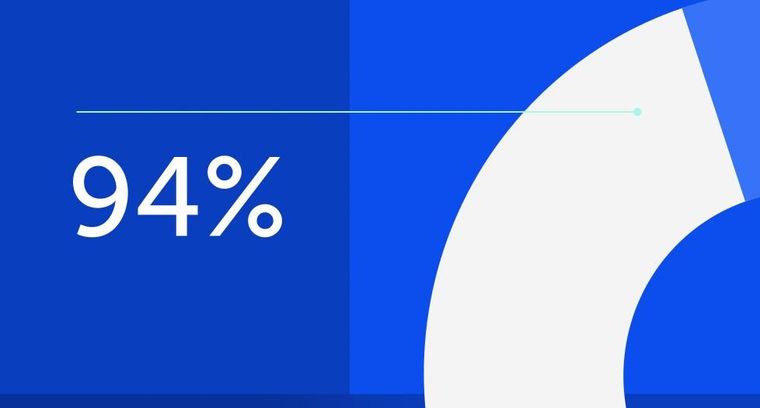
94% of researchers rate our articles as excellent or good
Learn more about the work of our research integrity team to safeguard the quality of each article we publish.
Find out more
REVIEW article
Front. Mech. Eng., 09 August 2022
Sec. Vibration Systems
Volume 8 - 2022 | https://doi.org/10.3389/fmech.2022.974464
The interior soundscape of a vehicle is an essential asset for experienced comfort and feedback of a car’s driver, especially in the premium automotive industry. Here we offer a literature review on the perception of acoustic characteristics of electrified vehicles (EVs) and the impressions and associations they convey to the individual—the driver, the customer, the user. The reduction of the overall sound pressure level (SPL) in EVs offers the opportunity to create exceptional quiet interior soundscapes. At the same time, the reduced SPL challenges NVH (Noise, Vibration, and Harshness) engineers to reduce remaining noises that are no longer masked by operational combustion while creating vehicle-adequate acoustics with pleasingly comfort-assets and operational feedback of the current driving mode. The analyzed body of literature covers research from the 21st century (2000–2022). We aim to comprise the current state of research highlighting specific achievements already made. Furthermore, we show evident gaps that need to be filled and considered in future research.
Concomitant with the technological shift to electrified powertrains is a change in the acoustic profile of vehicles which offers new opportunities but also brings new challenges to engineers in the automotive industries (Cerrato, 2009; Genuit, 2010; Meek et al., 2012; Allman-Ward et al., 2020; Gavric, 2020). Among these opportunities are novel configurations of active driving sound for electrified vehicles (EVs) (Bodden & Belschner, 2016; Cerrato, 2009; Fiebig, 2012; Genuit & Fiebig, 2011, 2014) or even the innovative shaping of our acoustic environment (Clendinning, 2018). Acoustic characteristics of vehicles are commonly subsumed under the umbrella term NVH (Noise, Vibration, and Harshness), and they are well known to play a crucial role in customer satisfaction (Qatu et al., 2009; Qatu, 2012; Zeller, 2018; Sinambari & Sentpali, 2020). For instance, the sensation of driving in all-electric mode can be described as quiet, smooth, and silent (Kurani et al., 2008) and Gärling (2001) found 80% of their sample reporting experiencing the same amount or even more pleasure driving an EV compared to driving an ICEV (Internal combustion engine vehicle), which was reportedly defined, among other things, by comfort and noiselessness. On the contrary, the analysis of social media and online forums by Krishna (2021) shows that consumers experience cars and their NVH characteristics as “soulful” (p. 5) entities, that they build emotional connections with their vehicles, especially through its sound, which was one of the most significant aspects in the analyzed data. Especially so-called petrolheads – a group of passionate car enthusiasts – seem to apprehend electrified driving as “sterile” (p. 5) and a “lackluster ‘appliance-like’ experience” (p. 6) (Krishna, 2021). As the demand for overall ride comfort, a property subjectively experienced through physical variables, increased over the years, the NVH attributes of ambient factors such as noise and dynamic factors such as vibrations play a crucial role in vehicle safety, performance, comfort, and brand image (Ormuz & Muftic, 2004). In terms of EV-NVH three main challenges are the frequently reported: 1) the EV’s silent nature leads to a lack of masking effects and new disturbing noise sources while impairing the dynamic character of the vehicle as they fall short of the accustomed operational feedback; 2) the novel NVH profile is still relatively unfamiliar to most people and especially high-frequent noise components might be perceived as very annoying; 3) the question of a suitable sound character for EVs, which Otto et al. (1999) described as “a double edged sword” (p.3), since the quietness of an EV can be seen as an unique selling point or vice versa pose the question about the suitable sound character in terms of applying active sound design to emphasize the exquisite vehicle dynamics of EVs (Otto et al., 1999; Cerrato, 2009; Genuit & Fiebig, 2011, 2014; Meek et al., 2012; Allman-Ward et al., 2014; Bodden & Belschner, 2014, 2016; Fiebig & Schulte-Fortkamp, 2019; Allman-Ward et al., 2020; Gavric, 2020; Kleinjohann, 2020; Streicher et al., 2021). Figure 1 compares an ICEV and a BEV (Battery Electric Vehicle) in full acceleration run-up, showing the main differences between conventional and electrified vehicles in their frequency spectra, overall sound pressure level (SPL), engine orders, and operation smoothness.
FIGURE 1. Comparison of ICEV and BEV in full acceleration run-up.Note. Campbell diagrams of an ICEV (320 kW; left side) and a BEV (300 kW; right side) in full acceleration run-up. Please note the different scaling of the x-axes in the diagrams as the frequency ranges depend on the type of powertrain system. The zigzag pattern in the lower frequencies of the ICEV spectrum is caused by gear shifting. These gear shifts are omitted with the electrified system.
The EVs’ acoustic characteristics of e-powertrain and applied sound design compose a so-called soundscape, defined by the International Organization for Standardization as the “acoustic environment as perceived or experienced and/or understood by a person or people, in context” (International Organization of Standardization, 2014). Despite the frequently reported and apparent challenges, a considerably smaller amount of research focuses on the interior acoustic experience of electrified driving than on exterior sonification and pedestrian safety in EVs (Melman et al., 2021). Therefore, the focus of this contribution will be on how people perceive the interior soundscape while driving an EV and evaluate the current state of research in this field of acoustic vehicle development and design. A short theoretical introduction of essential changes in the NVH profiles of EVs and cognitive concepts of perception and aesthetics is followed by an overview of the reviewed literature presented in this paper. We thematically divided the reviewed literature into three different segments: 1) literature discussing objectified measures of human perception in the context of EV-NVH; 2) combining perception-related data with approaches of computational sciences to predict the acoustic quality of EVs; 3) research evaluating human perception of different NVH characteristics of EVs via the assessment through participant samples.
In this section, we provide a brief overview of the changes in the acoustic profile of EVs compared to conventional ICEVs. Furthermore, basic psychological concepts of perception and design, as well as frequently used constructs for customer perception in the field of acoustic engineering and vehicle acoustics, will be apprehended.
The electrified powertrain concepts lead to a substantially lower SPL (Blickensdorff et al., 2019), allowing hitherto unnoticed disturbing noises from sources such as the external environment, wind, tires, driving-related operation of auxiliaries and power electronics, which so far have been masked by the louder combustion engine, to become apparent (Allman-Ward et al., 2014; Blickensdorff et al., 2019; Eisele et al., 2019; Gavric, 2020). Bodden and Belschner (2014) even describe a spectral gap between the now unmasked high-frequency wind noise and low-frequency road noise leading to an inharmonic noise-background.
However, not only the reduced SPL and thereby unmasked disturbing noises require challenging NVH refinement, but substantial changes in the characteristics of the EVs’ NVH profiles themselves also lead to novel challenges in the developmental process of acoustic comfort. The overall SPL seems to be a less critical indicator of sound quality than psychoacoustic parameters (Genuit & Fiebig, 2011), which aim to describe the relationship between physically defined acoustic events and their perceptual dimension in an objective and quantitative manner (Fastl, 2002). As the spectrum of typical e-powertrain noise is characterized by higher frequencies and single tonal components in spectral ranges from 1 – 10 kHz, where human hearing is especially sensitive (Blickensdorff et al., 2019; Gavric, 2020), it is potentially perceived as more annoying and less appealing to customers as psychoacoustic analyses show (Lennström et al., 2013; Lennström & Nykänen, 2015; Swart et al., 2016). Moreover, there is a vital discourse about the quiet nature of EVs and opportunities of possible sound enhancement strategies in the field (Genuit & Fiebig, 2014; Clendinning, 2018). For instance, some criticize the EV’s interior for suffering from a lack of operational feedback (Kleinjohann, 2020) or that its quiet nature might even lead to insecurities about whether the vehicle is ready to use (Knowles et al., 2012). Bodden and Belschner (2014) note that the little operational feedback stands in no proportion to the pronounced dynamic acceleration of EVs. Whereas ICE-typical broadband noise is dominated by its engine orders and harmonics in a frequency range below 1 kHz (Blickensdorff et al., 2019), Swart et al. (2016) found local SPL-minima in the range from 200 to 500 Hz, low roughness values and a lack of prominent orders for commercial EVs, from which they derive the unimpressive and lackluster sound character of natural EV acoustics. With the inherent NVH characteristics only contributing very little to the EV’s interior sound quality, Allman-Ward et al. (2014) see it as a requirement for manufacturers to enhance their products through synthetic sound applications if they seek to improve interior sound quality. Through the application of artificial sound design, it is not only possible to re-establish operational driving feedback or to mask disturbing noises in the interior, but also to create a customizable, emotionalizing driving experience (Allman-Ward et al., 2014; Bodden & Belschner, 2016; Streicher et al., 2021). Furthermore, enhancing EVs with artificial sound can help to express specific vehicle characteristics such as brand identity (Sottek et al., 2005; Allman-Ward et al., 2014; Bodden & Belschner, 2016; Kleinjohann, 2020) as well as to sharpen the product’s character through distinguishing features for specific vehicle types and segments (Allman-Ward et al., 2020; Streicher et al., 2021). Nevertheless, a clear recommendation for development strategies and conceptual orientation regarding EV sound design cannot yet be concluded, and different directions are conceivable. Altogether, there is a target conflict between the generally preferred quietness in electrically driven vehicles and the demand for an adequate operational feedback (Fiebig & Schulte-Fortkamp, 2019). A rather conservative approach would be to draw analogies to existing experiences with ICE-typical sound and to reference traditional expectations (Bodden & Belschner, 2016). Other strategies like focusing on the perseverance of authentic e-powertrain NVH characteristics and possibly refining it, or creating completely novel yet suitable sound concepts (Genuit, 2012; Genuit & Fiebig, 2014) need to be considered. Kleinjohann (2020) sums up current examples of manufacturers like Volkswagen or BMW, who both cooperate with established composers and music producers to create novel sound concepts for EVs. Cerrato (2009) even presumes a transition phase from less innovative sound concepts, referencing familiar and relatable sounds of the combustion engine, to increasingly innovative concepts daring novel approaches such as, for example, sci-fi analogies. As the association between acoustic feedback of a vehicle and its power and performance parameters have been built over decades, resolving such association patterns will become difficult (Cerrato, 2009; Clendinning, 2018; Genuit & Fiebig, 2011, 2014). However, conservative approaches should be questioned, as the conventional ICEV-soundscape is hard to be naturally transferred onto EVs after all. Distinctive and innovative sound design approaches for EVs now pose the chance to set a novel frame of reference and build new connections between electric power sources and innovative soundscapes.
