- 1Department of Mechanical & Manufacturing Engineering Technology, Vermont State University, Vermont, United States
- 2Department of Mechanical Engineering, College of Science and Technology, Royal University of Bhutan, Phuentsholing, Bhutan
- 3Department of Mechanical Engineering, School of Engineering and Technology, JECRC University, Jaipur, India
- 4University of Jeddah, Jeddah, Saudi Arabia
- 5Department of Orthodontics, Faculty of Dental Sciences, SGT University, Gurugram, India
- 6Department of Mechanical and Industrial Engineering, Manipal Institute of Technology, Manipal, India
- 7Department of Mechatronics, Manipal Institute of Technology, Manipal, India
The robotic arm is one of the vital components of robot assembly. The purpose of the robotic arm is to transmit power and conduct the desired motion, i.e., translation or rotation. Robotic limbs are designed and constructed to execute certain tasks with a high degree of speed, accuracy, and efficiency. This research focuses on to enhancing the strength-to-weight ratio of robotic arm using certain techniques of additive manufacturing, i.e., topology optimization and lattice structure. Employing the finite element analysis, the impact of weight reduction optimization on structural parameters such as stress and deformation in the current design is assessed using ANSYS R18.1 for FE analysis and Creo parametric 7.0 design software for computer-aided design modeling. Observations reveal that the 0.5 and .4 scale lattice structure designs have deformation of 0.01453mm and 0.01453 mm respectively though the generic design has 0.01043 mm deformation. Notably, the 0.5 scale lattice of the robotic arm exhibits a 31.08% higher equivalent stress than the generic design with 29.3%. reduction in mass of the robotic arm. These findings highlight the efficacy of lattice structures for optimizing the robotic arm’s performance, contributing to advancements in power-efficient robot assembly processes.
1 Introduction
Additive manufacturing (AM) encompasses a range of methodologies and technologies used for the fabrication of complex three-dimensional objects (Kumar et al., 2024). The procedure entails the incremental deposition of substances in successive strata, adhering to specified positions that correspond to digital cross-sections obtained from a computer-simulated model. The use of additive manufacturing has the capacity to fundamentally transform current production procedures, leading to a notable decrease in material wastage. Additive manufacturing offers many benefits compared to traditional production processes, including enhanced design flexibility and the capacity to produce personalized items. The procedure of three-dimensional (3D) printing, also known as additive manufacturing (AM), entails using a digital 3D model as a guide for the sequential printing of layers, resulting in the production of a desired object. The distinctive characteristic of this technology allows for the creation of complex shapes that were previously unachievable using traditional methods (Agarwal and Mthembu, 2021). Additive manufacturing (AM) is an advantageous asset that efficiently eradicates waste, streamlines production processes, and diminishes reliance on conventional manufacturing techniques. This technique facilitates the creation of personalized or complex models in a single operation. The increased “design freedom” may be largely ascribed to additive manufacturing (AM) (Kumar et al., 2023a). In the context of additive manufacturing, ‘design freedom’ signifies the capability to create intricate and customized shapes that were previously challenging or impossible to achieve using traditional manufacturing methods. Furthermore, there exists the potential to decrease the number of components, resulting in the elimination or substantial reduction of the need for assembly and the associated expenses (Maconachie et al., 2019). In addition, the use of on-demand manufacture for components has the capacity to optimize supply chains, reduce storage requirements, lower transportation costs, and accelerate the provision of essential replacement parts. Maconachie et al. (Maconachie et al., 2019) conducted research on the utilization of additive manufacturing (AM) techniques in production. Lattice structures have garnered significant study interest due to their ability to exhibit distinctive mechanical, electrical, thermal, and acoustic characteristics. The manipulation of various factors enables control over these qualities. The body of scholarly work pertaining to the mechanical properties of lattice structures produced using Selective Laser Melting (SLM) is growing steadily. Nevertheless, there is still a dearth of a complete study that integrates all the existing material. The objective of this work is to rectify the observed inadequacy by conducting a comprehensive examination of the experimental data pertaining to the mechanical characteristics of Selective Laser Melting (SLM) lattice structures. The objective of this study is to comprehensively examine the design, manufacturing, and performance elements pertaining to lattice structures produced by the process of Selective Laser Melting (SLM). Vaneker et al. (Vaneker et al., 2020) researched that the use of additive manufacturing (AM) has seen a notable transformation, transitioning from mostly fulfilling prototype requirements to emerging as a viable production technique in recent decades. The use of this technology is extensive across several industries, including the medical, aerospace, automotive, and other industrial domains. It is mostly used for the manufacturing of final components. The product has the potential to produce a diverse array of economically viable items, spanning from limited to extensive production runs, with a maximum output capability of 100,000 units. The production costs of metal additive manufacturing technologies are influenced by certain factors. These procedures are renowned for their somewhat sluggish pace in comparison to other production techniques. The effectiveness of the design framework will be shown via the provision of