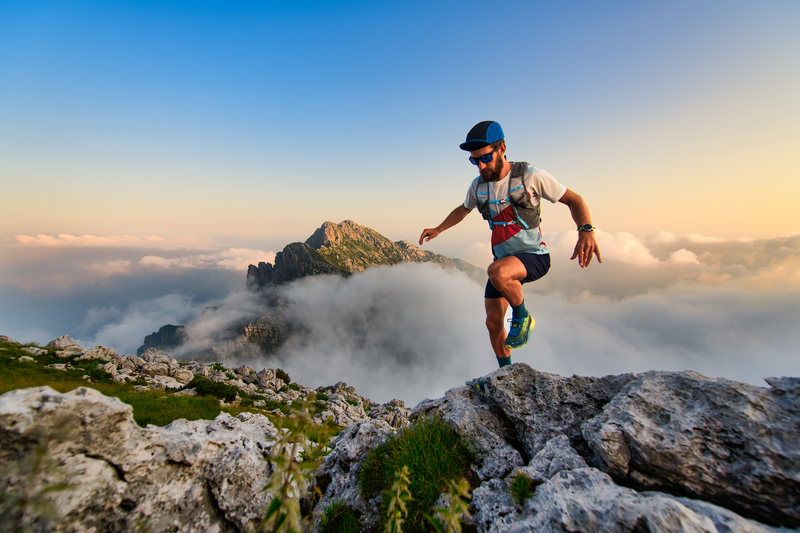
94% of researchers rate our articles as excellent or good
Learn more about the work of our research integrity team to safeguard the quality of each article we publish.
Find out more
SPECIALTY GRAND CHALLENGE article
Front. Med. Eng. , 31 March 2023
Sec. Computational Medicine
Volume 1 - 2023 | https://doi.org/10.3389/fmede.2023.1112763
Medical advances in treatment strategies against age-related global killers such as cardiovascular disease, cancer, and stroke have been responsible for the significant gains in the average global life expectancy observed since the second half of the 20th century (Hunter and Reddy, 2013). Still, medical research has been less successful at prolonging healthy life. Globally, one in three adults live with multiple chronic conditions (Hajat and Stein, 2018). In the US, 80% of the population older than 65 live with at least one chronic condition, and 50% live with two (Hunter and Reddy, 2013). As the aging population is growing rapidly, the incidence of age-related, costly, chronic conditions such as heart disease, cancer, diabetes, and Alzheimer’s is reaching epidemic proportions. In the US alone, healthcare spending is already composing far more of the national gross domestic product than any other sector including defense, education, energy, and transportation (US Government Spending, 2017). With annual total costs of age-related diseases expected to skyrocket, all nations are in pressing need to reduce the economic burden of population aging. Prolonging lifespan without prolonging health span is financially unsustainable for all nations.
Computational medicine emerged in the past decade as an interdisciplinary field dedicated to integrating advanced computational modeling, data-driven technologies, and supercomputing to derive new knowledge about the biological mechanisms of disease and deeper understanding of factors driving inter-patient variability (Bukowski et al., 2021). Such knowledge enables development of precision strategies to diagnose and treat disease, sustain wellbeing, and optimize utilization of healthcare resources (Winslow et al., 2012). Computational medicine has the potential to drive transformative advances in healthcare, extend health span, and reign in healthcare costs by i) enabling a more holistic understanding of the broad spectrum of all factors, processes, and their interplay impacting wellbeing at the individual and the population level, and by ii) translating such understanding into dynamically adaptive, personalized medical decisions to drive effective and sustainable health management practices. There are already several efforts demonstrating the potential of computational medicine across various diseases and conditions (e.g., (Louis et al., 2014; Mulder et al., 2018; Athanasiou et al., 2019; Bukowski et al., 2021; Yu and Kibbe, 2021; Hiram Guzzi et al., 2022; Toma et al., 2022).
To achieve its full potential, computational medicine should be able to build a digital twin of the human by mapping the human genome (i.e., genomic profile), phenome (i.e., physiologic status), and exposome (i.e., physical and social environment) in real-time and across the human lifetime. Understanding the human genome-phenome-exposome interplay is an ambitious endeavor which demands a multi-disciplinary team of biologists, physicists, chemists, engineers, mathematicians, computer scientists, and data scientists. Sitting at the intersection of these scientific domains, computational medicine faces grand challenges in three key areas.
