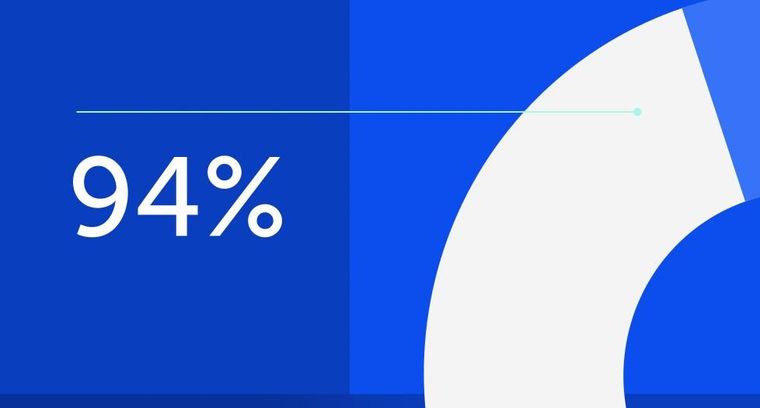
94% of researchers rate our articles as excellent or good
Learn more about the work of our research integrity team to safeguard the quality of each article we publish.
Find out more
REVIEW article
Front. Mech. Eng., 02 November 2023
Sec. Turbomachinery
Volume 9 - 2023 | https://doi.org/10.3389/fmech.2023.1261017
This article is part of the Research TopicExperimental Characterization of Wind Turbine Performance and Wind Farm Flow: Challenges and OpportunitiesView all 3 articles
This article provides a comprehensive review of the most recent advances in the planning, execution, and analysis of inflow and wake measurements from nacelle-mounted wind Doppler lidars. Lidars installed on top of wind turbines provide a holistic view of the inflow and wake characteristics required to characterize and optimize wind turbine performance, carry out model validation and calibration, and aid in real-time control. The need to balance the enhanced capabilities and limitations of lidars compared to traditional anemometers inspired a broad variety of approaches for scan design and wind reconstruction, which we discuss in this review. We give particular emphasis to identifying common guidelines and gaps in the available literature with the aim of providing an exhaustive picture of the state-of-the-art techniques for reconstructing wind plant flow using nacelle-mounted lidars.
Global electricity production from wind energy increased by 17% in 2021 compared to the previous year, which represents the largest growth in power production compared to all forms of power generation in 2021 (IEA, 2021). However, in order to meet the ambitious goal of Net Zero Emissions by 2050 (Forster et al., 2020), power generation from wind energy should grow annually on an average by 18% (Pryor et al., 2020). For the United States, some wind energy-focused studies (Marvel et al., 2013; Pryor et al., 2020) have shown that bringing the wind energy production from the current 9%–20% of the overall energy portfolio by 2030 is within the theoretical and current technological limits. In this regard, Veers et al. (2019) identified grand challenges in our ability to accurately characterize wind plant aerodynamics and the wind resource. The complex interaction between the synoptic and mesoscale processes driving the atmospheric boundary layer, coupled with perturbations introduced by local orography (or the ocean) produces spatio-temporal variations in the wind resource that are not fully predictable. Furthermore, the turbines themselves significantly modify the surrounding wind velocity field. The kinetic energy extracted by wind turbines creates wakes, which are also known to impact the local microclimate Barthelmie et al. (2010); El-Asha et al. (2017); Abkar and Porté-Agel (2015); Zhan et al. (2019); Bodini et al. (2021); Wu and Archer (2021).
Better characterization of the wind field would lead to a reduction of the levelized cost of energy through several mechanisms. First, by reducing the error in the predicted annual energy production (AEP) in the pre-construction phase, which is still affected by an average
Variability in the wind resource due to large turbulent eddies, thermal stratification, wind shear, ocean/wave dynamics, terrain, and wakes occur on spatial scales that are hardly detectable through traditional in situ measurements that collect high-frequency data at single points in space. This limitation spurred the interest in remote sensing technology, and in particular Doppler wind lidars, as an alternative and more effective way to characterize the atmospheric velocity field for wind energy applications (Liu et al., 2019). Modern commercial lidars enable measurement of high-resolution winds over large areas of interest, and are compact enough to be installed in locations that are otherwise difficult to access, such as sites with complex terrain, offshore platforms, and the nacelles of utility-scale wind turbines.
Several scanning technologies and strategies have been adopted to probe the atmospheric boundary layer for wind energy applications. Profiling lidars have been deployed for freestream resource assessment (Gottschall et al., 2012; Optis et al., 2021; Sharma et al., 2021), wake characterization (Heisel et al., 2018) and plant induction quantification (Jacquet et al., 2022; Letizia et al., 2022). Scanning lidars, i.e., those that feature the ability to direct the laser beam in arbitrary directions (among other abilties), offer additional flexibility and permit a more targeted characterization of the wind plant flow. These lidars have been extensively used, both on the ground and mounted on turbine nacelles, to characterize wakes in the horizontal (e.g., Trujillo et al., 2011; Kumer et al., 2015; Bodini et al., 2017; Zhan et al., 2019) and vertical plane (Iungo et al., 2013), as well as in a volumetric fashion (e.g., Iungo and Porté-Agel, 2014; Doubrawa et al., 2019; Letizia et al., 2021b).
Nacelle-mounted lidars (NMLs) provide unique capabilities for real-time characterization of the inflow and wakes of utility-scale wind turbines. The logistical complexity of deploying a lidar on a wind turbine nacelle is compensated by a better characterization of the wind resource at heights relevant to wind energy and by the effortless steering of the lidar onto the wind (and wake) direction for yaw-controlled turbines.
This article presents the state-of-the-art regarding the use of NMLs for wind energy research. The studies reviewed in this article utilize real or virtual NMLs spanning a broad range of applications, including.
• real-time measurements from forward-looking lidars injected into wind turbine or wind farm controllers enhanced with feed-forward control paths, aimed at adjusting the turbine or farm operation to adapt to incoming wind perturbations
• lidar measurements over long periods of time leveraged to assess wind turbine performance as an alternative to meteorological towers
• high-resolution lidar data assimilated into numerical simulations to assess dynamic performance and loads
• lidars probing the upstream region of wind turbines utilized to characterize the inflow for wake-focused studies
• rear-looking lidars used to characterize the statistics of the turbulent wake velocity field for model calibration and validation
• high-frequency lidar measurements producing time series of instantaneous wake characteristics used to investigate wake dynamics
• lidar simulators (i.e., virtual lidars) developed to 1) assess the error in measurements due to either scanning geometry or lidar acquisition during various atmospheric conditions and 2) optimize scanning strategies.
In spite of the significant potential of NMLs, multiple experts (Clifton et al., 2018; Simley et al., 2018) have identified several barriers that still hinder the assimilation of such technology into wind energy industrial standards. First and foremost, a consolidated scan design strategy and data processing technique have not yet emerged, partially due to the uniqueness of the individual experiments and the relative novelty of the lidar technology. The purpose of this review is to provide a detailed account of applications of NMLs and guidance to researchers using lidars and lidar data.
This paper is structured as follows: Section 2 presents a general overview of the lidar technology for nacelle applications; Section 3 discusses the most relevant studies utilizing forward-looking lidars for inflow characterization; Section 4 delves into the numerous field and virtual lidar experiments targeting the wake region; the most important aspects emerged from the present literature review are summarized at Section 5; finally, conclusive remarks are provided in Section 6.
The concept of NML refers to any configuration that includes a Doppler lidar capable of measuring the wind velocity field installed on a turbine nacelle. The deployment close to the hub of the turbine is particularly advantageous since it provides the best alignment of the lidar probe with the flow experienced by the rotor and also a privileged position to scan the wake region. Before discussing the specific uses of NLM, it is beneficial to review the main principles of lidar technology. This work, in particular, focuses on applications utilizing coherent (or heterodyne) Doppler lidars to measure wind speed. The measurement principle leverages the Doppler shift (Doppler, 1842) occurring between the light emitted from the instrument and the backscatter from aerosols (e.g., dust, pollen, droplets, etc.) in the atmosphere. The shift in frequency of the light is linearly proportional to the line-of-sight (LOS) or radial velocity, uLOS (i.e., the projection of the instantaneous velocity vector onto the laser beam), as follows:
where fout and fin are the emitted and received frequencies, respectively, and c is the speed of light. The two in the denominator signifies that the Doppler shift applies twice: once for the emitted light that hits the aerosol and once for the returned light arriving back at the instrument.
Coherent lidars can be classified into two categories, based on the way their signal is generated and analyzed.
• Continuous wave (CW) lidars process the backscatter detected from the whole span of the beam resulting from a continuous emission of light. For these lidars, the intensity of the backscattered signal is a function of the distance from the source r, and the center of volume of the backscatter intensity is referred to as focal distance, d.
• Pulsed lidars emit the light in the form of laser pulses and sample the backscattered light with a high frequency (on the order of 108 Hz). The return signal is divided into temporal windows that carry information on the air speed of different regions along the line-of-sight, a technique called range gating.
Pulsed lidars are therefore able to retrieve the radial velocity independently at each gate. However, they require a larger sampling time compared to CW lidars because the signal needs to travel back and forth from the lidar to the furthest gate before a new pulse is emitted to avoid contamination of the backscattered signal.
The LOS velocity retrieved by the lidar at each point is the result of the spectral analysis of the return signal backscattered by the aerosol particles and illuminated with different intensities by the laser pulse. A widely accepted simplification considers the LOS velocity to be equivalent to the mean of the backscattered signal along the laser beam weighted by a function that depends on the distance along the beam and the type of lidar. This process, referred to as probe averaging, is generally formalized as:
where uLOS(d, t) is the retrieval, u is the 3-D velocity vector at location r along the laser beam and time
FIGURE 1. Example lidar weighting functions: (A) continuous wave lidar, as a function of the focal distance and range, with the location of the maximum value and the ranges where the weighting function crosses half of the peak value (relevant for the definition of the full-width half-maximum) marked as red lines; (B) pulsed lidar, as a function of the range gate length, Δp, and for a pulse with a full-width half-maximum of Δr = 30 m. For more details, refer to Simley et al. (2014a).
Coherent lidars calculate the Doppler shift through a Fast-Fourier Transform of the recorded signal. They normally acquire data over a certain time window to build an average spectrum that guarantees a statistically significant prominence of the energy peak due to wind speed above the noise floor (a process called accumulation (Rye and Hardesty, 1993)). Temporal resolution is further reduced by the processing time and scanning head rotation, which may reduce the actual acquisition time to 25% of the total operation period.
From a technological standpoint, there are several factors that have contributed to the recent success of lidars for wind energy and atmospheric research, such as reduced costs and size, enhanced capabilities, and easier and safer operation compared to the initial prototypes. Improved electronic signal analysis techniques resulted in a considerably shorter processing time (i.e., more visibility at closer ranges, a higher sampling rate) and more accurate estimation of the Doppler shift (i.e., increased data availability and resolution) (Emeis et al., 2007). Furthermore, the development of fiber optics permitted drastic miniaturization of internal components (Fujii and Fukuchi, 2005). Finally, modern solid-state light sources emit lasers with frequencies within the range of eye-safe wavelengths, easing the safety requirements for deployment (Reitebuch, 2012).
Nevertheless, the acquisition process outlined above presents three major limitations that preclude the exact reconstruction of the instantaneous turbulent flow field. First, the combination of probe averaging and accumulation acts as a low-pass filter that dampens the smaller energy-containing turbulent scales. This effect leads to, for instance, a problematic estimation of second-order moments (Sathe et al., 2011). Second, the intrinsic reliance of the measurement on line-of-sight visibility and aerosol concentration leads to inconsistent data availability (Davoust et al., 2014) and contamination due to bandwidth noise (Penã et al., 2017). Fortunately, most of the outliers can be flagged based on their carrier-to-noise-ratio (CNR). The last limitation of lidars is their inability to measure the 3-D velocity but rather its projection onto the along-beam direction gives rise to the so-called “cyclops dilemma,” the indeterminacy caused by the lack of information on the cross-beam velocity components. Such an impasse is generally overcome in one of these three ways: either the flow field measured by multiple lidar beams emitted by a single lidar is assumed to be homogeneous (e.g., Sathe et al., 2015), or multiple lidars are deployed to perform multi-Doppler retrievals at the same point in space (e.g., Newsom et al., 2013), or a known wind direction and a negligible vertical velocity are assumed (see Zhan et al. (2019); Eq. 2). The last approach is hereinafter called wind speed de-projection (WSD). It is noteworthy that the breakdown of these assumptions on the flow field generates a geometrical error (i.e., the contamination of transverse velocity components onto the line-of-sight) on the mean velocity field (Simley et al., 2014a), and its second-order statistics (Fu et al., 2021).
In the past few years, several Doppler lidars have been adapted to allow installation on turbine nacelles, with several levels of customization, also including commercial NML models. The most common type of systems can be summarized as follows.
• Scanning lidars probe customized patterns and represent the most versatile technology to scan both inflow and wake; however, high costs and complex programming generally relegate this type of lidar to advanced research applications; the flexibility of scanning lidars allows the user to schedule several scanning patterns, the most common being the Plan Position Indicator (PPI, Figure 2A), the Range Height Indicator (RHI, Figure 2B), the volumetric scan (Figure 2C), and staring scans for special turbulent measurements (Figure 2D).
• Fixed-beam lidars emit multiple beams (e.g., Figures 2E,F) at fixed orientations to reconstruct basic wind flow information, such as mean wind speed, vertical wind shear, and wind direction. These lidars have been typically used to recalibrate the yaw controller to minimize power losses due to misalignment, and as for power performance validation and feedforward control. Such instruments represent the most common type of commercially available NML.
• Conical lidars scan circular paths at fixed focal distances upstream of a rotor to reconstruct mean wind speed, shear, and wind direction (Figure 2G). These lidars can be pulsed or CW, the latter being typically retrofitted vertical profilers that are re-adapted for turbine control applications.
• Spinner lidars, originally developed at the Technical University of Denmark (Mikkelsen et al., 2013), are continuous-wave profiling lidars that can be installed on the turbine spinner (Figure 2H). Rapid rotation of the internal optics (superposed to that of the mounting itself if spinner-mounted) allows the spinner lidar to scan with extremely high spatial resolution and a sampling frequency of 500 Hz when operating at a fixed focal distance (Machefaux et al., 2015). Such lidars can also be installed through the back wall of the turbine nacelle for fast wake measurements (Herges et al., 2017).
FIGURE 2. Schematic of different nacelle-mounted lidar scans: (A) PPI; (B) RHI; (C) volumetric; (D) stare scans; (E) fixed 2-beam; (F) fixed 4-beam; (G) conical, (H) spinner. Illustration by Besiki Kazaishvili (NREL).
Before moving into the details of prominent studies using NML data, it is worth discussing the general trends and evolution of scientific research on this topic. Figure 3 shows the temporal distribution of the journal articles discussed in this review, classified according to different criteria. Figure 3A highlights a prevalence of studies focusing on the inflow, especially before 2015, boosted by a proliferation of publications regarding wind turbine control (Figure 3B), a topic that received significant attention from the industrial community at that time, likely as a consequence of increased research funding and the creation of new lidar companies. The success of seminal field lidar campaigns (e.g., Bingöl, 2009; Krishnamurthy et al., 2013; Mikkelsen et al., 2013; Iungo and Porté-Agel, 2014; Machefaux et al., 2015) appears to be a key factor that raised the interest of the scientific community in this type of instrument after 2014, leading to a renewed interest in lidars and a more diverse panorama of research applications (Figure 3B). As expected, the larger availability of lidars in the past few years somewhat limited the adoption of numerical techniques (Figure 3C), given the clear preponderance of experimental studies compared to numerical methods, which were more popular before 2015. It is noteworthy that numerical studies on this topic routinely include so-called virtual lidars, namely, a numerical twin of a lidar that samples the velocity fields generated through simulations mimicking the acquisition process of a real instrument. Regardless of the accuracy of the simulated flow, discrepancies between virtual and real lidar measurements depend mainly on the fidelity of the numerical lidar model. In general, projection onto the LOS direction and probe averaging are the minimum requirements and the most commonly implemented features for a realistic virtual lidar, whereas real-world effects such as sight blockage, pointing error, and noise contamination are rarely simulated.
