of the true score.
Lastly, we analyzed the fit of the model with the root mean square (SRMR). This value was 0.068, below the accepted value of 0.08 (Valaei and Baroto, 2017). This calculation is reasonably new to PLS-SEM, but it is well-known in covariance-based structural equation modeling (Hair et al., 2017). SRMR is a tool to analyze model fit that measures the difference between observed and model-suggested correlations.
To validate the hypotheses, p-values <0.05 were employed (Table 5). Table 5 presents the path coefficients and p-values for the relationships between various constructs related to information-seeking effectiveness. From this analysis, we can see that all constructs are statistically significant (p-values) except for the relationship between Self-worth and Perceived Benefits. We can also see that of the constructs, Perceived Ease of Use, Perceived Usefulness, and Perceived Benefits have the highest statistical significance. Path analysis confirms our hypotheses in all constructs except reputation. (There is one correlation in reputation that showed a weak negative correlation with perceived severity).
There were a few constructs that had weak negative significance effects. These are demographics on emotional support (b = −0.202, p = 0.000) and self-worth (b = −0.427, p = 0.000); self-worth on perceived severity (b= −0.066, p = 0.000); perceived severity on information seeking effectiveness (b= −0.012, p = 0.000); and perceived benefits on information seeking effectiveness (b= −0.103, p = 0.000). The top five constructs with the strongest positive relationships are emotional support on perceived usefulness (b= 0.706, p = 0.000). Emotional support on perceived benefits (b= 0.698, p = 0.000), self-worth on perceived ease of internet use (b= 0.525, p = 0.000), emotional support on perceived barriers (b= 0.490, p = 0.000), and perceived ease of internet use on information seeking effectiveness (b= 0.469, p = 0.000).
This study substantiates several hypotheses within the framework of the Health Belief Model, Social Exchange Theory, and Technology Acceptance Model, contributing to the discourse on the adoption and acceptance of emerging healthcare technologies such as social media. Statistical evidence supports the significance of perceived severity, benefits, barriers, emotional support, and self-worth in the context of health information-seeking behavior among COPD patients using Facebook groups. However, the construct of reputation did not show a significant correlation and was subsequently removed from the model, underscoring the need to refine our understanding of how reputation influences technology acceptance in health-related online communities.
The findings indicate that user demographics do correlate with constructs of emotional support and self-worth, both showing negative correlations. This suggests that demographic constructs may influence the psychological support received from and participation in online health communities, which can inform targeted interventions to enhance user engagement.
Emotional support and self-worth demonstrate positive correlations with perceived disease severity, benefits, barriers, usefulness, and ease of Internet use. These relationships highlight the role of emotional and self-evaluative constructs in how users perceive and interact with healthcare technology, which is crucial for designing user-centric health information systems.
A weak but statistically significant positive relationship was found between perceived disease severity and information-seeking effectiveness. This suggests that while severity is recognized, it may not be the primary driver for seeking information online.
Both constructs correlate positively with information-seeking effectiveness, indicating that recognizing the advantages of and overcoming obstacles to using online health information are integral to patient engagement and effective self-management.
Strong positive relationships with information-seeking effectiveness were observed for both perceived usefulness and ease of Internet use. These findings affirm that the practicality and user-friendliness of online health resources are critical determinants in their adoption and sustained use by patients.
The insights gained from this study underscore the importance of considering a range of psychosocial and perceptual constructs when aiming to bridge the gap in technology adoption and acceptance in healthcare. As we move towards an increasingly digital healthcare environment, understanding these dynamics can aid in the development of more inclusive, supportive, and accessible online health communities that cater to the needs and preferences of diverse patient populations.
By aligning technology design and implementation with the identified constructs that influence information-seeking behavior, healthcare providers and technologists can enhance the effectiveness of online health resources, thereby empowering patients in their health management and potentially improving health outcomes.
A theoretical evaluation approach for information-seeking behavior in disease-specific online forums is presented in this study. Although this study has limitations since it relies on self-reported data obtained from survey responses (Podsakof and Organ, 1986), which may introduce bias, it nevertheless substantially contributes to our understanding of how healthcare innovations are adopted and accepted. Furthermore, the study’s conclusions will not apply to COPD patients who do not use Facebook. Lastly, different groups within a single social media platform will have different compositions of participants, such as mostly patients versus providers versus family member support groups, etc., and each will have different needs and cultures, each requiring a dedicated study.