illustrative instances derived from both academic research and practical industrial applications. The objective of the study by Norfolk et al. (Hehr and Norfolk, 2019) is to do a comprehensive examination of the historical background, technical progress, domains of application, and research domains pertaining to the ultrasonic additive manufacturing (UAM) method. The UAM technique refers to a novel approach in 3D metal printing that involves the integration of ultrasonic energy with metal foils in order to establish metallurgical links between successive layers. This procedure takes place at or in close proximity to ambient temperature. The solid-state 3D metal printing technology does not involve the melting of materials. This study provides a comprehensive examination of the underlying principles of the physics involved in the process, various techniques used for characterization, the mechanical characteristics connected with the process, previous and ongoing research domains, constraints inherent to the process, and future applications. McDonough et al. (McDonough, 2020) The present research offers a comprehensive examination of the prevailing condition of additive manufacturing within the domain of process engineering. A complete study has been conducted by compiling results and views from different reports available in the public domain. The performed investigation has led to the creation of a novel perspective on the use of additive manufacturing in the domain of heat transfer. Moreover, the study undertaken has effectively highlighted prospective future prospects within this specific domain. Furthermore, this paper undertakes a critical analysis of many facets pertaining to heat transfer within the realm of additive manufacturing. The aim of this study is to demonstrate how additive manufacturing effectively tackles obstacles in the quest of attaining new levels of intensification. Numerous advances have been achieved. The reduction in the size of TORBED® technology facilitates the more convenient evaluation of adsorbents for the purpose of carbon capture. There have been suggestions put forward about novel heat pipe wick shapes with the objective of enhancing the thermal efficiency of heat pipes. Advancements in the creation of complicated reactor geometries within the field of flow chemistry have been seen, with the primary objective of enhancing the scalability of the process.
Singh et al. (Kaur and Singh, 2021) studied that the production methods for heat exchangers (HXs) have undergone significant changes as a result of advancements in additive manufacturing (AM). The use of additive manufacturing (AM) enables the realization of intricate and unconstrained designs that were previously unattainable using traditional manufacturing techniques, which were constrained by inherent limits. The study places importance on the surface quality, meaning that surfaces of heat exchanger components strive for higher smoothness so as to be most refined. Further, dimensional accuracy has been the major aspect for which various highlights have also emerged with improvements that lead to high adherence with respect of design specifications. Additionally, the study also recognizes successes in smaller geometric scales which highlights that heat exchanger technology can be utilized efficiently to make improved efficiency observations even for designs accommodating a minimal available surface area. These innovations altogether form the part of advancement in heat exchanger technology that would guarantee efficient systems and better serviceability standards across diverse arenas. According to Niknam et al. (Niknam et al., 2021) designers and researchers are increasingly using additive manufacturing (AM) technology to facilitate advancements in human-computer interface (HCI) design and development, with the aim of ushering in a new age in this field. The prevalence of diverse applications and the constraints associated with conventional production methods contribute to this phenomenon. Ladani et al. (Ladani and Sadeghilaridjani, 2021) offers a full examination of the research approach, including a detailed analysis of frequently used materials and their respective applications. This article presents a comprehensive examination of the microstructure and quality of components produced via the use of powder bed technology. This study primarily focuses on materials that are often manufactured using this particular process. The research critically analyzes the conclusions derived from extant literature pertaining to the influence of various process factors on the ultimate outcome of the product. The approach discussed above is often used to generate a wide variety of things. The primary objective of this research is to investigate and analyze the developing innovations and unique approaches used in the production process of highly conductive metals, namely, copper and aluminum. The intricate thermal history of components and alloys manufactured using Powder Bed Fusion Additive Manufacturing (PBF-AM), including targeted heat extraction, many melting cycles, and rapid solidification, poses challenges in the investigation of microstructure and characteristics. This discovery has been proven. The development of finer alloy grains is a common occurrence in additive manufacturing (AM) due to the higher solidification rates. In addition, it is worth noting that the mechanical characteristics of materials fabricated via additive manufacturing (AM) are equivalent to those attained by conventional manufacturing techniques. The creation of metallic products using additive manufacturing methods becomes much more arduous and expensive. The use of lasers or electron beams to selectively dissolve and fuse powder particles in a sequential manner, known as powder bed technology, has emerged as the predominant technique employed by several organizations operating in the aerospace and biomedical sectors at present. Due to its recent emergence and significant demand within the manufacturing sector, there are some unresolved scientific inquiries pertaining to this technology. The