“Subjective” perception (in fact, perception as such, because perception is always a reality-constructing active cognitive act) is a crucial factor in market-relevant features such as comfort or product quality impression overall (Carbon, 2019). The present review focuses on the interior soundscape while driving an EV as it impacts a vehicle’s overall quality impression (Münder & Carbon, 2022). Though subjective dimensions are much harder to assess than physical or technical metrics (Genuit et al., 2010), especially the cognitive processes in subjective evaluation make such approaches even more interesting in terms of customer-oriented NVH development for EVs. NVH characteristics are immediately perceivable qualia shaping a customer’s product experience and therefore contribute to the overall customer satisfaction (Qatu, 2012; Zeller, 2018; Sinambari & Sentpali, 2020) as well as determine target agreements in product development (Blauert, 1986). In acoustic perceptual science, mere physical phenomena – acoustic events – are to be differentiated from perceptual constructs – the perceived quality of sound. Though perceptual constructs are related to specific physical phenomena and sensory information, they only emerge through human perception defined by the International Organization of Standardization (2014). The construct of the sound quality of an acoustic event is defined in manifold ways, such as its adequacy (Blauert & Jekosch, 1997) or suitability (Guski, 1997) of it, or even multi-dimensional definitions such as the suggestion by Genuit (1996) of three influential aspects, namely physical, psychoacoustical and psychological, with the latter including cognitive, affective, and situational factors (Blauert, 1986; Sottek et al., 2005; Zeitler, 2007). Stylidis et al. (2015) established a multi-dimensional model of overall perceived quality with a value-based perceived quality describing the total experience of a customer through its multimodal components and technical perceived qualities, each originating in the visual, acoustic, haptic, and olfactory domains of the assessed product. By accounting for further factors like context, customer behavior, and brand identity, the need to achieve high perceived quality for the given product can be identified (Stylidis et al., 2015). Also, Sottek et al. (2005) highlight the importance of including multimodal perception as the perception of sound is not limited to only hearing but also linked to vibration or visual perception. These aspects potentially influence the complex interplay of the overall customer experience. Fiebig (2012) distinguishes the general sound character from sound quality: the former is defined by the basic and physical attribute of the acoustic event and determinable in laboratory environments, whereas the latter is to be understood as a complex perceptional construct dependent on its context, product meaning, interaction, and cognition, where especially the sound’s adequacy to its context plays a key role (Fiebig & Schulte-Fortkamp, 2019). Thus, the subjective evaluation of perceptual constructs like sound quality and target sound design must be tested in externally valid context conditions (Genuit, 2010; Fiebig, 2012; Fiebig & Schulte-Fortkamp, 2019).
The mere number of versatile definitions of sound quality illustrates that subjective perception of acoustic events or soundscapes cannot be solely based on their nature. An auditory evaluation leads to an individual impression, strongly influenced by associative principles. For aesthetic theory, this central cognitive principle originates in the Aesthetic Association Principle (AAP) by Fechner (Ortlieb et al., 2020): an object transmits a specific impression by sensation and is then merged into a coherent percept with the individual’s former experiences. Thereby the principle is capable of guiding and creating certain associations in the acoustic domain (Ortlieb et al., 2020) and can be utilized in NVH development and EV sound design. For instance, Fiebig et al. (2020) show that even subtle acoustic information can influence the affective response to a contextual soundscape. Methods for subjective sound evaluation such as the Associated Imaginations on Sound Perceptions method (AISP) (Krebber et al., 2000), aiming to assess the affective reactions to vehicle interior noise, or the Explorative Vehicle Evaluation (EVE) method (Fiebig & Schulte-Fortkamp, 2019), a practical approach to evaluate a sound concept of a vehicle in its original on-road context, make use of the association principle. Other standard methods to evaluate vehicle sounds and sound quality are, among other things, ranking methods to determine sound preferences, semantic differentials to assess the meaning and suitability of a sound, category scaling, and magnitude estimation to evaluate differences in sound quality (Fastl, 2002). Factors such as cultural background (Fastl, 2002; Sottek et al., 2005) or the individual frame of reference, based on previous experiences (Genuit & Fiebig, 2014), should be considered as they might alternate the associated meaning of a sound. Thus, customer expectations are shaped through previous individual experiences with a product. Sound enhancement can potentially amplify the overall customer driving experience but, at the same time, requires a deepened understanding of what customers actually expect an EV to sound like (Allman-Ward et al., 2020). These expectations are still quite difficult to identify due to scarce internalized experience driving electrified and customers having difficulties in articulating their preferences as they lack reference points (Genuit, 2012; Genuit & Fiebig, 2014). Fiebig and Schulte-Fortkamp (2019) emphasize that the challenge lies in the evaluation of finding out how the majority assesses sound quality of EVs without any existing frame of reference while accounting for contextual variables of the specific acoustic event.
We reviewed the pertinent literature according to the following structure: the focal point of the review is research concerned with investigations on the human perception of EV interior soundscape, which results from acoustic NVH phenomena and active vehicle sound design. Investigations with participants and perception-related metrics were of our particular interest. Mere technical papers are of the highest importance for the vehicle’s NVH refinement but will not be elaborated on in this review. We included only automotive NVH-related literature published in the years 2000–2022 (effective 28th of February 2022). The used search terms for this contribution are depicted in Figure 2.
In total, we retrieved more than 200 articles from the period between 2000 and 2022 (effective 28th February 2022) that possibly dealt with the acoustic characteristics of EVs and/or their perception. After a thorough review of the articles, we excluded some articles from our list as they did not meet our criteria for investigating the human perception or perception-related metrics of EVs’ interior soundscapes and acoustics. Indicators to exclude articles were, for example, not dealing with the acoustic phenomena in an automotive or EV-specific context, not focusing on the subjective perception of these acoustic criteria, or dealing with the exterior noise and sound of EVs. This left us with 53 articles discussing the intended subject. We subdivided the final list of scientific work into different thematic clusters, which will be introduced in Results of the research analysis. At last, we would like to emphasize that this work provides an overview but is not necessarily an exhaustive representation of the interdisciplinary field of research.
We clustered the pertinent literature found with our search focus in three different main categories: A) studies focusing on psychoacoustic measures, which aim to provide an objective measure of subjective human acoustic perception (Cluster A: psychoacoustic metrics; N = 16); B) research from the field of computational sciences using subjective and/or objective data to form perception models (Cluster B: computational prediction models; N = 11); C) investigations with qualitative assessments and/or experimental study designs following a human-centered approach collecting subjective evaluation data from participants (Cluster C: subjective assessment and experimental studies; N = 26).
Psychoacoustic metrics are objective and quantifiable parameters developed to describe the relationship between subjective perception and physically defined acoustic events (Fastl, 2002) (see also Acoustical profile of electrified vehicles). The following section sums up current research on the perception of EV interior soundscape considering such psychoacoustic metrics. We identified two general groups of investigations: articles analyzing EV measurement data and taking objective psychoacoustic measures as sole indicators for subjective perception. On the other side, studies comparing these objective metrics with conducted subjective perception data from participants.
First, we like to look at the research investigating objective psychoacoustic parameters as sole measures for acoustic perception. Fang and Zhang (2014) analyzed the psychoacoustic parameters of loudness and sharpness in e-powertrain noise measurements from a test bench. Though the loudness increased with speed rise and sharpness was found to be unevenly distributed, they concluded the e-powertrain noise to be comfortable in terms of subjective perception (Fang & Zhang, 2014). Sarrazin et al. (2014) also investigated e-powertrain noise, specifically a 12/8 switched reluctance motor (SR), and evaluated parameters such as loudness, tonality, sharpness, and prominence ratio. Main findings show that the torque level had a rather big effect on tonality, loudness, but a smaller effect on sharpness, while the prominence ratio can serve as a detection tool for the various stator mode shapes (Sarrazin et al., 2014). Devillers et al. (2020) investigated on often as unpleasant perceived switching noise caused by the EV’s power electronics, also known as Pulse Width Modulation (PWM), which can vary in its switching frequency from 250 Hz to 20 kHz. In their analysis, they considered a couple of psychoacoustic parameters, which are assumed to cause unpleasantness in human perception. This potentially annoying nature of PWM is reflected in their findings of high prominence ratio and tonality values, as well as a strong roughness (Devillers et al., 2020). Moreover, they assume the lack of masking from wind and tire noise at lower driving speeds led to a predominance in the perception of switching noise. Due to its steady character, it lacks informational feedback about driving speed (Devillers et al., 2020). The main conclusion of Devillers et al. (2020) was that switching noise issues are worth considering in an early development stage as it can improve a vehicle’s NVH profile while reducing costs. Le Besnerais et al. (2018) also calculated psychoacoustic metrics – in this case, loudness (ISO532B), sharpness, and roughness – for isolated PWM samples at 5 kHz, 6 kHz, 7 kHz, 8 kHz, 9 kHz, and 10 kHz. Data showed that the higher the switching frequency was, the lower values resulted for roughness and loudness, suggesting the switching frequency has an impact on human hearing, though Le Besnerais et al. (2018) suggested further jury tests to determine a threshold level referring to the subjective acceptance for these metrics. Lennström and Nykänen (2015) specifically investigated the distribution of tonal components in EV noise and analyzed the number of prominent orders, their maximum levels, and frequency separation. Along these lines, they compared the tonality measures tone-to-noise ratio (TNR) and prominence ratio (PR). They recommend using TNR before PR when analyzing EV noise measurements with multiple closely related orders (Lennström & Nykänen, 2015). Stadtfeld (2021) investigated higher transmission input in electrified powertrains leading to pitch noise, generally higher frequencies and noise events even higher than the third mesh harmonic, which is beyond the audible threshold of human hearing. Stadtfeld (2021) cautions against the false conclusion that such frequencies are irrelevant to customer perception as conventional criteria such as frequencies below and above the third mesh harmonic or structure-borne frequencies still apply in further transmission stages of the EV. Specific configurational changes might therefore even facilitate changing previously annoying NVH characteristics to be perceived as a smoother, more unobtrusive noise (Stadtfeld, 2021). As former ICEV-related sound quality targets might not be applicable to the NVH criteria of EVs that are characterized with unusual narrow bands and a higher frequency spectrum, Mosquera-Sánchez et al. (2015) investigated on the complex interrelation between the psychoacoustic metrics of loudness, roughness and, sharpness as well as their cross effects. They concluded loudness as a single parameter to be insufficient and that further metrics need to be complemented to create a sound quality metric, which is suitable to analyze time-frequency patterns – for example, a loudness reduction may lead to an increase in roughness and sharpness – as well as to account for the metric’s case dependency for the specific product (Mosquera-Sánchez et al., 2015). In another study, Mosquera-Sánchez et al. (2018) investigated the sound quality specifically of hybrid electric vehicles (HEVs). They provided a framework for sound quality enhancement based on the psychoacoustic metrics of loudness, roughness, sharpness, and tonality. After acoustic measurements from HEVs’ passenger compartments were analyzed with the mentioned metrics, enhancement strategies were applied to actively control the sound quality and implement targets in real-time, and finally, the controlled sounds were again analyzed with the objective parameters to verify the applied measures (Mosquera-Sánchez et al., 2018). Qian et al. (2021) developed a sound quality synthesis model for EVs, which considers air- and structure-borne noise sources, as it is based on transfer path analysis (TPA) and transfer path synthesis (TPS). The synthesized and measured interior noise examples were compared in the psychoacoustic metrics of loudness, sharpness, and roughness. As the stimuli only differed by 7% in the analyses, Qian et al. (2021) concluded their model to reflect psychoacoustic physical characteristics of in-vehicle noise and the applied sound quality separation method to be an effective technique for sound design realization offering a technical basis for the evaluation and development of EV acoustics.