FIGURE 3. Temporal distribution of the NML studies included in the present review, classified based on (A) lidar orientation (forward vs. backward), (B) application, and (C) methodology used to collect the data.
Lidars installed on wind turbine nacelles have been increasingly used in the past 2 decades to detect the wind velocity field impinging on the rotor. In fact, NMLs have been recently included for the first time in the International Electrotechnical Committee Standards IEC (2022), which substantiates the increasing acceptance of this remote sensing technology in the wind energy industry (Clifton et al., 2018). It is noteworthy that the former document does not include guidelines on the raw data quality control, the wind reconstruction algorithm, nor the effects of complex terrain on measurement quality, because these topics are still the subject of ongoing research. From a pragmatic standpoint, NMLs represent an appealing alternative to met mast measurements for inflow sampling due to the larger field of view (Mikkelsen et al., 2013), and relatively low costs and maintenance (Slinger et al., 2013). Additionally, as the lidar yaws with the wind turbine, the measurements are guaranteed to always measure upstream of the rotor, whereas a met mast remains fixed and can be affected by the wind turbine wake for certain wind directions, thus reducing data availability, and decreasing the correlation with turbine response for large spanwise separation (Wagner et al., 2014). In this regard, Table 1 reports the main advantages of lidars relative to met masts in terms of their technical specifications and capabilities.
TABLE 1. Comparison of met masts, pulsed, and CW lidars for inflow measurements. For the entries related to capabilities (last 7 rows): ✓: provided directly or derived via traditional methods; •: derived via special methods; ✕: unavailable.
The reviewed literature reveals a vast breadth of approaches and techniques applied to NMLs for inflow measurements that can be broadly classified into four categories, based on the intended use of the nacelle lidar measurements: 1) characterization of the turbulent inflow to assist feed-forward control (Section 3.1), 2) general reconstruction of the dynamic turbulent field (Section 3.2), 3) performance and load assessment (Section 3.3), and 4) estimation of inflow turbulent statistics to study wake morphology and dynamics (Section 3.4). The following sections cover each subtopic separately, with particular emphasis on the technology employed, scanning strategies, and retrieval techniques. Furthermore, Table 2, which is included at the end of this section, summarizes the lidar scanning strategies and wind reconstruction techniques reviewed in this section.
TABLE 2. Summary of the reviewed scanning strategies and wind reconstruction techniques applied to nacelle-mounted lidars for inflow sampling. A zero-azimuth, θ = 0, corresponds to the direction of the rotor axis pointing upstream. Refer to the nomenclature at the beginning of the manuscript for the explanation of symbols and acronyms.
The development of NMLs inspired widespread studies of advanced control techniques for wind turbines that leverage the predictive knowledge of the incoming flow in the rotor-swept area to reduce the levelized cost of wind energy. In fact, state-of-the-art controllers use a feedback architecture, in which the disturbances caused by the turbulent wind velocity field are minimized through appropriate counteractions of the control system after they have impacted the rotor (Pao and Johnson, 2011). Therefore, one of the most promising types of lidar-assisted control (LAC) is feedforward control. With this type of control, preview measurements of the approaching flow are used to mitigate the impact of turbulent structures on the turbine operation without having to wait for the feedback controller to react to deviations of the turbine system from the reference state. The logic of modern variable speed-pitch regulated wind turbines is generally based on the classification of the operative regime of the turbine into three so-called power curve regions based on the inflow wind speed at the rotor, U∞ (Foti et al., 2018).
• Region 1 (0 ≤ U∞ < Ucut-in): the turbine is not producing active power and the blades are pitched to minimize the aerodynamic loads;
• Region 2 (Ucut-in ≤ U∞ < Urated): the power production is maximized by tracking the optimal cp point through the regulation of the generator torque;
• Region 3 (Urated ≤ U∞ < Ucut-off): the active power output is set to its rated value and the blades are actively pitched to maintain the rotation speed as close as possible to its set point.
Systematic research on this concept can be traced back to Harris et al. (2006), a seminal study that paved the way for many subsequent works on lidar-based feedforward control. Their study thoroughly analyzed the most relevant technical aspects of NMLs and selected three hypothetical lidar models that were simulated in TurbSim (Jonkman, 2006) while the turbine reaction was studied in FAST (Jonkman and Buhl, 2005). The possible benefits of LAC spanned the whole power curve, including enhanced efficiency in region 2, smoother transition between region 2 and 3 and startup/shutdown operations, improved speed regulation in region 3, and load reduction. However, only the latter two aspects were tested. A 10% reduction in Damage Equivalent Load (DEL) (IEC, 2005) for the flapwise blade root bending moment could be achieved thanks to the introduction of the lidar, with less clear advantages reported for speed regulation. Most importantly, those results were promising enough to spur the interest of the research community in this technology.
Since then, researchers have been focusing on the optimization of lidar scanning strategy and controller architecture, error quantification, performance analysis, and understanding the physics of the evolving turbulent wind field.
The last element, in particular, plays a crucial role in the context of LAC. Wind turbines operate in the lower part of the atmospheric boundary layer and are therefore exposed to a highly turbulent flow whose spatio-temporal characteristics are generally dictated by local climatology, mesoscale circulation, local orography (or ocean surface), and thermal stratification. These factors make the flow characterization remarkably challenging from both an experimental and theoretical standpoints (Veers et al., 2019). The intrinsic limitations of lidar technology outlined in Section 2, such as its spatial averaging and limited temporal resolution, confine the range of turbulent scales that can be observed. Moreover, in LAC applications, because of the near-range blind region of the lidar, the high geometrical error induced by scans with large coning angles (Simley et al., 2011), and the inevitable processing and actuation time, the flow is always sampled tens to hundreds of meters upstream of the rotor. This further complicates the estimation of the velocity sensed by the rotor due to the wind evolution, viz. the modification of the velocity field occurring as the turbulent structures are advected from the focal point of the lidar to the turbine. Indeed, the greatest body of research on the turbulence characteristics for LAC applications is somehow related to the problem of wind evolution.
The simplest approach to deal with wind evolution is that of assuming the Taylor-frozen hypothesis (TFH) (Taylor, 1938), namely, that turbulent structures are advected unchanged from the measuring plane to the rotor. More sophisticated approaches take into consideration the temporal evolution of smaller turbulent eddies. From a statistical standpoint, the degree of wind evolution is typically characterized in terms of longitudinal coherence, which quantifies the degree of correlation between wind speeds at two points as a function of the frequency and longitudinal separation, and is defined as:
where
The following sections describe the research effort regarding LAC, with particular emphasis on flow physics and experimental techniques. Additional details on the controller algorithms, which are beyond the scope of the present work, can be found in more specific publications (Scholbrock et al., 2016; Simley et al., 2018). Existing literature is classified into two categories: LAC for load reduction (and rotor speed stabilization) in region 3 (Section 3.1.1) and LAC for performance enhancement (3.1.2).
This review revealed that there is widespread consensus about the fact that load reduction in above-rated operation and rotor/generator speed stabilization is the most promising application for LAC (Scholbrock et al., 2016; Simley et al., 2018). It comes as no surprise that the vast majority of the published studies on LAC tackle this topic. In spite of the agreement on a conceptual level on the effectiveness of LAC in region 3, the claimed benefits of LAC span a wide range of values. Figure 4 reports the relative reduction of the most commonly used objective functions for turbine control, which range from a remarkable 30%–60% to more modest decrements. This is associated with the vast variety of experimental and/or numerical setups, controller schemes used, as well as case-specific inflow conditions and turbulence modeling.
FIGURE 4. Maximum reduction of objective functions when using lidar-assisted controls: root mean square (RMS) of generator speed error (A); damage equivalent load (DEL) of tower base fore-aft moment (B); and blade root flapwise bending moment (C) reported in the cited references.
In terms of methodology, it is observed that earlier studies generally relied on synthetic measurements, and leverage the TFH to model wind evolution. For instance, in the pioneering work by Laks et al. (2011) synthetic frozen turbulence (Taylor, 1938) generated by TurbSim was sampled from several simulations at different turbulent levels to reproduce rotating and non-rotating upstream measurements with a highly idealized nacelle lidar. They reported significantly reduced loads compared to the baseline in region 3 when injecting the rotating lidar preview into the IPC algorithm, but unsatisfactory results when more realistic stationary measurements are injected into the controller, especially for highly turbulent flow. Dunne et al. (2012) followed up with a study testing two lidar configurations for pitch control: one using three independent staring CW lidars installed on the blades (Dunne et al., 2011) and the other with a single pulsed lidar scanning in a conical fashion (Schlipf et al., 2010a). Although it was confirmed that the first approach results in a better estimation of the blade-equivalent velocities, the second is more realistic and even more accurate at measuring the rotor-equivalent wind speed.
The early work of Simley et al. (2011) dissected the sources of inaccuracy in the measurements of the hub-height wind speed introduced by a CW lidar scanning in a circle. They found that the geometrical error dominates the close ranges, whereas probe averaging becomes dominant for large focal distances (frozen turbulence was assumed in the studies). These two factors led to the important conclusion that an optimal preview distance can be identified by minimizing the root mean square (RMS) error of the hub-height wind speed as a function of the inflow conditions and the target scanning radius. Such findings were expanded in Simley et al. (2014a) with the introduction of measurement noise, a pulsed lidar, coherent turbulent structures, and the estimation of blade-equivalent wind speed. Another study that stands alone in this context is that of Wortmann et al. (2016). Those authors envisioned an ideal lidar-assisted IPC capable of compensating the vertical and horizontal shear, which are typically neglected. Although their results indicate a
The absence of wind evolution in the previous studies leads to the counter-intuitive result that the measurement error decreases monotonically with the preview distance for a pulsed lidar. This inconsistency spurred the interest in the investigation of the effect of wind evolution on LAC performances and design. Schlipf et al. (2010b) challenged the validity of the TFH in a field experiment and found that frozen turbulence can be assumed up to a wavenumber k = 0.125 rad m−1 (wavelength
The identification of the optimal scan geometry in this complex scenario is sensitive to the metric used to quantify the degree of coherence. Dunne and Pao (2013) confirmed the importance of maximizing coherence between measured and actual rotor wind speed. They adopted a highly idealized numerical setup in FAST to prove that increasing coherence bandwidth (i.e., the wavenumber at which coherence drops below a desired threshold) is beneficial (with diminishing returns) for the performance of a speed regulation and pitch actuation in region 3. The work was further expanded in Dunne and Pao (2016) with an improved optimal controller algorithm, which led to a reduction in the generator speed RMS error up to 50% compared to the baseline feedback controller. The independent work of Bossanyi (2013) builds upon the previous study and provides actionable guidelines by combining a realistic lidar model, similar to Simley et al. (2012), with a control-oriented approach like Dunne and Pao (2013). The authors mimicked several scanning modes into a time-evolving velocity field, and highlighted that the intrinsic spatio-temporal filtering performed by the lidar acquisition process can provide the desired damping of small-scale turbulent features. Such features exhibit a lower correlation with the turbine response and would cause detrimental control over-actuation. This filtering also assuaged the effect of wind evolution on the results. The same study also showed a significant reduction in fatigue and extreme loads attainable through lidar-assisted collective pitch control (CPC), but negligible gains for optimal cp tracking in region 2. In a following study, Laks et al. (2013) tested the findings of Bossanyi (2013) but for an individual pitch control (IPC) logic and confirmed the beneficial effect of the lidar probe averaging in removing uncorrelated high-frequency disturbances. Further insight into the effect of lidar processing on coherence is lent by the experimental study by Held and Mann (2019b), who calculated the coherence of the rotor-equivalent wind speed from two fixed-beam NMLs and found excellent agreement with the proposed spectral model. The authors reported a higher coherence for the lidar with more beams.
A more proactive approach to deal with wind evolution is that of intentionally low-pass filtering the uncorrelated small-scale turbulence via a lidar-to-turbine transfer function. Schlipf et al. (2012) used this method in a field test on the 600-kW two-bladed Controls Advanced Research Turbine (CART2) (Bossanyi et al., 2010) at the National Renewable Energy Laboratory’s Flatirons Campus (see Figure 5). The authors tested a feed-forward collective blade pitch controller to dampen fluctuations in the rotational speed of the rotor in region 3 caused by turbulence. The lidar used in their study was a retrofitted profiling WindCube scanning a circular trajectory scan with 6 points at 5 focal distances. The results showed a 30% reduction of the standard deviation of the rotational speed and a 10% decrease in tower loads during periods with a high correlation between lidar and turbine, whereas a performance decay could be observed during poorly correlated measurements. Spectral analysis revealed that mainly loads associated with low-frequency modes are lowered, in accordance with most of the previous studies. Scholbrock et al. (2013) carried out a similar experiment by deploying a 3-beam pulsed lidar on top of the 3-bladed Controls Advanced Research Turbine (CART3) turbine able to reconstruct the 3-D inflow velocity, assuming homogeneous flow. The lidar-assisted collective pitch controller in region 3, based on the work of Schlipf et al. (2013a), achieved a 14% reduction of rotor speed fluctuations compared to the standard feedback control logic, but less evident load reduction.
FIGURE 5. Different NMLs installed on the CART turbine: (A) retrofitted WindCube (Schlipf et al., 2012); (B) 2-beam Avent WindIris (Fleming et al., 2014); (C) BlueScout Technologies OCS (Scholbrock et al., 2013); (D) ZephIR (Haizmann et al., 2015; Scholbrock et al., 2015).
Also on this topic, Schlipf et al. (2013a) conducted a more in-depth investigation on the optimal degree of filtering of the lidar signal and provided an analytical expression for the transfer function from the measured wind speed to the rotor effective wind speed, which can be also approximated by a low-pass filter. The approach was validated against preliminary experimental data and used to carry out a design exercise for a 3-beam lidar. Haizmann et al. (2015) implemented a CPC strategy based on the measurements of a CW ZephIR lidar scanning in a circle focused 1.3D in front of the CART3 with a scan geometry that was optimized using the method by Schlipf et al. (2013a). They demonstrated a good correlation between the predicted wind speed and the rotor-effective wind speed and the theoretical and real lidar-to-turbine transfer function. A significant reduction in rotor speed fluctuations was also observed, but only for a short observational period. Kumar et al. (2015) tested feedforward CPC on the CART3 using an Avent five-beam pulsed lidar measuring at four range gates from 50 m to 80 m upstream of the lidar (1.2D–1.9D). The authors observed reductions in rotor speed variations, tower fatigue loads, and blade pitch actuation effort with feedforward control. They also reported that the coherence between the lidar-based and rotor-effective wind speed can be increased by averaging several range gates, thanks to a more effective coverage of the rotor-swept area. Thus, they highlighted a possible advantage of pulsed technology over the CW counterpart. Both Haizmann et al. (2015) and Kumar et al. (2015) found that loads could be reduced further (while still reducing rotor speed variations relative to the baseline feedback controller) by decreasing the feedback controller gains and relying more on feedforward control to regulate rotor speed.
The previously mentioned successful examples of low-pass filtering of the wind speed gave further momentum to frequency-domain approaches for scan optimization and controller design. In a comprehensive study, Schlipf et al. (2015) simulated atmospheric surface layer turbulence through a Kaimal spectral model and measured coherence by installing a staring retrofitted Windcube on the nacelle. The information was used to design the low-pass filter that alleviated the effects of high-frequency uncorrelated turbulence that was measured by the lidar and to optimize a circular scanning pattern that maximized the coherence bandwidth. An exhaustive scan design exercise encompassing time-based and frequency-domain techniques reviewed heretofore is also included in the last part of the review study by Simley et al. (2018), where the performance of pulsed and CW lidars with different scan configurations was tested through a frequency-domain approach. A novelty of their method is the closed-form calculation, not only of the coherence bandwidth, but also of the rotor-averaged wind speed error and the generator speed fluctuations. The conically scanning CW lidar, which thanks to its ability to quickly scan the whole rotor area produced the smallest error (in agreement with Bossanyi et al. (2014)), was further studied in the time domain to optimize the filter and the synchronization between measured wind speed signal and actuator action.