Multiscale computational modeling of complex biological systems has been an active field of research, leading to notable advances in both mechanistic and agent-based disease models. The overarching goal is quantitative representation of interconnected biological processes that cannot be easily delineated experimentally. Such model should be able to capture the spatiotemporal interdependencies across all scales—from genomic, transcriptomic, proteomic, and metabolomic scales to tissue and organ level, and ultimately to the individual and population levels (Bhattacharya et al., 2019; Tiwary, 2020). Recently, the interleaving of traditional computational modeling and simulation with artificial intelligence approaches has emerged as a strong theme to complex systems modeling (Alber et al., 2019; Tolk et al., 2022), with several applications in drug design, structural biology, and neuroscience to name a few (e.g., (Stephan et al., 2015; Huys et al., 2016; Romero et al., 2019; Liu et al., 2021; Paul et al., 2021; Ramanathan et al., 2021).
Since system complexity increases when bridging different scales, there are technical challenges as the number of system parameters rapidly increases. Furthermore, the integration of computational representations across different organ systems remains an outstanding challenge (Beard et al., 2012). Developing efficient computational tools that can manage multi-modal, multi-scale data as well as demonstrate effective utilization of high-performance computing resources are critical for us to overcome current barriers.
One outstanding and ever-increasing challenge with complex multi-scale computational models and the latest artificial intelligence models known as language models or transformers (Vaswani et al., 2017; Wei et al., 2022) is the growing demand for computational power (Liu et al., 2021). Model training, hyper-parameter optimization, uncertainty quantification, and validation become exponentially more demanding as efforts move from single to multiple scales (Renardy et al., 2019). As energy-efficiency becomes a bottleneck for large scale computational science, efficient algorithmic development will be necessary for scalable computational medicine. It has been proposed that a modular approach to multi-scale computational medicine, with interoperable and reusable computational tools, mimicking the first principles computational chemistry and physics modeling approaches maybe appropriate as it has been very successful in materials science (Ford Versypt, 2021). Although biological systems differ from physical systems, there is potential in investing in such effort to derive important building blocks that bridge a few spatiotemporal scales. Still, standards for reproducible research in computational medicine are lacking. Creating and maintaining data and model repositories are critical for ensuring reproducibility and reliability. As many of these endeavors become computationally very intensive, the burden of reproducibility is immense for the average researcher. We need to invest substantial resources in compute-and-data infrastructures to scientific integrity, reproducibility, and reliability of data and models.
The breadth of technical and algorithmic challenges exemplifies the need for strong collaborations across disparate scientific domains, across different and also competing approaches, as well as across different stakeholders. For example, in 2016, the National Cancer Institute (NCI) and the Department of Energy (DOE) in the US partnered in a collaboration to accelerate advances in predictive oncology. The collaboration brought together multidisciplinary experts in the biological, computational, data, and physical sciences to develop, demonstrate, and disseminate advanced computational capabilities that help answer driving scientific questions across molecular, cellular and population scales (Bhattacharya et al., 2019). The community effort is growing by adding new scientific challenges that build upon strong collaborations (Buchsbaum et al., 2022).
The concept of the digital twin has gained a lot of traction within computational medicine (Barbiero et al., 2021; Hassani et al., 2022). The digital twin is a virtual representation of a patient as a multi-modal system which incorporates patient data to inform personalized medical decisions related to disease prediction, diagnosis, therapeutic interventions, and prognosis. A digital twin can be created at different levels of detail (e.g., organ, individual, population, healthcare system) using various data sources as they become available. Merging non-traditional data sources (e.g., environmental factors, socioeconomic conditions, lifestyle choices) with multi-scale patient data, digital twins offer in silico modeling of patient health trajectories by taking into account the complex interplay of all factors and processes that affect wellbeing. Such models can be regularly interrogated to explore different scenarios (e.g., different treatments, lifestyle choices) to predict future risks and outcomes and empower individuals to make decisions at critical times and from the earliest stages in life. Furthermore, such longitudinal models can be dynamically adaptive and updated as new data becomes available. The potential to execute “what-if” scenarios completely in silico can be very empowering for patients, physicians, researchers, and healthcare systems as each tries to optimize outcomes based on individual criteria and incentives. References (Mulder et al., 2022; Okegbile et al., 2022) provides an insightful discussion of the potential of digital twins in the future of medicine while disease-specific examples are emerging (Allen et al., 2021; Hernandez-Boussard et al., 2021).