The second group of investigations in this cluster sums up research that analyzed psychoacoustic metrics and compared it with subjective perception data conducted in participant studies. Sarrazin et al. (2012) developed a sound synthesis tool for hybrid and electric vehicles and validated it with data from N = 29 NVH experts by letting them compare the synthesized sounds to original recordings in a subjective listening test. Therein participants were asked about the equivalence of the stimuli by evaluating categories of difference and similarity (Sarrazin et al., 2012). Furthermore, they analyzed the objective sound quality and psychoacoustic metrics of Stevens VII loudness, loudness ISO532A and B, fluctuation strength, roughness, pitch, tone-to-noise ratio (TNR), prominence ratio (PR), and sharpness (Sarrazin et al., 2012). As most stimuli were rated as identical, similar, or slightly different in the jury test and good overlaps in the analysis of the objective parameters were found, Sarrazin et al. (2012) concluded their product-specific sound synthesis tool to yield satisfying results. Bassett et al. (2014) investigated the tonal noise of EVs across a wide frequency band, the noise level as well as TNR, Stevens VI loudness, as well as tonality. They compared these metrics to subjective ratings of annoyance and the overall level of high-frequency noise in eight sound stimuli. The highest correlation with the subjective perception data was found for Stevens VI loudness: it considers the loudness of each octave band. It combines these partial loudness shares instead of being dominated by broadband masking noise or the most prominent tone in the signal as in other metrics (Bassett et al., 2014). Moreover, Bassett et al. (2014) found the metrics of PR and TNR to be good indicators to differentiate between tones but do not seem to be good predictors of perceived annoyance, as they did not align with the subjective ratings in their study. Ma et al. (2019) searched for an optimization method for permanent magnet synchronous motors (PMSM) using sensitive critical band (SCB) analysis. As objective parameters, they considered loudness, articulation index, tonality, roughness, fluctuation strength, sharpness, and the A-weighted SPL, which were all different depending on load torque and rotational speed (Ma et al., 2019). In a consequential study for the subjective evaluation of the PMSM noise samples, they had N = 22 participants rate the annoyance on a scale from 1 to 20 (the higher the value, the more annoying), representing five levels of annoyance (Ma et al., 2019). Their results show that with the same load torque, the annoyance gradually increased with rotational speed, while at a constant speed, the annoyance rose with load torque. The maximum annoyance was found in the operating condition of 5,800 rpm/100 Nxm (Ma et al., 2019). Through their method, Ma et al. (2019) were able to apply critical band pass filters, which could then be conducive to improving the sound quality of PMSM. Fang et al. (2015)1, established an objective evaluation parameter termed sensitive frequency-band energy ratio (SFBER) that correlated highly (0.958) with the subjectively evaluated annoyance, indicating to be of a better fit than other psychoacoustic parameters. The study by Pietila et al. (2019) supports a frequency dependence in the perception of annoyance by tones in the context of EV noise. A frequency-dependent annoyance curve was developed since common psychoacoustic metrics for tonality, such as TNR, hearing model tonality, and PR, focus on the quantification of tonal levels and detect their audibility in the presence of masking but do not intend to represent a function for perceived tonal annoyance (Pietila et al., 2019). To gain a better understanding of customers’ preferences in terms of EV tonality, N = 10 participants evaluated a total of eight EV drive-away sounds in moderate acceleration and significantly different frequency ranges in a paired comparison task (Pietila et al., 2019). The level of the stimuli got adjusted until perceived as equally annoying, whereby the built annoyance curve of Pietila et al. (2019) showed lower frequencies to be preferable and higher frequencies to be preferable the least, indicating a frequency dependence aspect to tonal annoyance. Doleschal et al. (2021) as well investigated tonal components of e-powertrain noise as they have the potential to diminish perceived pleasantness and, therefore, the acceptance of electrified driving systems. For the experiment, synthesized stimuli were systematically varied in their SPL and number of tonal components and then evaluated by N = 16 normal-hearing participants regarding the perceived magnitude of tonal components (MOTC) of the digitally generated sound samples (Doleschal et al., 2021). Doleschal et al. (2021) found the following parameters to have an influence on the perceived MOTC in their study: driving condition, the level of the 24th motor order, the presence of the 48th order, or structural resonances modeled as amplitude modulation. Moreover, psychoacoustic models and their ability to predict changes in perceived MOTC are referenced (Doleschal et al., 2021). Drichel et al. (2021) suggested an efficient prediction model for EV-NVH behavior and investigated the influence of its model fidelity level on the predictive quality of the perceived drivetrain-related airborne noise while considering human perception. The model development itself included the analyses of the psychoacoustic metrics loudness, sharpness, tonality, roughness, and psychoacoustic annoyance, which led Drichel et al. (2021) to the following observations: additional sidebands and friction-related excitations made the stimuli sound less tonal; more broad-band noise led to higher roughness; noise components in higher frequency ranges led to higher sharpness scores as the simulation might have underestimated the SPL in these ranges. For the validation of the model N = 30 participants were asked to evaluate multiple noise stimuli concerning a reference in regards to their similarity (from 0 = completely dissimilar to 100 = absolutely identical) in a MUSHRA test (Multi-Stimulus Test with Hidden Reference and Anchor) (Drichel et al., 2021). Table 1 gives an overview of the summarized literature assigned to this cluster and its analyzed parameters: a total of 16 articles, seven of them also including a comparison with subjective data.
In the following section we sum up research on computational prediction models for different NVH characteristics of EVs. Most articles utilized objective parameters as input variables for their prediction models, some furthermore considered subjective data for validation. All research discussed in this cluster applies techniques and approaches from the fields of computational science and artificial intelligence.
As the NVH profile of EVs and ICEVs differ substantially and subjective jury tests are time-consuming and labor-intensive, many researchers look out for a more efficient way to assess sound perception and thereby predict perceived sound quality. For instance, Mosquera-Sánchez et al. (2014) followed the approach of applying multi-objective algorithms to the sound quality optimization problem. To account for the elementary changes in electrically powered vehicles with more harmonic and tonal components, not only the parameter of loudness was considered, but also roughness, sharpness, and tonality were included in their models (Mosquera-Sánchez et al., 2014). In another study, Mosquera-Sánchez et al. (2016) tested active sound quality control algorithms to improve the sound quality of e-powertrain-induced noises, specifically in HEVs. Again, the sound quality metrics of loudness, roughness, sharpness, and tonality were included in the algorithms, which then reduced the disturbances and therefore were assumed to enhance the overall sound quality of the radiated noises (Mosquera-Sánchez et al., 2016).
The following contributions additionally considered subjective data for the validation of computed models. Fang et al. (2016)2, correlated psychoacoustic metrics of loudness, sharpness, roughness, fluctuation, and articulation index with subjective evaluation ratings of radiated e-powertrain noise. They established a predicting model for the sound quality of e-powertrains based on particle swarm optimization (PSO) and support vector machines (SVM) with higher prediction accuracy than models based on genetic algorithm (GA) methods and grid search methods (Fang et al., 2016). Moreover, their results indicate that subjective sensation could be reflected by sensitive frequency band energy ratio (SFBER) (Fang et al., 2016). Ma et al. (2016) developed an evaluation method for the sound quality of hub permanent magnet synchronous motors (HPMSM) in EVs by applying backpropagation (BP) neural network theory. First, they objectively evaluated the noise of HPMSM via (psycho-)acoustic metrics, including loudness, roughness, A-weighted SPL, tonality, sharpness, articulation index, and fluctuation strength, which they took as input variables for the BP neural network (Ma et al., 2016). Then they had N = 24 participants evaluate the stimuli subjectively regarding perceived annoyance through the grade evaluation method (GEM) and compared this data with the output of the BP neural network (Ma et al., 2016). In comparison, the evaluation of HPMSM noise by solely an A-weighted SPL appeared to be insufficient, possibly leading to false conclusions, whereas the established BP neural network reflected the physical characteristics of the noise and its influence on the human perception better with an error rate of only 3.97% (Ma et al., 2016). In another investigation, Ma et al., 2017b) again utilized BP neural network theory to develop a method to diagnose abnormal noise in interior permanent magnet synchronous motor (IPMSM) and evaluated its sound quality. The objective evaluation of IPMSM noise included the metrics of sharpness, roughness, loudness, articulation index, tonality, fluctuation strength and A-weighted SPL, which were then compared to the subjective evaluations of N = 24 participants that rated relative and absolute annoyance in a listening test by paired comparison method (PCM) and grade evaluation method (GEM) (Ma et al., 2017b). Instead of the A-weighted SPL, Ma et al., 2017b concluded sharpness to be the most influential factor in perceived annoyance, and their method showed improved detection accuracy for abnormal noise in IPMSM without needing a lot evaluation expertise. Fang and Zhang (2017a) objectively analyzed spectral frequencies, engine orders, and noise sources of e-powertrains and also followed up with a subjective evaluation of sound quality in terms of perceived annoyance. A sample of N = 21 participants evaluated measurements of ICEVs and EVs in a listening test, in which the ratings for the ICEV stimuli were found to be more consistent, presumably since these noises were more familiar (Fang & Zhang, 2017a). The psychoacoustic parameters of loudness, sharpness, fluctuation strength, roughness, sensitivity index, and articulation index were then analyzed and correlated with the subjective data, where very strong correlations for the metrics of sensitivity index (0.946) and sharpness (0.838) were found in regards of perceived annoyance (Fang & Zhang, 2017a). Finally, Fang and Zhang (2017a) established a sound quality prediction model through Support Vector Machine (SVM) algorithms, which showed to be effective in predicting the sound quality of e-powertrain noise with a relative error of only 2%. In another study, Fang and Zhang (2017b) had N = 30 participants evaluate the noise of PWM-fed electric powertrains in regards to their perceived annoyance, but this time established a prediction model for sound quality via a Support Vector Regression (SVR). The objective parameters of loudness, roughness, fluctuation strength, tonality, sensitivity index, and sharpness were analyzed, resulting in the two latter, again, showing the strongest correlations (0.917 and 0.842) with the subjective data (Fang & Zhang, 2017b). Moreover, results showed higher annoyance scores with increasing speed and high frequencies and high harmonic order components were perceived as annoying despite having a lower SPL (Fang & Zhang, 2017b). Qian et al. (2020) established a model for sound quality estimation based on a genetic algorithm-optimized back propagation artificial neural network (GA-BP ANN) and compared it to often used multiple linear regression (MLR) models. They criticize models based on MLR fail to map complex coherences between objective parameters and subjective perception, as well as often lack accuracy, which is why they see it as necessary to establish more accurate nonlinear models (Qian et al., 2020). Qian et al. (2020) had N = 32 participants evaluate different binaural EV measurements in a semi-anechoic chamber, asked to rate the perceived pleasantness of the stimuli on a scale from 1 to 11 via the grading method. Objective sound quality parameters such as loudness, sharpness, roughness, fluctuation strength, tonality, articulation index, and A-weighted SPL were evaluated and then correlated with the subjective rating data (Qian et al., 2020). Qian et al. (2020) found the used metrics and the subjective data to be correlated by 0.7 and higher, except for tonality with a much lower correlation coefficient. Overall, the GA-BP ANN model seems to offer advantages in terms of generalization and precision with higher accuracy. It showed a lower average percentage error than the MLR model (5.81 and 8.14%, respectively) for predicting subjective sound quality estimation (Qian et al., 2020). Following a similar approach, Qian and Hou (2021) established a sound quality evaluation model for the interior soundscape of electrified vehicles by utilizing intelligent algorithms and artificial neural