- 1Department of Biomedical Engineering, McKelvey School of Engineering, Washington University in St. Louis, St. Louis, MO, United States
- 2Department of Mechanical Engineering and Materials Science, McKelvey School of Engineering, Washington University in St. Louis, St. Louis, MO, United States
- 3Department of Orthopaedic Surgery, School of Medicine, Washington University in St. Louis, St. Louis, MO, United States
Collagen is a major structural component of nearly every tissue in the human body, whose hierarchical organization imparts specific mechanical properties and defines overall tissue function. Collagenous soft tissues are dynamic structures that are in a constant state of remodeling but are also prone to damage and pathology. Optical techniques are uniquely suited for imaging collagen in these dynamic situations as they allow for non-invasive monitoring with relatively high spatiotemporal resolution. This review presents an overview of common collagen dynamic processes associated with human health and disease and optical imaging approaches that are uniquely suited for monitoring, sensing, and diagnosing these changes. This review aims to 1) provide researchers with an understanding of the underlying optical properties of collagen that can be leveraged for extracellular matrix visualization and 2) present emerging opportunities for machine learning approaches to drive multiscale and multimodality solutions.
1 Introduction
Collagen, the most abundant protein in the human body, plays a pivotal role in the structure and function of biological soft tissues. Collagen has been studied in varying degrees as early as the 1800s (Whitslar, 1889; Bielajew et al., 2020). Modern research on the molecular nature of collagen, including efforts to image collagen, began in the 1950s with electron microscopy studies of acid-soluble collagen fibrils extracted from rat skin (Highberger et al., 1951; Mayne and Burgeson, 1987). Technological advancements in imaging modalities have allowed for increased spatiotemporal resolution as well as movement towards in vivo monitoring of collagen dynamics.
This review will discuss imaging approaches that have been used to visualize dynamic processes of collagen that occur in health and disease of biological soft tissues, namely damage, homeostasis, healing, and fibrosis. The focus of this review will be optical techniques that have been shown to be particularly useful in imaging collagen dynamics due to their broad spatiotemporal resolution, quantitative, non-invasive, and non-ionizing nature. While interesting and potentially useful for collagen analysis, non-optical imaging modalities (e.g., ultrasound, magnetic resonance, nuclear imaging, etc.) are outside the scope of this review and will be left for discussion elsewhere. The concluding section of this review will consider an emerging topic that has been shown to augment and revolutionize data acquisition and analysis in optical imaging: machine learning approaches. Machine learning algorithms have been shown to be useful for image segmentation, disease prediction, image registration, and image enhancement that will be discussed in the concluding section to drive multimodal and multiscale solutions to dynamic collagen imaging.
2 Collagen Structure
At the molecular level, collagen consists of three polypeptide chains wound into a right-handed triple helical structure; this monomeric building block is often referred to as a tropocollagen molecule (Shoulders and Raines, 2009). The hallmark of each individual strand is a repeating XYG amino acid triplet sequence, where X and Y represent two of many possible amino acids (e.g., proline, hydroxyproline, lysine, hydroxylysine, alanine, etc.) and G is glycine. The most common triplet observed is when X and Y consist of proline and hydroxyproline, respectively (Ramshaw et al., 1998; Shoulders and Raines, 2009). Enzymatic covalent collagen crosslinking between tropocollagen molecules serves to mature the collagen network at the molecular scale where crosslinking occurs either between hydroxylysine residues or between hydroxylysine and lysine residues and is mediated by the enzyme lysyl oxidase (LOX) (Eekhoff et al., 2018; Bielajew et al., 2020).
Many genetically different types of collagen have been observed, each with unique functions; however, over 90% of total collagen typically belongs to a fibril-forming class of collagens (e.g., Type I, II, III) (Gelse et al., 2003). In fibrillar collagens, tropocollagen molecules self-assemble into more complex structures that aggregate across length scales in a hierarchical fashion forming fibrils, fibers and ultimately fiber networks or fascicular structures (Figure 1A) (Bielajew et al., 2020; Lake et al., 2020). Fibrils are arranged in a quarter-staggered alignment giving rise to a characteristic d-banding at around 67 nm intervals associated with collagen structure (Eekhoff et al., 2018). Collagen’s organizational structure is highly conserved across tissues and across species. Due to the hierarchical organization serving as collagen’s base structural unit, as well as the presence of undulating crimp in collagen fibers and fibrils, an abundance of collagen typically imparts biomechanical characteristics to a tissue such as non-linear stress-strain response, high tensile strength and low flexural stiffness (Goth et al., 2016). The degree of crosslinking also contributes to the tensile properties of collagenous tissues, and can sometimes be even more determinant of tensile stiffness than overall collagen content (Eleswarapu et al., 2011).
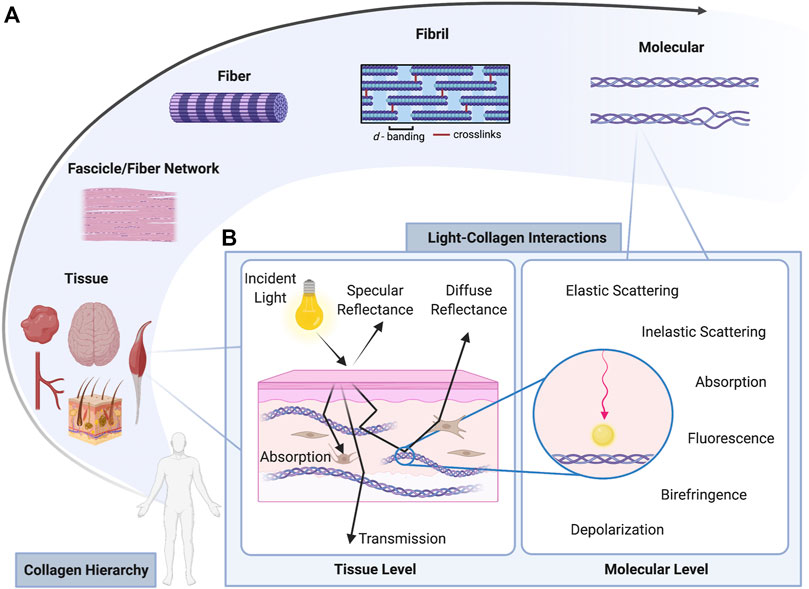
FIGURE 1. (A: Collagen Hierarchy) Collagenous tissues are arranged hierarchically where tissues are comprised of fascicles or fiber networks that are formed of fibers, which themselves are comprised of quarter-staggered and crosslinked fibrils. At the smallest length scale is the triple helical structure of collagen, which can become unwound when damaged or in periods of disease (B: Light-Collagen Interactions). When light is incident on a tissue, a portion of light is lost in the form of specular reflectance. The portion of light that enters the tissue interacts with the collagenous, cellular, and other ECM components via several mechanisms (scattering, absorption, polarization, fluorescence, etc). The light that penetrates the tissue travels until it is diffusely reflected, transmitted, or terminally absorbed. Created with BioRender.com.
3 Optical Properties of Collagen
Optical imaging of collagen and collagenous tissues have been performed for over half a century. Several rationales for the historical focus on optical techniques as medical imaging modalities for collagenous tissues were defined by Wang and Wu (2012). Most importantly, 1) optical techniques typically are non-ionizing and safer than other medical imaging approaches, 2) photon scattering spectra can provide biochemical information due to molecular conformation of tissue structures, 3) absorption of light can provide unique endogenous contrast and functionality in terms of blood flow or angiogenesis, 4) scattering of light can provide insights into underlying size, density, and other properties of structural elements like cells and proteins within tissue, 5) polarization state of light can be used to extract structural anisotropy of tissues, and 6) multiple exogenous contrast agents can be designed and added to a single system in order to enhance imaging of gene activity, biomarkers, or other features of interest (Wang and Wu, 2012). Optical modalities that have been developed for biomedical imaging leverage one or more of each of these unique principles to enable novel approaches to probing underlying structure, composition or function of tissue. Each imaging technique has unique spatiotemporal resolution, contrast mechanisms, as well as specific advantages and pitfalls that come from their unique optical mechanisms (Table 1). In the following sections, different optical properties associated with collagen related to these six areas will be described, before exploring central concepts in collagen dynamics and the imaging modalities best suited for visualizing each dynamic process.
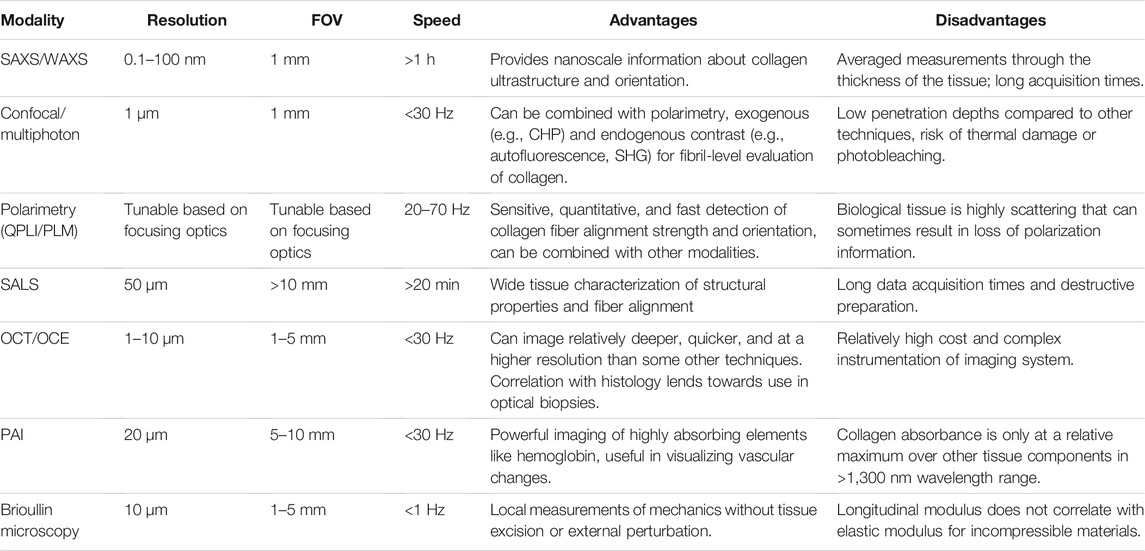
TABLE 1. Comparison of advantages and disadvantages associated with some identified and commonly used optical imaging modalities that are useful in imaging collagen dynamics.