The clinical implementation of the digital twin in computational medicine is still in its very early stages facing similar challenges as those outlined in the previous section, namely data quality, data integration, reproducibility, reliability, as well as continuous quality assurance due to its dynamic nature.
With computational medicine’s great promise comes an even greater responsibility. We must recognize the pitfalls and possible ill-intended uses of the computational models. Since these models rely heavily on patient data, there are many legal and ethical considerations related to data collection, sharing, and use. Access to large amounts of patient data is fundamental to understand individual and population-level health outcomes over time. However, liberating and providing access to patient data is both a technological and a policy challenge. Furthermore, to create a richer picture, medical data must be combined with other data points to provide context on a patient’s living conditions that has substantial implications in predicting patient health trajectories. Although we all recognize the scientific value of human data, the debate over data ownership is ongoing in terms of how best to balance the promise of transparent innovation with the risks of unethical data handling, intentional or unintentional privacy breaches, and adversarial data use by hostile or malicious actors (Kostkova et al., 2016). To maintain a strong ethical framework for computational medicine, we need to answer this fundamental question: Who owns the intellectual property of data-driven computational models in healthcare? The patient? The medical center collecting the data by providing the healthcare services? Or the model developer? Clearly, no single entity alone could deliver the breakthrough technology.
Next is the topic of data trustworthiness. There is plenty of evidence that low data quality and problematic data representativeness can compromise model validity (Winslow et al., 2012) by creating or exacerbate existing racial or societal biases in healthcare systems (Nordling, 2019). During the development phase, scientists should promote a rigorous statistical framework to monitor for potential biases in the collected data. During deployment phase, model developers should implement rigorous quality control, monitoring model performance across subgroups to confirm robust performance or identify performance gaps. We should work to communicate to patients and healthcare providers openly and clearly what they should expect from the technology so that they are informed consumers of the technology.
Other than data quality, data-driven models benefit from access to large volumes of representative medical data. With the explosive growth of large-scale deep learning models (LeCun et al., 2015), the need for data sharing is pressing. State of the art deep learning models have billions of parameters. Although these models can push boundaries in learning and generalizability (Sun et al., 2017; Wang et al., 2019), they require massive amounts of training data due to their large parameter space. Federated learning has emerged as a successful collaboration mechanism to address the privacy constraint with sensitive data sharing. Instead of sharing data, the collaborative entities share the model parameters after local training and fine tuning (Li et al., 2020). Several studies demonstrated that models trained using federated learning are as accurate as those trained using centrally hosted data sets and they are far more accurate than models trained with single institution data. Nevertheless, federated learning is in its early stages of technical development. There are still outstanding concerns with reverse engineering of the trained “super-model”, and legal implications if the model is broadly shared and used without proper authorizations in clinical care (Rieke et al., 2020; Zawad et al., 2022).
Another grand challenge is that the clinical translation of computational models is not straightforward. If the computational models are used for hypotheses generation or for knowledge discovery, they are easier to embrace as they are used as scientific instruments. However, if computational models are used to perform or assist clinical tasks, then acceptance expectations are much higher. Often these models must be regulated, and they need to clearly demonstrate efficacy (i.e., performance equivalent to medical experts) and safety. Previous experience with AI for clinical decision support demonstrated that AI is capable of performing narrowly defined, repetitive tasks exceptionally well. Still though, if physicians over-rely on such decision support technologies, they may lose critical skills needed for performing more difficult tasks (Darcy et al., 2016). Another challenge with clinical integration is ensuring that the computational model is capable of assessing its confidence (i.e., uncertainty quantification) and providing a justification (i.e., explainability) for its prediction (Begoli et al., 2019; Benrimoh et al., 2021). Since one of the key drivers of the ‘digital twin” is to empower the patients as they try to manage their disease and gain better understanding of the short-term and long-term implications of the decisions they need to make, how to convey the model’s reasoning and prediction confidence to the patient vs the healthcare provider is an understudied topic.
Ultimately, humans and computational models will have to work well together. But this synergy will not happen organically, as past health AI experiences have demonstrated. It is important to train both healthcare providers and patients in how to use computational models responsibly, and how to remain vigilant avoiding mistakes of over-reliance when supported by the models. Objective benchmarking of datasets and models against community consensus metrics to detect, monitor, and possibly correct dataset biases or inconsistent model performance must become part of the practice of computational medicine.