A characteristic of the foregoing studies is the use of coherence models applicable only to a specific range of wind conditions. Simley and Pao (2015) overcome this limitation by analyzing a variety of LES wind fields representing different atmospheric conditions to develop a longitudinal coherence model for wind evolution, including the dependence on mean wind speed, turbulence kinetic energy, and turbulence length scale. The authors observed that coherence decreases for a given longitudinal separation as the turbulence level increases, and the maximum coherence at low frequencies decreases as the turbulence length scale becomes smaller (typical of more stable conditions). Davoust and von Terzi (2016) evaluated the wind evolution model proposed by Simley and Pao (2015) using measurements from a nacelle-mounted five-beam pulsed lidar, confirming the general dependence of the longitudinal coherence on turbulence intensity and turbulence length scale, albeit with weaker overall trends. More recently, Chen et al. (2021) addressed the issue of wind evolution by means of machine learning. To this aim, they trained a regression Gaussian process to predict the coherence obtained through statistical analysis of stare scans from two field campaigns. Up to 12 predictors of streamwise coherence were tested (e.g., mean wind speed, stability, 2-nd, 3-rd and 4-th order moments of wind speed, etc.). Almost all predictors increased the accuracy of the model, which confirms the complexity of the wind evolution in real-world scenarios.
Whereas the spectral properties of the wind field have received large attention, there have been sporadic studies of other aspects of the inflow physics in the context of LAC. For instance, the implementation of a feedforward control scheme requires a careful estimation of the temporal properties of the flow, namely, the advection time between the sampling plane and the turbine. In this regard, Dunne et al. (2014) analyzed data from a field test on the CART2 and were able to achieve a 13% larger coherence bandwidth by improving the estimation of the advection time of the wind between the upstream measurement location and the rotor. Their approach requires the estimation of a variable time delay, which is challenging to perform online. In contrast, Held and Mann (2019b) showed a negligible bias when estimating the advection time by simply using the preview distance divided by the 10-min averaged wind speed, although their experimental data exhibited a significant scattering. An aspect largely neglected in many studies is the influence of the rotor thrust on the incoming velocity field, which is the focus of Simley et al. (2014b). The authors used LES and a virtual lidar to address the issue of the flow distortion caused by measurements performed in the induction zone. They reported that the optimal radius in case of induction is slightly reduced due to streamline expansion, although the effect is negligible compared to the impacts of line-of-sight measurements, limited measurement points within the rotor disk area, and wind evolution.
Overall, the present survey of the literature reveals a quite complex panorama of LAC applied to region 3 operation, with a variety of results and approaches. Interestingly, the analysis of the properties of the turbulent field and its impact on LAC have received remarkable attention. In particular, the effect of wind evolution has been thoroughly characterized both numerically and experimentally. However, regardless of the general consensus achieved on the physical mechanisms driving LAC, a common scanning strategy and controller architecture have not emerged yet, which represents a barrier to the application of LAC at an industrial scale (Clifton et al., 2018; Simley et al., 2018).
In spite of the preponderance of LAC application for region 3, several studies explored lidar preview for cp enhancements in region 2 and are the object of this section. As pointed out by Schlipf et al. (2011), two avenues can be taken in this field: 1) direct rotor speed control using the preview wind speed from the lidar to keep the tip-speed-ratio as close as possible to its optimal value, or 2) lidar-assisted yaw control where wind direction over the entire rotor is previewed to ensure rotor alignment. Schlipf et al. (2013b) estimated the benefit of direct speed control in region 2 by injecting in a FAST simulation real time series of rotor-effective wind speed and lidar measurement carried out on the CART3 turbine. In spite of a 40% reduction in the standard deviation of tip-speed ratio, power gains were negligible and fatigue load on the shaft doubled. Wang et al. (2013) looked deeper into this topic and simulated three different control strategies for cp enhancement: 1) the disturbance tracking control (aimed at maintaining constant tip-speed ratio), 2) optimal rotor control (which is a modified version of the traditional control scheme based on torque gain), and 3) a preview control tracking a lidar-based cp. Even in highly idealized conditions, the maximum increase in energy production is 0.5% which comes at the cost of a detrimental increase in DEL of up to 60% on the low-speed shaft.
Lidar-assisted yaw control has also been explored in several studies. Kragh et al. (2013) assessed the feasibility of an enhanced yaw control based on spinner lidar measurements using virtual sampling of synthetic frozen turbulence. They showed large coning angles are beneficial for estimating wind direction in highly turbulent inflows, a consideration that must be integrated with that of Simley et al. (2011, 2014a) who concluded that increasing the scan amplitude results in a worse estimate of the wind speed due to geometrical error. The implementation of the yaw reconstruction by Kragh et al. (2013) on a limited set of experimental data showed promising results. The authors also warned against the contamination of the results from horizontal wind shear or sloped inflow, which make the estimation of yaw quite complex due to the cyclops dilemma. Slinger et al. (2013) used the yaw information previewed by a nacelle-mounted CW ZephIR lidar to correct the misalignment of a 2-MW turbine and reported an AEP increase of 5%. Effects of atmospheric stability and of the induction zone were also observed. Similarly, Fleming et al. (2014) measured through a 2-beam WindIris lidar a 7–9° yaw error during turbine operation and used this finding to recalibrate the yaw controller of the CART2. A subsequent comparison of turbine data with and without lidar-based yaw correction showed mixed results in terms of power curve, although a 2.4% increase in AEP provided by the enhanced controller was estimated by the authors. Scholbrock et al. (2015) followed a different approach, by feeding the lidar wind direction directly into the control loop of the CART3 turbine. The additional technical challenges of this method compared to a simpler yaw calibration were compensated by improved power capture from correcting the 20° yaw error caused by electrical noise. More recently, Simley et al. (2021a) in their study on wake steering (see Section 4.2) reported a significant and wind speed-dependent bias between the yaw misalignment estimated from a 4-beam pulsed lidar and the nacelle vane. They attributed such discrepancy to a poor calibration of the wind vane and suggested that using NML for the detection of yaw misalignment can indeed improve not only single turbine performance but enhance the effectiveness of advanced wind farm control based on wake steering.
Some simulation studies even investigated the performance of LAC for holistic wind turbine control to enhance both power capture in region 2 and mitigate loads in region 3. In this context, Bossanyi et al. (2014) conducted a comprehensive virtual lidar experiment to evaluate the performance of different scanning strategies in pre-sensing wind speed, direction, and wind shear. They confirmed that large coning angles increase the accuracy of wind direction retrieval at the cost of higher errors in wind speed and shear. Several limitations of LAC were also pointed out, such as the negligible gains in cp in region 2, and the poor additional benefits of using a lidar within a conventional IPC loop. They also proposed a scan sampling
The surveyed literature appears to agree that LAC has uncertain advantages in region 2, where it may be useful more for yaw tracking rather than to optimize the rotor speed. The studies also exhibit a wide variety of approaches, which points to the fact that the optimal measurement strategy is dependent on the application and the specific quantities of interest required to inform the controller.
As already highlighted in the previous sections, lidars provide just partial information about the incoming turbulent flow, mainly due to the cyclops dilemma, probe averaging, and finite sampling speed. In order to get a more complete picture of the flow structures impacting turbine operation, it is necessary to apply flow reconstruction techniques, which span from the simple de-projection of the LOS velocity to more sophisticated data assimilation methods. In this section, we include studies that primarily deal with the reconstruction of the dynamic turbulent inflow through NMLs, focusing more on the wind field retrieval technique and flow physics and less on the engineering application of the lidar preview.
Early studies mentioning NML fall within this category. One of the first accounts of a lidar study of a turbine turbulent inflow is reported by Hardesty and Weber (1987). They anticipated the NML technology by operating a ground-based CW lidar coupled with a rotating mirror placed at different heights to mimic the sampling of the turbulent wind field experienced by a rotating turbine blade. They were able to find a good correspondence between theory and experimental data, which confirmed the spectral distortion caused by the rotation. However, the study that is widely considered the first actual application of NML is the one by Harris et al. (2007), in which a CW staring lidar was installed on top of a utility-scale turbine, with the main aim of testing the feasibility of such a setup to measure turbulent gusts. Their analysis is also relevant because it revealed that the lidar was able to sense the onset of thermally-driven daytime turbulence and quantified the LOS blockage due to the blade passage. Angelou et al. (2010) used a similar setup to conduct a more in-depth analysis of the effect of lidar retrieval on turbulence. Their study, although severely impaired by technical difficulties, provided evidence of the accuracy of the lidar in retrieving the wind speed through a comparison with sonic anemometer data, but also damping of the high-frequency fluctuations due to probe averaging. Mikkelsen et al. (2013) reported the first use of the Spinner lidar for inflow measurement during one nighttime stable observational period and one daytime unstable observational period. The authors observed a remarkable agreement of the horizontal wind speed of the lidar retrieval with the nearby met tower, but more uncertain results on the inflow angle, and were largely attributed to the geometrical error.
More recent research on this topic follows two main paths: on one hand, first- and second-order turbulent statistics (typically 10-min) have been reconstructed from LOS measurements; on the other hand, lidar observations have been assimilated into dynamic wind field models to generate data-driven instantaneous fields. The former approaches are deeply interconnected because the generated turbulence needs to satisfy the statistical properties of the turbulent flow under investigation.
The reconstruction of wind statistics requires adequate correction to overcome the limitation of the lidar acquisition and possible distortion on the freestream due to the turbine aerodynamics. In this regard, Borraccino et al. (2017) conducted a study based on the data from the previously mentioned Nørrekær Enge site, which targeted the estimation of the 10-min average vertical wind profile that partially borrowed the optimization approach by Raach et al. (2014). Their results agree remarkably well with met tower data, including at heights where no LOS measurements were available and in the region close to the rotor after a correction for the rotor induction. The reconstruction of second-order moments (e.g., the turbulence intensity) from the same data is the object of the investigation by Penã et al. (2017), who used both the CW and the 5-beam pulsed lidar to estimate the Reynolds stresses following two approaches. The first one leverages Mann’s turbulence model fitted to the experimental data coupled with a low-pass filter that mimics the lidar acquisition. The second one relies on the raw Doppler spectrum to retrieve the unfiltered LOS variance that is subsequently decomposed to calculate the individual Reynolds stresses. Both methods achieved a reasonable agreement with cup anemometer data for the streamwise component, although the first method suffers from noise contamination at high frequencies. The authors also warned of the detrimental effect of the narrow coning angle on the accuracy of the other components of the Reynolds stress tensor. This warning was not found in previous literature sources, which confirms the multi-faceted nature of the scan design problem. Held and Mann (2019a) also used the broadening of the Doppler spectrum associated with turbulence to detect the presence of wakes in the inflow of a wind turbine with an upstream-facing two-beam Doppler lidar and to overcome the assumption of uniform flow used in previous studies on yaw misalignment (e.g., Fleming et al., 2014; Scholbrock et al., 2015). By comparing the turbulence intensity between two lidar beams to each other and to free stream values, they could detect the presence of a wake faultlessly in a test run at the Risø DTU Høvsøre Test Centre. Furthermore, they observed a bias in the yaw misalignment, which was detected with the two-beam lidar in the presence of an impinging wake, and then provided a correction method. A more straightforward method to estimate the rotor-averaged TI was also proposed by Schlipf et al. (2020) based on a baseline Kaimal spectrum, which exhibited good accuracy but only in a limited numerical environment. More recently, the experimental study by Fu et al. (2021) explored several strategies to retrieve not only the mean and standard deviation of the streamwise velocity but all the terms of the Reynolds stress tensor. In that campaign, fixed-beam lidars and a spinner lidar (all CW) were installed on top of an 850-kW turbine. Among the techniques used, a reconstruction of the turbulence intensity based on the analysis of the Doppler spectrum dispersion from the spinner lidar turned out to be the most accurate way to estimate the Reynolds stresses when compared to sonic data. This is in contrast to the TI retrieved from the fixed-beam LOS velocity, which exhibited a severe underestimation.
In parallel, significant endeavors were devoted to the real-time reconstruction of the turbulent field by assimilating lidar measurement into wind models of increasing complexity. Raach et al. (2014) proposed a dynamic model to estimate mean wind speed and wind speed gradients from a nacelle CW lidar scanning at 5 focal distances with a half-opening angle θmax = 45°. The incoming flow was modeled through a frozen turbulent box subject to pitching and tilting, while the resulting wind field was the result of real-time optimization. One of the main advantages of such a method is the concurrent estimation of horizontal shear and yaw misalignment, which is inherently challenging due to the similar line-of-sight projection of these two flow perturbations and the cyclops dilemma (Kragh et al., 2013). Towers and Jones (2014) developed a dynamic flow algorithm to reconstruct the 2-D turbulent field approaching a wind turbine which was probed by a nacelle lidar emitting two horizontal beams angled 15° and 30°. The narrower scan allowed faster convergence of the computation. The algorithm used a Kalman filter together with a low-order flow model based on the Navier-Stokes equations, which predicted the new field based on the last state and the difference with the current observations. The flow model based on the TFH proved robust in a wide range of wind directions, stabilities, and noise levels.
The same outcome of creating lidar-driven turbulent wind fields can be achieved by generating Gaussian-constrained turbulence using the lidar measurements as a set of dynamic constraints. The method leverages assumptions on the statistical descriptors of the underlying turbulent field (i.e., power spectral density, Gaussianity) and aims to find a set of realizations that are compatible with the observations. An advantage of this method is the analytical evaluation of the variance of the residuals, namely, a spatial field representing the statistical uncertainty of the retrieval. Bos et al. (2016) proposed a technique based on constrained Gaussian turbulence based on Mann’s turbulence model (Mann, 1994) to assimilate the data of a 5-beam NML installed on a 5-MW turbine. This method aimed to reconstruct wind gusts, achieving good agreement with the rotor equivalent wind speed based on the shaft torque. Also, Schlipf and Raach (2016) used a simplified approach in which the phases of turbulent modes satisfying the Kaimal spectrum are optimized to fit an idealized wind gust. Dimitrov and Natarajan (2017) utilized the variance of the residuals to carry out a scan design optimization. Their purely numerical study showed that scans having a large number of points sampled in around 5 s leads to the lowest uncertainty. Their scan design guidelines are compatible with what is reported in LAC studies (e.g., (Bossanyi et al., 2014; Simley et al., 2018)), despite being the result of a completely different scan optimization technique. Pettas et al. (2020) presented a tool named ViConDar specifically conceived to assimilate NML measurements into generic Gaussian turbulence generators. To showcase the capabilities of this tool, they optimized a scan pattern aimed at measuring wind speed and vertical shear under different inflow conditions. The authors showed that, conversely to Dimitrov and Natarajan (2017), increasing the number of sampling points does not necessarily lead to better results if the coverage rotor area is not significantly improved.
To conclude, we would like to highlight that while this paper is limited to NML applications, data assimilation of line-of-sight velocity observations represents a vast topic that exceeds the scope of the present manuscript. Therefore, we suggest the interested reader refer to more general studies (e.g., Newsom and Banta, 2004; Xia et al., 2008; Krishnamurthy et al., 2013; Bauweraerts and Meyers, 2020).