network techniques of simulated annealing (SA) and genetic algorithm (GA) to further optimize the backpropagation neural network (BPNN) model. Binaural recordings of EV interior noise from different brands and models were analyzed regarding the objective parameters of A-weighted SPL, loudness, roughness, sharpness, fluctuation strength, articulation index, impulsiveness, and tonality (Qian & Hou, 2021). Subjective evaluation data was gathered from N = 36 participants that evaluated the sound quality of the noise samples played over headphones in random order in a laboratory setting in an anechoic chamber through paired comparison method (PCM) and rating scale method (RSM) (Qian & Hou, 2021). The derived SAGA-BPNN model for sound quality evaluation attained a high prediction accuracy, a low estimation error below 5% for estimated and actual scores, high correlation coefficients, and good generalization ability to forecast the sound quality of EVs (Qian & Hou, 2021). A weighted analysis showed that A-weighted SPL, loudness, sharpness, and roughness were important factors influencing perceived sound quality, sharpness even scoring the highest weighting of 32.62%, presumably due to high-frequency electromagnetic noise in EVs’ NVH profiles (Qian & Hou, 2021). Huang et al. (2021) criticized that most investigations in the field have been done on the interior noise of ICEVs with only limited validity for EV acoustics, and though there have been attempts to apply intelligent methods for sound quality prediction, these studies often only considered stationary noise, not applicable to dynamic use cases. Following up on these shortcomings, Huang et al. (2021) applied intelligent prediction methods and based their sound quality prediction model on tacho-tracking psychoacoustic metrics and deep convolutional neural networks (CNN) with adaptable learning rate trees (ALRT). Nonstationary noise of pure electric vehicles (PEVs) was recorded in use cases of normal and rapid acceleration as well as normal braking (Huang et al., 2021). N = 20 participants then evaluated these stimuli over high-fidelity headphones with the grade ranking method (scaled from 1 to 7) and tacho-tracking psychoacoustic indices of loudness, roughness, fluctuation strength, sharpness, articulation index, and tonality were analyzed (Huang et al., 2021). In comparison to other conventional methods that aim to quantify the contributions of different prediction metrics, the ALRT-CNN model, which combined subjective and objective data, showed an overperforming effectiveness (Huang et al., 2021). For further optimization, Huang et al. (2021) recommended to focus on the variety of sharpness, roughness, and loudness. For their investigations on the sound quality of power coupling mechanism noise in HEV, Lu et al. (2021) combined the methods of complementary ensemble empirical mode decomposition (CEEMD) and Hilbert transform (HT), as well as relevance vector machines (RVM) to acquire a sound quality prediction model. Psychoacoustic parameters of tonality, fluctuation, loudness, roughness, and sharpness served as input variables for the RVM model (Lu et al., 2021). To proof the model’s predictive capability, acoustical signals of the HEV power coupling mechanism under steady- and unsteady state operating conditions were presented in a laboratory environment over loudspeaker array to a sample of N = 30 participants that evaluated these stimuli through pairwise comparison in regards of perceived annoyance (Lu et al., 2021). Lu et al. (2021) concluded their CEEMD-HT model to have a higher predictive accuracy than the RVM-psychoacoustics model in terms of reflecting perceived sound quality and the prediction accuracy for steady operational conditions to be higher than for the unsteady state samples. Huang et al. (2022) focused specifically on tire/road structure-borne (TRS) noise that becomes apparent due to the EV’s muted nature and presented a novel method to tackle uncertainty optimization problems with the approach of improved interval analysis method (IIAM). Parameters like suspension shock absorber damping and spring stiffness were identified to contribute to the perceived sound quality of TRS, high correlations with the subjective data were found for loudness, roughness, and articulation index and the IIAM proved to outperform conventional approaches for SQ optimization like genetic algorithm (GA) models in a real-vehicle test (Huang et al., 2022). The described literature is summed up in Table 2.
In this third cluster, we sum up research that investigated the perception of the EV’s interior soundscape following a human-centered approach collecting subjective evaluation data from participants with methods, aside from mere objective metrics, such as qualitative assessments and/or experimental study designs.
Matuszewski and Parizet (2016) examined the validity of commonly used metrics for assessing sound quality in ICEVs in the context of EV interior noise in full-throttle acceleration mode. N = 19 participants evaluated recordings regarding perceived unpleasantness using two methods: pairwise comparison (12 stimuli, 66 pairs) and absolute evaluation using a slider bar that indicated five different states of unpleasantness (36 stimuli) (Matuszewski & Parizet, 2016). Matuszewski and Parizet (2016) found the conventional psychoacoustic metrics to be partially applicable as the A-weighted SPL remained an important predictor for perceived unpleasantness. However, metrics like PR should be endorsed due to the mid to high-frequency components in EV noise. Fang et al. (2018) focused the influence of acoustic harmonics in e-powertrain noise on the EV’s sound quality, subjective perception, and psychoacoustic measures. Stimuli were recorded in an anechoic chamber with an artificial head and then played back via headphones to N = 30 participants to be rated in terms of annoyance on a 10-point scale from very comfortable to very annoying (Fang et al., 2018). Fang et al. (2018) then fed the subject and objective data from a psychoacoustic analysis of the parameters loudness, fluctuation strength, roughness, sharpness, tonality, and sensitiveness into a Support Vector Machine (SVM) algorithm to establish a sound quality prediction model. Fang et al. (2018) found high associations with the subjective perception ratings for the parameters of sharpness and sensitivity, which seem to represent the characteristic high-frequency components of EV noise, ranging from the 20th to the 23rd 1/3 octave bands, to which the human hearing apparatus is especially prone. In an experiment, Lennström et al. (2019) specifically aimed to evaluate the coherences between the psychoacoustic metric of tonality and perceived pleasantness of the interior EV soundscape focusing on tonal components between 200 and 900 Hz. Binaural recordings of EV interior cabin noise from test track measurements were presented in varying sound levels to N = 20 participants, who were asked to rate the stimuli’s pleasantness on an 11-point scale from very unpleasant to very pleasant (Lennström et al., 2019). Ratings for low-frequency tones (200–400 Hz) turned out to be relatively constant, suggesting to be perceived not as disturbing independent of their sound level, whereas for high-frequency tones (600–900 Hz) the perceived pleasantness varied dependent on the SPL – the louder the tone, the less pleasant (Lennström et al., 2019). Beyond that, the detection rate for 200 Hz stimuli was found to be very low, independent of SPL, and increased in the frequency ranges of the 400 Hz stimuli and 600–900 Hz, respectively (Lennström et al., 2019). Lennström et al. (2019) moreover assumed more sensitive participants to be influenced stronger in their pleasantness rating, as a cluster analysis showed a group of participants to have a higher detectability performance and finding tones in the range of 600–900 Hz to be increasingly unpleasant with rising level, whereas a second group of participants generally rated lower on pleasantness independent of sound level and frequency. The subjective data was then compared to tonality values of different established metrics such as PR, DIN Tonality 45681, ECMA-74 Tonality, and ECMA-74 Tonality modified. Though all metrics demonstrated an accuracy of 80% and higher regarding tone detectability, no metric appeared to predict perceived pleasantness: the only significant correlation with pleasantness was found for ECMA-74 Tonality (r = −0.67). In an analysis excluding the seemingly undetectable 200 Hz stimuli, leaving the frequencies from 400 to 900 Hz, all correlation coefficients increased to 0.70 or higher (Lennström et al., 2019). The findings demonstrate that the discussed metrics could not model the sensation of pleasantness, as they merely aim to model tonality (Lennström et al., 2019).
Andersson et al. (2016) investigated magnetic noise from electrical machines in rear-wheel drive (RWD) EVs and evaluated the consequences on the perceived sound quality of the resulting noise when using different modulation techniques as well as applying different levels of switching frequency randomization. Six sound files, resulting from the combination of each modulation strategy and randomization level, were evaluated by N = 18 participants regarding their sound quality in terms of annoyance through the MUSHRA test method (Andersson et al., 2016). Results show that with space vector modulation, the randomization of the switching frequency did not seem to improve the sound quality (Andersson et al., 2016). For the first three speed segments, the perceived annoyance was higher for discontinuous pulse width modulation (DPWM), but until the field weakens, the randomized switching frequency affected the sound quality positively for DPWM stimuli (Andersson et al., 2016). Moreover, Andersson et al. (2016) found psychoacoustic metrics, such as different loudness metrics, the overall SPL and sharpness to have the greatest impact on perceived annoyance and tonality to be preferred as metric for tonal exposure, since the utilized variation parameters could easily bias PR and TNR. Münder and Carbon (2022) investigated the importance of e-powertrains noise to the perceived quality of EVs overall. Utilizing the Repeated Evaluation Technique (RET; Carbon and Leder, 2005), N = 65 participants evaluated ambisonic 3D measurements of different EVs recorded in four different use case scenarios presented to them in a sophisticated acoustic simulator (Münder & Carbon, 2022). It showed that the more perceivable the e-powertrain noise, the lower the perceived quality overall as a significant negative correlation of τ = −0.32 was found by Münder and Carbon (2022), demonstrating that acoustic characteristics in EVs clearly contribute to the overall perceived quality.
Yamauchi and Feng (2014) stress the function of vehicle noise to provide feedback about the vehicle’s condition and status. The generally quieter soundscape of EVs might complicate accessing information about the vehicles’ velocity or acceleration rate for the driver. Mixtures of one-third-octave band noises with controlled frequency shifting were utilized as driving sound stimuli and evaluated their acceleration impression in two subjective experiments – one in a laboratory set-up and one in a driving simulator environment–with the paired comparison method (Yamauchi & Feng, 2014). In the first experiment in the laboratory environment, participants evaluated the acceleration impression to validate the harmonization of the audio and visual impression of the stimuli (Yamauchi & Feng, 2014). The results showed the stimuli with frequency shift to be perceived as having greater acceleration, the 100 Hz component to have a lesser effect on this impression than the higher frequency bands, and a wider frequency shift to have a stronger association with acceleration (Yamauchi & Feng, 2014). The driving action itself, tested for in the second experiment in a driving simulator within an interactive scenario, did not affect the acceleration impression (Yamauchi & Feng, 2014). Küppers (2015) also reports on the dilemma of EVs giving less load feedback due to their natural quietness compared to ICEVs and that it, if it occurs, is often perceived as disturbing or unpleasant due to its high frequencies: the reduction of the disturbing powertrain noise accordingly may lead to a lack of informational and emotional feedback. In the study, synthesized sounds were evaluated in three different environments – a laboratory environment, a driving simulator, and a demonstration vehicle (Küppers, 2015). In a first step, Küppers (2015) validated the synthesized stimuli in the laboratory studio set-up, as participants showed high acceptance for the sound synthesis (27 out of N = 35 participants thought positively about the additional sounds). Results from the driving simulator experiment proved the sole wind roll noise to be accepted and perceived as pleasant; although load feedback was wished for in the comments, the genuine e-powertrain noise was rated as the least pleasant and paradoxically perceived as the most artificial sound (Küppers, 2015). Participants evaluated different sound variants in the vehicle demonstrator: three basic sounds, the sound turned off, and a self-configured sound from within the sample variants they could self-create by adding orthogonal sound parameters (for example, volume, timbre, reaction to throttle pedal) (Küppers, 2015). When participants freely configured the vehicle’s sound, only 14% of the sample preferred a switched-off sound, whereas most preferred the sound variants resembling combustion engines (Küppers, 2015). An exploratory study by Lee et al. (2016)3, showed that contextual information and quietness, taken as independent variables, influenced the general user experience of driving an EV. The interior driving sound influenced perceived satisfaction, emotions, and usefulness.