The convergence of personalized digital health technologies, computing power, and artificial intelligence have ushered a new era in healthcare delivery. Computational medicine holds immense promises for personalized disease management, from diagnosis, to treatment, to prognosis. Furthermore, computational medicine has the potential to offer much needed relief in healthcare costs by enabling deeper understanding of the interplay between biological and socioeconomic drivers to promote personalized proactive healthcare approaches, delay onset of chronic diseases, and prolong wellness. There are already numerous successful examples of computational medicine from the bench to the bedside, however several challenges remain to fully realize the potential of computational modeling and personalized data in clinical practice. The complexities of multiscale system modeling, integration and analysis of multimodal personalized data, longitudinal modeling and dynamic system optimization to maximize personal and population level outcomes, as well as practical issues in terms of clinical integration and safe utilization at scale are grand challenges. To support the realization of computational precision medicine we need engagement and collaboration of many scientific domains, given the truly interdisciplinary nature of this endeavor.
The author confirms being the sole contributor of this work and has approved it for publication.
The author declares that the research was conducted in the absence of any commercial or financial relationships that could be construed as a potential conflict of interest.
All claims expressed in this article are solely those of the authors and do not necessarily represent those of their affiliated organizations, or those of the publisher, the editors and the reviewers. Any product that may be evaluated in this article, or claim that may be made by its manufacturer, is not guaranteed or endorsed by the publisher.
Alber, M., Buganza Tepole, A., Cannon, W. R., De, S., Dura-Bernal, S., Garikipati, K., et al. (2019). Integrating machine learning and multiscale modeling-perspectives, challenges, and opportunities in the biological, biomedical, and behavioral sciences. NPJ Digit. Med. 2, 115. doi:10.1038/s41746-019-0193-y
Allen, A., Siefkas, A., Pellegrini, E., Burdick, H., Barnes, G., Calvert, J., et al. (2021). A digital twins machine learning model for forecasting disease progression in stroke patients. Appl. Sci. 11 (12), 5576. doi:10.3390/app11125576
Athanasiou, L., Nezami, F. R., and Edelman, E. R. (2019). Computational cardiology. IEEE J. Biomed. health Inf. 23 (1), 4–11. doi:10.1109/jbhi.2018.2877044
Barbiero, P., Viñas Torné, R., and Lió, P. (2021). Graph representation forecasting of patient's medical conditions: Toward a digital twin. Front. Genet. 12, 652907. doi:10.3389/fgene.2021.652907
Beard, D. A., Neal, M. L., Tabesh-Saleki, N., Thompson, C. T., Bassingtwaighte, J. B., Shimoyama, M., et al. (2012). Multiscale modeling and data integration in the virtual physiological rat project. Ann. Biomed. Eng. 40, 2365–2378. doi:10.1007/s10439-012-0611-7
Begoli, E., Bhattacharya, T., and Kusnezov, D. (2019). The need for uncertainty quantification in machine-assisted medical decision making. Nat. Mach. Intell. 1 (1), 20–23. doi:10.1038/s42256-018-0004-1
Benrimoh, D., Israel, S., Fratila, R., Armstrong, C., Perlman, K., Rosenfeld, A., et al. (2021). Editorial: ML and AI safety, effectiveness and explainability in healthcare. Front. big Data 4, 727856. doi:10.3389/fdata.2021.727856
Bhattacharya, T., Brettin, T., Doroshow, J. H., Evrard, Y. A., Greenspan, E. J., Gryshuk, A. L., et al. (2019). AI meets exascale computing: Advancing cancer research with large-scale high performance computing. Front. Oncol. 9, 984. doi:10.3389/fonc.2019.00984
Buchsbaum, J. C., Jaffray, D. A., Ba, D., Borkon, L. L., Chalk, C., Chung, C., et al. (2022). Predictive radiation oncology–A new NCI–DOE scientific space and community.