3.1 Scattering and Absorption
The optical properties of collagenous tissues have been defined as including the scattering coefficient (μs), scattering anisotropy (g), absorption coefficient (μa), and refractive index (n) (Jacques, 2013). Taken together, these properties describe the typical behavior of light-matter interaction and, in particular, the balance between photon scattering and absorption (Figure 1B). These parameters can also be used to extract information about penetration depth, energy deposition, and underlying structure when imaging whole tissues as they are material and wavelength dependent (Wang and Wu, 2012).
Scattering is an interaction where the photon trajectory is changed due to interaction with local structures (Figure 1B). Collagenous tissues are highly scattering structures, where scattering interactions can be with collagen itself or with other cellular and sub-cellular structures such as cell nuclei, mitochondria, and other organelles (Mourant et al., 1998). The direction of scattering is governed by a scatterer’s geometry and size relative to the wavelength of incident light (Wang and Wu, 2012). For biological structures, scattering events are typically biased in the forward direction. These types of interactions, called elastic scattering (Wang and Wu, 2012), involve no amount of energy transfer to or from the incident photon from the scattering element. In contrast, during inelastic Raman scattering, molecular structures that vibrate at high frequencies transfer energy to the photon changing its wavelength (Goth et al., 2016). During inelastic Brillouin scattering, photons interact with the acoustic phonons of the molecular structures in a material of interest (Muanenda et al., 2019). The energy transfer in both types of inelastic scattering allow for the measurement of a tissue’s chemical composition or mechanical properties in Raman or Brillouin scattering, respectively.
Non-collagenous constituents of the extracellular matrix (ECM; e.g., fat, hemoglobin, melanin) are typically the primary absorbing elements within a tissue. The mean path length that a photon travels before a scattering event occurs in typical biological media is on the order of 0.1 mm whereas the typical absorption pathlength can be as far as 10–100 mm (Wang and Wu, 2012). Propagation of photons in collagenous tissues is characterized by multiple scattering events before collection by a detection system or, less likely, terminal absorption within the tissue (Figure 1B). The relatively high likelihood of photon scattering during propagation in turbid biological media is the key physical occurrence that enables signal generation in optical imaging techniques, but simultaneously also represents each modality’s Achilles’ heel. For example, the helical nature of collagen, and specifically its non-centrosymmetric structure, causes a non-linear optical effect called second harmonic generation (SHG) when photons interact with collagen (Shih et al., 2018). This phenomenon is unique in collagen-photon interactions and does not occur when light scatters with most other non-collagenous ECM proteins. Therefore, SHG is often used as an endogenous source of contrast and method for collagen identification during multiphoton imaging of collagenous tissues. However, the multiple scattering in the microscopy process causes rapid light attenuation along the propagation path, leading to low penetration depths, a problem common to most optical imaging techniques.
3.2 Birefringence
Due to the fibrillar nature of collagen, most collagenous tissues are structurally and optically anisotropic (Ghosh and Vitkin, 2011; Tuchin, 2016). Anisotropy gives rise to a property called birefringence that is readily leveraged in polarization sensitive optical techniques (Figure 1B). Propagating light travels faster along the long axis of collagen than across its cross-section due to differences in relative refractive indices in each direction, leading to double refraction, or linear birefringence (Tuchin et al., 2006). Collagen exhibits this optical property at the molecular level, so collagen molecules have been defined as a linearly birefringent monomeric building block (Maitland and Walsh, 1997). This structural, or intrinsic birefringence, aggregates across length scales and is maintained at the fiber level. At the tissue or fiber network level, the overall optical properties become dominated by birefringence of form, which manifests due to anisotropic alignment of materials in an overall isotropic media (Maitland and Walsh, 1997). In biological tissues, overall birefringence contributions are estimated to be ∼70% form and ∼30% intrinsic (Naylor, 1953; Maitland and Walsh, 1997). Birefringence is highly apparent in highly organized tissues like tendon, which consist of densely packed collagen fibers predominantly aligned in a preferred direction based on the orientation of in vivo load (He et al., 2021). Birefringence is also present in tissues with more disorganized collagen networks such as myocardium (Wood et al., 2010) or skin (Pham et al., 2018), but to a lesser degree.
As mentioned, birefringence refers to the difference between the refractive indices of the two axes of a sample. The directionality of the birefringence, positive or negative, implies if the extraordinary or ordinary axes are greater, respectively. Interestingly, different collagen types have different directional birefringence signatures at the molecular level; for example, collagen type I has been shown to be highly positively birefringent, whereas collagen type III exhibits weaker negative birefringence (Wang and Wu, 2012). Some methodologies have attempted to leverage these differences to differentiate between collagen types using polarized light microscopy analysis of stained histological tissue sections (Kirby et al., 2018). However, there is some debate in the literature about the reliability of this method for detecting collagen types since form birefringence is typically dominant over intrinsic birefringence (Dayan et al., 1989; Rich and Whittaker, 2017; Cui et al., 2019). There are also some contributions to the linear birefringence of a tissue from other ECM components such as elastin fibers, but to a lesser extent than collagen due to the smaller relative percentage (He et al., 2021).
Since optical anisotropy is linked to structural anisotropy, measuring changes in birefringence in response to loading allows for non-invasive assessment of dynamic fiber realignment (York and Gruev, 2012; York et al., 2014a; York et al., 2014b; Skelley et al., 2015; Goth et al., 2016). Additionally, since the structural integrity and anisotropy of biological tissues are not static over time (Ghosh et al., 2010), polarimetry principles can be used to explore dynamic tissue processes of disease, remodeling and repair. However, interpretation of polarimetry data from imaging of biological tissues can be nuanced due to the balance of (A) increasing polarization when light travels through an overall birefringent media and (B) multiple scattering events resulting in depolarization (Ghosh and Vitkin, 2011; Tuchin, 2016).
3.3 Fluorescence
Fluorescence is a linear optical process that occurs where a fluorophore absorbs and emits a single photon (Figure 1B) (Poole and Mostaço-Guidolin, 2021). This process can be exploited to visualize specific regions or components of biological tissues through endogenous or exogenous fluorescence. Collagen itself is autofluorescent, a property that is disadvantageous when seeking to detect other fluorophores in procedures like immunofluorescence because of the resulting background signal. However, collagen autofluorescence can also be leveraged for non-invasive, label free monitoring of ECM dynamics. Collagen’s autofluorescence is derived from its enzymatic (LOX-mediated) and non-enzymatic (glycation) molecular crosslinks (Kirkpatrick et al., 2006; Marcu et al., 2015). Therefore, collagen fluorescence can vary based on crosslinking density, fibril organization, and environmental factors like temperature and pH (Kirkpatrick et al., 2006). Additionally, other proteins like elastin and keratin, as well as enzyme cofactors like reduced nicotinamide adenine dinucleotide (NADH) and flavin adenine dinucleotide (FAD), also exhibit autofluorescence that can be monitored endogenously (Marcu et al., 2015). As mentioned previously, the non-centrosymmetric nature and non-linear scattering of light from collagen allows for generation of SHG signal, a property of collagen that is leveraged in in vitro and in vivo imaging modalities (Kirkpatrick et al., 2006). These intrinsic collagen properties are uniquely suited for dynamic processes as changes in collagen structure can be directly sensed via monitoring of fluorescence or SHG signal. For example, during skin photodamage, there are reductions in overall collagen crosslinking and total collagen synthesis that manifest as an increased SHG signal and a shorter fluorescence lifetime (Lutz et al., 2012; Marcu et al., 2015). There are also a number of exogenous fluorescent probes that can be conjugated to useful targeting elements (e.g., collagen hybridizing peptide specifically targets damaged collagen).
In summary, collagenous tissues exhibit several unique optical properties (scattering, absorption, birefringence, fluorescence) that enable optical imaging techniques to monitor dynamic processes across the length scales of its natural hierarchy (Figure 1B). Optical imaging modalities leverage these properties of collagen to offer unique insights into microstructure, mechanical properties, composition, and function (Table 1). In the next sections, the different dynamic processes relevant for collagenous tissues will be presented, followed by a discussion of the relevance of specific optical imaging modalities for each process.
4 Collagen Damage
Damage of soft tissue affects the structure of collagen at multiple length scales (Figure 2A) (Zitnay et al., 2017). The mechanisms of damage to collagen have been characterized in response to mechanical loading including tension, compression, and shear, in both traumatic acute and chronic fatigue scenarios. Additionally, collagen is susceptible to physical damage from heat and electromagnetic radiation, as well as enzyme-mediated degeneration. Specific approaches for imaging these types of damage will be covered in the subsequent section.
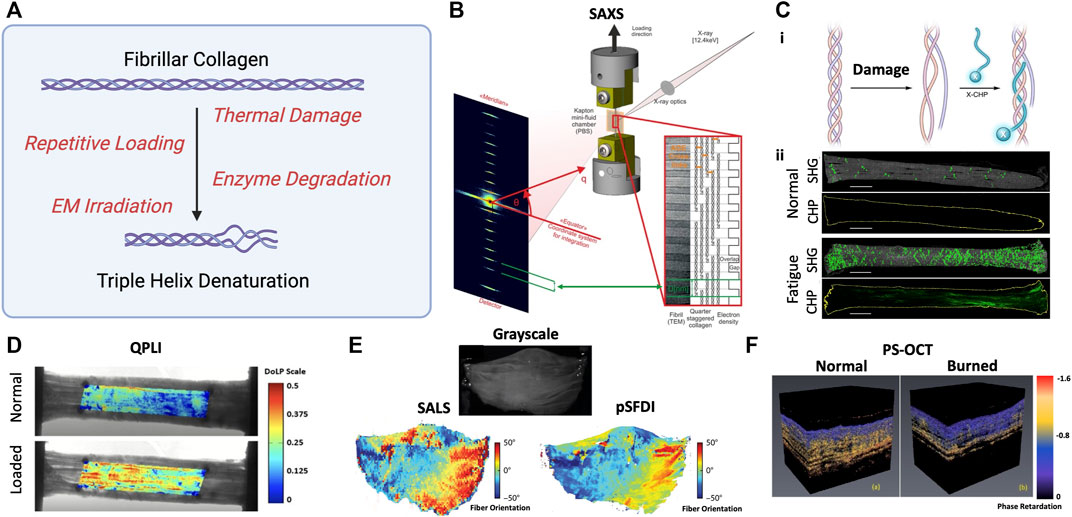
FIGURE 2. (A) Damage to fibrillar collagen is characterized by a loss in the triple helical structure of the collagen molecule and a “helix-to-coil” transition. Created with BioRender.com. (B) SAXS is performed on a tendon during mechanical loading and can discern changes in the d-banding of the collagen fibril. Reproduced with permission from Fessel et al. (2014). (C) i) Fluorescently labeled CHP forms triple helices with denatured collagen after damage. Reproduced with permission from Hwang et al. (2017a). ii) CHP staining and SHG imaging can be used simultaneously to visualize regional collagen damage due to fatigue loading via fluorescence and fiber kinking, respectively. Reproduced with permission from Szczesny et al. (2018). (D) Transmission mode QPLI can be used to quantify collagen fiber alignment in real time during dynamic loading. Reproduced with permission from Solon et al. (2021). (E) Both pSFDI and SALS are used to obtain fiber alignment maps of valve leaflets, but pSFDI attains a higher spatial resolution than SALS. Reproduced with permission from Goth et al. (2016). (F) Phase maps from PS-OCT can discern between normal and burned skin. Reproduced with permission from Hoang et al. (2018).