present publication offers an introductory overview of the methodology, along with illustrative instances of customary materials and practical applications. The aforementioned technique is often used in the manufacturing process to generate a diverse array of components. This paper examines the manufacturing processes and properties of highly conductive metals, including copper and aluminum. Additionally, it explores the possibilities for future advancements and developments in this sector. According to Galati et al. (Galati and Iuliano, 2018) Electron beam melting (EBM) is an additive manufacturing technique that use an electron beam to fuse metallic particles, resulting in the formation of the desired object’s geometry. Within these fields of study, the use of this technique is employed to manufacture intricate components made from high-quality materials, hence addressing the challenges associated with the production of such components utilizing other methodologies, which may be arduous or financially burdensome. The scientific community has been dedicating significant effort towards enhancing the reliability of the Electron Beam Melting (EBM) process, owing to the considerable interest shown by the industry in this technology. The modeling of the evidence-based medicine (EBM) process has significant relevance due to the potential time-consuming nature of refining the process via experiential learning, which currently represents the prevailing approach. A thorough examination is conducted on the many endeavors undertaken in the field of numerical modeling. The categorization of these research into their various groups is contingent upon the amount of approximation used in the modeling process. Initially, the simulations were categorized based on the particle modeling technique used, either the mesoscopic or finite element (FE) method. Subsequently, the study was bifurcated into two independent domains, namely, coupled modeling techniques and uncoupled modeling approaches in relation to finite element-based simulations. The evaluation of several approaches presently used was conducted, with particular emphasis placed on the researchers’ modeling of the evidence-based medicine (EBM) technique. Several factors need to be considered, including the assumptions made, the modeling of material properties, the change in material state, and the presence of a heat source. Kashani et al. (Ngo et al., 2018) Additive manufacturing, often referred to as 3D printing, offers many significant benefits, including enhanced design freedom, the potential for mass customization, reduced waste generation, the capacity to fabricate complicated structures, and expedited prototype capabilities. A comprehensive investigation was conducted on the primary methodologies of 3D printing, the many types of materials used, and the notable developments seen in commonly employed applications. Significant attention was directed to the novel uses of additive manufacturing (AM) in the biomedical, aerospace, construction, and protective structures sectors. The talk included a comprehensive discussion on the current state of materials development, focusing on concrete, ceramics, polymer composites, and metal alloys. Furthermore, this study aimed to investigate the underlying processing difficulties related to anisotropic behavior, the generation of vacancies, the limitations imposed by computer architecture, and the manifestation of layers. In summary, the objective of this study was to provide a comprehensive examination of three-dimensional printing and establish a foundation for forthcoming research and advancement via the implementation of a survey assessing the merits and drawbacks of this technology.
Moon et al. (Moon et al., 2021) used a genetic algorithm to conduct shape optimization for the purpose of designing a new heat exchanger (HX) that could be manufactured using additive manufacturing (AM) methods. The primary goal of the evolutionary algorithm was to optimize the thermal efficiency of the system by maximizing the overall surface area while ensuring compliance with the specified design restrictions. The empirical inquiry demonstrated a noteworthy enhancement in the overall efficacy of the recently improved HX generated using additive manufacturing. This finding was established by doing a comparative study, whereby a common commercial tube-in-tube gadget was used as a reference point.
Based on an extensive analysis of thermal-hydraulic properties, the HX exhibited a significant specific power of 15.7 kW/kg and a power density of 26.6 W/cm3, indicating exceptionally high levels. The device we have developed, utilizing the additive manufacturing technique, demonstrates a significant twenty-fold enhancement in specific power when compared to a conventional tube-in-tube heat exchanger lacking fins that are currently available in the market. Kumar et al. (Kumar et al., 2023b) The selection of appropriate materials for intended constructions has significant significance within the realm of additive manufacturing (AM), emphasizing the paramount relevance of materials in this discipline. To attain success, materials must meet three fundamental characteristics. Kumaran et al. (Kumaran et al., 2023) suggested a structure to improve the design of an AI-based robotic arm. This framework is designed to predict a lightweight structure for the robotic arm and to study the deformation of the metal alloys which are created by the powder-based AM. The study uses topology optimization and generative design concepts in the alternative design process. Through the use of these methods, the framework reduces the weight of the robotic arm and at the same time, the same positioning accuracy is maintained. The results show that the inert payload is decreased by 10%–50% through the design optimization. Kumar et al. (Kumar et al., 2023c) have researched various types of additive manufacturing processes. The additive manufacturing process involves various steps. The execution of these steps is necessary for the completion of the system. Table 1 gives an overview of the literature review summary.
