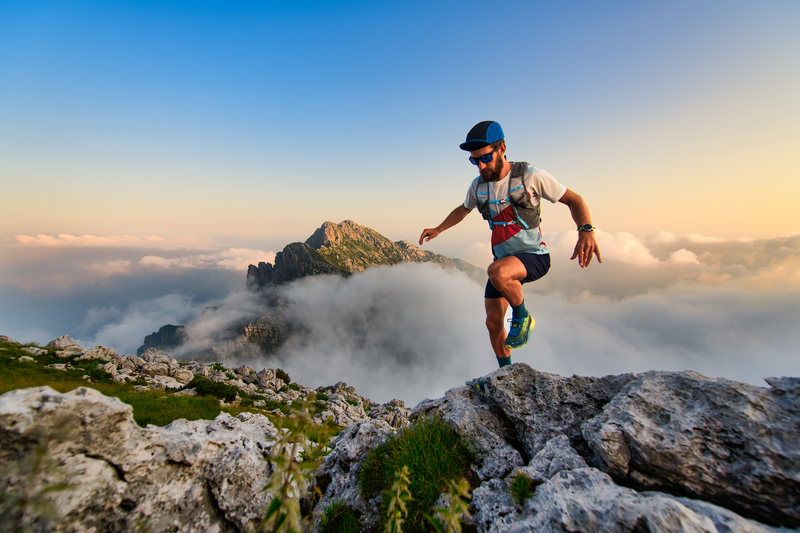
94% of researchers rate our articles as excellent or good
Learn more about the work of our research integrity team to safeguard the quality of each article we publish.
Find out more
ORIGINAL RESEARCH article
Front. Mech. Eng.
Sec. Engine and Automotive Engineering
Volume 11 - 2025 | doi: 10.3389/fmech.2025.1478081
This article is part of the Research Topic Exploring Opportunities and Challenges for Viable Ammonia Use in Transportation View all articles
The final, formatted version of the article will be published soon.
You have multiple emails registered with Frontiers:
Please enter your email address:
If you already have an account, please login
You don't have a Frontiers account ? You can register here
Ammonia (NH 3 ) stands out as a promising candidate for fueling internal combustion engines, owing to its high hydrogen content and well-established production and transport infrastructure.Nevertheless, its inherently low flame speed and reactivity pose a significant challenge to achieving rapid and complete combustion. One potential solution is the use of multi-spark ignition, wherein multiple spark plugs distribute ignition sites throughout the combustion chamber, thereby accelerating flame propagation across the entire charge. In this study, a three-dimensional 3D-CFD model of multi-spark, spark-ignited NH 3 internal combustion engine is developed and validated using optical engine experiments. The optical data provide critical insights into early flame kernel development, guiding refinements to two combustion submodels (SAGE and Gequation). Results underscore the importance of sufficiently refined mesh resolution-particularly near the spark plugs-and the incorporation of detailed spark plug geometries to accurately capture the early stages of ignition in low-reactivity fuels such as NH 3 . Overall, the close qualitative agreement between measured flame luminosity and simulated flame evolution demonstrates the robustness of the proposed CFD framework.
Keywords: Ammonia, Optical engine experiments, computational fluid dynamics, sage, G-equation
Received: 09 Aug 2024; Accepted: 04 Mar 2025.
Copyright: © 2025 Menaca, Silva, Uddeen, Almatrafi, Tang, Turner and Im. This is an open-access article distributed under the terms of the Creative Commons Attribution License (CC BY). The use, distribution or reproduction in other forums is permitted, provided the original author(s) or licensor are credited and that the original publication in this journal is cited, in accordance with accepted academic practice. No use, distribution or reproduction is permitted which does not comply with these terms.
* Correspondence:
Rafael Menaca, King Abdullah University of Science and Technology, Thuwal, Saudi Arabia
Disclaimer: All claims expressed in this article are solely those of the authors and do not necessarily represent those of their affiliated organizations, or those of the publisher, the editors and the reviewers. Any product that may be evaluated in this article or claim that may be made by its manufacturer is not guaranteed or endorsed by the publisher.
Research integrity at Frontiers
Learn more about the work of our research integrity team to safeguard the quality of each article we publish.