NMLs represent an appealing alternative to traditional in situ anemometers to characterize the operation of utility-scale wind turbines in terms of power and loads, for the reasons outlined at the beginning of Section 3. In particular, because energy production represents the most relevant performance indicator for the wind energy stakeholder, the number of power performance testing campaigns has been increasing globally, with the majority of these campaigns today performed in North America and Europe. Current estimates (Vaisala, 2023) indicate that about 500 power performance testing campaigns will be conducted per year across the globe in this decade, with about 1/5 taking place offshore. With the growing lidar acceptance within the industrial community and the release of the IEC 61400-50-3 (IEC, 2022), the number of campaigns using NMLs will continuously grow as well. Figure 6 shows the expected trend in the yearly number of power performance tests executed using NMLs for onshore and offshore sites, which highlights the significant expansion of this remote sensing technology.
FIGURE 6. Past estimates and future predictions of the total number of power performance tests carried out using NMLs worldwide. Source: Vaisala (2023).
The development of commercial NMLs in this sector comes after at least a decade of scientific studies that assessed the feasibility and accuracy of this methodology. The pioneering work of Mikkelsen et al. (2013), which paved the way for the utilization of spinner lidars, includes one of the first reported power performance tests using lidars. They used the incoming wind speed derived from the lidar to reconstruct the low-speed part of the power curve of a 2.3-MW turbine that exhibited less scatter than the met tower measurement, seemingly due to a more representative measurement of the rotor-equivalent wind speed achievable with the spinner lidar. This advantage of lidars, along with many others, is reiterated by Slinger et al. (2013) who installed a CW ZephIR lidar on the hub of a 2-MW turbine to scan horizontally at several upstream focal points. Their main findings were that the lidar significantly improved the yaw estimation and that the wind turbine power curve, which was reconstructed using the horizontal wind speed from the lidar, matched satisfactorily the nominal one, after a proper correction for the rotor induction. The large data availability from this study also allowed the detection of the influence of atmospheric stability on power capture. Subsequently, Wagner et al. (2014) used a 2-beam pulsed lidar to compute the power curve of a multi-megawatt wind turbine and compared it to the analogous function derived from met mast data They found a difference of 2.3% in the resulting AEP with the one obtained from met mast measurements. Although a univocal explanation was not provided for such discrepancy, they observed less scattering in the lidar-derived power curve and associated that with the dynamic alignment of the lidar probe volume with the wind direction. Wagner et al. (2016) extended the previous analysis by conducting a thorough uncertainty quantification and concluded that, although lidar has higher instrumental uncertainty than the met tower, it compensates by allowing more data availability and thus higher statistical significance. Overall, the lidar-based AEP showed only 1.1% higher uncertainty than the reference one derived from cup anemometer. Shin et al. (2019) confirmed the former result after comparing the power curves obtained from a 2-beam WindIris and from a cup anemometer, with and without rotor-equivalent wind speed correction based on a ground-based lidar. Using the same setup, Shin and Ko (2019) extended the calculation of the power curve to turbines located more than 4 rotor diameters from the reference met mast through a lidar-based nacelle transfer function approach (IEC, 2013). Namely, the bias in the nacelle anemometer due to local flow distortion was corrected on a statistical basis using the hub-height wind speed from the NML. The resulting power curve calculated from the corrected nacelle anemometer had a
The characterization of wind turbine loads is also important to estimate the lifetime and maintenance costs of wind plants. On this front, NMLs can provide important insight into the incoming turbulent field. Because the fluctuating component of the wind field plays a major role in the build-up of fatigue and extreme loads, contrary to power performance tests that focus on mean wind speed, lidar-based load analysis requires high-frequency reconstruction of the turbulent inflow and/or second-order statistics. The previously cited numerical study by Dimitrov and Natarajan (2017) already proved the effectiveness of the proposed lidar-constrained turbulence optimization in the reduction of the uncertainty of those components of loads connected to the streamwise velocity fluctuations. Later on, Dimitrov (2019) assessed the capability of lidars to estimate fatigue loads on a 2.3-MW turbine at the Nørrekær Enge site. They used a 5-beam pulsed and a CW lidar, scanning in a conical fashion, stacked on the nacelle roof of the turbine, which was instrumented with load sensors. The wind reconstruction algorithm allowed them to retrieve mean wind speed, shear, corrected turbulence intensity (see Penã et al., 2017), and the spectra to be injected into an aeroelastic simulator alongside sonic anemometer data. Their model takes into account turbulent damping due to the lidar acquisition and induction zone, and showed an equal or lower error of lidar-based load estimation relative to loads calculated using met tower observations. Conti et al. (2020) extended the former analysis to cases where incoming wakes affect the flow field. The wake was detected based on the wake-generated turbulence measured by the lidar, and load simulation results were classified based on fully, partially, and non-waked conditions. The accuracy of the load estimation was observed to drop during waked conditions. Interestingly, power, although mainly driven by the mean wind speed, was also poorly estimated under waked conditions (up to 7% bias). This calls into question the ability of the scanning strategy that was to properly characterize an inherently 3-D and inhomogeneous flow. The problem of load estimation in waked flow was further examined by Conti et al. (2021b) who leveraged virtual lidar measurements in the dynamic wake meandering (DWM) (Larsen et al., 2008) framework to reconstruct the turbulent field used as an input for load calculations. The wake velocity field was reconstructed using both constrained Gaussian turbulence (Dimitrov and Natarajan, 2017) and a method that superposes a Gaussian velocity deficit to a random turbulent field to reproduce the meandering of an upstream wake. Out of the several scanning strategies implemented, those sampling the rotor area using a large number of points provided the most accurate results, thus confirming the guidelines of LAC studies (Bossanyi et al., 2014; Simley et al., 2018). The accuracy was negatively affected by probe averaging, but not by sampling time, as long as it remained below the typical wake meandering period (Larsen et al., 2008). This consideration seems to indicate pulsed lidar as the most suitable technology to scan intra-plant flow for measurement distances where CW lidar probe volumes become significantly large.
Power performance tests that leverage NMLs are on the verge of becoming an industrial standard. This is primarily because of the accuracy of NMLs in reconstructing mean wind speed and the larger data availability compared to met towers, stemming from the ability to measure the wind inflow for all wind directions. A lidar system can also be conveniently relocated on different turbines, with a substantial reduction in time and cost compared to more rigid in situ sensors. On the other hand, load estimation from lidar, although promising, is still relegated to more advanced research studies, due to the complicated process of retrieving high order statistics from the lidar.
Studies addressing turbine wakes, which will be extensively described in Section 4, are inherently intertwined with an adequate characterization of the turbulent inflow to the turbine, which ultimately affects the wake morphology. Wake studies encompassing an inflow measurement through an NML are scarce. This is because most of the attention is generally focused on the wake area due to the logistical and financial hurdles that the installation of multiple lidars on one nacelle entails. Because of their unique approaches, wake studies that include NML inflow measurements are treated separately in this subsection.
For instance, in the complex experimental setup described by Machefaux et al. (2015) for the characterization of double-wake flow, a CW lidar was installed on the downwind turbine as a redundant measurement of the incoming wake from the upstream rotor. The authors also addressed the issue of data loss caused by the line-of-sight blockage due to the blade roots. Carbajo Fuertes et al. (2018) and Brugger et al. (2019) used a pair of NMLs to scan the inflow and wake of a wind turbine. The forward-looking lidar performed a sequence of scans including 5 min of PPI scans to detect wind direction, 5 min of RHI scans to characterize vertical shear, and 10-min stares in the streamwise and spanwise direction, respectively, to quantify turbulence intensity (TI). The data were used to normalize and categorize wake observations for model validation. A different configuration is reported by Brugger et al. (2020) who leveraged a 4-beam WindIris in conjunction with a ground-based WindCube profiler to characterize the wind speed and direction for an experimental assessment of the performance of wake steering. Unlike the cited studies that focused on wake statistics, Brugger et al. (2022) investigated wake dynamics using the setup reported in Carbajo Fuertes et al. (2018). They executed fixed stare scans in the lateral direction to detect turbulence intensity and integral time scales relevant for wake meandering analysis. This straightforward scanning strategy has the advantage of providing turbulent quantities of interest from direct statistical analysis of radial velocity data.
In spite of the sporadic use of NML for inflow detection in past wake studies, such application is expected to become more popular in the future due to greater use of lidars in wind energy research and the increasing complexity of wake studies, which often require a detailed and local inflow description not attainable through traditional sensors.
Wind turbine wakes increase the cost of energy for wind plants because they negatively affect downstream turbines by reducing power production and increasing mechanical loads (El-Asha et al., 2017). Wind researchers can mitigate wake effects by incorporating wake models into the wind plant planning, but also during operation through smart control strategies, such as wake steering and induction control. Both approaches require detailed knowledge of the wake structure and the wake physics. Wake measurements using NMLs have made significant contributions across those areas.
First, we will discuss a series of pioneering experiments that developed techniques and methods for nacelle-based wake scanning that were used by many of the studies to follow. After that, we will review the full range of current applications for wake measurements with NML. Specifically, we will discuss in Section 4.1 the development of wake data analysis techniques, in Sec. 4.2 studies on wake steering, in Section 4.3 wake model validation and calibration, in Section 4.4 investigations of the wake structure in general, and in Section 4.5 error analysis based on virtual lidars. Finally, an overview of the reviewed wake scanning literature is presented in Table 3.
TABLE 3. Wake growth rates (k*) as a function of the streamwise turbulence intensity (TI) for the Bastankhah and Porté-Agel (2014) model determined from field experiments with NMLs.
This introductory section summarizes the pioneering efforts using NML for wake detection, which are relevant not only from a historical perspective but most and foremost because they introduced methods for data analysis that have been used and adapted by most of the following studies.
The first field experiment with wake-scanning NMLs was performed at the Risø DTU Høvsøre Test Centre in Denmark from 2004 to 2006 by Bingöl et al. (2010) and Trujillo et al. (2011). They mounted a continuous-wave Doppler lidar on the nacelle of a test wind turbine (38-m rotor diameter) and performed different scan patterns throughout the campaign, including both horizontal cross-sections and vertical cross-sections of the wake.
The first publication on the data set by Bingöl et al. (2010) focused on validating the passive advection hypothesis used in the DWM model (Larsen et al., 2008). The model validation was accomplished by visually comparing the instantaneous wake position observed in the velocity fields that were measured by the lidar, with the wake center positions predicted by the DWM model. The authors could confirm the passive advection hypothesis in large part, but also observed that better agreement between model and observations was achieved with a downstream transport velocity that was slower than the freestream value.
The second publication on the data set by Trujillo et al. (2011) developed a quantitative method for wake tracking by fitting a Gaussian function to the velocity deficit. This allowed them to compare the mean velocity deficit and the turbulence intensity in the meandering frame of reference (MFOR) and nacelle frame of reference (NFOR). The approach is illustrated in Figure 7 and is used to remove the effect of wake meandering from the measurements. A wake-tracking method based on the calculation of the center-of-mass of the velocity deficit was also tested, but spikes in the velocity measurement of the lidar led to biased results. Further, the authors found that the mean velocity deficit had a larger amplitude and smaller width in the MFOR compared to the NFOR and they showed the turbulence that was added by wake meandering. They also observed that the velocity deficit of the wake was slanted (or tilted) in the vertical cross-section instead of having a circular shape.
FIGURE 7. A time series of lateral profiles of the velocity in the far wake of a utility-scale wind turbine. The nacelle frame of reference (A) is relative to the rotor axis of the wind turbine and it is a fixed frame of reference in absence of yaw activity. The meandering frame of reference (B) is a coordinate system that follows the instantaneous wake center. The wake center (blue line) was detected with a Gaussian fit to the velocity deficit. Measurement data from Brugger et al. (2022) was used to create this figure.
The methods developed for this field experiment have been used or adapted by many studies that followed, especially the main scanning patterns, the comparison between MFOR and NFOR, and the wake center detection for wake tracking, which have remained in use up to the present day. After this experiment at a 95-kW test wind turbine, the first wake measurements with an NML at a utility-scale wind turbine were conducted only several years later by Aitken and Lundquist (2014). This paper and other subsequent literature will be discussed in the following sections organized by application.
Wake steering is a wind turbine control technique to enhance the total power production of a wind plant by introducing an intentional yaw misalignment or tilt to an upstream wind turbine. The goal is to deflect the wake away from the downstream wind turbines (Dahlberg and Medici, 2003; Boersma et al., 2017). Wake measurements of NMLs are used for studying the impact of wake steering on the velocity field, and as an input to hypothetical feedback control loops for active yaw control.
Several authors have experimentally observed a direct proportionality between wake deflection and yaw misalignment as a function of the downstream distances using NMLs. In addition to this shared finding, each study further investigated different aspects related to wake steering. Specifically, Bromm et al. (2018) presented an insightful analysis of the effect that a downward tilting of the lidar measurement plane, due to the deflection of the tower subject to axial thrust, can have on the detected wake position. Tracking the wake based on the minimum power of a hypothetical downstream turbine, the authors showed that the wake deflection increases with the yaw misalignment. An uncertainty study accompanying their findings revealed that a downward tilting of the lidar scanning plane leads to an artificial bias in the observed wake deflection at large downstream distances. Using volumetric measurements, Annoni et al. (2018) showed a wake deformation proportional to the yaw misalignment. They also compared the mean velocity deficit for aligned and yawed operating conditions to three analytical wake models. They found good agreement between the measured velocity deficits and a Gaussian wake model (Bastankhah and Porté-Agel, 2016), which accounts for the impact of TI. Brugger et al. (2020) validated the outcome of three analytical models against their findings for the wake deflection and showed that wake steering can have detrimental effects on the combined power of a pair of wake steering wind turbines if the wind direction input of the wake steering turbine is biased.
Besides validating the effectiveness of wake steering, the potential of NMLs to enhance wake steering during live operation has also been studied. Raach et al. (2017) presented a method to use wake tracking with an NML as input for a closed-loop wake steering controller. They detected the wake position based on the minimum power of a hypothetical downstream turbine and used it to inform the controller to steer the wake to the desired position. The development was based on synthetic lidar measurements in a simulated wind field with a volumetric scan from the nacelle in the downstream direction. In connection to closed-loop wake steering controllers, the method to detect wakes from the measurements of a downstream facing two-beam Doppler lidar shown by Castillo et al. (2020) is of interest. Two-beam Doppler lidars are cheaper than scanning lidars and are easier to deploy on a nacelle due to their smaller size, thus reducing hurdles for industry adoption. The wake detection method itself is based on peaks in spectral energy of the longitudinal velocity at multiples of the rotor revolution frequency. Similarly to Raach et al. (2017), Castillo et al. (2020) did not use real measurements and instead tested their algorithm with simulated lidar measurements in a wind tunnel experiment.
Recurring challenges in several of the aforementioned studies were the detection of the wake and the physical alignment of the lidar with the rotor axis. Both Raach et al. (2017) and Bromm et al. (2018) used the minimum power of a hypothetical downstream turbine to track the wake. Brugger et al. (2020) tracked the wake by fitting a Gaussian function for comparison to a Gaussian wake model, while Held and Mann (2019a) (discussed in Section 3.2) and Castillo et al. (2020) identified the wake based on different turbulence characteristics compared to the free stream. Further, Trujillo et al. (2011) leveraged the center-of-mass analogy. Another wake-tracking method based on image processing will be mentioned in later sections, and further methods exist in literature that have not yet been applied to NMLs. There is no consensus about which of the wake tracking methods is the best, but it stands to reason that it ultimately depends on the specific application. For example, applications concerning power production might preferably use a power-based wake tracking method, while a wake tracking based on turbulence might be more appropriate for load analysis.
The second challenge was the alignment of the lidar measurements with the rotor axis. Bromm et al. (2018) checked the alignment using hard-target detection, GPS measurements, and cross-comparison with LES. Brugger et al. (2020) used time periods when the wake steering was not active to verify that the lidar measurements were centered. The two approaches have an important difference: the former detects the alignment of the measurements with the rotor axis, the latter detects the alignment of measurements with respect to the actual wind direction. Ideally, both are known to identify possible yaw biases of the wind turbine.
In conclusion, NMLs provided important contributions to the validation of wake steering and show a potential to enhance wake steering during live operation in the future. The ability of NMLs to scan a large area of the wake quickly enables direct wake tracking, which overcomes the limitation of single-point measurements from traditional meteorological masts also in the context of wake analysis.