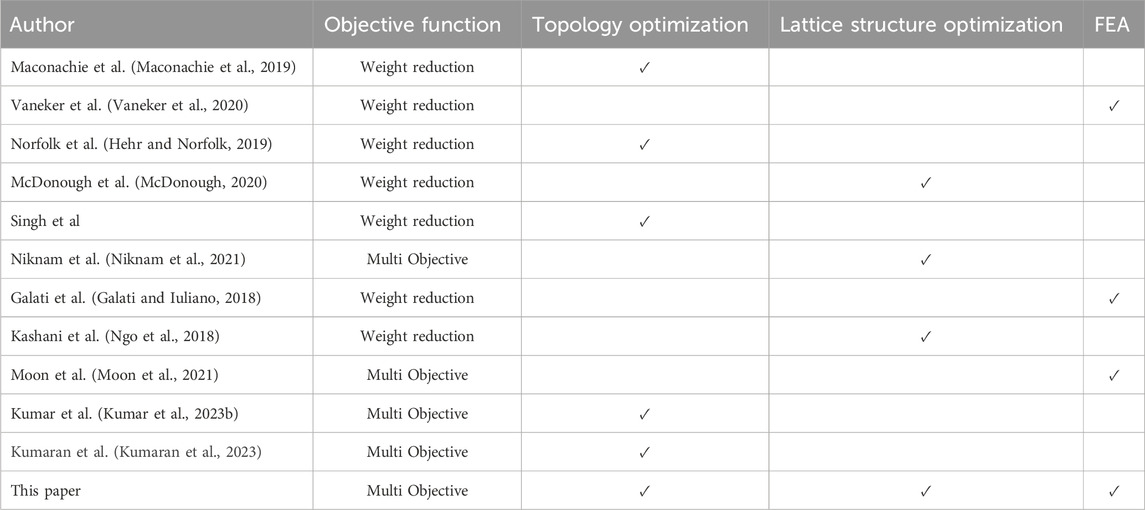
Table 1. These research works have contributed to the Structural Efficiency of Robotic Arms through Topological and Lattice-Based AM Optimization.
The novelty of the study is stressed in Table 1, where it is shown that the study has key differences from the study of the previous literature. In contrast to the research before which was concerned with motion and kinematic studies of robotic arms, our study is different in the fact that we are studying the weight reduction using additive manufacturing techniques, topology optimization, and lattice structures. The particular approach is the main reason for the optimized robotic arm design, which in turn, is being sought. The robotic arm is a dynamic component of robot assembly. The purpose of the robotic arm is to transmit power and conduct the desired motion, i.e., translation or rotation. Most of the existing research on robotic arms is based on motion studies and kinematic studies. However, none of the research is based on the weight reduction of robotic arm using certain techniques of additive manufacturing, i.e., topology optimization and lattice structure (Kareemullah et al., 2023; Peng et al., 2023; Hernandez-Sanchez et al., 2023). Topology optimization is a computational technique to identify the best arrangement of material throughout in given domain, so as to achieve optimum function. According to the analysis of robot arms, this approach is obligatory for performance efficiency resulting in different level component’s structure optimization, reductions in weight, and overall improvement strength. The use of topological optimization allows for reaching the best compromise between strength and material resources which is reflected in higher energy efficiency, and manoeuvrability for robotic arms (Letsatsi et al., 2021). Therefore, this study aims to optimize the current design of the robotic arm by implementing topological and lattice structures. The Finite Element Method is employed to evaluate the impact of weight reduction optimization on structural parameters such as stress and deformation in the current design. Finite element (FE) analysis is conducted using ANSYS software, while computer-aided design (CAD) modeling is carried out using Creo design software.
The study is organized into four sections. Section 1 provides the background. Outlines the approach that will be taken. Section 2 explains the methodology, in detail. Presents practical demonstrations and analyses to show how our approach can be applied. In Section 3 we carefully analyze the results. Finally, in Section 4, we discuss the accomplishments and importance of this paper emphasizing its contributions and potential impact.
2 Methodology
The methodology of analysis involves different stages, i.e., modeling, meshing, and loading conditions. Initially, advanced CAD tools like Creo are employed to accurately define the arm’s physical features. Following this, in the meshing stage, the model is broken down into smaller elements for efficient computation while preserving accuracy. Consequently, loading conditions are applied to simulate various scenarios, enabling an assessment of the robotic arm’s performance. Figure 1 below illustrates the model of the robotic arm, which was created via the use of several Creo design software tools. The use of Creo design software in the methodology is crucial for developing the CAD model of the robotic arm. The parametric modeling developed by Creo enables accurate design, quick prototyping, and variation study through streamlined processes (Agarwal and Mthembu, 2022). The simulation capabilities of the software ensure proper alignment between structural integrity and engineering specifications, while compatibility with various manufacturing processes facilitates a smooth path from virtual model to physical prototype; as such, it is an essential tool for developing complex designs of robotic arm that are optimized. The parts of the robotic arm were established by a comprehensive analysis of existing research (Eranki and Reddy Gurudu, 2017).