4.1 Tensile Loading
The response of collagen to, and damage from, mechanical loading is a function of the magnitude, rate, and duration of the applied load (Henao-Murillo et al., 2018). Under tension, collagen fibers straighten from their sinusoidally crimped structure and reorient along the direction of stretch (Billiar and Sacks, 1997; Wu et al., 2018); fibrils can elongate by more than 30% of their original length, reaching an ultimate tensile strength between 10 and 100 MPa (Iqbal et al., 2019). Dynamic changes in the microstructure of collagen result from mechanical damage when excessive loading is experienced. At the molecular level, collagen denaturation occurs during loading and is linked to delamination at the collagen fibril surface (Zitnay et al., 2017; Iqbal et al., 2019). The onset of collagen damage is internal, and may be present even before appreciably affecting tissue level mechanical function (Henao-Murillo et al., 2018). Moreover, macroscale mechanical properties of collagenous soft tissues change as a result of mechanical loading (Quinn and Winkelstein, 2008). For example, increased laxity, decreased stiffness, and changes in the viscoelastic response of ligaments and tendons has been observed as a result of sub-failure loading (Quinn and Winkelstein, 2008). When a tendon or ligament is subjected to a load initially, a toe-region in the stress-strain curve is observed as the collagen fibers straighten and reorient (Mienaltowski and Birk, 2014). As the applied load increases, the stress-strain curve transitions into a linear region, where microscopic damage will occur above a certain strain threshold (Mienaltowski and Birk, 2014). Accumulation of microscale damage yields to macroscopic damage, evidenced as the stress-strain curve becomes nonlinear, eventually leading to soft tissue rupture (Mienaltowski and Birk, 2014). Interestingly, the nature of in vivo functional demands on a tendon affects its damage mechanism. Specifically, plastic damage occurs along the collagen fibrils in tendons that fulfill a “positional” physiological role (i.e., relatively stiff, subject to low strain), whereas brittle fracture with little damage away from the failure location is observed in tendons that provide an “energy-storing” functional role (i.e., relatively compliant, subject to high strain) (Iqbal et al., 2019). Since microscopic and macroscopic mechanical responses of soft tissues are associated with pathophysiological conditions (Quinn and Winkelstein, 2008), understanding mechanisms of mechanical damage has profound clinical implications for improving patient outcomes (Henao-Murillo et al., 2018).
Collagenous soft tissues are commonly subjected to ex vivo uniaxial tensile fatigue testing to elucidate the changes in the mechanical properties of the soft tissue as a result of cyclic loading (Martin and Sun, 2015). Collagen fibers on the microstructural scale become increasingly disorganized with expanding space between the fibers as they experience kinking and plastic deformation with increasing levels of fatigue damage (Martin and Sun, 2015). Macroscopically, fatigue loading of collagen results in a longer toe-region of the stress-strain curve, decreased stiffness, and decreased ultimate tensile strength (Martin and Sun, 2015). However, ex vivo fatigue tests are limited since they don’t accurately replicate the physiological and mechanical environment of soft tissues in vivo, while also neglecting healing and complex in vivo loading mechanisms; thus, results may have reduced clinical relevance compared with in vivo fatigue damage studies (Martin and Sun, 2015).
4.2 Compressive and Shear Loading
Contrasting with tensile loading, compression of soft tissues results in collagen fibril reorganization in a direction perpendicular to the applied force, so that both tensile and compressive loading drives collagen to become more highly oriented in the direction of positive strain (Sizeland et al., 2020). However, this observed increase in collagen fiber alignment may only be valid under high compressive loads as decreased collagen fiber alignment has been observed when tissues are subjected to low compressive forces (Shah et al., 2016). Besides being a function of loading magnitude, collagen damage due to compression is also dependent on loading rates, with each of these parameters affecting microscopic and macroscopic mechanical damage differently (Henao-Murillo et al., 2018). Macroscopic damage is most affected by slow loading rates, whereas microscopic damage is susceptible to fast loading rates due to a dependency on the amount of time water can flow through the matrix during compression (Henao-Murillo et al., 2018).
Additionally, collagenous soft tissues are often loaded in shear, with shearing occurring either through the tissue thickness or across the width (Marshall et al., 2020). As collagenous tissues are hierarchically organized, tensile strain on tissues is often attenuated through the length scales due to sliding of the collagen fibers and fibrils past one another, loading them in shear (Kondratko-Mittnacht et al., 2015). In shear, the reorganization of collagen during dynamic shear testing affects the mechanical properties of the tissue. Collagen fascicles are reported to have decreased peak load, steady state load, and stiffness in shear loading compared with uniaxial tensile loading (Kondratko-Mittnacht et al., 2015). Interestingly, due to the mechanisms in which loads are transferred within a soft tissue, shear loads have been reported to be transferred primarily between collagen fibers rather than fascicles (Kondratko-Mittnacht et al., 2015). Collagen damage accumulation associated with shear loading has been relatively understudied in collagenous tissues, especially relative to compression and tension.
4.3 Hyperthermia
Thermal damage has shown to alter the structure of collagen as well as induce collagen denaturation (Schroeder et al., 2020). At supraphysiological temperatures (60–70°C and above), a permanent helix-to-coil transition occurs in collagen molecules (Wells et al., 2005; Ignatieva et al., 2007). At larger length scales, this transition manifests as tissue swelling, thickening, and loss of birefringence (Wells et al., 2005). A loss of SHG signal and birefringence is also evident at temperatures of 54°C and above (Sun et al., 2006; Meador et al., 2022). Interestingly, there has been shown to be a protective effect of isometric loading on heat damage, indicating that the physical constraint due to loading on the molecular structure prevents the initiation of hyperthermic damage and the formation of denaturation “nucleation sites” (Wells et al., 2005).
4.4 Electromagnetic Radiation
Photodamage is a process often associated with natural aging due to sun exposure, and the progressive accumulation of electromagnetic (e.g., ultraviolet and gamma) irradiation leads to the denaturation of collagen. UV light and gamma radiation cause structural damage to the triple helix of collagen, primarily by the formation of free radicals due to direct cleavage of bonds (Akkus et al., 2005; Rabotyagova et al., 2008). This conformational change and loss of helical integrity leads to a loss of bioactivity (Rabotyagova et al., 2008). Photodamage is concerning as part of the natural aging process, but also in gamma and UV mediated sterilization of collagenous allografts and biomaterials for tissue regeneration. Previous studies have shown some efficacy in using free radical scavengers to prevent collagen denaturation associated with irradiation damage, further emphasizing free radical activity as a main driver in photodamage of collagen (Akkus et al., 2005). At the tissue level, there is a loss in structural anisotropy and decreased alignment associated with photodamage that is detectable through polarimetry techniques (Dong et al., 2017).
4.5 Enzyme-Mediated Degeneration
Cellular driven, enzyme-mediated degradation of fibrillar collagen is essential for tissue homeostasis as a natural part of ECM turnover and maintenance (Sprangers and Everts, 2019). However, imbalances in this degradative process drive a number of tissue related pathologies. As one example, tendons in the rotator cuff of the shoulder are progressively degraded with chronic use or aging that is associated with increased matrix metalloproteinase (MMPs) activity that can lead to chronic tearing, pain, and loss of quality of life (Lake et al., 2009; Garofalo et al., 2011). MMPs and other proteolytic enzymes act to 1) identify and target collagen molecules, 2) unwind the triple helix, and 3) cleave individual strands during their digestion processes (Sprangers and Everts, 2019). MMP activity is also associated with cancer progression and metastasis, as well as the onset of osteoarthritis (Hwang et al., 2017a). Similar to hyperthermia, there is also a protective effect of isometric loading on enzyme cleavage of collagen through the sequestration of cleavage sites (Saini et al., 2020). Beyond the molecular length scale, enzymatic digestion has been shown to reduce the SHG signal due to disruption of the fibrillar structure but simultaneously increase the amount of diffuse reflectance by producing digestion products that act as scatterers in the ECM (Kim et al., 2000).
In summary, damage to collagen occurs across its hierarchical length scales and can be caused by one or more mechanisms. A commonality, regardless of damage mechanism, is the loss of the helical structure of collagen at the molecular level. As optical properties like intrinsic birefringence and second harmonic generation are highly based on the helical nature of collagen, damage results in loss of these properties that can be leveraged to non-invasively detect damage. Damage also aggregates across length scales, meaning that overall strength and uniformity of collagen fibril and fiber alignment is disrupted in periods of damage. Therefore, changes in fiber structure can be leveraged in wide field imaging modalities to discern local regions of damage and can then be correlated with mechanics to infer structure-function relationships. Because of the differences in optical properties that manifest across the different length scales with collagen damage, the next section of this review is stratified into smaller (nano- to micro-) and larger (macro-) scale imaging approaches.