Models and simulations of wind turbine wakes are important tools for incorporating wake effects into wind plant planning. NML wake measurements have an important role here because they can be used to validate and calibrate of those models. Validations quantify the error between model and measurements (most commonly the RMS error), while calibrations are typically done by fitting the results of the model to the measurement data by tweaking specific free parameters.
The analytical wake model of Bastankhah and Porté-Agel (2014) has been calibrated using NMLs in several instances. Trabucchi et al. (2017) and Carbajo Fuertes et al. (2018) used horizontal cross-sections of the mean velocity deficit obtained from PPI scans to calibrate the wake growth rate, which is a constant describing the wake recovery with downstream distance. The two studies differ in the measurement setup, post-processing, and validation method. Trabucchi et al. (2017) used an offshore site in the German North Sea with inflow information from a meteorological mast, while Carbajo Fuertes et al. (2018) conducted their measurements at an on-land site in Iowa, US, with a second inflow scanning Doppler lidar. Trabucchi et al. (2017) converted the model output to radial velocities. In contrast, Carbajo Fuertes et al. (2018) converted the radial velocity to the longitudinal velocity and interpolated them on a regular grid. Lastly, Trabucchi et al. (2017) fitted the model to horizontal cross-sections of the mean velocity deficit (i.e., fitting the model to the full measurement domain at once). Thus, they prescribed an assumed linear wake growth, while Carbajo Fuertes et al. (2018) fitted individual Gaussian functions to separate downstream distances, which would allow for a non-linear wake growth. A third study by Brugger et al. (2019) used volumetric wake scans from the same experiment as Carbajo Fuertes et al. (2018) to calibrate the wake growth rate. Using volumetric measurements has the benefit of avoiding an artificially enhanced wake recovery if the plane of the PPI scan is tilted upwards or downwards. All three studies found similar relationships between the wake growth rate and the turbulence intensity below rated wind speeds (Table 4). Other studies also showed a connection between wake growth rate and ambient turbulence intensity, but did not provide a quantitative relationship (Aitken et al., 2014; Machefaux et al., 2015). Beyond the wake growth rate below rated wind speeds, Trabucchi et al. (2017) investigated the wake evolution above rated wind speeds and found a weaker dependency on the turbulence intensity compared to below rated wind speeds. Carbajo Fuertes et al. (2018) focused on the impact of turbulence intensity on the near wake length showing a non-linear increase of the near wake length with decreasing turbulence intensity. And finally, Brugger et al. (2019) found that the wake became more tilted with increasing wind veer across the rotor area and an analytical model can reproduce the wake tilt well.
TABLE 4. Summary of the reviewed scanning strategies and wind reconstruction techniques applied to NMLs for wake measurements. A zero-azimuth corresponds to the direction of the rotor axis pointing downstream.
The DWM model was also the object of a calibration and validation effort with an NML (Reinwardt et al., 2020). The measurement setup consisted of two NMLs installed on top of two utility-scale wind turbines at an onshore wind plant in northern Germany. Using a wake tracking method based on Gaussian fitting, the mean velocity deficit was computed in the NFOR and the MFOR. They observed that the mean velocity deficit in the MFOR was more pronounced than in the NFOR, and that the MFOR exhibited double-Gaussian profiles at close distances and a slower recovery for low ambient turbulence intensities. The calibration of the dynamic wake meandering model was done by fitting the quasi-steady velocity deficit to the measurements of the minimum wind speed in the MFOR and adjusting a coupling function in the eddy viscosity parametrization.
Moving away from analytical and engineering wake models, NMLs have also been used for the validation of more complex simulations of wind turbine wakes. A paper by Aitken et al. (2014) used the same data set as Aitken and Lundquist (2014) for validation of an actuator disk parametrization of wind turbines in the Weather Research and Forecasting (WRF) model that included tower and nacelle effects. Their results showed good agreement for the velocity deficit recovery and wake growth. A field experiment was conducted at the DTU Risø Campus test site by Machefaux et al. (2016b), which extended the setup of Bingöl et al. (2010) with two CW Doppler lidars on the nacelle of a second wind turbine located downstream (one scanning the inflow and one scanning the merged wake of both turbines). Data from this experiment were used to validate an LES simulation. Comparisons between the measurement data and the LES showed good agreement in terms of mean velocity deficit and wake-added turbulence.
Finally, Doubrawa et al. (2019) presented a benchmark data set for model validation that was obtained with a wake-scanning CW spinner lidar at the Scaled Wind Farm Technology (SWiFT) facility in Texas, United States. The benchmark data set consists of vertical cross-sections of the velocity deficit under neutral, unstable, and stable conditions that are complemented by an inflow characterization with a meteorological mast. Wake properties like symmetry and recovery with downstream distance are shown for the three cases. The increasing complexity of the three benchmark cases is intended to allow for the identification of shortcomings in model performance. The benchmark data set has been used in several cases in literature for comparison to LES (e.g., Doubrawa et al., 2020; Yang et al., 2020; Hsieh et al., 2021; Kale et al., 2022), to higher-order Gaussian wake models (Blondel and Cathelain, 2020), and for calibration and validation of the DWM model (Conti et al., 2021a).
One challenge in the aforementioned studies is bridging the inherent differences between measurement data and model output. The measurements of a lidar typically consist of (filtered) radial velocities on an often incomplete spherical grid. Models, on the other hand, typically provide the Cartesian velocity components on a regular grid. A common element in the studies discussed above is that either the lidar measurements are converted and interpolated to match the output of the wake model, or the wake model is extended to replicate the measurement properties of the lidar with a virtual lidar. A widespread technique to achieve the former scope is converting the mean radial velocity to the mean longitudinal velocity, assuming that the average lateral and vertical components are negligible through the WSD approach. It is noteworthy that there is generally a lack of agreement on the interpolation techniques adopted (Letizia et al., 2021a) and that the interpolation can be a significant source of error (Carbajo Fuertes et al., 2018), as discussed more profusely in Section 4.5.
Another common challenge pertains to the assumptions necessary to simplify the flow problem in engineering wake models (e.g., axisymmetric wake, neutral stratification, steady flow, see Hamilton et al. (2020)). Experimental studies aimed at validating or calibrating a model usually include extensive data filtering to make sure that the model assumptions are fulfilled as closely as possible in the measured data. However, the significant rejection rate due to the high occurrence of experimental data not matching the idealized flow conditions required by the model is itself an indicator of the intrinsic limitations of such studies. This shortcoming in the description of real-world flows is given less room in the discussion compared to more typical error metrics.
Nevertheless, NMLs are a powerful tool for model validation and calibration. The good spatial coverage of the wake compared to a meteorological mast, and the independence from specific wind direction sectors compared to ground-based lidars are the main benefits of nacelle-based lidars for this purpose.
Beyond wake steering and model validations, NMLs have been used to study the general wake structure and provide insights into the wake physics. Both the near-wake and the far-wake of wind turbines in terms of shape, position, meandering, and depth have been investigated.
We will begin this section by discussing several aspects of wake meandering that have been investigated with NMLs. One of them is the shape of the velocity deficit in the MFOR, which the DWM model assumes as stationary and symmetric. Herges et al. (2017) studied the shape of the wake by applying a detection method conceived for image processing to spanwise cross-sections of the velocity captured with a nacelle-based CW lidar. They showed that the wake became skewed with increasing wind veer and that what appeared as a flow instability occurred for unstable conditions, which resulted in irregular wake edges. Brugger et al. (2022) also noted a variability of the velocity deficit in the MFOR for some of the time periods they investigated. Contrary to the previous two studies, Machefaux et al. (2016a) found that the mean velocity deficit in the MFOR was invariant to the atmospheric stability conditions. Therefore, more investigations on this research question should be conducted.
Another aspect of wake meandering that has received attention is the downstream transport process, which initially has been assumed to be a passive advection with the mean wind speed (Larsen et al., 2008). As already mentioned in Section 4.1, Bingöl et al. (2010) observed better visual agreement between the observed wake position and the wake position predicted by the DWM model by adopting an effective advection velocity that is slower than the mean wind speed. This observation was later verified quantitatively by Machefaux et al. (2015) by determining the cross-correlation between the wake center position at different downstream distances, which they tracked from vertical cross-sections of the wake at multiple downstream distances. They found that the downstream transport velocity was approximately half of the ambient mean wind speed. They also investigated the lateral advection velocity of wake meandering and found it to be constant (The paper provides an extensive investigation into several other aspects, which are beyond the scope of this review.) Brugger et al. (2022) studied the downstream transport velocity with a measurement setup consisting of two NMLs providing horizontal cross-sections of the wake and the lateral velocity component of the inflow simultaneously. Using two different cross-correlation approaches and a large data set covering a wider range of ambient conditions compared to Machefaux et al. (2015), they found good agreement between the advection velocity and the average of the ambient mean wind speed and the velocity at the wake center. Further, they found that the correlation between the lateral component of the velocity and the wake center position decreased with downstream distance, and was proportional to the integral time scale of the lateral turbulence component normalized by the advection time. While several expressions for the downstream transport velocity of wake meandering are proposed in the literature, and there is no general agreement, there is consensus from the experiments that it is slower than the ambient mean wind speed.
The last aspect regarding wake meandering that is discussed here is its relationship to the ambient turbulence. Aitken and Lundquist (2014) showed that the wake meandering strength increased with the ambient turbulence intensity. This finding was confirmed by Brugger et al. (2022), who also showed that the added wake turbulence and recovery of the mean velocity deficit also scaled with the wake meandering strength. Both studies used PPI scans of nacelle-based lidar at an onshore, utility-scale wind turbine, but adopted different wake tracking methods.
Finally, we will mention studies that addressed particular sub-topics that are generally omitted in the aforementioned sources. For instance, although most of the mentioned applications focus on the far wake physics, NML measurements have also been used to investigate the near wake, which is defined as the region immediately downstream of the rotor (x ≲ 2 − 4D) where the wake profile is affected by the axial induction distribution imparted by the local aerodynamic forces of the blades (Porté-Agel et al., 2019). It is also the region where the sub-atmospheric pressure behind the turbine recovers to its upstream value. Due to their simplified description of the axial induction, the parabolic formulation (i.e., the streamwise pressure gradient is neglected), and the typical separation distance between adjacent turbines, engineering wake models are typically targeted at the far wake and treat the near wake as an initial condition. Trujillo et al. (2016) presented one of the few studies focusing on the near wake of a wind turbine. The measurement setup included a short-range, pulsed Doppler lidar mounted on the nacelle of an offshore wind turbine in northern Germany that scanned spanwise cross-sections of the wake at a downwind distance between 0.6D and 1.4D. By fitting single and double Gaussian functions to the mean velocity deficit, the authors tracked the wake position in the near wake. They found a correspondence between yaw misalignment and wake position with a delayed onset of the wake displacement by one rotor diameter in the downstream direction. Similar to other studies, they mentioned the difficulties in capturing the full wake at close ranges due to limitations in the opening angle of the lidar scans. The relatively scarce literature on the near wake is not only due to the complexity of the flow in that region, but also due to the difficulty of rear-facing NMLs in measuring at close ranges due to the blind region of lidars and high geometrical error. Shin and Ko (2022) attempted to overcome these limitations by measuring the near wake of a 3-MW turbine using a forward-facing fixed-beam lidar located on the nacelle of a downstream turbine. Using an a priori estimate of the wake region based on the Jensen wake model Jensen (1983) to define a wake region for averaging, they showed the characteristic double-Gaussian shape of the velocity deficit in the near wake due to the nacelle jet and a decrease of the turbulence intensity in the wake region with increasing wind speeds. Finally, they investigated the velocity deficit as a function of the downstream distance, possibly detecting the signature of the pressure recovery.
The effect of the terrain around the turbine on the wake behavior is also a challenging aspect of wake modeling that is generally disregarded. In this respect, an investigation into the interaction between topography and wind turbine wakes was conducted by Machefaux et al. (2016a), using a similar setup and post-processing as Machefaux et al. (2015). They employed cluster averaging according to the wind speed and the atmospheric stability and tracked the wake by fitting a Gaussian function. The results showed that the downstream transport of the wake followed the contours of the terrain under stable conditions, while for unstable conditions it moved on the horizontal plane.
As has been shown, NMLs can provide unprecedented insights into the wake physics. Field experiments can reveal factors influencing the wake that were not previously considered in modeling or through more idealized analytical approaches. Also, field experiments provide an opportunity to study effects that are difficult to replicate in a wind tunnel or numerical simulation.
As seen in previous sections, wake measurements with NML are used to derive quantitative information about the wake across a large spatial volume. A traditional validation of NML measurements with other in situ instrumentation, which samples only a limited volume, is therefore generally not sufficient for a comprehensive error analysis. Virtual lidars that simulate a Doppler lidar’s measurement properties and scan configurations within numerical environments (typically LES) act as an alternative means of validation. The difference between the underlying LES (regarded as the truth in this context) and the flow properties reconstructed from the virtual lidar measurements within the LES can then provide insights into the errors.
Fuertes Carbajo and Porté-Agel (2018) analyzed the errors of volumetric scan patterns with isotropic angular resolutions between 1° and 5° and a moderate opening angle in both azimuth and elevation (θmax = βmax = 16.7°) that resulted in completion times for a single volumetric scan between 10 min and less than half a minute. They used an LES of an 80-m-diameter wind turbine with a virtual lidar located on the nacelle. By analyzing the resulting errors for the mean and the standard deviation of the longitudinal velocity component due to lidar acquisition, interpolation, and statistical uncertainty, they found that the best overall error performance was achieved with an angular resolution of 3° and that the reconstruction of the standard deviation from the virtual lidar measurements is of lower quality than the mean.
The two companion papers Beck and Kühn (2019a) and Beck and Kühn (2019b) present physiscs-informed interpolation and up-sampling methods to mitigate the trade-offs between spatial and temporal resolution inherent to NML wake scanning. The first paper (Beck and Kühn, 2019b) presented a temporal up-sampling method to fill the temporal gaps between successive RHI or PPI sweeps of an NML. The up-sampling method is based on a local advection scheme that can be understood as a TFH applied to small spatial and temporal scales that are advected at a rate prescribed by the local velocity. The second paper (Beck and Kühn, 2019a) presented a reconstruction scheme for the complete three-dimensional, longitudinal velocity field of the wake from a cross-shaped scan configuration with a PPI and an RHI through the rotor axis, which can be completed in a significantly shorter time than the volumetric scans of Fuertes Carbajo and Porté-Agel (2018). The reconstruction relies heavily on the framework of the DWM model of Larsen et al. (2008) and uses linear interpolation between the two lidar planes along a curve with a constant radius to generate a volumetric velocity field (i.e., assuming a conditional rotational symmetry of the wake in the MFOR). The two methods presented in Beck and Kühn (2019b) and Beck and Kühn (2019a) were validated with a virtual lidar in an LES and showed an overall satisfying quality. However, limitations existed in the near wake and the underlying assumptions to fill the gaps in the measurements are extensive.
Letizia et al. (2021a) also leveraged three nacelle-mounted virtual lidars (an ideal one performing pointwise sampling, one operating in step-stare mode, and another scanning continuously) to quantify the error of the proposed interpolation and scan design algorithm. They found that the mean wind speed could be accurately reconstructed regardless of the lidar type, but the turbulence intensity suffered from an increasingly severe underestimation based on the amount of spatio-temporal filtering of the instrument. They also showed that the optimal scanning strategy (θmax = βmax = 10°, Δθ = Δβ = 2.5°) could be identified using a few key parameters representative of the wake flow, which represents an actionable solution in cases where high-fidelity simulations are not available.
Letizia et al. (2023) built on the previous work and defined a more general methodology for the design of multi-instrument NML campaigns. They used idealized lidar samples collected according to the technical specifications of a real lidar to evaluate several combinations of scan geometries and durations. The best scanning strategy is selected based on a triple-objective optimization aimed to maximize spatial coverage, statistical convergence, and sampling rate. It is noteworthy that they had to use a complex sequence encompassing different scans to meet the scientific goals of their experiments.