The reasons for choosing 0.4 and 0.5 scale lattice structures in our study are dictated by a strategic rationale that aligns with the research goals. The 0.4 scale lattice enables a better understanding of micro-structural structures through deeper observation of the intricacies thus enabling examination into details that might affect general performance within an individual robotic arm. In contrast, the 0.5 scale provides a compromise between detail and computational efficiency in finding an optimal procedure for structural analysis that preserves distinctive features. The CAD model is generated by the use of an extrusion method in conjunction with a circular tool. The model encompasses around eight distinct features as shown in Figures 2, 3.
The ANSYS Design Modeler is a software application used for the assessment of sharp boundaries, geometric deficiencies, and data degradation. The functionality is seen in Figures 4–6 respectively.
The use of tetrahedral elements provides a feasible alternative for generating a mesh structure for the model of the robotic arm (Letsatsi et al., 2021). The complexity of the element is increased as a result of the existence of a lattice structure inside the arm and the occurrence of abrupt fluctuations in geometry (Molwane et al., 2020). The tetrahedral element is preferred for certain reasons, such as geometric simplicity, computational accuracy, and its proficiency in capturing complex geometries and irregular shapes, making it a well-suited choice for accurately representing the intricate details and structural nuances of the robotic arm in our analysis.
The model that was developed has a total of 7,613 elements as shown in Figure 7. The phenomenon of meshing is often seen in many robotic arm configurations, especially those that integrate a lattice framework characterized by either a 0.5 or 0.4 scale density. The region exhibiting a lattice structure has a higher density of elements in comparison to the adjacent sections. Both Figure 8 and Figure 9 provide the lattice structure mesh model, each illustrating it in a distinct manner while Figure 10 depicts the load and boundary conditions.
The hand computations are used in the second phase of the methodology using customized geometric modifications. In particular, the structure is considered to be an ideal solid linear beam that possesses a uniform cross-section and, in this way, it does not include gaps or holes. In an effort to improve computational efficiency, the curving cross-section is assumed as a straight line. This streamlined approach allows for targeted analysis of particular fields that are of interest, delivering precise analytical results. Treating the geometry as a solid linear beam in the loads and boundary conditions section simplifies the analysis, providing computational efficiency without compromising essential structural characteristics.
Volume V = 0.00359,787 m cubic, Density equals 2,700 kg/m3.
9.714 kg is mass M = volume * density.
Mass *g = weight = 95.29N (Eranki and Reddy Gurudu, 2017)
Using the computations from before, a precise load of 95.29N has been delivered to the robotic arm’s cylindrical end. Additionally, a fixed support configuration is used to secure the robotic arm’s left support, providing stability and well-defined boundary conditions for our research. It is noteworthy to emphasize that aluminum 5,083, a material well-known for its mechanical qualities and appropriateness for structural evaluations, was used for this investigation. The aluminum series of 5,083 elements was specifically chosen for the robotic arm analysis, due to its outstanding corrosion resistiveness resulting from a ratio in magnesium content. Although non-heat treatable, the alloy has outstanding mechanical strength that guarantees structural integrity and load-bearing capability. This makes its production faster and increases overall structural integrity. Aluminum 5,083 with its low density, provides a light structure that minimizes energy and agility. The alloy has formability which makes it possible for easy moulding to suit specific designs and thermal stability that ensures its performance remains stable in a variety of settings.
3 Results and discussion
The primary objective of doing a finite-element analysis (FEA) is to assess the structural characteristics of a robotic arm, with particular attention given to stress and deformation. This critical analysis provides the opportunity for us to understand how this robotic arm reacts when forces are externally applied. The controlled loading conditions are analyzed, and the usefulness of stress and deformations assessment in understanding arises from layering robotic arm integrity. Topological and lattice structures are applied in this study to improve the functioning of robotic arms. Topology optimization enables the discovery of material distribution for the enhancement of the strength-to-weight ratios. Lattice structures are structurally efficient and yet lightweight design options. The combination of both methods helps to achieve the optimal design strategy to deal with major design issues in robotic arm development. Visual representation of compression-induced deformation on cylindrical supports as shown in Figures 2, 11 serves to guide physical behaviour for structural response under applied loads, thereby reinforcing the need for analysis stresses and displacement with the computation process. The observation of red colored in these images indicates the occurrence of substantial load-induced deformation on the cylindrical supports during the application of the load.