5 Approaches for Imaging Collagen Damage
5.1 Nano/Microscale Imaging of Collagen Damage
5.1.1 Small- and Wide-Angle Light Scattering (SAXS/WAXS)
Modalities utilized to image dynamic collagen damage on the nano- to microscale include SAXS and WAXS. These modalities are based on the principle that incident electromagnetic radiation scatters in an anisotropic manner when encountering a tissue; analysis of this scattering allows for characterization of the underlying molecular and structural properties at the nanoscale level (Campbell et al., 2018). By measuring the angle of x-ray deflection from a crystalline structure, SAXS and WAXS are able to discern the d-spacing of collagen fibrils on the order of nanometers or Angstroms, respectively (Wei and Li, 2016). Within tissue collagen, SAXS and WAXS can measure the change in d-spacing, fibril diameter, density, and local orientation (Sizeland et al., 2020). Additionally, these techniques can be performed at discrete time points during mechanical testing to visualize structural changes associated with loading. For example, Sizeland et al. (2020) utilized SAXS to demonstrate that collagen fibers become more anisotropically oriented and with increased d-spacing when compressing bovine lateral meniscal fibrocartilage (Figure 2B). Additionally, SAXS and WAXS allow for quantification of properties like hydration and degree of chemical fixation, which has been demonstrated in the characterization of molecular changes and damage to collagen in the leather making process (Buchanan et al., 2019). WAXS enables the measurement of molecular strains within collagen fibers subjected to macroscopic mechanical testing (Bianchi et al., 2016). In addition, WAXS and SAXS measure collagen fiber orientation, which can be leveraged to create maps detailing the degree of collagen fiber alignment (Coudrillier et al., 2015). However, these maps are at a lower resolution than can be produced from other modalities explored in later sections as SAXS/WAXS only provides an average value for the degree of fiber alignment for a region of a tissue through its thickness (Coudrillier et al., 2015). Thus, SAXS and WAXS are limited in that collagen alignment heterogeneity within a region cannot be observed (Coudrillier et al., 2015).
5.1.2 Confocal/Multiphoton Microscopy
At the nano- to micrometer length scale, exogenous and endogenous contrast agents can be used to detect molecular damage to collagen, namely degradation of the triple helical structure in combination with microscopy techniques. Confocal and two-photon (2PM) microscopy are commonly utilized to observe these agents, enabling quantitative analysis of damage. 2PM possesses the advantage of increased penetration depth due to a longer excitation wavelength compared with confocal, but at the cost of increased rate of photobleaching and heat generation (Wang and Wu, 2012; Goth et al., 2016).
Collagen hybridizing peptide (CHP) has proven to be one of the most useful exogenous contrast agents in detecting collagen damage. CHP is a small, synthetic collagen mimetic peptide that binds to denatured collagen. CHP can be conjugated with several fluorescent probes for confocal microscopy techniques or with gold nanoparticles for use in electron microscopy. In addition to detecting failure, CHP staining can indicate sub-failure damage on the molecular level as the collagen triple helix is disrupted (Zitnay et al., 2017; Converse et al., 2018; Chen et al., 2019; Iqbal et al., 2019). Further, CHP has been used to detect damage associated with hyperthermia (Hwang et al., 2017b) and enzyme-mediated degradation (Bennink et al., 2018) through similar mechanisms.
One of the most useful applications for CHP staining is in detecting mechanically mediated damage. Soft tissues that have been stained with CHP after being mechanically tested in uniaxial tension (Zitnay et al., 2017; Iqbal et al., 2019) or fatigue loaded (Chen et al., 2019) have demonstrated increased levels of damage compared with unloaded controls. A direct relationship between level of strain and fluorescence of CHP-stain bound to unfolded collagen has been demonstrated (Iqbal et al., 2019). Since collagen is a structural protein, and its organization imparts mechanical properties to tissues, detection of mechanically mediated damage can indirectly infer loss of tissue mechanical properties. Importantly, CHP stain has been shown to bind heterogeneously throughout damaged tissue with concentrated regions of increased fluorescence, demonstrating its ability to detect local regions of microstructural damage (Zitnay et al., 2017; Converse et al., 2018). The ability to localize microstructure changes is of particular interest in identifying areas with propensity to damage in heterogeneous tissues. For example, CHP staining was used to determine that the media and adventitia of middle cerebral arteries in sheep are mainly damaged by different mechanisms; the former is prone to damage due to circumferential loading, whereas the latter becomes damaged with axial loading (Converse et al., 2018).
Beyond CHP, other collagen-targeting probes have been developed to visualize microstructural changes in collagen (Wahyudi et al., 2016). For example, Megens et al. (2010) described an exogenous fluorescent label conjugated with CNA35, a collagen binding adhesion protein involved in wound infection that was discovered in S. aureus (Baues et al., 2020). Interestingly, CNA35 can bind to both fibrillar and non-fibrillar collagens (Boerboom et al., 2007). In one study, CNA35 was conjugated with fluorescent probes or quantum dots then used to visualize collagen changes in arteries associated with atherosclerosis.
5.1.3 Second Harmonic Generation
Endogenous autofluorescence and SHG have also been widely used to identify the crimping pattern and orientation of collagen fibers in soft tissues. A key advantage is that SHG does not require samples to be labeled, fixed, or even sectioned (Campbell et al., 2018). SHG can be used to analyze and compare the orientation of collagen fibers in neighboring regions to find damage; areas are deemed to be damaged if the collagen fiber orientation abruptly changes relative to the alignment in surrounding regions (Sereysky et al., 2012). The total intensity of the SHG signal can also be indicative of damage (Brockbank et al., 2008). Further, SHG analysis can be used in combination with exogenous staining (e.g., CHP) to identify regions of localize damage as well as quantify fiber structure simultaneously (Figure 2C). In one study, SHG was combined with exogenous CHP staining to detect collagenous architecture disruption and degradation associated with AGEs in intervertebral disc degeneration (Hoy et al., 2020). Another study used SHG and fluorescent CHP staining to characterize collagen damage in skin biopsies from burn patients (Schroeder et al., 2020).
As mentioned earlier, one of the more unique properties of collagen is intrinsic and form birefringence. Taking advantage of this optical property, imaging techniques using polarized light can be used to rapidly quantify strength and orientation of collagen alignment across length scales (Tuchin, 2016). Polarimetry techniques can be used on their own or in conjunction with other imaging modalities such as SHG. Polarization-resolved SHG (pSHG) enables more comprehensive analyses on collagen orientation and organization beyond unpolarized SHG alone (Wang J.-Y. et al., 2021). The addition of a polarization state analyzer (PSA) in the beam path prior to detection allows for calculation of an anisotropy parameter that describe the relationship of the parallel and perpendicular polarized light in proportion to the overall SHG signal (Gusachenko et al., 2012). pSHG has been used to quantify oxidative damage to collagen fibers associated with the aging process (Miler et al., 2021). In another study, pSHG was used during mechanical testing of soft tissues that were subjected to constant strain (Wang J.-Y. et al., 2021). Wang J.-Y. et al. (2021) utilized pSHG to demonstrate that collagen fibers within intervertebral discs become increasingly disorganized during cyclic loading once they have been punctured with a needle compared with uninjured controls.
5.2 Macroscale Imaging of Collagen Damage
5.2.1 Polarization Imaging and Microscopy
On their own, polarimetry techniques are particularly useful due to their quantitative nature, ability to probe different length scales based on focusing optics, low cost, minimal equipment needed and real time data acquisition (Goth et al., 2016). Quantitative polarized light imaging (QPLI) or polarized light microscopy (PLM) (depending on the length scale of interest) are among the most common modalities implemented when imaging collagen macroscopically in vitro. In these systems, a light source is placed in series with a polarization state generator (PSG) that induces a particular polarization state of light (e.g., linearly or circularly polarized) (York et al., 2014a). The polarized light beam is then incident on a collagenous tissue of interest, which changes the polarization state of light in accordance with its optical anisotropy. The output light beam is then passed through a PSA and collected for analysis. The optical path can either involve transillumination of the sample (i.e., transmission mode) or collection of light that has been backscattered via diffuse reflection (i.e., reflectance mode) (Tuchin, 2015). Transmission mode imaging offers the advantage of calculating full thickness average values of alignment at the cost of requiring tissue excision and thinning. Reflectance mode imaging allows for in situ analysis of fiber alignment but results in loss of some polarimetric information due to collection of multiply scattered photons. Reflectance mode QPLI has been used in conjunction with spatial frequency domain imaging (SFDI, without polarization; pSFDI, with polarization) to enhance the ability to control imaging depth and separately discern superficial and deep structures (Yang et al., 2015). Due to the fast rate of data capture and the ability to calculate regional anisotropy across a large field of view, QPLI has been used to observe collagen fibers in dynamic loading conditions (Chakraborty et al., 2016). Dynamic changes in microstructural organization can be recorded and analyzed via the creation of collagen fiber alignment maps (Figure 2D) (Quinn and Winkelstein, 2008; Lake et al., 2010; Buckley et al., 2013; Skelley et al., 2015; Shah et al., 2016; Zhang et al., 2016; Barnum et al., 2017; Wu et al., 2018; Smith et al., 2019). These spatial maps of collagen organization then can be correlated with tissue microstructural and mechanical properties to link structure-function relationships (Skelley et al., 2015; Skelley et al., 2016; Wright et al., 2016; Smith et al., 2019; Solon et al., 2021).
In addition to capturing dynamic collagen maps, QPLI can also be implemented to detect the onset of damage (Quinn and Winkelstein, 2008). For example, Quinn and Winkelstein (2008) used QPLI to demonstrate that the initiation of collagen damage is correlated with a decrease in tissue stiffness in ligaments of the cervical spine. QPLI has been performed while soft tissues were subjected to different types of mechanical loading, including tensile (Quinn and Winkelstein, 2008; Lake et al., 2010; Buckley et al., 2013; Skelley et al., 2015; Shah et al., 2016; Zhang et al., 2016; Barnum et al., 2017; Wu et al., 2018; Smith et al., 2019) and compressive (Shah et al., 2016) tests. In addition to mechanical damage, outputs from other biomedical polarimetry systems, like Mueller matrix polarimetry, have been used to monitor photodamage from UV light exposure over time, as well as to evaluate the protective effect of sunscreen on preventing damage (Dong et al., 2017). Further, polarized light has been used in the detection of collagenase mediated degeneration of cartilage by monitoring the reduction in birefringence of digested tissue over time (Långsjö et al., 2002). PLM can also be used to enhance data from histology, such as in picrosirius red stained tissue sections to evaluate regional changes in birefringence, that can sometimes indicate regions of damage (Rich and Whittaker, 2017).