Bukowski, R., Schulz, K., Gaither, K., Stephens, K. K., Semeraro, D., Drake, J., et al. (2021). Computational medicine, present and the future: Obstetrics and gynecology perspective. Am. J. Obstetrics Gynecol. 224 (1), 16–34. doi:10.1016/j.ajog.2020.08.057
Darcy, A. M., Louie, A. K., and Roberts, L. W. (2016). Machine learning and the profession of medicine. JAMA 315 (6), 551–552. doi:10.1001/jama.2015.18421
Ford Versypt, A. N. (2021). Multiscale modeling in disease. Curr. Opin. Syst. Biol. 27, 100340. Epub 2021 May 8. PMID: 35310906; PMCID: PMC8932948. doi:10.1016/j.coisb.2021.05.001
Hajat, C., and Stein, E. (2018). The global burden of multiple chronic conditions: A narrative review. Prev. Med. Rep. 12, 284–293. doi:10.1016/j.pmedr.2018.10.008
Hassani, H., Huang, X., and MacFeely, S. (2022). Impactful digital twin in the healthcare revolution. Big Data Cognitive Comput. 6 (3), 83. doi:10.3390/bdcc6030083
Hernandez-Boussard, T., Macklin, P., Greenspan, E. J., Gryshuk, A. L., Stahlberg, E., Syeda-Mahmood, T., et al. (2021). Digital twins for predictive oncology will be a paradigm shift for precision cancer care. Nat. Med. 27 (12), 2065–2066. doi:10.1038/s41591-021-01558-5
Hiram Guzzi, P., Petrizzelli, F., and Mazza, T. (2022). Disease spreading modeling and analysis: A survey. Briefings Bioinforma. 23 (4), bbac230. doi:10.1093/bib/bbac230
Hunter, D. J., and Reddy, K. S. (2013). Noncommunicable diseases. N. Engl. J. Med. 369, 1336–1343. doi:10.1056/nejmra1109345
Huys, Q. J. M., Maia, T. V., and Frank, M. J. (2016). Computational psychiatry as a bridge from neuroscience to clinical applications. Nat. Neurosci. 19 (3), 404–413. doi:10.1038/nn.4238
Kostkova, P., Brewer, H., De Lusignan, S., Fottrell, E., Goldacre, B., Hart, G., et al. (2016). Who owns the data? Open data for healthcare. Front. public health 4, 7. doi:10.3389/fpubh.2016.00007
LeCun, Y., Bengio, Y., and Hinton, G. (2015). Deep learning. Nature 521, 436–444. doi:10.1038/nature14539
Li, T., Sahu, A. K., Talwalkar, A., and Smith, V. (2020). “Federated learning: Challenges, methods, and future directions,” in IEEE signal processing magazine (IEEE), 37, 50–60.
Liu, Z., Roberts, R. A., Lal-Nag, M., Chen, X., Huang, R., and Tong, W. (2021). AI-based language models powering drug discovery and development. Drug Discov. Today 26 (11), 2593–2607. doi:10.1016/j.drudis.2021.06.009
Louis, D. N., Gerber, G. K., Baron, J. M., Bry, L., Dighe, A. S., Getz, G., et al. (2014). Computational pathology: An emerging definition. Archives pathology laboratory Med. 138 (9), 1133–1138. doi:10.5858/arpa.2014-0034-ed
Mulder, S., Hamidi, H., Kretzler, M., and Ju, W. (2018). An integrative systems biology approach for precision medicine in diabetic kidney disease. Diabetes, Obes. Metabolism 20, 6–13. doi:10.1111/dom.13416
Mulder, S. T., Omidvari, A. H., Rueten-Budde, A. J., Huang, P. H., Kim, K. H., Bais, B., et al. (2022). Dynamic digital twin: Diagnosis, treatment, prediction, and prevention of disease during the life course. J. Med. Internet Res. 24 (9), e35675. doi:10.2196/35675
Nordling, L. (2019). A fairer way forward for AI in health care. Nature 573 (7775), S103–S105. doi:10.1038/d41586-019-02872-2
Okegbile, S. D., Cai, J., Yi, C., and Niyato, D. (2022). Human digital twin for personalized healthcare: Vision, architecture and future directions. IEEE Network.