The maximum deformation obtained on the original design of the robotic arm is 0.01034 mm at the zone of applied load which is in close agreement with results in the literature (Eranki and Reddy Gurudu, 2017). The deformation at the other end is 0.0075 mm. A crucial component of the study, the GIT (grid independence test) findings, are rigorously reported in Table 2 and offer critical insight underlying the stability and dependability of the computing process.
Figure 12 shows the deformation plot of 0.5 scale lattice design. The maximal equivalent stress area is influenced by the variability in the exact design of the lattice structure used as shown in Figure 13. The maximum equivalent stress is obtained on the lateral surface of a structure with a .5 lattice scale lattice while Figure 14 shows the deformation plot of the 0.4 scale lattice design.
For 0.4 scale lattice structure design, the maximum equivalent stress is obtained at the lattice zone (initial 2 beam lattices) as depicted in Figure 15. The zone of load application has an almost uniform value of equivalent stress with a magnitude of 0.179 MPa.
Figure 16 depicts the topological density plot for the robotic arm. Based on the available information, it is evident that the vacant area situated between the arms has the most potential for material reduction. The plot in Figure 17 clearly illustrates that the regions in close proximity to the central portion of the robotic arm exhibit decreased levels of stress.
The use of topological optimization techniques in the design of the robotic arm leads to a deformation of 0.01297 mm at the loaded extremity as shown in Figure 17. The level of distortion diminishes progressively when proximity to the support zone is attained, as seen by the above visual representation.
A thorough comparison of the data is provided in Table 3, which further clarifies the differences in stress distribution between the various robotic arm sections. Here, the term “generic design” refers to the initial, non-optimized design of the robotic arm without any specific modifications or enhancements. These results are then further discussed in Figures 18 through 20, each of which offers comprehensive insights into the particular stress patterns and changes within the structure of the arm.
Figure 19 illustrates the equivalent stress plot, which reveals a significant disparity in magnitude between the solid mass and the supporting zone on the upper surface. The equivalent stress at the support region is 0.85665 MPa and the equivalent stress at the top face of arm is 0.286 MPa.
The graphical representation effectively demonstrates that the robotic arm’s topologically optimal construction exhibits the least amount of comparable stress. Conversely, it is noteworthy to observe that the lattice configuration at a size of 0.4scale demonstrates the farthest magnitude of comparable stress. Both designs are derived from the same design. The deformation plot exhibits a consistent pattern, as seen in Figure 20. The robotic arm, characterized by a lattice structure with a density of 0.5 exhibits the most substantial degree of deformation. Conversely, a design that adheres to topological optimization principles and conforms to a generic design demonstrates minimum deformation.
With regard to other designs with a 0.4 scale lattice structure, the topologically optimized model has the lowest mass, as demonstrated by the mass comparison in Figure 21. This thorough examination of the structural behavior of the robotic arm has provided insightful information about how well it functions mechanically in various configurations. Significant stress differences between the topologically optimized model and the 0.4 scale lattice structure, which are derived from the identical design, have been found numerically. The application of topological optimization has been crucial in mitigating deformation and stress, hence improving the structural integrity of the arm. Additionally, our study highlights how important lattice size is in determining stress distribution, with the 0.5 scale lattice structure showing higher stress levels.
4 Conclusion
ANSYS software is used to do the FEA study on the robotic arm, and topological and lattice structure methods are used to optimize the generic design. The practical significance of industrial robotic arm design is in the optimization of topology and lattice structure. The optimizations can result in improved performance metrics, for example, the stiffness-to-weight ratio and durability, thus, the development of lighter, more efficient robotic arms that consume less energy and need less maintenance. The optimized mass of the robotic arm is analyzed using the finite element method to determine stresses and deformation. The detailed conclusions are as follows:
1. The .5 scale lattice structure design has .01469 mm deformation and the generic design has .01043 mm deformation.
2. The .4 scale lattice structure design has .01453 mm deformation and the generic design has .01043 mm deformation.
3. The equivalent stress on the .5 scale lattice of the robotic arm is 31.08% higher than the generic design.
4. The equivalent stress on the .4 scale lattice of the robotic arm is 2.16 higher than the generic design.
5. The .5 scale lattice structure design has a 29.3% weight reduction as compared to the generic design.
6. The .4 scale lattice structure design has a 28.8% weight reduction as compared to the generic design.
This study brings attention on the benefit of a lattice architecture in pioneering ways that robotic arms can be designed. By conducting a thorough study, which includes aspects like amount (effective size), stress deformation as well as weight that can be reduced, our research presents useful data for optimizing the load capacity program of robotic arms. Nevertheless, it is important to note some limitations such as the DSI problems being set in idealized settings and assumptions concerning material properties. The continued need for real-world validation is essential. Future studies should concentrate on studying a wide range of lattice networks and testing our results through practical experiments. These innovations may lead to the development of adaptive robotic arms with many benefits in sectors like automotive and aerospace. The fact is that the result of elevational and weight variance will be manufacturing a light-weight robotic arm, eventually decreasing the electrical energy needed for its rotation. The second step forward in this domain can utilize different optimization methods such as response surface method and genetic algorithms to improve the productivity of robot arms.