5.2.2 Small Angle Light Scattering
Similar to SAXS/WAXS, SALS is a technique based on the anisotropic scattering of electromagnetic radiation, using visible to near-infrared light instead of x-rays. In SALS, a laser beam is transmitted through the biological tissue; the resulting scattered light image is recorded and used to calculate the alignment and organization of the collagen fibers (Whelan et al., 2021). The data collected from SALS can then be used to develop contour plots detailing fiber angles and the relative strength of alignment (Whelan et al., 2021). In addition, SALS can be leveraged to quantify collagen fiber alignment and orientation nondestructively (Whelan et al., 2021). Billiar and Sacks (1997) utilized SALS to demonstrate that collagen fibers reorganize themselves along the axis on which a tissue is subjected to tension. Other studies have used SALS to evaluate collagen fiber alignment in the presence of bacterial collagenase, which has elucidated the protective effect of strain on the enzymatic digestion of collagen fibers (Robitaille et al., 2011; Gaul et al., 2018).
Although an effective macroscale imaging modality, SALS has some limitations. First, SALS is applicable to thin or optically cleared tissues only (<500 µm), so imaging biological tissue in situ is challenging (Billiar and Sacks, 1997). Additionally, for 2D imaging of tissues macroscopically, bidirectional translation of the sample is required, resulting in long scanning times (>1 h per ∼5 cm2) even at modest (200 µm) translation steps (Goth et al., 2019). For these reasons, SALS is not used as frequently as QPLI or other polarimetric approaches in macroscale imaging of collagenous soft tissues in vitro. Goth et al. (2019) performed a comparative analysis of SALS and pSFDI, demonstrating improved resolution of the latter as well as the ability to control sampling depth that is not possible using transmissive SALS (Figure 2E).
5.2.3 Optical Coherence Tomography
Optical coherence tomography is a noninvasive imaging modality also employed macroscopically to look at tissue architecture. In OCT, the analysis scale is defined by choice in focusing optics, so OCT can be used to study collagen across length scales; typically, OCT is used at the fiber-to-tissue level (Laurence et al., 2021). Rooted in Michelson interferometry, OCT often is referred to as an optical equivalent of ultrasound, in that it measures the optical path length of photons after backscattering from tissue (Wang and Wu, 2012). As it utilizes infrared light, OCT operates with a depth-to-resolution ratio greater than 100, providing high-resolution images at a fast rate (Wang and Wu, 2012). OCT captures 3D collagenous microstructure via collagen scattering optics and can elucidate localized regions of damage in collagenous soft tissue due to local changes in optical properties (Laurence et al., 2021). For example, Laurence et al. (2021) utilized OCT to show variable thickness in aneurysm tissue and localized damage in the collagenous medial layer. OCT approaches are widely used in ophthalmology, cardiology, orthopaedics, and dermatology. For example, OCT has been used to examine collagen changes associated with sun damage or in the cases of burns, as well as to assess and differentiate normal, chronologically aged, and photodamaged skin in vivo and ex vivo (Mamalis et al., 2015).
Polarized light can be incorporated with OCT in a modality known as polarization sensitive optical coherence tomography (PS-OCT). PS-OCT is an effective imaging modality that provides information about the structure and birefringence of collagen, similar to other polarization sensitive modalities (Figure 2F) (Le et al., 2015). Le et al. (2015) used PS-OCT and SHG to obtain tissue birefringence and collagen orientation index (detailing collagen fiber orientation data), respectively, and discovered that these quantities were linearly correlated. Also advantageous, birefringence information can be collected during dynamic loading of collagenous soft tissue, so PS-OCT can be used to detect mechanical damage to tissue strcuctures (Shin et al., 2018). PS-OCT is also commonly used in vivo during ophthalmologic imaging to detect damage associated with macular degeneration of the retina (Baumann, 2017).
6 Collagen Remodeling/Repair
Extracellular matrix remodeling occurs in response to damage, but also during normal maintenance of tissue homeostasis. In both normal remodeling and repair, there is a delicate interplay within the ECM to maintain a balance between anabolic and catabolic processes (Figure 3A). A lack of the former can lead to chronic disease and inability to appropriately heal from damaging stimuli, whereas an absence of the latter leads to fibrosis and cancer.
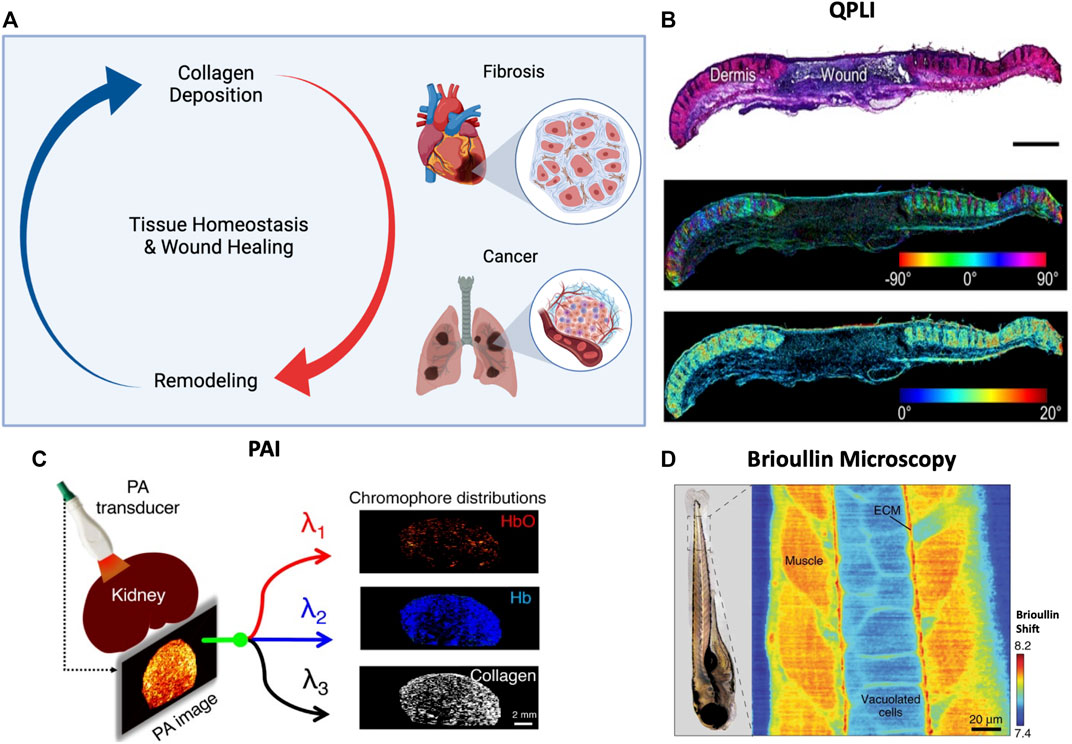
FIGURE 3. (A) Proper tissue homeostasis and wound healing is governed by an intricate balance of collagen deposition and remodeling. When this cycle becomes dysfunctional, pathologies like fibrosis and cancer can become prevalent. Created with BioRender.com. (B) QPLI has been used to monitor wound healing in dermis via tracking changes in collagen fiber orientation and birefringence. Reproduced with permission from Woessner et al. (2019). (C) Spectral unmixing in PAI is used to isolate quantify collagen content and hemoglobin in kidney. Reproduced with permission from Hysi et al. (2020). (D) Brillouin frequency shift maps in zebrafish tail discern local difference in mechanics between muscle and ECM. Reproduced with permission from Prevedel et al. (2019).
6.1 Tissue Homeostasis
The maintenance of tissue homeostasis and normal remodeling is essential for proper regulation of cell and tissue function. Homeostatic remodeling underpins processes in development, the normal inflammatory response, and wound healing, but is dysregulated in diseases like osteoarthritis, pulmonary fibrosis, and cardiovascular disease (Cox and Erler, 2011; Karamanos et al., 2019). A baseline level of collagen synthesis is carried out by the native cell population of a soft tissue, that is then kept in check by cellular signaling cascades and subsequent proteinase activity. This continuous process occurs even in the absence of any macroscopic topological changes to the tissue (Cox and Erler, 2011). In tendon, for example, fibroblasts produce procollagen, forming aggregates in the Golgi complex, before secretion into the ECM (Thankam et al., 2018). These procollagens are then subjected to a number of modifications before being self-assembled into a thermodynamically stable triple helical form (Canty and Kadler, 2005; Thankam et al., 2018). A thorough overview of collagen synthesis, trafficking, processing and fibrillogenesis is described elsewhere (Canty and Kadler, 2005). Collagen synthesis is kept in balance by MMP and other proteinase activity. MMPs are regulated by an additional set of enzymes called tissue inhibitors of metalloproteinases (TIMPs) (Brew and Nagase, 2010). Broader ECM synthesis and remodeling are also driven by cellular senescence (Campisi and d’Adda di Fagagna, 2007; Karamanos et al., 2019). The senescence-associated secretory phenotype (SASP) of fibroblasts can promote the proliferation of neighboring cells, instigate inflammatory responses, and initiate degradation of surrounding matrix via MMP production (Campisi and d’Adda di Fagagna, 2007; Karamanos et al., 2019).
6.2 Tissue Healing
Wound healing in soft tissues is characterized by phases of inflammation, re-epithelization or matrix deposition, and tissue remodeling (Hildebrand et al., 2005; Karamanos et al., 2019). Immediately after injury, hemostasis and coagulation take place, trafficking immune cells and initiating the inflammatory response (Velnar et al., 2009). Once the immune response initiates fibroblast-like and mesenchymal stem cell migration and activation/differentiation, the cellular population begins to form newly synthesized collagen and other ECM components (Hildebrand et al., 2005; Velnar et al., 2009). The deposited collagen not only provides initial mechanical stability to the wound, but also provides adhesion sites for cell migration (Keane et al., 2018). Deficits in this phase of the wound healing process result in chronic wounds and other pathologies (Wells et al., 2016; Karamanos et al., 2019). Skin is commonly taken as a representative example of soft tissue healing: the balance of collagens in uninjured skin is ∼80% collagen type I and 20% type III, whereas type III takes up about 40% of the newly synthesized matrix in this proliferative stage of healing (Velnar et al., 2009). Type III collagen itself is mechanically inferior to type I collagen, such that tissue in this stage of healing is characterized by a reduction in overall tissue mechanical properties (Voleti et al., 2012). Remodeling of the newly deposited matrix occurs in the final phase of tissue healing. Collagen type I synthesis dominates over type III synthesis, collagen bundles increase in diameter, and MMPs act to degrade the inferior, disorganized, temporary scar matrix in favor of more aligned and mature ECM (Velnar et al., 2009; Voleti et al., 2012). This remodeling process can take days to years depending on the tissue and species, and, in most cases, the scar tissue that has formed is only about 80% of the original tensile strength compared to the unwounded even in the best case scenario (Velnar et al., 2009; Voleti et al., 2012).