Paul, D., Sanap, G., Shenoy, S., Kalyane, D., Kalia, K., and Tekade, R. K. (2021). Artificial intelligence in drug discovery and development. Drug Discov. today 26 (1), 80–93. doi:10.1016/j.drudis.2020.10.010
Ramanathan, A., Ma, H., Parvatikar, A., and Chennubhotla, S. C. (2021). Artificial intelligence techniques for integrative structural biology of intrinsically disordered proteins. Curr. Opin. Struct. Biol. 66, 216–224. doi:10.1016/j.sbi.2020.12.001
Renardy, M., Hult, C., Evans, S., Linderman, J. J., and Kirschner, D. E. (2019). Global sensitivity analysis of biological multi-scale models. Curr. Opin. Biomed. Eng. 11, 109–116. doi:10.1016/j.cobme.2019.09.012
Rieke, N., Hancox, J., Li, W., Milletari, F., Roth, H. R., Albarqouni, S., et al. (2020). The future of digital health with federated learning. NPJ Digit. Med. 3 (1), 119–127. doi:10.1038/s41746-020-00323-1
Romero, R., Ramanathan, A., Yuen, T., Bhowmik, D., Mathew, M., Munshi, L. B., et al. (2019). Mechanism of glucocerebrosidase activation and dysfunction in Gaucher disease unraveled by molecular dynamics and deep learning. Proc. Natl. Acad. Sci. 116 (11), 5086–5095. doi:10.1073/pnas.1818411116
Stephan, K. E., Iglesias, S., Heinzle, J., and Diaconescu, A. O. (2015). Translational perspectives for computational neuroimaging. Neuron 87 (4), 716–732. doi:10.1016/j.neuron.2015.07.008
Sun, C., Shrivastava, A., Singh, S., and Gupta, A. (2017). “Revisiting unreasonable effectiveness of data in deep learning era,” in Proceedings of the IEEE international conference on computer vision (IEEE), 843–852.
Tiwary, B. K. (2020). Computational medicine: Quantitative modeling of complex diseases. Briefings Bioinforma. 21 (2), 429–440. doi:10.1093/bib/bbz005
Tolk, A., Barry, P., and Doskey, S. C. (2022). Using modeling and simulation and artificial intelligence to improve complex adaptive systems engineering. Int. J. Model. Simul. Sci. Comput. 13 (02), 2241004. doi:10.1142/s1793962322410045
Toma, M., Singh-Gryzbon, S., Frankini, E., Wei, Z. A., and Yoganathan, A. P. (2022). Clinical impact of computational heart valve models. Materials 15 (9), 3302. doi:10.3390/ma15093302
US Government Spending (2017) US government spending. Available at: http://www.usgovernmentspending.com/year_spending_2017USbn_18bs2n#.
Vaswani, A., Shazeer, N., Parmar, N., Uszkoreit, J., Jones, L., Gomez, A. N., et al. (2017). “Attention is all you need,” in Advances in neural information processing systems, 5998–6008.
Wang, F., Casalino, L. P., and Khullar, D. (2019). Deep learning in medicine—Promise, progress, and challenges. JAMA Intern. Med. 179, 293–294. doi:10.1001/jamainternmed.2018.7117
Wei, J., Tay, Y., Bommasani, R., Raffel, C., Zoph, B., Borgeaud, S., et al. (2022). Emergent abilities of large language models. arXiv preprint arXiv:2206.
Winslow, R. L., Trayanova, N., Geman, D., and Miller, M. I. (2012). Computational medicine: Translating models to clinical care. Sci. Transl. Med. 4 (158), 158rv11. doi:10.1126/scitranslmed.3003528
Yu, P., and Kibbe, W. (2021). Cancer data science and computational medicine. JCO Clin. Cancer Inf. 5, 487–489. doi:10.1200/cci.21.00006
Keywords: computational disease models, computational models of healthcare delivery, computational models of population health, data-driven personalized medicine, biomedical computational and mathematical modeling
Citation: Tourassi G (2023) Computational medicine: Grand challenges and opportunities for revolutionizing personalized healthcare. Front. Med. Eng. 1:1112763. doi: 10.3389/fmede.2022.1112763
Received: 30 November 2022; Accepted: 07 December 2022;
Published: 31 March 2023.
Edited and Reviewed by:
Dominique P. Pioletti, Swiss Federal Institute of Technology Lausanne, SwitzerlandCopyright © 2023 Tourassi. This is an open-access article distributed under the terms of the Creative Commons Attribution License (CC BY). The use, distribution or reproduction in other forums is permitted, provided the original author(s) and the copyright owner(s) are credited and that the original publication in this journal is cited, in accordance with accepted academic practice. No use, distribution or reproduction is permitted which does not comply with these terms.
*Correspondence: Georgia Tourassi, dG91cmFzc2lnQG9ybmwuZ292
Disclaimer: All claims expressed in this article are solely those of the authors and do not necessarily represent those of their affiliated organizations, or those of the publisher, the editors and the reviewers. Any product that may be evaluated in this article or claim that may be made by its manufacturer is not guaranteed or endorsed by the publisher.
Research integrity at Frontiers
Learn more about the work of our research integrity team to safeguard the quality of each article we publish.