A couple of studies compared the effect of different sound design concepts for active driving sounds to enhance EVs acoustically. Govindswamy and Eisele (2011) compared the NVH characteristics of an EV with that of its conventional ICE-powered production counterpart and evaluated its perceived pleasantness and dynamic impression through subjective on-road driving tests and a jury test in an audio laboratory set-up. Sounds were objectively analyzed, and synthetically modified, and the applied sounds were then evaluated: a reduction of tonal high-frequency noise shares was found to improve pleasantness and preference ratings (Govindswamy & Eisele, 2011). Though the ICEV was perceived as more dynamic (and less pleasant), the mere addition of ICEV-resembling noise shares to the EV – attempting to improve the dynamic character or to mask unpleasant noise shares with a synthetic admixed sound – worsened the pleasantness and preference ratings, leading to the conclusion to consider sound design needs for each vehicle type individually (Govindswamy & Eisele, 2011). Gwak et al. (2014a) modified sound samples of real EV measurements by adding subharmonics to the existing high-frequency components, which otherwise were perceived as annoying and reduced the dynamic impression of the vehicle. They had N = 27 participants evaluate the sound samples regarding preference and on a semantic differential. The proposed sound modification method by Gwak et al. (2014a) proved to be effective in enhancing the perception of the vehicles’ interior soundscape: the level, number of tones, and frequency should be chosen carefully and to create a more dynamic sound impression, the tones should be under 600 Hz. Moreover, different customer groups seemed to have different preferences regarding EV sound enhancement strategies (Gwak et al., 2014a). In another study, Gwak et al. (2014b) tested two different sound modification approaches against each other and had N = 30 participants evaluate the specifically modified additive sound samples in regards of their effect on the perceived intensity of acceleration sensation, their sound image (multiple choice of describing adjectives) and their overall preference. Both applied sound modification methods were found to be effective in increasing the sensation of acceleration (Gwak et al., 2014b). While adding subharmonics to the high-frequency components led to a large loudness increment, the adjustment method of time gap improved the acoustic feedback without such significant level increment though attained only low preference ratings (Gwak et al., 2014b). Sontacchi et al. (2015) investigated active sound generation for EVs and down-sized ICEVs to improve their acoustic feedback and their potential effect on driving behavior and experience overall. Though many people preferred quiet vehicles a load-dependent loudness seemed important for the interior sound acceptance, while the as barely sporty described sound of electric engines could be improved regarding their acceleration impression by pitch shifting (Sontacchi et al., 2015). Samples of full-load run-ups were presented to the participants which were asked to identify the most different sound within a stimuli triplet and describe this difference semantically and rate it on an attribute scale (Sontacchi et al., 2015). Sontacchi et al. (2015) concluded that sound descriptions are biased by gender as only male participants named positive energy-related terms, while females focused on versatile descriptors. Additionally, they assumed an increase in amplitude modulation and loudness to elicit impressions of sportiness and acceleration, which could assist drivability and improve the product experience overall (Sontacchi et al., 2015). Sontacchi et al. (2015) advocate the active sound enhancement of EV interior soundscape and a more conservative approach in sound design strategy as they suggested ICEV-resembling sound characteristics and maintaining traditional manufacturer-specific sounds inspired by established experiences. Maunder and Munday (2017) also see an opportunity to enhance dynamics in the driving experience and benefit from positioning a certain brand image in actively controlling the acoustic response inside the EV’s cabin while aiming to maintain authenticity. In a survey, Maunder and Munday (2017) conducted information of N = 30 participants regarding the perceived acoustic feedback of EVs as well as their agreement with certain statements for each of the stimuli. In general, participants seemed to expect more conventional vehicle sound and rumble noise as it is referenced as powerful or sporty (Maunder & Munday, 2017). In contrast, similarities to typical ICEV noise did not meet their expectations of indicating an environmentally friendly character (Maunder & Munday, 2017). Despite widespread opinions regarding the expectations for EV sound, the authors were able to segment the sample into three distinct groups: the largest group focused on the power aspect and commented on a need for acoustic augmentation in the interior, preferring conventional sound options; secondly, a group recognizing the need for acoustic augmentation but refusing ICEV-resembling sound and appreciating novel approaches; and thirdly, a group of participants preferring the genuine EV interior sound as most suitable, not recognizing a requirement for additional sound augmentation (Maunder & Munday, 2017). Allman-Ward et al. (2020) developed a pre-set of 18 EV interior sounds, which was evaluated within the project team and of which eight sounds were taken for the final assessment in a jury test. Participants were asked to make a full ranking for preference of the eight stimuli, of which then two final sounds were applied in a real vehicle testing scenario allowing the participants to experience the sounds while driving (Allman-Ward et al., 2020). Though one of the modified sound concepts reached a common preference of 50% among the participants, the most appropriate sound for a sporty EV was the basic interior noise without any additional sound modifications, picked as the best solution by 8% of the sample, indicating the characteristic EV quietness to be appealing for customers as well (Allman-Ward et al., 2020). By comparing subjective perception data and objective metrics, Lanslots et al. (2020) investigated different sound design concepts to emotionalize the acoustic feedback and tackle the oft-cited problem of EVs lacking operational feedback and not doing their great acceleration justice. Designed sound concepts were applied to an EV and then recorded through a binaural headset at the driver’s ears positions in defined standard driving conditions of run-ups with an acceleration rate of 13.9 m/s (equivalent to a run-up from 0 to 50 km/h) (Lanslots et al., 2020). Aiming to quantify subjective perception criteria, psychoacoustic sound quality metrics were analyzed and compared across all stimuli, recognizing differences in loudness, sharpness, articulation index, tonality, prominence ratio, but roughness staying relatively the same (Lanslots et al., 2020). Finally, four sound concepts developed through granular synthesis, order-based synthesis, and other modifications, specified in Lanslots et al. (2020), were compared to the baseline sound with paired comparison method by an expert panel (N = 10) in a listening test: the sound resembling a V8 motor scored the highest preference, followed by a sound resembling a pickup truck (Lanslots et al., 2020). Although the preference for ICEV-resembling sound concepts might have been influenced by the sample’s driving experience (62.5% driving an ICEV, 25% a hybrid and 12.5% an EV), Lanslots et al. (2020) recommended aiming for a balanced proportion of powerful ICEV-resemblance and naturalistic EV sound depending on the load condition. Valeri and Pietila (2020) as well investigated EV sound enhancement as a method to improve NVH-related customer experience by accentuating the natural quiet sound character of EVs, while masking novel and annoying high-frequency components as well as offering feedback on vehicle performance. Differently enhanced sounds were recorded at the driver’s ears positions in a full pedal drive-away scenario and subsequently evaluated for preference in a paired comparison task as well as on a semantic differential to describe the experience (Valeri & Pietila, 2020). Valeri and Pietila (2020) found the following results: a reduction of tones (compared to baseline) led to a less sporty, exciting, or futuristic impression while at the same time it promoted a more smooth, natural, luxurious, effortless, pleasant, and harmonious impression; the addition of order harmonics enhanced the impression of sportiness and excitement while it reduced perceived smoothness, effortlessness, and the harmonious impression; a musical approach of sound enhancement through additional tones with spectral spacing led to a more futuristic, pleasant and harmonious impression, but was perceived as less sporty, exciting and natural. Finally, the results from the paired comparison indicated the diversity of customer preferences and the ability of sound enhancement to impact the product impression, as no sound is preferred overall and only subgroups could be extracted: 25% liked the sound without any modulation significantly better, and 17% preferred the modulation resembling a V8 motor (Valeri & Pietila, 2020). Melman et al. (2021) compared the effects on the perceived sportiness of EVs through measures of Modified Throttle Mapping (MTM) and Artificial Engine Sound (AES) to a baseline condition and a high-performant sports ICEV. The experiment followed a within-participant design, where each of the N = 32 participants evaluated the illusion of sportiness in different experimental trials with different road environments and driving tasks, realized in a fixed-base driving simulator with environmental scenery, visualized car interior, and a digitally applied speedometer (Melman et al., 2021). Each test trial was followed up by the assessment of perceived task effort, vehicle impression, and a semantic differential to describe the experience (Melman et al., 2021). AES proved to increase the illusion of sportiness in EVs and improved the speed control compared to baseline, whereas MTM did not significantly change the perception and led to more fluctuating pedal movement (Melman et al., 2021).