These limitations might be overcome in future studies by including different online communities or COPD patients who avoid social media. Finally, additional diseases could be studied in order to expand the generalization to chronic illnesses beyond COPD.
In the digital age, where technology increasingly mediates healthcare delivery, this study stands as a testament to the pivotal role of online health communities in enhancing patient care for conditions such as Chronic Obstructive Pulmonary Disease (COPD). We have identified key factors that drive effective engagement with health technologies by examining demographics, psychological characteristics such as emotional support and self-worth, and users’ perceptions about disease severity, benefits, barriers, usefulness, and ease of internet use.
The findings reveal that user engagement with health technologies is not merely a function of the technologies themselves but is intricately linked to the personal and psychological factors of the users. Significantly, the ease of using online platforms emerged as a pivotal factor, enhancing user adoption and acceptance. Thus, user-friendly design is paramount for the success of health information systems.
Our study also acknowledges the unique challenges faced by patients and caregivers who may be reticent to express their health concerns openly. Online forums offer a distinct advantage for these individuals by providing a less intimidating platform for communication as compared to traditional face-to-face interactions. This is especially beneficial for shy individuals or those with communication apprehensions, as they may find the anonymous or pseudonymous nature of online interactions a safer avenue for expressing their concerns and seeking information (Mo and Coulson, 2014; Urbanova et al., 2023).
The correlation between these constructs and information-seeking behavior underscores the necessity for healthcare technologists and professionals to prioritize intuitive design and address the psychosocial needs of users. Such insights are crucial in bridging the technological gap and ensuring that emerging healthcare technologies are not only accepted but also effectively adopted by those with chronic illnesses.
Moreover, this study highlights the importance of online health communities in supporting the ongoing management and improving the health outcomes of COPD patients. Healthcare professionals, developers, and manufacturers of such technology must acknowledge and integrate the utility of these platforms into comprehensive care strategies, facilitating an environment where technology becomes a seamless part of patient empowerment and disease management.
In conclusion, by fostering a deeper understanding of the interplay between user characteristics and technology use, this research contributes to the development of more effective, empathetic, and user-centered health information technologies, ultimately aiming to enhance the quality of life for patients with disease-specific conditions such as COPD.
The datasets presented in this article are not readily available because this data is kept in a secured location as stated in the IRB through the University of North Texas. Requests to access the datasets should be directed to LeAnn Boyce, bGVhbm4uYm95Y2VAdW50LmVkdQ==.
The study was conducted according to the guidelines of the Declaration of Helsinki and approved by the Institutional Review Board of the University of North Texas (IRB-19-176, dated 6-17-2020). The studies were conducted in accordance with the local legislation and institutional requirements. The participants provided their written informed consent to participate in this study.
LB: Conceptualization, Data curation, Formal Analysis, Investigation, Methodology, Project administration, Resources, Software, Validation, Visualization, Writing–original draft, Writing–review and editing. GP: Supervision, Writing–review and editing. VP: Supervision, Writing–review and editing.
The author(s) declare that no financial support was received for the research, authorship, and/or publication of this article.
The authors declare that the research was conducted in the absence of any commercial or financial relationships that could be construed as a potential conflict of interest.
All claims expressed in this article are solely those of the authors and do not necessarily represent those of their affiliated organizations, or those of the publisher, the editors and the reviewers. Any product that may be evaluated in this article, or claim that may be made by its manufacturer, is not guaranteed or endorsed by the publisher.
Abraham, C., and Sheeran, P. (2015). “The health belief model,” in Predicting and changing health behavior. Editors M. Conner, and P. Norman (New York, NY, United States: McGraw-Hill).
