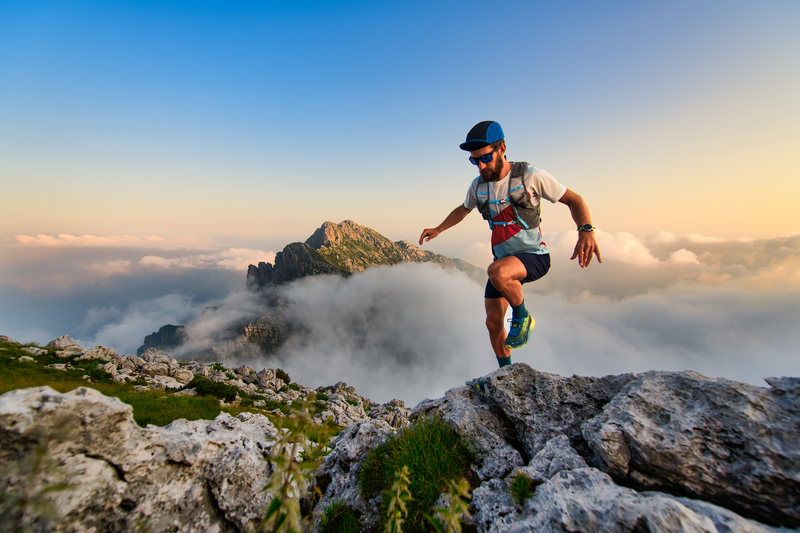
94% of researchers rate our articles as excellent or good
Learn more about the work of our research integrity team to safeguard the quality of each article we publish.
Find out more
ORIGINAL RESEARCH article
Front. Mech. Eng.
Sec. Vibration Systems
Volume 11 - 2025 | doi: 10.3389/fmech.2025.1553759
This article is part of the Research Topic Recent Advances in Mechanical Design and Vibration View all articles
The final, formatted version of the article will be published soon.
You have multiple emails registered with Frontiers:
Please enter your email address:
If you already have an account, please login
You don't have a Frontiers account ? You can register here
This article presents experimental results on the algebraic identification of the magnitude and phase angle of unbalance in a rotor-bearing system in a Jeffcott configuration. The algebraic identifier is designed based on a simplified mathematical model of the system and it uses only the measurement of the lateral vibration amplitude of the rotor. The proposed algebraic identifier is first validated by numerical simulation. For experimental implementation, a SpectraQuest Machinery Fault \& Rotor Dynamics Simulator is used. The designed identifier is evaluated in two scenarios. In the first, the rotor-bearing system is balanced using the traditional coefficients of influence method, after which a known unbalance is induced and compared with the identified magnitude and phase values. In the second case, the unbalance magnitude and phase values obtained by the algebraic identifier from an unknown original unbalanced configuration are used to balance the rotor-bearing system. The vibration amplitude reduction is quantified to evaluate the identified values. The main contribution of this work is the discussion of practical aspects that cannot be appreciated in simulation, but must be considered in the experimental implementation of the algebraic identification method, as they can limit the performance of the designed identifiers.
Keywords: Rotor-bearing system, experimental algebraic identification, Unbalance, Rotating machinery, Vibration
Received: 31 Dec 2024; Accepted: 18 Mar 2025.
Copyright: © 2025 Quiroz-Bautista, Arias-Montiel, Mendoza-Larios and Vázquez-Sánchez. This is an open-access article distributed under the terms of the Creative Commons Attribution License (CC BY). The use, distribution or reproduction in other forums is permitted, provided the original author(s) or licensor are credited and that the original publication in this journal is cited, in accordance with accepted academic practice. No use, distribution or reproduction is permitted which does not comply with these terms.
* Correspondence:
Manuel Arias-Montiel, Technological University of the Mixteca, Huajuapán de León, Mexico
Disclaimer: All claims expressed in this article are solely those of the authors and do not necessarily represent those of their affiliated organizations, or those of the publisher, the editors and the reviewers. Any product that may be evaluated in this article or claim that may be made by its manufacturer is not guaranteed or endorsed by the publisher.
Research integrity at Frontiers
Learn more about the work of our research integrity team to safeguard the quality of each article we publish.