6.3 Fibrosis
Fibrosis is characterized by excessive deposition of ECM, namely collagen, in a dysregulated tissue homeostatic feedback loop that is often the result of an abnormal response to organ injury or other irritation. Fibrosis underlies pathology in nearly every tissue of the human body, like heart failure with cardiac fibrosis, progressive dyspnea with pulmonary fibrosis, liver cirrhosis and cancer (Cox and Erler, 2011; Li et al., 2018). In fibrosis, the balance in MMP and TIMP expression is disrupted, leading to a lack of matrix degradation, as well as increased collagen synthesis and upregulation of collagen crosslinking enzymes like LOX. The overproduction of new matrix coupled with degradation of other ECM components, leads to denser and stiffer tissues with an altered biochemical makeup compared to native (Cox and Erler, 2011). Each soft tissue’s resident cell type has a “stiffness phenotype,” meaning that function is driven, in part, by the normal amount of isometric tension or force cells experiences in situ (Butcher et al., 2009) Since cells within these tissues are mechanosensitive, cellular phenotype can change based on the underlying stiffness, leading to aberrations in behavior and eventual pathogenesis in stiff microenvironments (Cox and Erler, 2011). Loss of native cellular behavior creates a negative feedback loop where misfiring of signaling cascades take place, incomplete matrix remodeling occurs, and irreversible fibrosis takes over (Karamanos et al., 2019).
6.4 Cancer and Metastasis
Like fibrosis, cancer in soft tissues is in part characterized by dysfunction in the normal homeostatic collagen synthesis-remodeling feedback loop. Tumor stroma is compositionally, structurally and mechanically different from the normal stroma from which it previously arose (Cox and Erler, 2011). Tumors are much stiffer than native tissue, up to tenfold in breast tissue (1.5 kPa vs. 150 Pa) (Butcher et al., 2009; Cox and Erler, 2011). Increased stiffness is, in part, due to the increased relative collagen density within the cancerous tissue (Cox and Erler, 2011). Upregulation of LOX is also common in cancerous tissue and is even used as a marker for cancer invasiveness and prognosis in individuals with head and neck cancers (Le et al., 2009). Increased LOX-mediated crosslinking, fiber realignment, and stiffening of the stroma are common in pre-malignant tissue (Cox and Erler, 2011). Collagen fibers adjacent to the invasive front of a tumor become more aligned as tumor cells migrate along them to facilitate invasion and intravasation (Condeelis and Pollard, 2006). MMP activity is also typically high in tumorous tissue, acting to destroy native ECM that is then replaced with malignant tissue (Scherer et al., 2008). Finally, a hallmark of tumor tissue is increased micro-vascularization and angiogenesis compared to native (Heijblom et al., 2011).
In summary, collagenous tissues are dynamic structures that are in a constant state of anabolic new matrix deposition and catabolic remodeling. Pathology like fibrosis and cancer are caused when either or both processes become dysregulated. Excess matrix deposition leads to localized heterogeneous collagen buildups. Overactive crosslinking enzymes and under or overactive remodeling enzymes cause regional changes in fiber alignment and matrix connectivity. The heterogeneity in structure that develops in a largely homogenous tissue can be leveraged for non-invasive sensing and early detection in the progression of these pathologies. Further, as structure of collagenous tissues define the mechanical properties that support its overall function, the development of matrix heterogeneity typically correlates with deficits in local mechanics. How these two commonalities in pathology (i.e., matrix heterogeneity, local changes in mechanical properties) are exploited to optically image dysregulated collagen remodeling will be described in the following section.
7 Approaches for Imaging Collagen Remodeling/Repair
7.1 Imaging Collagen Heterogeneity During Tissue Remodeling
7.1.1 Polarimetry-Based Approaches
Like their utility in monitoring tissue damage, polarimetry techniques are uniquely suited to detect regions of heterogeneity in collagen concentration and alignment due to the change in total amount and anisotropy of birefringent material present. QPLI has been used to evaluate dermal wound healing and showed non-linear changes in the fiber orientation during the healing response, along with progressive increases in collagen fiber thickness (Figure 3B) (Woessner et al., 2019). Cancerous tissues are also associated with a loss of retardance and microstructural organization, such that they can be clearly differentiated from neighboring tissue using reflectance QPLI (Pierangelo et al., 2013; Alali and Vitkin, 2015). On the smallest scale, polarized light techniques has been used to differentiate between cancerous and non-cancerous cells in suspension (Hielscher et al., 1997). At the tissue level, polarimetry has been used to detect cancerous lesions of the cervix (Mourant et al., 2009), lung (Kunnen et al., 2015), breast (Dremin et al., 2020), and more (Nishizawa et al., 2021). Further, fibrosis is characterized by similar increases in overall collagen concentration, such that polarized light can be used to monitor progression of normal wound healing into pathological fibrosis. Fibrotic tissue also has an abnormally high ratio of collagen type III to collagen type I; these two collagen types can be detected with polarization SHG due to differences in relative birefringence (Campbell and Campagnola, 2017). Therefore, polarized light can be used to quantify the relative amounts of collagens to classify a region as fibrotic or healthy. However, as mentioned earlier, birefringence of form tends to dominate over intrinsic birefringence in tissue level polarization measurements. This can lead to difficulty in interpreting contribution of individual collagen types in wide-field polarization imaging applications. Further, the minimal instrumentation involved in many polarimetry based techniques lends itself well towards deployment in clinically based imaging techniques such as endoscopic integration for minimally invasive cancer and fibrosis detection (Solomon et al., 2011; Qi and Elson, 2016; Garcia and Gruev, 2017; Qi and Elson, 2017; Trout et al., 2022). Endoscopes have been developed that utilize PS-OCT, but these devices are unable to detect depolarization because of limitations associated with the interferometry used in OCT (Jiao et al., 2000; Qi and Elson, 2016).
7.1.2 Photoacoustic Imaging
PAI is a powerful imaging technique that combines the spatial resolution of typical optical microscopy techniques with the penetration depth of ultrasound. Infrared light typically irradiates a tissue of interest, causing a temperature and subsequent pressure rise (Tuchin, 2016). The pressure propagates from the optically excited tissue as an ultrasonic wave that is proportional to the optical absorption of the tissue (Lin and Wang, 2021). PAI is incredibly tunable, in that choice of optical wavelength and acoustic detection can change the spatiotemporal resolution and depth of penetration (Brown et al., 2019).
PAI has historically been used to visualize collagen in chronic fibrotic diseases like Crohn’s disease and atherosclerosis (Brown et al., 2019). As the PAI signal is driven by the absorption coefficient of the tissue region being probed, the wavelength of light chosen should be dominated by absorption of the component of interest (i.e., hemoglobin, lipid, collagen) (Figure 3C). For example, collagen’s absorption coefficient reaches a relative maximum around 1,300 nm, so studies using PAI for fibrosis have used this value as the probing wavelength for collagen investigation (Lei et al., 2016). At a wavelength of 1,300 nm, PAI was able to distinguish chronic fibrosis from acute inflammation in ex vivo rat colon (Lei et al., 2016).
A large advantage of PAI for applications in healing, fibrosis and cancer is in the imaging of newly developed microvasculature. Angiogenesis is a hallmark of tumor formation and wound healing that differentiates the cancerous or remodeled tissue from the surrounding stroma. Because of hemoglobin’s high absorption coefficient, absorption at critical wavelength ranges can be used to indirectly infer vascularity of tissue (Wang and Wu, 2012). PAI can also be endoscopically implemented in a methodology similar to polarimetry to allow for in vivo and in situ analysis of stromal vasculature (Yang et al., 2009). Similar phenomena are leveraged in diffuse optical spectroscopy and, at a higher length scale, diffuse optical tomography, to look at vascular networks, oxygen saturation and blood flow to tissues (Wang and Wu, 2012). Use of these techniques in combination with other more collagen fiber-centric imaging approaches could allow for unique insights into collagen remodeling as it relates to angiogenesis in healing, fibrosis, and cancer.
7.1.3 Confocal and Multiphoton Microscopy
There are many similarities in the use of 2P/confocal microscopy for imaging collagen damage and homeostasis/remodeling. SHG is uniquely suited for detection of heterogeneity in collagen concentration that are typical in pathologies like fibrosis and cancer because of the signal’s collagen specificity. The assessment of fibrosis using SHG has been performed in tissues like kidney, liver, and lung (Cox et al., 2003; Strupler et al., 2007; Gailhouste et al., 2010; Ricard-Blum et al., 2018). pSHG is well suited to monitor local changes in fiber alignment that are linked to cell migration and progression towards metastasis in cancer (Ricard-Blum et al., 2018). The combination of SHG with TPEF allows for label-free imaging of collagen density, alignment and crosslinking (Marturano et al., 2014). Additionally, there are exogenous labels that can allow for visualization of nascent ECM formation like functional noncanonical amino acid tagging (FUNCAT) (Mcleod and Mauck, 2016). These types of fluorescent probes allow for quantification of new matrix segmented out from existing ECM.
7.2 “Imaging” Local Mechanics During Tissue Remodeling
7.2.1 Brillouin Spectroscopy and Microscopy
Brillouin scattering is an inelastic scattering process (similar to Raman) that is due to acoustic fluctuations in the molecules of a tissue that can be related to its mechanical properties (Goth et al., 2016). Brillouin microscopy was only used in a biological context for the first time 15 years ago (Scarcelli and Yun, 2008). and is an emerging tool in mechanobiology that allows for 3D “imaging” of viscoelastic mechanical properties in a non-contact manner (Prevedel et al., 2019). By measuring the shift in the frequency of light after interacting with acoustic phonons, mechanical properties can be inferred and mapped volumetrically (Figure 3D) (Goth et al., 2016). Brillouin spectrometers have also been used in concert with fluorescence imaging to enable correlation of mechanics with fluorescently labelled microstructural elements (Elsayad et al., 2016).