Swart and Bekker (2014) investigated the subjective evaluation of EV interior noise and the overall customer satisfaction using a ranking method and a bi-polar semantic differential. Recorded noise samples from an EV’s wide-open-throttle acceleration (interior and under-hood noise), two ICEV samples, and an artificially generated sound from a modified EV noise sample were used as stimuli and evaluated by N = 17 jurors in a laboratory test setting. The results show that exterior sound samples were generally perceived as louder and less pleasant compared to the interior sound of ICEVs (Swart & Bekker, 2014). Although semantics such as powerful, rumbling, and deep were commonly used to describe the EV sounds, the sporty ICEV sound was still preferred, and the dimensions of power and sportiness seemed to be expressed stronger (Swart & Bekker, 2014). In another study, Swart et al. (2018) put a particular focus on the sound character by investigating the descriptive dimensions and the overall perceived sound quality of EVs. N = 31 participants evaluated EV motor bay recordings and modifications in a listening test regarding overall satisfaction and conveyed semantic values (Swart et al., 2018). The subjective evaluation proceeded in two consequential steps: first, the preference for the stimuli with the differently enhancements was assessed through forced choice comparison ranking method and in a second step, the most preferred stimuli were evaluated on a bipolar 12-pair semantic differential scale (Swart et al., 2018). Sound enhancement seemed to be generally preferred compared to the standard production sound signature of the measured vehicles, especially enhancement methods such as low-frequency amplification, high-frequency filtering, low order addition, and pitch transposition achieved high scores (Swart et al., 2018). Furthermore, Swart et al. (2018) found the factors Power, Comfort, and Deepness, for the semantic description of the EV sounds and the highest correlations with satisfaction for the semantic attributes pleasant, exciting, and comfortable. In the context of semantic analysis Ma et al., 2017a) used a neural network approach to establish a sound quality prediction model based on the A-weighted SPL and six psychoacoustic parameters (loudness, roughness, fluctuation strength, tonality, sharpness, articulation index). These parameters were compared to semantic evaluation indexes conducted in a subjective listening test. A sample of N = 20 participants evaluated the interior noise of EVs on a semantic differential scale with five bipolar pairs (annoying – pleasing, weak – powerful, harsh – sweet, unobservable – perceptible, promiscuous – pure) (Ma et al., 2017a). Results show that at low speeds, the noise profile was described as pleasing, sweet, and pure, but weak and unobservable, presumably leading to the perception of a weak dynamic vehicle character and possible lack of vigilance, whereas at higher speeds, the profile was described as annoying, harsh, powerful, promiscuous, and perceptible compromising on perceived sound quality (Ma et al., 2017a). Swart and Bekker (2019) proposed a new metric to efficiently predict the perceived customer satisfaction regarding EV interior sound signatures and utilized psychoacoustic measures as well as subjective data. Binaural recordings of different EV sound signatures and enhanced, modified versions were subjectively evaluated by N = 31 participants in an anechoic chamber via headphones on a 12-pair bipolar semantic differential and a 10-point satisfaction scale (Swart & Bekker, 2019). The semantic analysis showed three main factors of Comfort, Power and a Futuristic factor and semantic descriptors such as exciting and quiet with weaker associations regarding customer satisfaction, whereas pleasant, comfortable, and calm were found to be highly correlated with perceived satisfaction (Swart & Bekker, 2019). Several significant correlations between objective psychoacoustic metrics and subjective ratings were reported, for example, for sharpness, loudness, and impulsiveness, while for the semantic descriptor sporty, no significant associations were found, which Swart and Bekker (2019) ascribed to the lack of a dynamic sound character in EVs. Aiming to bridge the gap between objective and subjective measures and accurately predict the subjective perception of customer satisfaction, Swart and Bekker (2019) followed the approach of single value approximation, including the objective metrics of sharpness, impulsiveness, and fluctuation strength through multiple linear regression, and established a single value reduction model. The results emphasize the importance of parameter sharpness in EV sound signatures as it predominantly influences customer satisfaction ratings (Swart & Bekker, 2019).
The testing environment and the given experimental context can have a relevant influence on participants’ perception. Depending on the target variable, different evaluation environments might be suitable, and the specific context should carefully be considered before data acquisition. Cocron et al. (2011) questioned N = 40 test drivers equipped with EVs in a naturalistic driving study (NDS) for a period of 6 months in the metropolitan area of Berlin about their experience. Though the focus of their investigation on driver’s perception primarily focused on possible safety issues due to the reduced noise emissions of EVs, the conducted data shows a high awareness for the lack of driving feedback and the EVs’ quietness, as well as expressed concerns regarding pedestrians’ and bicyclists’ safety in the beginning of the study (Cocron et al., 2011). These concerns decreased after 6 months, and expected substantial problems with the low noise emission level seemed to be obsolete as only a few incidents were reported (Cocron et al., 2011). Active sound design was not favored by the participants after 3 months of driving, but in the final interview, technical solutions, for example, a speed-dependent, the temporary sound was mentioned by a substantial part of the sample (Cocron et al., 2011). Labeye et al. (2016) followed a similar approach and conducted information regarding the driving experience with EVs in a longitudinal study with N = 36 participants over 6 months with two measurement time points between (T0 = beginning and T1 = after 3 months). Although the EV’s quietness was mostly seen as a safety-related topic due to their low recognizability for pedestrians, it was as well stated as an advantage regarding the vehicle experience by the questioned drivers (Labeye et al., 2016). Genuit and Fiebig (2014) followed the Explorative Vehicle Evaluation (EVE) method to interview N = 10 participants about their subjective impressions of three different EV sound concepts. Results showed a preference for quiet EVs, especially at low speeds, while at the same time, it was asked for operational acoustic feedback adequately matching the driving situation (Genuit & Fiebig, 2014). Though a slight preference for quiet sound concepts became apparent, the general acceptance of new sound concepts as well as tangible recommendations for EV sound design remain unclear as the participants’ comments were quite inconsistent, possibly due to a lack of reference and previous experience driving electrified (Genuit & Fiebig, 2014). Fiebig and Schulte-Fortkamp (2019) also applied the EVE method to conduct subjective data on spontaneous associations, emotions, feelings, and thoughts regarding the perception of different EV sound concepts from N = 10 participants in a realistic driving context. Sound concept variation was achieved by altered parameters of spectral content, total loudness, sound character, loudness gain, modulation, implementation of idle sound, and level load mapping, resulting in three different sound concepts (ICEV oriented, modern/unconventional, inconspicuous/modest) (Fiebig & Schulte-Fortkamp, 2019). Additionally, an individual sound concept, created by the participants themselves, was compared to the original driving noise of the test vehicle (Fiebig & Schulte-Fortkamp, 2019). While more negative than positive comments were reported overall, all sound concepts provoked positive as well as negative feedback, and synthetic sounds provoked more feedback in general (Fiebig & Schulte-Fortkamp, 2019). The results from Fiebig and Schulte-Fortkamp (2019) once again demonstrate the target conflict between the general preference for quiet EVs and the request for a load adequate acoustic operational feedback. Lennström et al. (2011) focused on the influence of the test environment on the sound perception using an 8-pair semantic differential to let participants evaluate different EV driving sounds. They found no significant difference between the testing conditions of laboratory test, vehicle demonstrator, and on-track evaluation regarding perceived sound quality (Lennström et al., 2011). However, a significant effect in the participants’ rating consistency showed: participants with an in-car experience (co-driving the EV) prior to the laboratory assessment showed smaller variances in their ratings compared to participants without such an exposition, as typical EV sound might have appeared as unfamiliar at first (Lennström et al., 2011). Another analysis of the preferences towards typical tonal components showed that an increased level of high-frequency tonal components and decreased mid-frequency tonal components ranked high on sharpness, annoyance, powerfulness, and toughness/aggressiveness. In contrast, a modification of the frequency components and vice versa achieved high rankings on overall satisfaction (Lennström et al., 2011). Table 3 summarizes all N = 26 articles in this cluster.
The pertinent literature of over two decades of research investigating the subjective perception of the interior soundscapes of EVs is summed up and elaborated in the review at hand. Although EVs are only gradually finding their way into our everyday lives, numerous investigations on the perceived quality of their NVH characteristics have already been done. Findings cover a wide range of investigations following different approaches of assessing qualitative feedback from customer studies, collecting subjective data in experimental studies, analyzing measurements for psychoacoustic metrics, or computing intricate models with conducted perception data to improve the prediction of perceived sound quality and customer satisfaction. Nevertheless, the general question of how the interior soundscape of electrified vehicles should be designed remains unclear. Though the displayed research bears valuable evidence on what might acoustically be preferred or rejected in a customer’s point of view, it also becomes evident that not even customers are yet aware of their needs and expectations regarding the composition of interior soundscapes in EVs. The findings so far are volatile due to minimal experience driving electrified and most likely also a lack of knowledge about design possibilities of acoustic enhancement strategies. For now, the general strategy of how to acoustically configure or even stage EVs is left up to each vehicle manufacturer and might possibly look like suggested by Sinambari and Sentpali (2020): either leaving the natural soundscape as it is with its typical occurring NVH characteristics due to the vehicle’s electrification and possibly refining this inherent noise, or adding artificially generated sound to the vehicle amplifying its operational feedback and enhancing specific product features – whether these sound concepts reminisce familiar combustion noise characteristics or embody a completely novel soundscape conveying innovative impressions is yet to be determined. The long-lasting association of vehicle power and noise with increasing acceleration seems to be etched in our minds, but now with the potential of silent EVs is challenged and described by Borg (2014) to be contradicting “a lifetime of experiences and expectations about cars and sound” (p.287), as it has been formed over decades. History, though, reveals contemporary associations between performance parameters of vehicles and their acoustic feedback, as silent automobiles have been appreciated and declared luxurious in the first half of the 20th century (Krebs, 2016). The now presented opportunity of freely creating novel soundscapes in EVs poses a challenge but at the same time, might help break up such conventions and possibly enrich the variety of how to shape driving experiences through novel soundscapes in the future.
The discussed research in this article highlights the importance of scientifically substantiated approaches to gather subjective data on the perception of the matter. Classic acoustical measures like the overall SPL or analyses of frequency bands will not sufficiently explain variances in customers’ perception of the product. Nor will psychoacoustic measures, attempting to objectify subjective perception data, be sufficient on their own if not validated through an adequate data sample of subjective assessments in the targeted application context. Especially context-related and novel evaluation approaches should be endorsed in future research to understand how customers evaluate novel soundscapes for EVs and how they build their expectations towards the respective product. Approaches like the EVE-method (Genuit & Fiebig, 2014; Fiebig & Schulte-Fortkamp, 2019), as well as naturalistic driving studies (Cocron et al., 2011) help to gain externally valid insights on customer behavior and evaluation. To better understand dynamic effects in customer perception, multiple measurement time points are needed to compare the evaluation data over the course of time. This can be done with multiple measurement points through, for example, interviews in externally valid environments (Labeye et al., 2016), or through approaches such as Repeated Evaluation (Carbon and Leder, 2005) that proved to be effective in controlled laboratory environments to offer a high degree of stimuli evaluation in a pretty short period of time. Moreover, person-related variables such as general driving experience, age, or former experience in driving electrified should be considered to gain customer-group-specific insights on how to target specific sound profiles in EVs.
The reviewed literature in this overview illustrates the uncertainties in customer expectations regarding the configuration and design of EV noise and sound. Human-centered, systematic, and experimental approaches to assess data on the human perception of EV interior soundscapes are needed to counter these yet volatile circumstances and challenges in customer needs. Participants need to be offered more extended elaboration with novel sound concepts in externally valid contextual settings, allowing them to explore their preferences and build their expectations. Only this way, we will be able to better understand dynamic effects in perceived quality and preferences regarding novel soundscapes, as well as to collect valid evaluation data on different enhancement strategies to derive design and target guidelines for acoustic automotive development.
MM had the initial idea to summarize the current research achievements on perception of interior soundscapes in electrified vehicles to determine the state-of-the-art in the field. C-CC contributed insights from aesthetic and perception research in automotive contexts and supported in matters of conceptualization and structure. MM and C-CC further worked on the manuscript.
The authors would like to thank the reviewers for their valuable feedback on the initial draft of this paper.
The authors declare that the research was conducted in the absence of any commercial or financial relationships that could be construed as a potential conflict of interest.
All claims expressed in this article are solely those of the authors and do not necessarily represent those of their affiliated organizations, or those of the publisher, the editors and the reviewers. Any product that may be evaluated in this article, or claim that may be made by its manufacturer, is not guaranteed or endorsed by the publisher.
1Please note that these findings are conducted from the article’s English abstract only, whereas the article of Fang et al. (2015) itself was written in Chinese and not available to us in English language in an authorized version.
2Please note that these findings are conducted from the article’s English abstract only, whereas the article of Fang et al. (2016) itself was written in Chinese and not available to us in English language in an authorized version.
3Please note that these findings are conducted from the article’s English abstract only, whereas the article of Lee et al. (2016) itself was written in Korean and not available to us in English language in an authorized version.