To conclude, virtual lidars have proven to be a valuable tool to carry out error analysis for NML wake measurement, albeit their use has been less systematic than what is observed for the inflow studies discussed in Section 3, likely due to the greater complexity that the simulation of a turbine wake implies compared to an undisturbed wind field.
The review of NMLs for wind energy reveals a vast and diverse panorama in terms of applications, approaches, and experimental strategies.
Regarding the applications, there is a substantial body of literature reporting significant advantages in load reduction and rotor speed stabilization, achievable by means of lidar-assisted feed-forward control. Future development of such technology at an industrial scale will depend on the future reliability, flexibility, and costs of lidars, the degree to which the benefits of LAC address challenges faced by new wind turbines, and the development of general guidelines for the calibration and implementation of LAC systems. Less clear advantages emerge in the context of yaw control; the main claimed benefit of improved power capture is dependent on the accuracy of the baseline wind direction sensors. Several authors agree on the redundancy of a lidar-based feed-forward loop for power enhancement in region 2 of a wind turbine power curve, where the state-of-the-art torque controllers have already achieved a high level of maturity, which may make additional gains unworthy.
Still, in the context of inflow detection, power performance testing is probably the industrial application where NML has had the largest success. NMLs have not only replaced more costly and rigid meteorological towers, but have also provided additional benefits in terms of data availability and correlation with turbine response. Existing techniques for the reconstruction of the mean flow from NMLs exhibit an accuracy comparable to that of traditional methods based on in situ sensors. However, lidar-based higher-order statistics (e.g., TI, Reynolds stresses, spectra) still suffer from damping, geometrical error, and contamination due to the lidar acquisition process, and complex methods are being developed to correct the retrieval to mitigate such effects. This is also why the assessment of loads through lidars still represents an active research area.
The use of NMLs for wake measurement is generally reported in the context of advanced wind energy and atmospheric science research, although some potential practical applications of NMLs for wake tracking have been proposed. Thanks to their ability to scan large volumes, NMLs have shed light on phenomena like wake meandering, recovery, shape, and their connection to atmospheric conditions. NMLs undeniably contributed to expanding the fundamental knowledge on this topic. Further, the use of NMLs for inflow and wake measurements for wind farm control applications, such as wake steering, is expected to grow in the future as the control technology becomes more widely adopted.
This review also highlighted a significant disparity in the methodologies used for inflow and wake studies. Inflow studies have employed a preponderance of numerical methods adopted to simulate and optimize the lidar system (especially for LAC). In contrast, wake measurements are mostly carried out experimentally. The difference could be due to the relatively easier generation of undisturbed atmospheric turbulence compared to a more complex wake velocity field, but also to the different backgrounds of researchers investigating inflow LAC (engineering control) and wake physics (atmospheric science).
Certainly, both virtual and real lidar observations are essential for the development of NMLs for wind energy, the former being best suited to characterize the errors and develop scanning strategies, whereas the latter gives a more realistic picture of the flow physics. More research seems to be needed to bridge the gap between these two approaches. For example, bringing more real-world knowledge into inflow studies would make the LAC more appealing to the industry, while numerical techniques could improve the uncertainty quantification and assist scan design and data processing for wake measurements.
Indeed, a great variety of experimental strategies and technologies have been utilized in the literature surveyed in this review. This complex scenario is the result of mostly three factors: first, the different quantities of interest needed for each application; second, the great scanning flexibility offered by lidars; and third, the still relative infancy of this technology. While the first two elements are desirable, the last calls for more research efforts aimed at identifying general guidelines that can assist lidar users in the planning and execution of their measurement campaigns.
The most relevant documented applications of nacelle-mounted lidars to the best of the authors’ knowledge have been reviewed. In spite of the novelty of this technology, a substantial body of scientific and technical literature has been already produced.
The different uses of NMLs have been broadly classified into inflow detection and wake measurements, the first being mainly performed to study lidar-assisted control and conduct power performance tests, while the second to investigate wake physics. The above classification and the even more granular sub-categorization into narrow research applications is meant to facilitate the understanding of the vast literature on NMLs and guide the reader interested in specific topics. Another contribution of the present work is the survey of the numerous scanning strategies and flow reconstruction techniques previously adopted which can provide guidance to future NML users. Finally, the reported advantages and current limitations of NMLs used for either engineering applications or more fundamental research have been summarized.
This review also revealed quite clearly the aspects that future research would need to address to fully exploit the potential of NML and therefore foster their use among the wind energy community. First, the reliability of the lidar/turbine system should be enhanced, as the already relatively scarce field applications seem to suffer from severe data losses due to the significant technical challenges associated with the operation of NMLs, especially for turbine control. Second, further research is necessary to quantify the cost-effectiveness of applications such as lidar-assisted control or active wake-tracking techniques with acceptable and documented uncertainty. In fact, the extremely diverse numerical and experimental approaches used to quantify the advantages of industrial applications of NMLs led so far to a great deal of different outcomes, while generalized and conclusive results are still lacking. Third, a significant effort is still needed to synthesize a standardized scanning strategy and flow reconstruction technique from the numerous experimental methods used to date. Instead of using rule-of-thumb approaches or optimizing the scan design just for niche applications, researchers should apply or alternatively propose generalized principles to design lidar experiments, similarly to what is reported in some papers on control cited in section 3 and in section 4.5 for wake analysis.
To conclude, the present work, by surveying the existing accounts of NML applications, is indeed meant to guide future researchers willing to take on the foregoing challenges with the ultimate goal of expanding our knowledge of wind plant flows and making remote sensing a major driver for the development of profitable and efficient wind plants.
SL: Conceptualization, Investigation, Project administration, Supervision, Visualization, Writing–original draft, Writing–review and editing. PB: Conceptualization, Investigation, Supervision, Visualization, Writing–original draft, Writing–review and editing. NB: Supervision, Writing–review and editing. RK: Writing–review and editing. AS: Writing–review and editing. ES: Writing–review and editing. FP-A: Funding acquisition, Writing–review and editing. NH: Funding acquisition, Writing–review and editing. PD: Funding acquisition, Writing–review and editing. PM: Funding acquisition, Writing–review and editing.
The author(s) declare financial support was received for the research, authorship, and/or publication of this article. This work was authored in part by the National Renewable Energy Laboratory, operated by Alliance for Sustainable Energy, LLC, for the U.S. Department of Energy (DOE) under Contract No. DE-AC36-08GO28308. Funding was provided by the U.S. Department of Energy Office of Energy Efficiency and Renewable Energy Wind Energy Technologies Office. The views expressed in the article do not necessarily represent the views of the DOE or the U.S. Government. The U.S. Government retains and the publisher, by accepting the article for publication, acknowledges that the U.S. Government retains a nonexclusive, paid-up, irrevocable, worldwide license to publish or reproduce the published form of this work, or allow others to do so, for U.S. Government purposes. This work was supported by the Swiss National Science Foundation (Grant Numbers: 200021_172538 and 200021_215288) and the Swiss Federal Office of Energy (Grant No. SI/502135-01). Pacific Northwest National Laboratory (PNNL) is operated by Battelle Memorial Institute for the U.S. Department of Energy under Contract DE-AC05-76RL01830.
The authors thank Daniel Houck (Sandia National Laboratories) for his valuable help in the description of the Spinner lidars and Vaisala for sharing the data reported in Figure 6. Also, a special thanks to all the members of the American WAKE ExperimeNt (AWAKEN) and the Rotor Aerodynamics Aeroelastics and Wake (RAAW) team who spurred the interest for this work.
The authors declare that the research was conducted in the absence of any commercial or financial relationships that could be construed as a potential conflict of interest.
All claims expressed in this article are solely those of the authors and do not necessarily represent those of their affiliated organizations, or those of the publisher, the editors and the reviewers. Any product that may be evaluated in this article, or claim that may be made by its manufacturer, is not guaranteed or endorsed by the publisher.
Abkar, M., and Porté-Agel, F. (2015). Influence of atmospheric stability on wind-turbine wakes: a large-eddy simulation study. Physics of Fluids 27. doi:10.1063/1.4913695
Aho, J., Pao, L., and Hauser, J. (2013). “Optimal trajectory tracking control for wind turbines during operating region transitions,” in Proceedings of the American Control Conference, Washington, DC, USA, 17-19 June 2013 (IEEE), 1424–1429.
Aitken, M. L., Kosović, B., Mirocha, J. D., and Lundquist, J. K. (2014). Large eddy simulation of wind turbine wake dynamics in the stable boundary layer using the weather research and forecasting model. J. Renew. Sustain. Energy 6, 033137. doi:10.1063/1.4885111
Aitken, M. L., and Lundquist, J. K. (2014). Utility-scale wind turbine wake characterization using nacelle-based long-range scanning lidar. J. Atmos. Ocean. Technol. 31, 1529–1539. doi:10.1175/jtech-d-13-00218.1
Angelou, N., Mann, J., Courtney, M., Sjöholm, M., and Angelou, A. N. (2010). Doppler lidar mounted on a wind turbine Risø-R-Report. Tech. rep. Denmark Technical University.
Annoni, J., Fleming, P., Scholbrock, A., Roadman, J., Dana, S., Adcock, C., et al. (2018). Analysis of control-oriented wake modeling tools using lidar field results. Wind Energy Sci. 3, 819–831. doi:10.5194/wes-3-819-2018
Barthelmie, R. J., Pryor, S. C., Frandsen, S. T., Hansen, K. S., Schepers, J. G., Rados, K., et al. (2010). Quantifying the impact of wind turbine wakes on power output at offshore wind farms. J. Atmos. Ocean. Tech. 27, 1302–1317. doi:10.1175/2010jtecha1398.1
Bastankhah, M., and Porté-Agel, F. (2014). A new analytical model for wind-turbine wakes. Renew. 70, 116–123. doi:10.1016/j.renene.2014.01.002
Bastankhah, M., and Porté-Agel, F. (2016). Experimental and theoretical study of wind turbine wakes in yawed conditions. J. Fluid Mech. 806, 506–541. doi:10.1017/jfm.2016.595
Bauweraerts, P., and Meyers, J. (2020). Reconstruction of turbulent flow fields from lidar measurements using large-eddy simulation. J. Fluid Mech. 10.1017/jfm.2020.805 906, A17. doi:10.1017/jfm.2020.805
Beck, H., and Kühn, M. (2019a). Reconstruction of three-dimensional dynamic wind-turbine wake wind fields with volumetric long-range wind Doppler lidar measurements. Remote Sens. 11 11, 2665. doi:10.3390/rs11222665
Beck, H., and Kühn, M. (2019b). Temporal up-sampling of planar long-range Doppler LiDARWind speed measurements using space-time conversion. Remote Sens. 11 11, 867. doi:10.3390/rs11070867
Bingöl, F. (2009). Complex terrain and wind lidars. DTU PhD Thesis Risø-PhD-52, Tech. Univeristy Den. DTU Vindenergi Nils Koppels Allé Bygning 403 2800 Kgs. Lyngby.
Bingöl, F., Mann, J., and Larsen, G. C. (2010). Light detection and ranging measurements of wake dynamics. Part I: one-dimensional scanning. Wind Energy 13, 51–61. doi:10.1002/we.352
Blondel, F., and Cathelain, M. (2020). An alternative form of the super-Gaussian wind turbine wake model. Wind Energy Sci. 5, 1225–1236. doi:10.5194/wes-5-1225-2020
Bodini, N., Lundquist, J. K., and Moriarty, P. (2021). Wind plants can impact long-term local atmospheric conditions. Sci. Rep. 11, 22939. doi:10.1038/s41598-021-02089-2
Bodini, N., Zardi, D., and Lundquist, J. K. (2017). Three-dimensional structure of wind turbine wakes as measured by scanning lidar. Atmos. Meas. Tech. 10, 2881–2896. doi:10.5194/amt-10-2881-2017
Boersma, S., Doekemeijer, B. M., Gebraad, P. M. O., Fleming, P. A., Annoni, J., Scholbrock, A. K., et al. (2017). “A tutorial on control-oriented modeling and control of wind farms,” in In Proc. American Control Conf, Seattle, WA, USA, 1–18.
Borraccino, A., Schlipf, D., Haizmann, F., and Wagner, R. (2017). Wind field reconstruction from nacelle-mounted lidar short-range measurements. Wind Energy Sci. 2, 269–283. doi:10.5194/wes-2-269-2017
Bos, R., Giyanani, A., and Bierbooms, W. (2016). Assessing the severity ofwind gusts with lidar. Remote Sens. 8, 758–813. doi:10.3390/rs8090758
Bossanyi, E. (2013). Un-freezing the turbulence: application to LiDAR-assisted wind turbine control. IET Renew. Power Gener. 7, 321–329. doi:10.1049/iet-rpg.2012.0260
Bossanyi, E. A., Kumar, A., and Hugues-Salas, O. (2014). Wind turbine control applications of turbine-mounted LIDAR. J. Phys. Conf. Ser. 555 555, 012011. doi:10.1088/1742-6596/555/1/012011
Bossanyi, E., Wright, A., and Fleming, P. (2010). Controller field tests on the NREL CART2 turbine. Tech. rep., National Renewable Energy Lab. (NREL), Golden, CO (United States).
Bossuyt, J., Scott, R., Ali, N., and Cal, R. B. (2021). Quantification of wake shape modulation and deflection for tilt and yaw misaligned wind turbines. J. Fluid Mech. 917, A3–28d. doi:10.1017/jfm.2021.237
Bottasso, C. L., Pizzinelli, P., Riboldi, C. E., and Tasca, L. (2014). LiDAR-enabled model predictive control of wind turbines with real-time capabilities. Renew. Energy 71, 442–452. doi:10.1016/j.renene.2014.05.041
Bromm, M., Rott, A., Beck, H., Vollmer, L., Steinfeld, G., and Kühn, M. (2018). Field investigation on the influence of yaw misalignment on the propagation of wind turbine wakes. Wind Energy 21, 1011–1028. doi:10.1002/we.2210
Brugger, P., Debnath, M., Scholbrock, A., Fleming, P., Moriarty, P., Simley, E., et al. (2020). Lidar measurements of yawed-wind-turbine wakes: characterization and validation of analytical models. Wind Energy Sci. 5, 1253–1272. doi:10.5194/wes-5-1253-2020
Brugger, P., Fuertes, F. C., Vahidzadeh, M., Markfort, C. D., and Porté-Agel, F. (2019). Characterization of wind turbine wakes with nacelle-mounted Doppler lidars and model validation in the presence of wind veer. Remote Sens. 11 11, 2247. doi:10.3390/rs11192247
Brugger, P., Markfort, C., and Porté-Agel, F. (2022). Field measurements of wake meandering at a utility-scale wind turbine with nacelle-mounted Doppler lidars. Wind Energy Sci. 7, 185–199. doi:10.5194/wes-7-185-2022
Carbajo Fuertes, F., Markfort, C. D., and Porté-Agel, F. (2018). Wind turbine wake characterization with nacelle-mounted wind lidars for analytical wake model validation. Remote Sens. 10 10, 668. doi:10.3390/rs10050668
Castillo, R., Bayne, S., Pol, S., and Westergaard, C. (2020). Wind turbine wake position detection and rotor speed-based wake steering validation in a wind tunnel wake simulator. Wind Eng. 44, 483–493. doi:10.1177/0309524x19852350
Chen, Y., Schlipf, D., and Wen Cheng, P. (2021). Parameterization of wind evolution using lidar. Wind Energy Sci. 6, 61–91. doi:10.5194/wes-6-61-2021
Clifton, A., Clive, P., Gottschall, J., Schlipf, D., Simley, E., Simmons, L., et al. (2018). IEA wind task 32: wind lidar identifying and mitigating barriers to the adoption of wind lidar. Remote Sens.-Basel 10, 406–422. doi:10.3390/rs10030406
Conti, D., Dimitrov, N., and Peña, A. (2020). Aeroelastic load validation in wake conditions using nacelle-mounted lidar measurements. Wind Energy Sci. 5, 1129–1154. doi:10.5194/wes-5-1129-2020
Conti, D., Dimitrov, N., Peña, A., and Herges, T. (2021a). Probabilistic estimation of the dynamic wake meandering model parameters using spinnerlidar-derived wake characteristics. Wind Energy Sci. 6, 1117–1142. doi:10.5194/wes-6-1117-2021
Conti, D., Pettas, V., Dimitrov, N., and Peña, A. (2021b). Wind turbine load validation in wakes using wind field reconstruction techniques and nacelle lidar wind retrievals. Wind Energy Sci. 6, 841–866. doi:10.5194/wes-6-841-2021
Dahlberg, J., and Medici, D. (2003). Potential improvement of wind turbine array efficiency by active wake control. In Proc. European Wind Energy Conference, Madrid, Spain.