As there are changes in local tissue stiffness associated with collagen deposition and crosslinking during remodeling and pathology, Brillouin could prove to be a powerful tool in evaluating these dynamic scenarios. For example, Brillouin spectroscopy has already been used as a tool in evaluating tumor margins in malignant melanoma (Troyanova-Wood et al., 2016). The measured output parameter from Brillouin is M’, the longitudinal inelastic storage modulus, which assumes no deformations in directions other than the probing direction. One limitation of the mechanical assessment provided by Brillouin spectroscopy is that in very hydrated tissues (e.g., hydrogels) where the incompressibility of water dominates the response, M’ does not correlate with the more common metric of Young’s modulus (Prevedel et al., 2019). For a comprehensive overview of Brillouin’s potential in mechanobiology, as well as a discussion of existing challenges in the biological translation of this technique, see Prevedel et al. (2019).
7.2.2 Optical Coherence Elastography
OCE is an OCT based imaging technique that is used to estimate local mechanical properties, similar to Brillouin spectroscopy. However, OCE uses a classical mechanics approach: as a compressive load is applied, the tissue level deformation is measured via OCT and mapped into an elastogram (Kennedy et al., 2017). OCE is similar to ultrasound or magnetic resonance elastography, but can attain a much higher spatiotemporal resolution (Liang et al., 2008). OCE has been shown to be able to distinguish between tumor and normal regions of breast tissue during ex vivo imaging (Liang et al., 2008). OCE also has been shown to be sensitive enough to monitor degradation of tendon tissue from enzymatic digestion (Guan et al., 2013), suggesting it may be able to visualize regions that have been locally degraded during the remodeling process.
8 Machine Learning Applications in Optical Imaging of Collagen
Machine learning (ML), a subset of artificial intelligence, has the potential to dramatically impact and augment optical imaging of soft tissues. ML algorithms are analytical methods that allow computers to learn patterns, predict, and accomplish tasks in a data-driven way. Developing ML algorithms is task/goal-based and often achieved through methods of supervised learning and unsupervised learning. In supervised learning, the input dataset has a labeled output, whereas in unsupervised learning, the input data set does not. Conventional ML algorithms (e.g., logistic regression, support vector machine, and random forest) and deep learning algorithms (e.g., neural networks such as U-Net) can be used for various applications related to imaging (e.g., segmentation, disease classification, and image registration). To date, ML approaches have been successfully used in several applications related to optical imaging of soft tissues including image segmentation, disease prediction/classification, multi-modal image registration, image enhancement, and tissue property prediction (Figure 4).
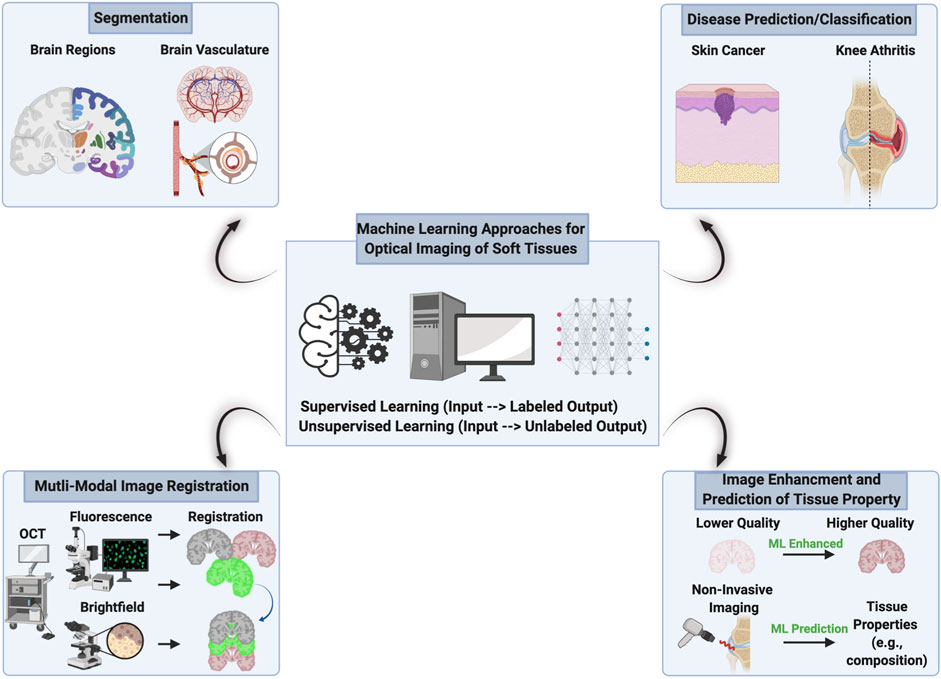
FIGURE 4. Machine learning approaches for optical imaging of soft tissues. This schematic summarizes some of the current and near-future work utilizing machine learning (ML) approaches for optical imaging of soft tissues. These applications of ML are not limited to those shown and will likely increase diversity over time as more researchers expand into this field. Created with BioRender.com.
ML approaches in optical imaging of soft tissues have largely been developed for (A) image segmentation and (B) disease classification. Image segmentation is a critical step in image analysis. Conventionally, the user must manually segment tissues or objects of interest within an image, which is prone to human error and bias, while also being laborious and time-consuming. Automatic image segmentation via classic image analysis techniques can help overcome some manual segmentation tasks but doesn’t always generalize well with normal variation between images in a dataset. ML approaches can help alleviate the manual burden required by humans and account for variation in the imaging data itself. To this end, ML has been used for segmentation in images of various tissues (e.g., brain, skin, heart, lung, liver, and cartilage) derived from microscopy techniques of fluorescence (Xiao et al., 2021; Qu et al., 2022), autofluorescence (Todorov et al., 2020), reflectance confocal (D’Alonzo et al., 2021), and bright-field (Rytky et al., 2021) imaging. Segmented regions of interest or the un-segmented original can be used for further quantitative image analysis and as inputs to ML algorithms to classify the disease state of the tissue. For disease classification, ML algorithms often use images as the model input and then output the probability of whether the tissue in the image is healthy or diseased. In this context, ML has been to classify disease state of various tissues (e.g., skin and cartilage) in images derived from ex vivo confocal microscopy (D’Alonzo et al., 2021; Ruini et al., 2021; Shavlokhova et al., 2021), second harmonic generation imaging (Saitou et al., 2018; Wang G. et al., 2021; Wang Q. et al., 2021), and near-infrared spectroscopy (Afara et al., 2020). Overall, the ability of ML to segment, quantify, and predict/classify diseases using optical imaging in the preclinical and clinical settings can profoundly improve the speed and accuracy of disease diagnosis and treatment prognosis.
ML has also been used in optical imaging of soft tissues to register (i.e., align) images from multiple imaging modalities to link data from different imaging techniques/length scales and accelerate discovery of disease mechanisms. For example, ML-based image registration has been applied in the mouse brain (fluorescence and magnetic resonance images (Xiao et al., 2021; Qu et al., 2022)) and for cells (confocal and wide-field images (Wang et al., 2019)). Once registered, images can be fused together to create new images/data and/or quantified separately. Notably, registering and combining images across modalities might enable the development of ML models to predict tissue information from one imaging modality to another, which might help overcome specialized ex vivo tissue processing required for cellular level information/diagnosis clinically. For instance, non-invasive optical imaging (e.g., OCT/reflectance confocal microscopy) can provide tissue-level information detail yet lacks cellular resolution, which requires tissues to be excised and processed for histological and immunohistochemical staining to determine cellular-level details. If images are aligned properly (with or without ML), pixel (e.g., intensity) or informational (e.g., pathologist’s assessment) level assessment might be used to develop a ML algorithm capable of predicting cellular information non-invasively. Towards the idea of linking images across different modalities, ML has also been used to obtain histological detail of various tissues (e.g., salivary gland, thyroid, kidney, liver and lung) from wide-field autofluorescence images (Rivenson et al., 2019). In addition, ML algorithms have been used to discern collagen from elastin fibers in Mueller matrix polarimetry images, which were trained using SHG/TPEF images (Roa et al., 2021). Beyond combining images from different modalities, imaging data can be combined with other tissue properties (e.g., composition or biomechanics) or clinically relevant biomarkers (e.g., patient pain or blood makers). Integrating data across imaging modalities and sources will increase the complexity that ML can accommodate and evaluate with non-linear and higher dimension analysis through techniques such as dimensionality reduction and unsupervised learning (e.g., principal component analysis coupled with k-means clustering). Collectively, combining data across modalities and data types might unlock disease patterns that humans do not think of or naturally comprehend (e.g., multiply higher-order dimensions).
Other uses of ML have included image enhancement and prediction of tissue properties from imaging data. Image enhancement (i.e., obtaining higher quality images from lower resolution images) is important because it may reduce the image objectives, resolution, and power necessary to obtain high quality images. In this context, ML has been used to obtain high resolution confocal data from lower resolution wide-field images for cells (Wang et al., 2019), which can be easily extended to many other imaging modalities and tissues. Lastly, prediction of tissue properties from imaging modalities would be quite useful in the clinical setting to enable better assessment of tissue quality/disease stage. In one study, ML was used to predict articular cartilage composition and functional integrity using near-infrared imaging (Kafian-Attari et al., 2020).
Ultimately, ML for optical imaging of soft tissues can be transformative for a wide range of purposes; yet there are aspects worth considering that can impact the success and generalizability of ML algorithms for each imaging modality, task, and tissue type. One should consider the appropriate training/testing data needed to develop ML algorithms for each purpose, since there is not a one-size-fits-all ML algorithm. For example, aspects of training/testing data include the data type (e.g., images and/or numerical values), number of train/test samples, image properties input size/aspect ratio, magnification/resolution, and sample variation (e.g., images quality and disease severity), and any image pre- or post-processing (e.g., smoothing, scaling, padding, color normalization or additional filtering). Many of these aspects are often determined by trial and error during development, and the accuracy/success of a ML is task dependent. Besides the appropriate training/test data, it’s important to consider that different ML algorithms might need to be developed based on whether image data is 1) from an in vitro, ex vivo, or in vivo imaging configuration, 2) in two or three dimensions, 3) from one or multiple modalities, and 4) acquired in real-time imaging or via post-mortem analysis. Additionally, computational capabilities might represent a rate limiting aspect of ML development, particularly for ML algorithms that utilize computationally demanding networks (e.g., convolutional neuron networks). Importantly, while ML approaches might eventually replace the manual burden of image analysis in the optical imaging of soft tissues, there will still likely be a need for domain expertise in the field (e.g., tissue-specific pathologist) to help drive the development and validation of ML approaches. Overall, these considerations are paramount for generating appropriate data to develop ML algorithms and ensuring their ability to generalize and scale successfully from preclinical studies to clinical applications.