Data availability statement
The original contributions presented in the study are included in the article/Supplementary material, further inquiries can be directed to the corresponding authors.
Author contributions
RB: Conceptualization, Writing–original draft. AA: Conceptualization, Methodology, Writing–review and editing. AG: Conceptualization, Investigation, Writing–review and editing. AK: Conceptualization, Methodology, Supervision, Writing–review and editing. FA: Methodology, Writing–review and editing. ND: Formal Analysis, Methodology, Writing–review and editing. VHM: Methodology, Supervision, Writing–review and editing. DC: Investigation, Methodology, Writing–review and editing. AA: Investigation, Software, Writing–review and editing.
Funding
The author(s) declare that no financial support was received for the research, authorship, and/or publication of this article.
Conflict of interest
The authors declare that the research was conducted in the absence of any commercial or financial relationships that could be construed as a potential conflict of interest.
Publisher’s note
All claims expressed in this article are solely those of the authors and do not necessarily represent those of their affiliated organizations, or those of the publisher, the editors and the reviewers. Any product that may be evaluated in this article, or claim that may be made by its manufacturer, is not guaranteed or endorsed by the publisher.
References
Agarwal, A., and Mthembu, L. (2022). Structural analysis and optimization of heavy vehicle chassis using aluminium P100/6061 Al and Al ga 7-230 mmc. Processes 10 (2), 320. doi:10.3390/pr10020320
Agarwal, A., and Mthembu, L., “Numerical modelling and multi objective optimization analysis of heavy vehicle chassis,” Processes, vol. 9, no. 11, p. 2028, 2021, doi:10.3390/pr9112028
Eranki, V. K. P., and Reddy Gurudu, R. (2017). Design and structural analysis of a robotic arm. Blekinge Institute of Technology, Department of Mechanical Engineering. Available at: https://www.diva-portal.org/smash/get/diva2:1068547/FULLTEXT02 (Accessed: July 26, 2023).
Galati, M., and Iuliano, L. (2018). A literature review of powder-based electron beam melting focusing on numerical simulations. Addit. Manuf. 19, 1–20. doi:10.1016/j.addma.2017.11.001
Hehr, A., and Norfolk, M. (2019). A comprehensive review of ultrasonic additive manufacturing. Rapid Prototyp. J. 26 (3), 445–458. doi:10.1108/RPJ-03-2019-0056
Hernandez-Sanchez, A., Chairez, I., Matehuala-Moran, I., Alfaro-Ponce, M., and Molina, A. (2023). Trajectory tracking controller of a robotized arm with joint constraints, a direct adaptive gain with state limitations approach. ISA Trans. 141, 276–287. doi:10.1016/j.isatra.2023.07.004
Kareemullah, H., Najumnissa, D., Shajahan, M. S. M., Abhineshjayram, M., Mohan, V., and Sheerin, S. A. (2023). Robotic Arm controlled using IoT application. Comput. Electr. Eng. 105, 108539. doi:10.1016/j.compeleceng.2022.108539
Kaur, I., and Singh, P. (2021). State-of-the-art in heat exchanger additive manufacturing. Int. J. Heat. Mass Transf. 178, 121600. doi:10.1016/j.ijheatmasstransfer.2021.121600
Kumar, A., Kumar, P., Mittal, R. K., and Gambhir, V. (2023b). “Materials processed by additive manufacturing techniques,” in Advances in additive manufacturing artificial intelligence, nature-inspired, and biomanufacturing (Elsevier), 217–233. doi:10.1016/B978-0-323-91834-3.00014-4
Kumar, A., Kumar, P., Mittal, R. K., and Singh, H. (2023c). “Preprocessing and postprocessing in additive manufacturing,” in Advances in additive manufacturing artificial intelligence, nature-inspired, and biomanufacturing (Elsevier), 141–165. doi:10.1016/B978-0-323-91834-3.00005-3
Kumar, A., Kumar, P., Sharma, N., and Srivastava, A. K. (2024). 3D printing technologies: digital manufacturing, artificial intelligence, industry 4.0.