Davoust, S., Jehu, A., Bouillet, M., Bardon, M., Vercherin, B., Scholbrock, A. K., et al. (2014). Assessment and optimization of lidar measurement availability for wind turbine control. Tech. Rep. May, national renewable energy laboratory
Davoust, S., and von Terzi, D. (2016). Analysis of wind coherence in the longitudinal direction using turbine mounted lidar. J. Phys. Conf. Ser. 753, 072005. doi:10.1088/1742-6596/753/7/072005
Dimitrov, N. (2019). Surrogate models for parameterized representation of wake-induced loads in wind farms. Wind Energy 22, 1371–1389. doi:10.1002/we.2362
Dimitrov, N., and Natarajan, A. (2017). Application of simulated lidar scanning patterns to constrained Gaussian turbulence fields for load validation. Wind Energy 20, 79–95. doi:10.1002/we.1992
Doppler, C. (1842). On the coloured light of the binary stars and some other stars of the heavens. Proc. R. Bohemian Soc. Sci. Prague (Part V) 465, 482.
Doubrawa, P., Debnath, M., Moriarty, P. J., Branlard, E., Herges, T. G., Maniaci, D. C., et al. (2019). Benchmarks for model validation based on LiDAR wake measurements. J. Phys. Conf. Ser. 1256, 012024. doi:10.1088/1742-6596/1256/1/012024
Doubrawa, P., Quon, E. W., Martinez-Tossas, L. A., Shaler, K., Debnath, M., Hamilton, N., et al. (2020). Multimodel validation of single wakes in neutral and stratified atmospheric conditions. Wind Energy 23, 2027–2055. doi:10.1002/we.2543
Dunne, F., Pao, L., Wright, A., Jonkman, B., Kelley, N., and Simley, E. (2011). Adding feedforward blade pitch control for load mitigation in wind turbines: non-causal series expansion, preview control, and optimized fir filter methods. 49th AIAA Aerospace Sciences Meeting including the New Horizons Forum and Aerospace Exposition. doi:10.2514/6.2011-819
Dunne, F., and Pao, L. Y. (2013). Benefit of wind turbine preview control as a function of measurement coherence and preview time. Proc. Am. Control Conf. 647–652d. doi:10.1109/acc.2013.6579910
Dunne, F., and Pao, L. Y. (2016). Optimal blade pitch control with realistic preview wind measurements. Wind Energy 19, 2153–2169. doi:10.1002/we.1973
Dunne, F., Pao, L. Y., Schlipf, D., and Scholbrock, A. K. (2014). Importance of lidar measurement timing accuracy for wind turbine control. Proc. Am. Control Conf., 3716–3721. doi:10.1109/ACC.2014.6859337
Dunne, F., Schlip, D., Pao, L. Y., Wright, A. D., Jonkman, B., Kelley, N., et al. (2012). Comparison of two independent lidar-based pitch control designs. Tech. Rep. August, Natl. Renew. Energy Lab. doi:10.2514/6.2012-1151
El-Asha, S., Zhan, L., and Iungo, G. V. (2017). Quantification of power losses due to wind turbine wake interactions through SCADA, meteorological and wind lidar data. Wind Energy 20, 1823–1839. doi:10.1002/we.2123
Emeis, S., Harris, M., and Banta, R. M. (2007). Boundary-layer anemometry by optical remote sensing for wind energy applications. Meteorol. Z. 16, 337–347. doi:10.1127/0941-2948/2007/0225
Fleming, P. A., Scholbrock, A. K., Jehu, A., Davoust, S., Osler, E., Wright, A. D., et al. (2014). Field-test results using a nacelle-mounted lidar for improving wind turbine power capture by reducing yaw misalignment. J. Phys. Conf. Ser. 524 524, 012002. doi:10.1088/1742-6596/524/1/012002
Forster, P. M., Maycock, A. C., McKenna, C. M., and Smith, C. J. (2020). Latest climate models confirm need for urgent mitigation. Nat. Clim. Change 10, 7–10. doi:10.1038/s41558-019-0660-0
Foti, D., Yang, X., Campagnolo, F., Maniaci, D., and Sotiropoulos, F. (2018). Wake meandering of a model wind turbine operating in two different regimes. Physical Review Fluids 3. doi:10.1103/PhysRevFluids.3.054607
Fu, W., Peña, A., and Mann, J. (2021). Turbulence statistics from three different nacelle lidars. Wind Energy Sci. 7, 831–848. doi:10.5194/wes-7-831-2022
Fuertes Carbajo, F., and Porté-Agel, F. (2018). Using a virtual lidar approach to assess the accuracy of the volumetric reconstruction of a wind turbine wake. Remote Sens.-Basel 10, 721–820. doi:10.3390/rs10050721
Gottschall, J., Courtney, M. S., Wagner, R., Jørgensen, H. E., and Antoniou, I. (2012). Lidar profilers in the context of wind energy—a verification procedure for traceable measurements. Wind Energy 15, 147–159. doi:10.1002/we.518
Haizmann, F., Schlipf, D., Raach, S., Scholbrock, A., Wright, A., Slinger, C., et al. (2015). Optimization of a feed-forward controller using a CW-lidar system on the CART3. Proc. Am. Control Conf. 2015-July, 3715–3720. doi:10.1109/ACC.2015.7171907
Hamilton, N., Bay, C. J., Fleming, P., King, J., and Martínez-Tossas, L. A. (2020). Comparison of modular analytical wake models to the Lillgrund wind plant. J. Renew. Sustain. 12, 053311. doi:10.1063/5.0018695
Hardesty, R. M., and Weber, B. F. (1987). Lidar measurement of turbulence encountered by horizontal-axis wind turbines. J. Atmos. Ocean. Tech. 4, 191–203. doi:10.1175/1520-0426(1987)004<0191:lmoteb>2.0.co;2-0191:LMOTEB-2.0.CO;2
Harris, M., Bryce, D. J., Coffey, A. S., Smith, D. A., Birkemeyer, J., and Knopf, U. (2007). Advance measurement of gusts by laser anemometry. J. Wind Eng. Industrial Aerodynamics 95, 1637–1647. doi:10.1016/j.jweia.2007.02.029
Harris, M., Hand, M., and Wright, A. D. (2006). Lidar for turbine control. Tech. Rep. NREL/TP-500–39154.
Heisel, M., Hong, J., and Guala, M. (2018). The spectral signature of wind turbine wake meandering: a wind tunnel and field-scale study. Wind Energy 21, 715–731. doi:10.1002/we.2189
Held, D. P., and Mann, J. (2019a). Detection of wakes in the inflow of turbines using nacelle lidars. Wind Energy Sci. 4, 407–420. doi:10.5194/wes-4-407-2019
Held, D. P., and Mann, J. (2019b). Lidar estimation of rotor-effective wind speed - an experimental comparison. Wind Energy Sci. 4, 421–438. doi:10.5194/wes-4-421-2019
Herges, T. G., Maniaci, D. C., Naughton, B. T., Mikkelsen, T., and Sjöholm, M. (2017). High resolution wind turbine wake measurements with a scanning lidar. J. Phys. Conf. Ser. 854 854, 012021. doi:10.1088/1742-6596/854/1/012021
Hsieh, A. S., Brown, deVelder, K. A., Herges, T. G., Knaus, R. C., Sakievich, P. J., et al. (2021). High-fidelity wind farm simulation methodology with experimental validation. J. Wind Eng. Industrial Aerodynamics 218, 104754. doi:10.1016/j.jweia.2021.104754
IEA (2021). Wind power. Available at: https://www.iea.org/reports/wind-power.(Accessed: 2022-08-01).
IEC (2022). Iec 61400-50-3. Tech. rep. Geneva, Switzerland: International Electrotechnical Commision.
IEC (2017). Wind energy generation systems – Part 12-1: power performance measurements of electricity producing wind turbines. Geneva, Switzerland: International Standard 61400-12-2, International Electrotechnical Commission (IEC).
IEC (2013). Wind turbine generator systems - Part 12-2: power performance of electricity-producing wind turbines based on nacelle anemometry. Geneva, Switzerland: International Standard 61400-12-2, International Electrotechnical Commission (IEC).
IEC (2005). Wind turbines part 1: design requirements. International Standard 61400–1. Geneva, Switzerland: International Electrotechnical Commission (IEC).
Iungo, G. V., and Porté-Agel, F. (2014). Volumetric lidar scanning of wind turbine wakes under convective and neutral atmospheric stability regimes. J. Atmos. Ocean. Tech. 31, 2035–2048. doi:10.1175/jtech-d-13-00252.1
Iungo, G. V., Wu, Y., and Porté-Agel, F. (2013). Field measurements of wind turbine wakes with lidars. J. Atmos. Ocean. Tech. 30, 274–287. doi:10.1175/jtech-d-12-00051.1
Jacquet, C., Apgar, D., Chauchan, V., Storey, R., Kern, S., and Davoust, S. (2022). Farm blockage model validation using pre and post construction LiDAR measurements. J. Phys. Conf. Ser. 2265, 022009. doi:10.1088/1742-6596/2265/2/022009
Jensen, N. O. (1983). A note on wind generator interaction. Tech. rep. Riso National Laboratory Roskilde. Riso-M-2411
Johnson, K., and Fritsch, G. (2012). Assessment of extremum seeking control for wind farm energy production. Wind Eng. 36, 701–715. doi:10.1260/0309-524x.36.6.701
Jonkman, B. J. (2006). TurbSim user’s guide. Tech. rep. Golden, CO (United States): National Renewable Energy Lab. (NREL).
Jonkman, J. M., and Buhl, M. L. (2005). FAST user’s guide, Vol. 365. USA: National renewable energy laboratory golden, CO.
Kale, B., Buckingham, S., van Beeck, J., and Cuerva-Tejero, A. (2022). Implementation of a generalized actuator disk model into wrf v4.3: a validation study for a real-scale wind turbine. Renew. Energy 197, 810–827. doi:10.1016/j.renene.2022.07.119
Kragh, K. A., Hansen, M. H., and Mikkelsen, T. (2013). Precision and shortcomings of yaw error estimation using spinner-based light detection and ranging. Wind Energy 16, 353–366. doi:10.1002/we.1492
Krishnamurthy, R., Choukulkar, A., Calhoun, R., Fine, J., Oliver, A., and Barr, K. S. (2013). Coherent Doppler lidar for wind farm characterization. Wind Energy 16, 189–206. doi:10.1002/we.539
Kumar, A., Bossanyi, E., Scholbrock, A., Fleming, P., Boquet, M., and Krishnamurthy, R. (2015). Field testing of LIDAR assisted feedforward control algorithms for improved speed control and fatigue load reduction on a 600 kW wind turbine. In Proc. European Wind Energy Association (EWEA) Annual, Paris, France.
Kumer, V. M., Reudera, J., Svardalc, B., Sætrec, C., and Eecen, P. (2015). Characterisation of single wind turbine wakes with static and scanning WINTWEX-W lidar data. Energy Proced. 80, 245–254. doi:10.1016/j.egypro.2015.11.428
Laks, J., Pao, L., Wright, A., Kelley, N., and Jonkman, B. (2011). The use of preview wind measurements for blade pitch control. Mechatronics 21, 668–681. doi:10.1016/j.mechatronics.2011.02.003
Laks, J., Simley, E., and Pao, L. (2013). A spectral model for evaluating the effect of wind evolution on wind turbine preview control. Proc. Am. Control Conf., 3673–3679. doi:10.1109/acc.2013.6580400
Larsen, G. C., Madsen, H., Thomsen, K., and Larsen, T. J. (2008). Wake meandering: a pragmatic approach. Wind Energy 11, 377–395. doi:10.1002/we.267
Lee, J. C. Y., and Fields, M. J. (2021). An overview of wind-energy-production prediction bias, losses, and uncertainties. Wind Energy Sci. 6, 311–365. doi:10.5194/wes-6-311-2021
Letizia, S., Bodini, N., Brugger, P., Scholbrock, A., Hamilton, N., Porté-Agel, F., et al. (2023). Holistic scan optimization of nacelle-mounted lidars for inflow and wake characterization at the RAAW and AWAKEN field campaigns. J. Phys. Conf. Ser. 2505, 012048. doi:10.1088/1742-6596/2505/1/012048
Letizia, S., Moss, C., Puccioni, M. J., Apgar, D., Iungo, G. V., and Valerio Iungo, G. (2022). Effects of the thrust force induced by wind turbine rotors on the incoming wind field: a wind LiDAR experiment. J. Phys. Conf. Ser. 2265, 022033. doi:10.1088/1742-6596/2265/2/022033
Letizia, S., Zhan, L., and Valerio Iungo, G. (2021a). LiSBOA (LiDAR Statistical Barnes Objective Analysis) for optimal design of lidar scans and retrieval of wind statistics – Part 1: theoretical framework. Atmos. Meas. Tech. 14, 2065–2093. doi:10.5194/amt-14-2065-2021
Letizia, S., Zhan, L., and Valerio Iungo, G. (2021b). LiSBOA (LiDAR Statistical Barnes Objective Analysis) for optimal design of lidar scans and retrieval of wind statistics – Part 2: applications to lidar measurements of wind turbine wakes. Atmos. Meas. Tech. 14, 2095–2113. doi:10.5194/amt-14-2095-2021
Liu, Z., Barlow, J. F., Chan, P. W., Fung, J. C. H., Li, Y., Ren, C., et al. (2019). A review of progress and applications of pulsed Doppler wind LiDARs. Remote Sens.-Basel 11, 2522–2547. doi:10.3390/rs11212522
Machefaux, E., Larsen, G. C., Koblitz, T., Troldborg, N., Kelly, M. C., Chougule, A., et al. (2016a). An experimental and numerical study of the atmospheric stability impact on wind turbine wakes. Wind Energy 19, 1785–1805. doi:10.1002/we.1950
Machefaux, E., Larsen, G. C., Troldborg, N., Gaunaa, M., and Rettenmeier, A. (2015). Empirical modeling of single-wake advection and expansion using full-scale pulsed lidar-based measurements. Wind Energy 18, 2085–2103. doi:10.1002/we.1805
Machefaux, E., Larsen, G. C., Troldborg, N., Hansen, K. S., Angelou, N., Mikkelsen, T., et al. (2016b). Investigation of wake interaction using full-scale lidar measurements and large eddy simulation. Wind Energy 19, 1535–1551. doi:10.1002/we.1936
Mann, J. (1994). The spatial structure of neutral atmospheric surface-layer turbulence. J. Fluid Mech. 273, 141–168. doi:10.1017/s0022112094001886
Marvel, K., Kravitz, B., and Caldeira, K. (2013). Geophysical limits to global wind power. Nat. Clim. Change 3, 118–121. doi:10.1038/nclimate1683
Meyers, J., Bottasso, C., Dykes, K., Fleming, P., Gebraad, P., Giebel, G., et al. (2022). Wind farm flow control: prospects and challenges. Wind Energy Sci. 7, 2271–2306. doi:10.5194/wes-7-2271-2022
Mikkelsen, T., Angelou, N., Hansen, K., Sjöholm, M., Harris, M., Slinger, C., et al. (2013). A spinner-integrated wind lidar for enhanced wind turbine control. Wind Energy 16, 625–643. doi:10.1002/we.1564
Newsom, R. K., and Banta, R. M. (2004). Assimilating coherent Doppler lidar measurements into a model of the atmospheric boundary layer. Part I: algorithm development and sensitivity to measurement error. J. Atmos. Ocean. Tech. 21, 1328–1345. doi:10.1175/1520-0426(2004)021<1328:acdlmi>2.0.co;2-1328:ACDLMI-2.0.CO;2
Newsom, R., Larry, K., William, J., and Fischer, M. (2013). Turbine-scale wind field measurements using dual-Doppler lidar. Wind Energy 18, 219–235. doi:10.1002/we.1691
Optis, M., Bodini, N., Debnath, M., and Doubrawa, P. (2021). New methods to improve the vertical extrapolation of near-surface offshore wind speeds. Wind Energy Sci. 6, 935–948. doi:10.5194/wes-6-935-2021
Pao, L. Y., and Johnson, K. E. (2011). Control of wind turbines. IEEE Control Syst. Mag. 31, 44–62. doi:10.1109/MCS.2010.939962
Penã, A., Mann, J., and Dimitrov, N. (2017). Turbulence characterization from a forward-looking nacelle lidar. Wind Energy Sci. 2, 133–152. doi:10.5194/wes-2-133-2017
Pettas, V., García, F. C., Kretschmer, M., Rinker, J. M., Clifton, A., and Cheng, P. W. (2020). A numerical framework for constraining synthetic wind fields with lidar measurements for improved load simulations. AIAA Scitech 2020 Forum 1 PartF. doi:10.2514/6.2020-0993
Porté-Agel, F., Bastankhah, M., and Shamsoddin, S. (2019). Wind-turbine and wind-farm flows: a review. Bound.-Lay. Meteorol. 174, 1–59. doi:10.1007/s10546-019-00473-0
Pryor, S. C., Barthelmie, R. J., and Shepherd, T. J. (2020). 20% of US electricity from wind will have limited impacts on system efficiency and regional climate. Sci. Rep. 10, 541–614. doi:10.1038/s41598-019-57371-1
Raach, S., Schlipf, D., and Cheng, P. W. (2017). Lidar-based wake tracking for closed-loop wind farm control. Wind Energy Sci. 2, 257–267. doi:10.5194/wes-2-257-2017
Raach, S., Schlipf, D., Haizmann, F., and Cheng, P. W. (2014). Three dimensional dynamic model based wind field reconstruction from lidar data. J. Phys. Conf. Ser. 524 524, 012005. doi:10.1088/1742-6596/524/1/012005
Reinwardt, I., Schilling, L., Dalhoff, P., Steudel, D., and Breuer, M. (2020). Dynamic wake meandering model calibration using nacelle-mounted lidar systems. Wind Energy Sci. 5, 775–792. doi:10.5194/wes-5-775-2020
Reitebuch, O. (2012). Wind lidar for atmospheric research. in Atmospheric physics (Springer), 487–507.