Allman-Ward, M., Franks, G., Di Nenno, G., and Miglietta, P. (2020). “A method for designing and delivering interior and exterior noise of an electric vehicle,” in Proceedings. Automotive acoustics conference 2019. Editor W. Siebenpfeiffer (Wiesbaden: Springer Fachmedien), 47–63. doi:10.1007/978-3-658-27669-0_4
Allman-Ward, M., Williams, R., Heinz, T., and Demontis, M. (2014). “Designing and delivering the right sound for quiet vehicles,” in INTER-NOISE and NOISE-CON Congress and Conference Proceedings, 4443–4451.2493
Andersson, A., Lennström, D., and Nykänen, A. (2016). Influence of inverter modulation strategy on electric drive efficiency and perceived sound quality. IEEE Trans. Transp. Electrific. 2 (1), 24–35. doi:10.1109/TTE.2015.2514162
Bassett, T. W., Tate, S., and Maunder, M. (2014). “Study of high frequency noise from electric machines in hybrid and electric vehicles,” in INTER-NOISE and NOISE-CON Congress and Conference Proceedings.
Blauert, J., and Jekosch, U. (1997). Sound-quality evaluation - a multi-layered problem. Acta Acust. 83, 747–753.
Blickensdorff, J., Boulliung, M., Burkard, M., Dold, C., Emretsson, B.-G., Genuit, K., et al. (2019). “Akustik,” in Elekrtifizierung des Antriebsstrangs. Editors H. Tschöke, P. Gutzmer, and T. Pfund (Springer Vieweg), 113, 303–359.
Bodden, M., and Belschner, T. (2014). “Comprehensive automotive active sound design part 1: Electric and combustion vehicles,” in INTER-NOISE and NOISE-CON Congress and Conference Proceedings, 3214–3219.2494
Bodden, M., and Belschner, T. (2016). “Principles of active sound design for electric vehicles,” in INTER-NOISE and NOISE-CON Congress and Conference Proceedings, 1693–1697.
Borg, K. L. (2014). Introduction: Constructing sociotechnical environments - aurality, air quality, and automobiles. Technol. Cult. 55 (2), 287–298. doi:10.1353/tech.2014.0049
Carbon, C.-C. (2019). Empirical approaches to studying art experience. J. Percept. Imaging 2 (1), 105011–105017. doi:10.2352/j.percept.imaging.2019.2.1.010501
Carbon, C.-C., and Leder, H. (2005). The Repeated Evaluation Technique (RET). A method to capture dynamic effects of innovativeness and attractiveness. Appl. Cogn. Psychol. 19 (5), 587–601. doi:10.1002/acp.1098
Cerrato, G. (2009). Automotive sound quality - powertrain, road and wind noise. Sound. Vib. 43 (4), 16–24.
Clendinning, E. A. (2018). Driving future sounds: Imagination, identity and safety in electric vehicle noise design. Sound. Stud. 4 (1), 61–76. doi:10.1080/20551940.2018.1467664
Cocron, P., Bühler, F., Franke, T., Neumann, I., and Krems, J. F. (2011). “The silence of electric vehicles - blessing or curse?,” in Proceedings of the 90th Annual Meeting of the Transportation Research Board.
Devillers, E., Gning, P., Degrendele, K., and Le Besnerais, J. (2020). Sound quality aspects of electric vehicles. ATZ Worldw. 07-08, 26–31. doi:10.1007/s38311-020-0254-5
Doleschal, F., Rottengruber, H., and Verhey, J. L. (2021). Influence parameters on the perceived magnitude of tonal content of electric vehicle interior sounds. Appl. Acoust. 1813, 108155. doi:10.1016/j.apacoust.2021.108155
Drichel, P., Jaeger, M., Möller-Giebeler, M., Berroth, J., Schroder, M., Behler, G., et al. (2021). “Influence of model detail level on the prognosis quality of the perceived sound of an electrified passenger car powertrain,” in IOP Conf. Ser, Mater. Sci. Eng., 012010. doi:10.1088/1757-899X/1097/1/01201010971
Eisele, G., Kauth, M., Steffens, C., and Glusk, P. (2019). “Automotive megatrends and their impact on NVH,” in Proceedings. 19. Internationales Stuttgarter Symposion. Editors M. Bargende, H.-C. Reuss, A. Wagner, and J. Wiedemann (Wiesbaden: Springer Fachmedien), 553–569. doi:10.1007/978-3-658-25939-6_45
Fang, Y., Chen, H., and Zhang, T. (2018). Contribution of acoustic harmonics to sound quality of pure electric powertrains. IET Electr. Power Appl. 12 (6), 808–814. doi:10.1049/iet-epa.2017.0824
Fang, Y., Zhang, T., Chen, F., and Guo, R. (2015). A subjective and objective evaluation model for psychoacoustical quality of electric vehicle noise. J. Xian Jiaot. Univ. 49, 97–101.
Fang, Y., Zhang, T., Chen, F., and Guo, R. (2016). Sound quality prediction of electric power train noise based on particle swarm optimization and support vector machine. J. Xian Jiaot. Univ. 50 (1), 41–46.
Fang, Y., and Zhang, T. (2014). Modeling and analysis of electric powertrain NVH under multi-source dynamic excitation. SAE Technical Paper. 2014-01-2870. doi:10.4271/2014-01-2870
Fang, Y., and Zhang, T. (2017a). Sound quality investigation and improvement of an electric powertrain for electric vehicles. IEEE Trans. Ind. Electron. 65 (2), 1149–1157. doi:10.1109/TIE.2017.2736481
Fang, Y., and Zhang, T. (2017b). Sound quality of the acoustic noise radiated by PWM-fed electric powertrain. IEEE Trans. Ind. Electron. 65 (6), 4534–4541. doi:10.1109/TIE.2017.2767558
Fiebig, A., Jordan, P., and Moshona, C. C. (2020). Assessments of acoustic environments by emotions - the application of emotion theory in soundscape. Front. Psychol. 11, 573041. doi:10.3389/fpsyg.2020.573041
Fiebig, A. (2012). “Psychoacoustics and jury testing for automotive applications – today and tomorrow,” in Proceedings of the Korean Society for Noise and Vibration Engineering Conference (The Korean Society for Noise and Vibration Engineering), 437–438.
Fiebig, A., and Schulte-Fortkamp, B. (2019). “Acceptance of synthetic driving sounds in the interior of electric vehicles,” in Proceedings. Automotive acoustics conference 2015. Editor W. Siebenpfeiffer (Wiesbaden: Springe Fachmedien), 18–34.
Gavric, L. (2020). “NVH refinement issues for BEV,” in Proceedings automotive acoustics conference 2019. Editor W. Siebenpfeiffer (Wiesbaden: Springer Fachmedien), 1–9. doi:10.1007/978-3-658-27669-0_1
Genuit, K., and Fiebig, A. (2014). “Sound design of electric vehicles - challenges and risks,” in INTER-NOISE and NOISE-CON Congress and Conference Proceedings, 3492–3501.249
Genuit, K., and Fiebig, A. (2011). Vehicle acoustics and sound design over the course of time. ATZ Worldw. 113 (7-8), 4–9. doi:10.1365/s38311-011-0070-z
Genuit, K. (1996). Objective evaluation of acoustic quality based on a relative approach. Proc. Institue Acoust., 3233–3238.
Genuit, K. (2012). (R)Evolution in vehicle acoustics—Sound design, warning signals and quiet cities. J. Acoust. Soc. Am. 131, 3301. doi:10.1121/1.4708343
Genuit, K., Schulte-Fortkamp, B., Fiebig, A., and Haverkamp, M. (2010). “Bewertung von Fahrzeuggeräuschen,” in Sound-Engineering im Automobilbereich. Editor K. Genuit (Springer Berlin Heidelberg), 14, 109–181. doi:10.1007/978-3-642-01415-4_4
Genuit, K. (2010). The future of NVH research - a challenge by new powertrains. SAE Technical Paper. 2010-36-0515.
Govindswamy, K., and Eisele, G. (2011). Sound character of electric vehicles. SAE Technical Paper. 2011-01-1728. doi:10.4271/2011-01-1728
Guski, R. (1997). Psychological methods for evaluating sound quality and assessing acoustic information. Acta Acust. 83, 765–774.
Gwak, D. Y., Yoon, K., Seong, Y., and Lee, S. (2014a). Application of subharmonics for active sound design of electric vehicles. J. Acoust. Soc. Am. 136 (6), 391–397. doi:10.1121/1.4898742
Gwak, D. Y., Yoon, K., Seong, Y., and Lee, S. (2014b). “Subjective evaluation of additive sound designed to reinforce acoustic feedback of electric vehicle,” in INTER-NOISE and NOISE-CON Congress and Conference Proceedings, 2157–2161.2496
Huang, H., Huang, X., Ding, W., Yang, M., Fan, D., Pang, J., et al. (2022). Uncertainty optimization of pure electric vehicle interior tire/road noise comfort based on data-driven. Mech. Syst. Signal Process. 165, 108300. doi:10.1016/j.ymssp.2021.108300
Huang, H., Wu, J., Lim, T. C., Yang, M., and Ding, W. (2021). Pure electric vehicle nonstationary interior sound quality prediction based on deep CNNs with an adaptable learning rate tree. Mech. Syst. Signal Process. 148 (1), 107170. doi:10.1016/j.ymssp.2020.107170
International Organization of Standardization (2014). Acoustics – soundscape – Part 1: Definition and conceptual framework. International Organization of Standardization. Availableat: https://www.iso.org/obp/ui/#iso:std:iso:12913:-1:ed-1:v1:en:ref:6 (Accessed June 20, 2022).
Kleinjohann, M. (2020). “Einsatzfelder des Acoustic Branding,” in essentials. Marketingkommunikation mit Acoustic Branding. Editor M. Kleinjohann (Wiesbaden: Springer Fachmedien), 19–45.
Knowles, M., Scott, H., and Baglee, D. (2012). The effect of driving style on electric vehicle performance, economy and perception. Int. J. Electr. Hybrid. Veh. 4 (3), 228. doi:10.1504/ijehv.2012.050492
Krebber, W., Adams, M., Brandl, F., Chouard, N., Genuit, K., Hempel, T., et al. (2000). Objective evaluation of interior car sound - the OBELICS project.
Krebs, S. (2016). Silent by design? Tesla's model S and the discourse on electric vehicle sound. Sound. Stud. 2 (1), 93–95. doi:10.1080/20551940.2016.1154406
Krishna, G. (2021). Understanding and identifying barriers to electric vehicle adoption through thematic analysis. Transp. Res. Interdiscip. Perspect. 10, 100364. doi:10.1016/j.trip.2021.100364
Küppers, T. (2015). Results of a structured development process for electric vehicle target sounds. Available at: https://www.researchgate.net/publication/286936646_Results_of_a_structured_development_process_for_electric_vehicle_target_sounds (Accessed June 20, 2022).
Kurani, K. S., Heffner, R. R., and Turrentine, T. S. (2008). Driving plug-in hybrid electric vehicles: Reports from U.S. Drivers of HEVs converted to PHEVs, circa 2006-07.