Kumar, A., Mittal, R. K., and Haleem, A. (2023a). Advances in additive manufacturing artificial intelligence, nature-inspired, and biomanufacturing (Elsevier).
Kumaran, M., Senthilkumar, V., and Sathies, T. (2023). “Design and optimization of artificial intelligence robot arm printable by a metal-based additive manufacturing process,” in Advances in additive manufacturing artificial intelligence, nature-inspired, and biomanufacturing (Elsevier), 449–461. doi:10.1016/B978-0-323-91834-3.00025-9
Ladani, L., and Sadeghilaridjani, M. (2021). Review of powder bed fusion additive manufacturing for metals. Met. (Basel) 11 (9), 1391. doi:10.3390/met11091391
Letsatsi, M. T., Agarwal, A., and Pitso, I. (2021). The finite element evaluation of support bracket using the application of topological optimization. J. Eng. Res., 1–13. doi:10.36909/jer.ICIPPSD.15505
Maconachie, T., Leary, M., Lozanovski, B., Zhang, X., Qian, M., Faruque, O., et al. (2019). SLM lattice structures: properties, performance, applications and challenges. Mater Des. 183, 108137. doi:10.1016/j.matdes.2019.108137
McDonough, J. R. (2020). A perspective on the current and future roles of additive manufacturing in process engineering, with an emphasis on heat transfer. Therm. Sci. Eng. Prog. 19, 100594. doi:10.1016/j.tsep.2020.100594
Molwane, O. B., Agarwal, A., and Marumo, R. (2020). “Industrial computational analysis of aerodynamic characteristics of delta-shaped aircraft,” in Advances8 in lightweight materials and structures. Editors A. Praveen Kumar, T. Dirgantara, and P. V. Krishna (Singapore: Springer), 8, 761–770. doi:10.1007/978-981-15-7827-4_77
Moon, H., McGregor, D. J., Miljkovic, N., and King, W. P. (2021). Ultra-power-dense heat exchanger development through genetic algorithm design and additive manufacturing. Joule 5 (11), 3045–3056. doi:10.1016/j.joule.2021.08.004
Ngo, T. D., Kashani, A., Imbalzano, G., Nguyen, K. T. Q., and Hui, D. (2018). Additive manufacturing (3D printing): a review of materials, methods, applications and challenges. Compos B Eng. 143, 172–196. doi:10.1016/j.compositesb.2018.02.012
Niknam, S. A., Mortazavi, M., and Li, D. (2021). Additively manufactured heat exchangers: a review on opportunities and challenges. Int. J. Adv. Manuf. Technol. 112 (3–4), 601–618. doi:10.1007/s00170-020-06372-w
Peng, J., Wu, H., Zhang, C., Chen, Q., Meng, D., and Wang, X. (2023). Modeling, cooperative planning and compliant control of multi-arm space continuous robot for target manipulation. Appl. Math. Model. 121, 690–713. doi:10.1016/j.apm.2023.05.019
Keywords: lattice structures, finite element analysis, structural optimization, ANSYS, additive manufacturing, topological optimization, robotic arm
Citation: Batista RC, Agarwal A, Gurung A, Kumar A, Altarazi F, Dogra N, H. M. V, Chiniwar DS and Agrawal A (2024) Topological and lattice-based AM optimization for improving the structural efficiency of robotic arms. Front. Mech. Eng 10:1422539. doi: 10.3389/fmech.2024.1422539
Received: 24 April 2024; Accepted: 21 May 2024;
Published: 06 June 2024.
Edited by:
Kanak Kalita, Vel Tech Dr. RR & Dr. SR Technical University, IndiaReviewed by:
Neeraj Sharma, National Institute of Technology Agartala, IndiaElangovan Muniyandy, Saveetha Medical College and Hospital, India
Gaydaa AlZohbi, Prince Mohammad bin Fahd University, Saudi Arabia
Copyright © 2024 Batista, Agarwal, Gurung, Kumar, Altarazi, Dogra, H. M., Chiniwar and Agrawal. This is an open-access article distributed under the terms of the Creative Commons Attribution License (CC BY). The use, distribution or reproduction in other forums is permitted, provided the original author(s) and the copyright owner(s) are credited and that the original publication in this journal is cited, in accordance with accepted academic practice. No use, distribution or reproduction is permitted which does not comply with these terms.
*Correspondence: Ajay Kumar, ajay.kumar30886@gmail.com; Vishwanatha H. M., vishwanatha.hm@manipal.edu; Dundesh S. Chiniwar, dundesh.sc@manipal.edu; Ashish Agrawal, ashish.agrawal@manipal.edu