Rye, B., and Hardesty, M. (1993). Discrete spectral peak estimation in incoherent backscatter heterodyne lidar. I: spectral accumulation and the cramer-rao lower bound. IEEE Trans. Geosci. Remote Sens. 31, 16–27. doi:10.1109/36.210440
Sathe, A., Mann, J., Gottschall, J., and Courtney, M. S. (2011). Can wind lidars measure turbulence?. J. Atmos. Ocean. Tech. 28, 853–868. doi:10.1175/jtech-d-10-05004.1
Sathe, A., Mann, J., Vasiljevic, N., and Lea, G. (2015). A six-beam method to measure turbulence statistics using ground-based wind lidars. Atmos. Meas. Tech. 8, 729–740. doi:10.5194/amt-8-729-2015
Schlipf, D., Cheng, P. W., and Mann, J. (2013a). Model of the correlation between lidar systems and wind turbines for lidar-assisted control. J. Atmos. Ocean. Technol. 30, 2233–2240. doi:10.1175/jtech-d-13-00077.1
Schlipf, D., Fleming, P., Haizmann, F., Scholbrock, A., Hofsäß, M., Wright, A., et al. (2012). Field testing of feedforward collective pitch control on the CART2 using a nacelle-based lidar scanner. J. Phys. Conf. Ser. 555, 012090–12113. doi:10.1088/1742-6596/555/1/012090
Schlipf, D., Fleming, P., Kapp, S., Scholbrock, A., Haizmann, F., Belen, F., et al. (2013b). “Direct Speed Control using LIDAR and turbine data,” in Proceedings of the American Control Conference, Washington, DC, USA, 17-19 June 2013 (IEEE), 2208–2213.
Schlipf, D., Guo, F., and Raach, S. (2020). Lidar-based estimation of turbulence intensity for controller scheduling. J. Phys. Conf. Ser. 1618, 032053. doi:10.1088/1742-6596/1618/3/032053
Schlipf, D., Haizmann, F., Cosack, N., Siebers, T., and Cheng, P. W. (2015). Detection of wind evolution and lidar trajectory optimization for lidar-Assisted wind turbine control. Meteorol. Z. 24, 565–579. doi:10.1127/metz/2015/0634
Schlipf, D., Kapp, S., Anger, J., Bischoff, O., Hofsäß, M., Rettenmeier, A., et al. (2011). Prospects of optimization of energy production by LIDAR assisted control of wind turbines. EWEA 2011 Conf. Proc., 1–10.
Schlipf, D., and Raach, S. (2016). Turbulent extreme event simulations for lidar-assisted wind turbine control. J. Phys. Conf. Ser. 753 753, 052011. doi:10.1088/1742-6596/753/5/052011
Schlipf, D., Schlipf, D. J., and Kühn, M. (2013c). Nonlinear model predictive control of wind turbines using LIDAR. Wind Energy 16, 1107–1129. doi:10.1002/we.1533
Schlipf, D., Schuler, S., Grau, P., and Martin, K. (2010a). Look-ahead cyclic pitch control using LIDAR. Proc. Sci. Mak. Torque Wind.
Schlipf, D., Trabucchi, D., Bischoff, O., Hofsäß, M., Mann, J., Mikkelsen, T., et al. (2010b). Testing of frozen turbulence hypothesis for wind turbine applications with a scanning LIDAR system. Detaled Program ISARS. 12, 5410.
Scholbrock, A., Fleming, P., Fingersh, L., Wright, A., Schlipf, D., Haizmann, F., et al. (2013). Field testing LIDAR-based feed-forward controls on the NREL controls advanced research turbine. 51st AIAA Aerospace Sciences Meeting including the New Horizons Forum and Aerospace Exposition.
Scholbrock, A., Fleming, P., Schlipf, D., Wright, A., Johnson, K., and Wang, N. (2016). Lidar-enhanced wind turbine control: past, present, and future. Proc. Am. Control Conf. 2016, 7525113. doi:10.1109/ACC
Scholbrock, A., Flemingy, P., Wright, A., Slinger, C., Medley, J., and Harris, M. (2015). Field test results from lidar measured yaw control for improved yaw alignment with the NREL controls advanced research turbine. 33rd Wind Energy Symposium. doi:10.2514/6.2015-1209
Sharma, P. K., Gautam, A., Warudkar, V., Ahmed, S., and Bhagoria, J. L. (2021). Analysis of wind characteristics parameters with the application of lidar and mast. Wind Energy 24, 413–427. doi:10.1002/we.2580
Shin, D., and Ko, K. (2019). Application of the nacelle transfer function by a nacelle-mounted light detection and ranging system to wind turbine power performance measurement. Energies 12 12, 1087. doi:10.3390/en12061087
Shin, D., and Ko, K. (2022). Experimental study on application of nacelle-mounted lidar for analyzing wind turbine wake effects by distance. Energy 243, 123088. doi:10.1016/j.energy.2021.123088
Shin, D., Ko, K., Kang, M., Ryu, D., Kang, M., and Kim, H. (2019). Comparison of wind turbine power curves using cup anemometer and pulsed Doppler light detection and ranging systems. J. Mech. Sci. Technol. 33, 1663–1671. doi:10.1007/s12206-019-0318-x
Simley, E., Fleming, P., Girard, N., Alloin, L., Godefroy, E., and Duc, T. (2021a). Results from a wake-steering experiment at a commercial wind plant: investigating the wind speed dependence of wake-steering performance. Wind Energy Sci. 6, 1427–1453. doi:10.5194/wes-6-1427-2021
Simley, E., Fleming, P., King, J., and Sinner, M. (2021b). “Wake steering wind farm control with preview wind direction information,” in In Proc. American Control Conf, New Orleans, LA, USA, 1783–1789.
Simley, E., Fürst, H., Haizmann, F., and Schlipf, D. (2018). Optimizing lidars for wind turbine control applications-Results from the IEA Wind Task 32 workshop. Remote Sens. 10, 863–932. doi:10.3390/rs10060863
Simley, E., and Pao, L. Y. (2015). A longitudinal spatial coherence model for wind evolution based on large-eddy simulation. Proc. Am. Control Conf. 3708–3714. doi:10.1109/ACC.2015.7171906
Simley, E., Pao, L. Y., Frehlich, R., Jonkman, B., and Kelley, N. (2014a). Analysis of light detection and ranging wind speed measurements for wind turbine control. Wind Energy 17, 413–433. doi:10.1002/we.1584
Simley, E., Pao, L. Y., Frehlich, R., Jonkman, B., and Kelley, N. (2011). “Analysis of wind speed measurements using continuous wave LIDAR for wind turbine control,” in 49th AIAA Aerospace Sciences Meeting Including the New Horizons Forum and Aerospace Exposition, Orlando, Florida, 4 - 7 January 2011.
Simley, E., Pao, L. Y., Gebraad, P., and Churchfield, M. (2014b). Investigation of the impact of the upstream induction zone on LIDAR measurement accuracy for wind turbine control applications using large-Eddy simulation. J. Phys. Conf. Ser. 524 524, 012003. doi:10.1088/1742-6596/524/1/012003
Simley, E., Pao, L. Y., Kelley, N., Jonkman, B., and Frehlich, R. (2012). “LIDAR wind speed measurements of evolving wind fields,” in 50th AIAA Aerospace Sciences Meeting Including the New Horizons Forum and Aerospace Exposition.
Slinger, C., Leak, M., Pitter, M., and Harris, M. (2013). Relative power curve measurements using turbine mounted, continuous-wave lidar. European Wind Energy Conference and Exhibition, EWEC. 2013 2, 1336–1344.
Taylor, G. (1938). The spectrum of turbulence. Proceedings of the royal society of London A: mathematical, physical and engineering sci-G. I. Taylor, “the spectrum of turbulence,” proceedings of the royal society of London A: mathematical,. Phys. Eng. Sci. 164, 476–490. doi:10.1098/rspa.1938.0032
Towers, P., and Jones, B. L. (2014). Real-time wind field reconstruction from LiDAR measurements using a dynamic wind model and state estimation. Wind Energy 19, 133–150. doi:10.1002/we.1824
Trabucchi, D., Trujillo, J.-J., and Kühn, M. (2017). Nacelle-based lidar measurements for the calibration of a wake model at different offshore operating conditions. Energy Procedia 137, 77–88. doi:10.1016/j.egypro.2017.10.335
Trujillo, J.-J., Bingöl, F., Larsen, G. C., Mann, J., and Kühn, M. (2011). Light detection and ranging measurements of wake dynamics. part ii: two-dimensional scanning. Wind Energy 14, 61–75. doi:10.1002/we.402
Trujillo, J. J., Seifert, J. K., Würth, I., Schlipf, D., and Kühn, M. (2016). Full-field assessment of wind turbine near-wake deviation in relation to yaw misalignment. Wind Energy Sci. 1, 41–53. doi:10.5194/wes-1-41-2016
Vaisala (2023). Estimation of power performance testing using nacelle-mounted lidars: 2020-2030 data. Personal communication.
Veers, P., Dykes, K., Lantz, E., Barth, S., Bottasso, C. L., Carlson, O., et al. (2019). Grand challenges in the science of wind energy. Science 366, eaau2027–9. doi:10.1126/science.aau2027
Wagner, R., Courtney, M. S., Pedersen, T. F., and Davoust, S. (2016). Uncertainty of power curve measurement with a two-beam nacelle-mounted lidar. Wind Energy 19, 1269–1287. doi:10.1002/we.1897
Wagner, R., Pedersen, T. F., Courtney, M., Antoniou, I., Davoust, S., and Rivera, R. L. (2014). Power curve measurement with a nacelle mounted lidar. Wind Energy 17, 1441–1453. doi:10.1002/we.1643
Wang, N., Johnson, K. E., and Wright, A. D. (2013). “Comparison of strategies for enhancing energy capture and reducing loads using LIDAR and feedforward,” in IEEE Transactions on Control Systems Technology (IEEE), 1129–1142.Vol. 21.
Wortmann, S., Geisler, J., and Konigorski, U. (2016). Lidar-assisted feedforward individual pitch control to compensate wind shear and yawed inflow. J. Phys. Conf. Ser. 753 753, 052014. doi:10.1088/1742-6596/753/5/052014
Wu, S., and Archer, C. L. (2021). Near-ground effects of wind turbines: observations and physical mechanisms. Mon. Weather Rev. 149, 879–898. doi:10.1175/mwr-d-20-0186.1
Xia, Q., Lin, C. L., Calhoun, R., and Newsom, R. K. (2008). Retrieval of urban boundary layer structures from Doppler lidar data. Part I: accuracy assessment. J. Atmos. Sci. 65, 3–20. doi:10.1175/2007jas2328.1
Yang, X., Foti, D., Kelley, C., Maniaci, D., and Sotiropoulos, F. (2020). Wake statistics of different-scale wind turbines under turbulent boundary layer inflow. Energies 13 13, 3004. doi:10.3390/en13113004
Zhan, L., Letizia, S., and Iungo, G. V. (2019). LiDAR measurements for an onshore wind farm: wake variability for different incoming wind speeds and atmospheric stability regimes. Wind Energy 23, 1–27. doi:10.1002/we.2430
Keywords: nacelle-mounted lidars, wind energy, feed-forward control, wind turbine wake, power performance test
Citation: Letizia S, Brugger P, Bodini N, Krishnamurthy R, Scholbrock A, Simley E, Porté-Agel F, Hamilton N, Doubrawa P and Moriarty P (2023) Characterization of wind turbine flow through nacelle-mounted lidars: a review. Front. Mech. Eng 9:1261017. doi: 10.3389/fmech.2023.1261017
Received: 18 July 2023; Accepted: 10 October 2023;
Published: 02 November 2023.
Edited by:
Florent Ravelet, Arts et Metiers Institute of Technology, FranceReviewed by:
Agnimitra Biswas, National Institute of Technology, Silchar, IndiaCopyright © 2023 Letizia, Brugger, Bodini, Krishnamurthy, Scholbrock, Simley, Porté-Agel, Hamilton, Doubrawa and Moriarty. This is an open-access article distributed under the terms of the Creative Commons Attribution License (CC BY). The use, distribution or reproduction in other forums is permitted, provided the original author(s) and the copyright owner(s) are credited and that the original publication in this journal is cited, in accordance with accepted academic practice. No use, distribution or reproduction is permitted which does not comply with these terms.
*Correspondence: Stefano Letizia, c3RlZmFuby5sZXRpemlhQG5yZWwuZ292
Disclaimer: All claims expressed in this article are solely those of the authors and do not necessarily represent those of their affiliated organizations, or those of the publisher, the editors and the reviewers. Any product that may be evaluated in this article or claim that may be made by its manufacturer is not guaranteed or endorsed by the publisher.
Research integrity at Frontiers
Learn more about the work of our research integrity team to safeguard the quality of each article we publish.