Labeye, E., Hugot, M., Brusque, C., and Regan, M. A. (2016). The electric vehicle: A new driving experience involving specific skills and rules. Transp. Res. Part F Traffic Psychol. Behav. 37 (4), 27–40. doi:10.1016/j.trf.2015.11.008
Lanslots, J., Ryck, L. D., Oltarzewska, A., Bodden, M., and Belschner, T. (2020). “Sound quality of interior active sound enhancement,” in INTER-NOISE and NOISE-CON Congress and Conference Proceedings, 4922–4933.2612
Le Besnerais, J., Dendiviel, C., Régniez, M., and Boussard, P. (2018). Sound quality optimization of electric powertrains for e-mobility applications.
Lee, D., Shim, H. R., and Choi, J. (2016). User experience design of interior driving sound for electric vehicle: Focusing on the contextual information and quietness. J. Korea Contents Assoc. 16 (2), 14–24. doi:10.5392/JKCA.2016.16.02.014
Lennström, D., Argen, A., and Nykänen, A. (2011). Sound quality evaluation of electric cars - preferences and influence of the test environment. Proc. Aachen Acoust. Colloquium, 95–100.
Lennström, D., Gutierrez Melian, A., Nykänen, A., Becker, J., and Sottek, R. (2019). “Perception of tones below 1 kHz in electric vehicles (No. 6),” in INTER-NOISE and NOISE-CON Congress and Conference Proceedings, 3675–3685.259
Lennström, D., Lindbom, T., and Nykänen, A. (2013). “Prominence of tones in electric vehicle interior noise,” in INTER-NOISE and NOISE-CON Congress and Conference Proceedings, 508–515.1
Lennström, D., and Nykänen, A. (2015). Interior sound of today's electric cars: Tonal content, levels and frequency distribution. SAE Technical Paper. 2015-01-2367. doi:10.4271/2015-01-2367
Lu, Y., Zuo, Y., Wang, H., and Wu, C. (2021). Sound quality prediction for power coupling mechanism of HEV based on CEEMD-HT and RVM. Neural Comput. Appl 33 (14), 8201–8216. doi:10.1007/s00521-020-04934-3
Ma, C., An, Y., Liu, L., Degano, M., Ning, X., Zhang, J., et al. (2019). Sound quality diagnosis method of permanent magnet synchronous motor for electric vehicles based on critical band analysis. IET Electr. Power Appl. 13 (10), 1613–1621. doi:10.1049/iet-epa.2019.0088
Ma, C., Chen, C., Liu, Q., Gao, H., Li, Q., Gao, H., et al. (2017a). Sound quality evaluation of the interior noise of pure electric vehicle based on neural network model. IEEE Trans. Ind. Electron. 64 (12), 9442–9450. doi:10.1109/TIE.2017.2711554
Ma, C., Li, Q., Deng, L., Chen, C., Liu, Q., Gao, H., et al. (2017b). A novel sound quality evaluation method of the diagnosis of abnormal noise in interior permanent-magnet synchronous motors for electric vehicles. IEEE Trans. Ind. Electron. 64 (5), 3883–3891. doi:10.1109/TIE.2017.2652718
Ma, C., Li, Q., Liu, Q., Wang, D., Gao, J., Tang, H., et al. (2016). Sound quality evaluation of noise of hub permanent-magnet synchronous motors for electric vehicles. IEEE Trans. Ind. Electron. 63 (9), 5663–5673. doi:10.1109/TIE.2016.2569067
Matuszewski, M., and Parizet, E. (2016). “Sound quality inside electric vehicles,” in NVH analysis techniques for design and optimization of hybrid and electric vehicles. Editors N. Campillo-Davo, and A. Rassili (Düren: Shaker Verlag), 329–342.
Maunder, M., and Munday, B. (2017). System for augmenting the in-cabin sound of electric vehicles. SAE Technical Paper. 2017-01-1757. doi:10.4271/2017-01-1757
Meek, B., Janssens, K., Sarrazin, M., and van der Auweraer, H. (2012). Sound engineering for hybrid and electrical vehicles.
Melman, T., Visser, P., Mouton, X., and Winter, J. (2021). Creating the illusion of sportiness: Evaluating modified throttle mapping and artificial engine sound for electric vehicles. J. Adv. Transp., 1–15. doi:10.1155/2021/4396401
Mosquera-Sánchez, J. A., Janssens, K., Desmet, W., de Oliveira, L. P. R., and Leopoldo, P. R. (2016). “Active noise control in hybrid powertrains guided multi-objective optimization of sound quality metrics,” in International Conference on Noise and Vibration Engineering (ISMA), 205–220.
Mosquera-Sánchez, J. A., Janssens, K., Desmet, W., de Oliveira, , and Leopoldo, P. R. (2015). Towards sound quality metrics balance on hybrid powertrains.
Mosquera-Sánchez, J. A., Sarrazin, M., Janssens, K., Oliveira, L. P. R., and Desmet, W. (2018). Multiple target sound quality balance for hybrid electric powertrain noise. Mech. Syst. Signal Process. 99, 478–503. doi:10.1016/j.ymssp.2017.06.034
Mosquera-Sánchez, J. A., Villalba, J., Janssens, K., and Oliveira, L. P. R. (2014). “A multi objective sound quality optimization of electric motor noise in hybrid vehicles,” in Proceedings of International Conference on Noise and Vibration Engineering, 1371–1386.
Münder, M., and Carbon, C.-C. (2022). Howl, whirr, and whistle: The perception of electric powertrain noise and its importance for perceived quality in electrified vehicles. Appl. Acoust. 185 (4), 108412. doi:10.1016/j.apacoust.2021.108412
Ormuz, K., and Muftic, O. (2004). “Main ambient factors influencing passenger vehicle comfort,” in Proceedings International Ergonomics Conference, 77–84.2
Ortlieb, S. A., Kügel, W. A., and Carbon, C.-C. (2020). Fechner (1866): The aesthetic association principle - a commented translation. I-Perception 11 (3), 204166952092030. doi:10.1177/2041669520920309
Otto, N. C., Simpson, R., and Wiederhold, J. (1999). Electric vehicle sound quality. SAE Technical Paper. 1999-01-1694.
Pietila, G., Seldon, W., Roggenkamp, T., and Bohn, T. (2019). Tonal annoyance metric development for automotive electric vehicles. SAE Technical Paper. 2019-01-1467. doi:10.4271/2019-01-1467
Qatu, M. S., Abdelhamid, M. K., Pang, J., and Sheng, G. (2009). Overview of automotive noise and vibration. Int. J. Veh. Noise Vib. 5, 1. doi:10.1504/ijvnv.2009.029187
Qatu, M. S. (2012). Recent research on vehicle noise and vibration. Int. J. Veh. Noise Vib. 8 (4), 289. doi:10.1504/ijvnv.2012.051536
Qian, K., and Hou, Z. (2021). Intelligent evaluation of the interior sound quality of electric vehicles. Appl. Acoust. 173. 107684. doi:10.1016/j.apacoust.2020.107684
Qian, K., Hou, Z., Liang, J., Liu, R., and Sun, D. (2021). Interior sound quality prediction of pure electric vehicles based on transfer path synthesis. Appl. Sci. 11, 4385. doi:10.3390/app11104385
Qian, K., Hou, Z., and Sun, D. (2020). Sound quality estimation of electric vehicles based on GA-BP artificial neural networks. Appl. Sci. 10 (16), 5567. doi:10.3390/app10165567
Sarrazin, M., Gillijns, S., Janssens, K., van der Auweraer, H., and Verhaeghe, K. (2014). “Vibro-acoustic measurements and techniques for electric automotive applications,” in Inter-Noise and noise-con congress and conference proceedings, 5128–5137.2492
Sarrazin, M., Janssens, K., and van der Auweraer, H. (2012). Virtual car sound synthesis technique for brand sound design of hybrid and electric vehicles. SAE Technical Paper Series. 2012-36-0614.
Sinambari, G. R., and Sentpali, S. (2020). Ingenieurakustik: Physikalische Grundlagen, Anwendungsbeispiele und Übungen. 6th ed. Springer Vieweg.
Sontacchi, A., Frank, M., and Höldrich, R. (2015). “In-car active sound generation for enhanced feedback in vehices with combustion engines or electric engines,” in International Conference on Auditory Display (ICAD).
Sottek, R., Krebber, W., and Stanley, G. (R. (2005). Tools and methods for product sound design of vehicles. SAE Technical Paper Series. 2005-01-2513.
Streicher, B., Lange, S., Eisele, G., and Steffens, C. (2021). Effiziente Methoden im Active Sound Design. ATZ Automob. Z. 123 (1), 58–61. doi:10.1007/s35148-020-0623-9
Stylidis, K., Wickman, C., and Söderberg, R. (2015). Defining perceived quality in the automotive industry: An engineering approach. Procedia CIRP 36, 165–170. doi:10.1016/j.procir.2015.01.076
Swart, D. J., Bekker, A., and Bienert, J. (2016). The comparison and analysis of standard production electric vehicle drive-train noise. Int. J. Veh. Noise Vib. 12 (3), 260. doi:10.1504/IJVNV.2016.080140
Swart, D. J., Bekker, A., and Bienert, J. (2018). The subjective dimensions of sound quality of standard production electric vehicles. Appl. Acoust. 129 (4), 354–364. doi:10.1016/j.apacoust.2017.08.012
Swart, D. J., and Bekker, A. (2019). The relationship between consumer satisfaction and psychoacoustics of electric vehicle signature sound. Appl. Acoust. 145, 167–175. doi:10.1016/j.apacoust.2018.09.019
Swart, H., and Bekker, A. (2014). “The subjective evaluation of interior noise produced by electric vehicles,” in 9th South African Conference on Computational and Applied Mechanics, 16.14
Valeri, F., and Pietila, G. (2020). Improved customer experience through electric vehicle sound enhancement. SAE Int. J. Adv. Curr. Pract. Mobil. 2 (4), 2411–2417. doi:10.4271/2020-01-1361
Yamauchi, K., and Feng, J. (2014). Effect of frequency shifting of additional sound for electric vehicles on acceleration impression. Forum Acusticum.
Keywords: psychoacoustics, NVH, perception, electrified vehicle sound, electric powertrain noise, automotive, sound quality
Citation: Münder M and Carbon C-C (2022) A literature review [2000–2022] on vehicle acoustics: Investigations on perceptual parameters of interior soundscapes in electrified vehicles. Front. Mech. Eng 8:974464. doi: 10.3389/fmech.2022.974464
Received: 21 June 2022; Accepted: 08 July 2022;
Published: 09 August 2022.
Edited by:
Haidong Shao, Hunan University, ChinaReviewed by:
Jinrui Wang, Shandong University of Science and Technology, ChinaCopyright © 2022 Münder and Carbon. This is an open-access article distributed under the terms of the Creative Commons Attribution License (CC BY). The use, distribution or reproduction in other forums is permitted, provided the original author(s) and the copyright owner(s) are credited and that the original publication in this journal is cited, in accordance with accepted academic practice. No use, distribution or reproduction is permitted which does not comply with these terms.
*Correspondence: Mara Münder, bWFyYS5tdWVuZGVyQHVuaS1iYW1iZXJnLmRl
Disclaimer: All claims expressed in this article are solely those of the authors and do not necessarily represent those of their affiliated organizations, or those of the publisher, the editors and the reviewers. Any product that may be evaluated in this article or claim that may be made by its manufacturer is not guaranteed or endorsed by the publisher.
Research integrity at Frontiers
Learn more about the work of our research integrity team to safeguard the quality of each article we publish.