Ahadzadeh, A., De, M., Sharif, S., and Ong, F. (2015). Integrating health belief model and technology acceptance model: an investigation of health-related internet use. J. Med. Internet Res. 17, 1–17. doi:10.2196/jmir.3564
Ashtari, S., and Taylor, A. (2023). Patients with rare diseases and the power of online support groups: implications for the medical community. JMIR Form. Res. 7 (7), e41610. doi:10.2196/41610
Atanasova, S., Kamin, T., and Petric, G. (2018). The benefits and challenges of online professional-patient interaction: comparing views between users and health professional moderators in an online health community. Comput. Hum. Behav. 83 (83), 106–118. doi:10.1016/j.chb.2018.01.031
Bijak, J., Courgeau, D., Franck, R., and Silverman, E. (2018). Methodological investigations in agent-based modelling. Berlin, Germany: Springer. doi:10.1007/978-3-319-72408-9_9
Bitto Urbanova, L., Madarasova Geckova, A., Dankulincova Veselska, Z., Capikova, S., Holubcikova, J., van Dijk, J. P., et al. (2022). Technology supports me: perceptions of the benefits of digital technology in adolescents. Front. Psychol. 13, 970395. doi:10.3389/fpsyg.2022.970395
Bock, G., Zmud, R. W., Kim, Y., and Lee, J. (2005). Behavioral intention formation in knowledge sharing: examining the roles of extrinsic motivators, Social-Psychological Forces, and Organizational Climate. MIS Q. 29 (1), 87–111. doi:10.2307/25148669
Centers for Disease Control and Prevention (2023). Trends in the prevalence of chronic obstructive pulmonary disease among adults aged ≥18 years – United States. Available at: https://www.cdc.gov/mmwr/volumes/72/wr/mm7246a1.htm#:∼:text=Differences%20by%20Sociodemographic%20Characteristics,had%20COPD%20(Table%201) (Accessed March 3, 2023).
Ciaglia, A. (2017). Why is high quality health information important for patients? Available at: https://www.patientresearchexchange.org/stories/detail/why-is-high-quality-health-information-important-for-patients (Accessed June 23, 2022).
Davis, F. D. (1989). Perceived usefulness, perceived ease of use, and user acceptance of information technology. MIS Q. 13 (3), 319–340. doi:10.2307/249008
Feng, B., Li, X., and Lin, L. (2021). Valenced social identities and the digital divide in online health communities. Comput. Hum. Behav. 122, 106812. doi:10.1016/j.chb.2021.106812
Gerber, B., and Eiser, A. (2001). The patient-physician relationship in the Internet Age: future prospects and the research agenda. J. Med. Internet Res. 3 (3), e15–e17. doi:10.2196/jmir.3.2.e15
Global Initiative for Chronic Obstructive Lung Disease (2024). 2042 GOLD reports. Global initiative for chronic obstructive lung disease - GOLD. Available at: https://goldcopd.org/2024-gold-report/(Accessed January 20, 2024).
Gustafson, D., and Shelkhm, K. (1999). Computer-based support systems for women with breast cancer. J. Am. Med. Assoc. 280 (15), 1305. doi:10.1001/jama.280.15.1305
Hair, J., Thomas, G., Hult, M., Ringle, C., and Sarstedt, M. (2017). A primer on partial least squares structural equation modeling (PLS_SEM). Thousand Oaks, California, United States: Sage Publications.
Hatcher (1994). A step-by-step approach to using the SAS system for factor analysis and structural equation modeling. Cary: SAS Institute, Inc.,
Hodgkin, P., Horsley, L., and Metz, B. (2018). The emerging world of online health communities (SSIR). Stanf. Soc. Innov. Rev., doi:10.48558/9JGH-H283
Jeyaraman, M., Ramasubramanian, S., Kumar, S., Jeyaraman, N., Selvaraj, P., Nallakumarasamy, A., et al. (2023). Multifaceted role of social media in healthcare: opportunities, challenges, and the need for quality control. Cureus 15 (5), e39111. doi:10.7759/cureus.39111
Kerlinger, F., and Lee, H. (2000). Foundations of behavioral research. Wadsworth: United States: Thomson Learning.
Lazard, A. J., Watkins, I., Mackert, M. S., Xie, B., Stephens, K. K., and Shalev, H. (2016). Design simplicity influences patient portal use: the role of aesthetic evaluations for technology acceptance. J. Am. Med. Inf. Assoc. 23 (e1), 157–e161. doi:10.1093/jamia/ocv174
Lewin, K. (1951). Field theory in social science: selected theoretical papers. United States: Harpers.
Li, M., Shi, J., and Chen, Y. (2019). “Analyzing patient decision making in online health communities,” in IEEE International Conference on Healthcare Informatice, Xi'an, China, June, 2019, 1–8.
Lopez, L., Kearney, A., Washington, I., Valdes, I., Yilma, H., and Hamel, L. (2023). KFF health misinformation tracking poll pilot. Available at: https://www.kff.org/coronavirus-covid-19/poll-finding/kff-health-misinformation-tracking-poll-pilot/[Accessed January 20, 2024].
McLachlan, S. (2024). 45 Facebook statistics marketers need to know in 2024. Available at: https://blog.hootsuite.com/facebook-statistics/#:∼:text=The%20number%20of%20daily%20active,that’s%20up%205%25%20since%202023 (Accessed January 20, 2024).
