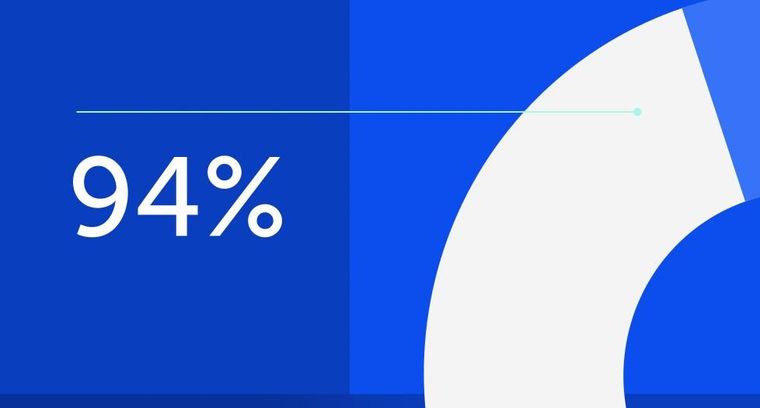
94% of researchers rate our articles as excellent or good
Learn more about the work of our research integrity team to safeguard the quality of each article we publish.
Find out more
ORIGINAL RESEARCH article
Front. Mech. Eng.
Sec. Heat Transfer Mechanisms and Applications
Volume 11 - 2025 | doi: 10.3389/fmech.2025.1547819
The final, formatted version of the article will be published soon.
Select one of your emails
You have multiple emails registered with Frontiers:
Notify me on publication
Please enter your email address:
If you already have an account, please login
You don't have a Frontiers account ? You can register here
This paper proposes an enhanced-search form of the newly designed artificial hummingbird algorithm (AHA), named oppositional chaotic artificial hummingbird algorithm. The proposed OCAHA methodology incorporates the oppositional learning (OBL) in the population-initialization and at the ending event of each iteration for a faster convergence, and the chaos-embedded sequences of Gauss/mouse map to replace the random sequences of the three population-updating iterative stages of AHA, viz. guided, territorial and migration foraging to employ more diverse population for more solutional accuracy. The effectiveness of the method has been evaluated in two phases. OCAHA, the four state of the art algorithms, namely PSO, DE, GWO and WOA, their recently developed effective variants, namely SLPSO, MTDE, SOGWO and EWOA, and the inspiring optimizer AHA have been implemented on the 29 unconstrained CEC 2017 benchmark functions in the first phase. In the second phase, OCAHA has been verified on 10 challenging engineering cases, and compared with the concerned leading performances. Comprehensive analysis of the simulated outcomes using various statistical metrics and of the convergence profiles demonstrates that, the optimization ability of OCAHA on CEC 2017 is superior to all the comparing algorithms except MTDE. For engineering cases, OCAHA provides better searching performance, solution precision, robustness and convergence rate than all competing designs, and, on average, it has lowered the computational cost by 57.5% & 22.63% in term of function evaluations and the fitness objective by 2.4% & 0.23% in comparison to AHA & the chaotic version CAHA,respectively.
Keywords: optimization, Metaheuristic algorithms, Artificial Hummingbird Algorithm, Opposition-based learning rule, Chaotic maps, Engineering design optimization problem
Received: 18 Dec 2024; Accepted: 10 Mar 2025.
Copyright: © 2025 Bhattacharjee, Roy, Tejani and Mousavirad. This is an open-access article distributed under the terms of the Creative Commons Attribution License (CC BY). The use, distribution or reproduction in other forums is permitted, provided the original author(s) or licensor are credited and that the original publication in this journal is cited, in accordance with accepted academic practice. No use, distribution or reproduction is permitted which does not comply with these terms.
* Correspondence: Seyed Jalaleddin Mousavirad, Mid Sweden University, Sundsvall, 851 70, Västernorrland, Sweden
Disclaimer: All claims expressed in this article are solely those of the authors and do not necessarily represent those of their affiliated organizations, or those of the publisher, the editors and the reviewers. Any product that may be evaluated in this article or claim that may be made by its manufacturer is not guaranteed or endorsed by the publisher.
Supplementary Material
Research integrity at Frontiers
Learn more about the work of our research integrity team to safeguard the quality of each article we publish.