Mo, P. K. H., and Coulson, N. S. (2014). Are online support groups always beneficial? A qualitative exploration of the empowering and disempowering processes of participation within HIV/AIDS-related online support groups. Int. J. Nurs. Stud. 51 (7), 983–993. doi:10.1016/j.ijnurstu.2013.11.006
Nambisan, P. (2011). Information seeking and social support in online health communities: impact on patients’ perceived empathy. J. Am. Med. Inf. Assoc. 18 (18), 298–304. doi:10.1136/amiajnl-2010-000058
Pew Research Center (2021). Internet/broadband fact sheet. Available at: https://www.pewresearch.org/internet/fact-sheet/internet-broadband/(Accessed January 2, 2023).
Podsakoff, P., and Organ, D. (1986). Self-reports in organizational research: problems and prospects. J. Manag. 12 (4), 531–544. doi:10.1177/014920638601200408
Prasad, A. (2023). Local SEO for doctors during the modern patient journey. Available at: https://www.forbes.com/sites/forbesagencycouncil/2023/10/05/local-seo-for-doctors-during-the-modern-patient-journey/?sh=673d613f6635 (Accessed January 20, 2024).
Rayland, A., and Andrews, J. (2023). From social network to peer support network: opportunities to explore mechanisms of online peer support for mental health. JMIR Ment. Health 10, e41855. doi:10.2196/41855
Rosenstock, I. (1974). Historical origins of the health belief model. Health Educ. Monogr. 2 (2), 328–335. doi:10.1177/109019817400200403
Salkind, N. J. (2010). Encyclopedia of research design. Thousand Oaks, California, United States: SAGE Publications, Inc., doi:10.4135/9781412961288
Schmidt, W. (1997). World-Wide Web survey research: benefits, potential problems, and solutions. Behav. Res. Methods, Instrum. Comput. 29 (29), 274–279. doi:10.3758/BF03204826
Sun, Z., Zang, G., Wang, Z., Ge, S., Liu, W., and Wang, K. (2023). Determining factors affecting the user's intention to disclose privacy in online health communities: a dual-calculus model. Front. Public Health 11, 1109093. doi:10.3389/fpubh.2023.1109093
Valaei, N., and Baroto, M. (2017). Modelling continuance intention of citizens in government Facebook page: a complementary PLS approach. Comput. Hum. Behav. 73 (73), 224–237. doi:10.1016/j.chb.2017.03.047
Villines, Z. (2022). How to show emotional support: tips and examples. Available at: https://www.medicalnewstoday.com/articles/emotional-support (Accessed January 20, 2023).
Wang, X., Zhao, K., and Street, N. (2017). Analyzing and predicting user participations in online health communities: a social support perspective. J. Med. Internet Res. 19 (4), e130. doi:10.2196/jmir.6834
Wong, C., Yeung, D., Ho, H., Tse, K., and Lam, C. (2014). Chinese older adults’ Internet use for health information. J. Appl. Gerontology 33 (3), 316–335. doi:10.1177/0733464812463430
Keywords: COPD facebook participants, online forums, PLS-SEM, information-seeking effectiveness model, text mining, healthcare technology
Citation: Boyce L, Prybutok G and Prybutok V (2024) Online groups show how technology supports healthcare needs for patients and families: an illustrative model for COPD facebook groups. Front. Med. Eng. 2:1374272. doi: 10.3389/fmede.2024.1374272
Received: 21 January 2024; Accepted: 15 April 2024;
Published: 07 May 2024.
Edited by:
Habib Shah, King Khalid University, Saudi ArabiaReviewed by:
Akhtar Badshah, University of Malakand, PakistanCopyright © 2024 Boyce, Prybutok and Prybutok. This is an open-access article distributed under the terms of the Creative Commons Attribution License (CC BY). The use, distribution or reproduction in other forums is permitted, provided the original author(s) and the copyright owner(s) are credited and that the original publication in this journal is cited, in accordance with accepted academic practice. No use, distribution or reproduction is permitted which does not comply with these terms.
*Correspondence: LeAnn Boyce, bGVhbm4uYm95Y2VAdW50LmVkdQ==
Disclaimer: All claims expressed in this article are solely those of the authors and do not necessarily represent those of their affiliated organizations, or those of the publisher, the editors and the reviewers. Any product that may be evaluated in this article or claim that may be made by its manufacturer is not guaranteed or endorsed by the publisher.
Research integrity at Frontiers
Learn more about the work of our research integrity team to safeguard the quality of each article we publish.