While ML might have tremendous benefits for optical imaging of soft tissues, there are some notable limitations and barriers in the development and deployment of ML in this context. First and foremost, generating the appropriate data and the labeling required for ground truth in supervised learning that many applications utilize is labor intensive, time consuming, and subject to the accuracy of human user. For smaller research groups, this can be a large barrier to overcome. Professional companies or crowdsourcing (via Amazon Mechanical Turk or other platforms) could be beneficial from a labor/time perspective but might introduce inconsistency in labeling or tracing of training/testing samples, impacting the accuracy of ML models. Labeling or tracing might need to be performed by specialists (e.g., radiologist or pathologist), which can present access issues too. Besides concerns with human input, training data might be limited by sample availability and/or unbalanced classes/groups (e.g., many more samples of healthy compared to diseased tissue), which can induce lack of generalizability to new data sets or biases in an algorithm (i.e., over or underfitting). Often, there is limited a priori knowledge about the number of samples needed or the appropriate level of accuracy deemed successful for each ML task; ultimately, this is likely best determined on a case-by-case basis with continuous reevaluation. Limitations in sample numbers and data variance can be overcome somewhat by data augmentation techniques (i.e., creating images or data by artificial means like data averaging and image rotation/translation). In situations where access to sufficient training/testing data is limited, transfer learning approaches (i.e., retraining a pretrained network for another purpose) can also be helpful in allowing for faster ML development. Furthermore, one limitation, particularly in applications using deep learning/neural networks, is the interpretability of “black box” decisions arrived at by the algorithm for a particular task. For instance, the unique features of an image (i.e., tissue properties) that enable successful U-Net segmentation are unknown to the user; however, while not fully accessible, some aspects of these properties may be explored using activation layers at different steps of the algorithm. Lastly, some limitations might provide challenges based on whether ML tasks are for preclinical or clinical imaging applications (e.g., clinical data may contain sensitive patient information). Overall, limitations in implementing ML approaches in optical imaging analysis might only be applicable for specific problems; furthermore, solutions to these challenges will likely become available as the use of ML continues to develop and expand.
9 Opportunities and Remarks
Collagen is a protein that is essential to function in nearly every tissue in the human body. Its hierarchical nature makes visualizing structure difficult to accomplish across all the physiological relevant length scales, as no one modality can attain multiscale fields of view at sufficient spatiotemporal resolution. This is problematic as damage, remodeling and repair can be present at the molecular scale before manifestation at the tissue level. Therefore, multiscale imaging approaches are pivotal in the development of clinically relevant diagnostic tools and monitoring systems. The tunable focusing optics of techniques like polarimetry, photoacoustic tomography, and optical coherence tomography can be leveraged to create systems that are capable of gleaning insights with a single system, minimizing the total instrumentation required to attain multiscale analysis. Such an approach would be advantageous compared to needing multiple systems (e.g., multiphoton and SALS to see micro and macrostructure), which is generally more expensive and time consuming.
Even within a single length scale, there are great advantages associated with combination of multi-modality approaches to imaging collagen dynamics. One successful example is the augmentation of polarized light imaging with SHG, OCT and histology. Polarized light techniques allow for addition of orientation information to be gleaned with the addition of just a few optical filters in the optical train. Another advantage of multimodality approaches would be the possible opportunity for collagen-targeted theranostic approaches (Wahyudi et al., 2016). With the advent and widespread use of collagen targeting probes like CHP and CNA35 to label denatured and intact collagen, respectively, comes opportunities for conjugation of therapeutic agents that can directly target collagen damage or pathology. Use of these theranostic agents will likely require multimodality systems to localize and visualize targeting agents, while simultaneously monitoring and managing the systemic response.
Further, with the delicate interplay between structure, composition, and function of soft tissues, it logically follows that imaging approaches that offer coherence between monitoring mechanical properties and structure are of the utmost importance. Emerging elastography techniques serve to bridge the gap between structure and function that are crucial when evaluating a therapeutic’s effect on restoring quality of life clinically or evaluating disease severity. The combination of polarization sensitive techniques with elastographic approaches, like in polarization sensitive optical coherence elastography (PS-OCE), allows for visualization of dynamic microstructure with changes in local mechanics that provide unique insights into tissue function (Miyazawa et al., 2019).
In the future, ML-based approaches are going to play a significant role in bridging gaps across modalities used in multiscale, multimodality and multiproperty imaging techniques. Data registration and novel segmentation techniques will allow for unique aggregation of data sets and quantification methods that can hopefully allow for unique insights into disease progression.
Collagen structure across the length scales underpins function in nearly every tissue of the human body. Therefore, the ability to visualize collagen and quantify dynamic processes are critical in maintaining and understanding human health. Optical approaches offer the best spatiotemporal resolution for non-invasive imaging of collagen. A summary comparing some of the major imaging modalities explored in this review can be seen in Table 1. Damage to collagen is characterized by a loss in triple helical structure that manifests in changes in optical properties associated with collagen (i.e., scattering, birefringence) that can be monitored with polarimetry-based techniques, optical coherence tomography, and with exogenous fluorescent probes like CHP in concert with multiphoton imaging and SHG. Imaging modalities that can discern local differences in collagen density, alignment, and crosslinking are uniquely suited for detecting aberrations in the remodeling and repair processes in collagenous tissues and progression towards pathology. Further, elastography techniques like Brillouin microscopy that allow for “imaging” of local mechanical properties also provide approaches that may prove useful in linking structure-function relationships in both normal and pathological tissue. Emerging machine learning approaches aimed at improving diagnostic ability, data quality, and bridging knowledge from both optical and non-optical modalities, offer new avenues in exploring collagen dynamics in pre-clinical and clinical applications.
Author Contributions
LI and SL developed the idea for the review. The article was researched, written, edited, and approved by LI, CD, MD, and SL.
Funding
The authors would like to acknowledge funding support from NIH T32EB014855 (LI) and NSF 1761561 (SL).
Conflict of Interest
The authors declare that the research was conducted in the absence of any commercial or financial relationships that could be construed as a potential conflict of interest.
Publisher’s Note
All claims expressed in this article are solely those of the authors and do not necessarily represent those of their affiliated organizations, or those of the publisher, the editors and the reviewers. Any product that may be evaluated in this article, or claim that may be made by its manufacturer, is not guaranteed or endorsed by the publisher.
References
Afara, I. O., Sarin, J. K., Ojanen, S., Finnilä, M. A. J., Herzog, W., Saarakkala, S., et al. (2020). Machine Learning Classification of Articular Cartilage Integrity Using Near Infrared Spectroscopy. Cell Mol. Bioeng. 13, 219–228. doi:10.1007/s12195-020-00612-5
Akkus, O., Belaney, R. M., and Das, P. (2005). Free Radical Scavenging Alleviates the Biomechanical Impairment of Gamma Radiation Sterilized Bone Tissue. J. Orthop. Res. 23, 838–845. doi:10.1016/J.ORTHRES.2005.01.007
Alali, S., and Vitkin, A. (2015). Polarized Light Imaging in Biomedicine: Emerging Mueller Matrix Methodologies for Bulk Tissue Assessment. J. Biomed. Opt. 20, 061104. doi:10.1117/1.jbo.20.6.061104
Barnum, C. E., Fey, J. L., Weiss, S. N., Barila, G., Brown, A. G., Connizzo, B. K., et al. (2017). Tensile Mechanical Properties and Dynamic Collagen Fiber Re-Alignment of the Murine Cervix are Dramatically Altered throughout Pregnancy. J. Biomech. Eng. 139, 061008. doi:10.1115/1.4036473
Baues, M., Klinkhammer, B. M., Ehling, J., Gremse, F., van Zandvoort, M. A. M. J., Reutelingsperger, C. P. M., et al. (2020). A Collagen-Binding Protein Enables Molecular Imaging of Kidney Fibrosis In Vivo. Kidney Int. 97, 609–614. doi:10.1016/j.kint.2019.08.029
Baumann, B. (2017). Polarization Sensitive Optical Coherence Tomography: A Review of Technology and Applications. Appl. Sci. 7, 474. doi:10.3390/APP7050474
Bennink, L. L., Li, Y., Kim, B., Shin, I. J., San, B. H., Zangari, M., et al. (2018). Visualizing Collagen Proteolysis by Peptide Hybridization: From 3D Cell Culture to In Vivo Imaging. Biomaterials 183, 67–76. doi:10.1016/J.BIOMATERIALS.2018.08.039
Bianchi, F., Hofmann, F., Smith, A. J., and Thompson, M. S. (2016). Probing Multi-Scale Mechanical Damage in Connective Tissues Using X-Ray Diffraction. Acta Biomater. 45, 321–327. doi:10.1016/J.ACTBIO.2016.08.027
Bielajew, B. J., Hu, J. C., and Athanasiou, K. A. (2020). Collagen: Quantification, Biomechanics and Role of Minor Subtypes in Cartilage. Nat. Rev. Mater. 5, 730–747. doi:10.1038/s41578-020-0213-1
Billiar, K. L., and Sacks, M. S. (1997). A Method to Quantify the Fiber Kinematics of Planar Tissues Under Biaxial Stretch. J. Biomech. 30, 753–756. doi:10.1016/S0021-9290(97)00019-5
Boerboom, R. A., Krahn, K. N., Megens, R. T. A., van Zandvoort, M. A. M. J., Merkx, M., and Bouten, C. V. C. (2007). High Resolution Imaging of Collagen Organisation and Synthesis Using a Versatile Collagen Specific Probe. J. Struct. Biol. 159, 392–399. doi:10.1016/J.JSB.2007.04.008
Brew, K., and Nagase, H. (2010). The Tissue Inhibitors of Metalloproteinases (TIMPs): An Ancient Family with Structural and Functional Diversity. Biochim. Biophys. Acta - Mol. Cell Res. 1803, 55–71. doi:10.1016/J.BBAMCR.2010.01.003
Brockbank, K. G. M., MacLellan, W. R., Xie, J., Hamm-Alvarez, S. F., Chen, Z. Z., and Schenke-Layland, K. (2008). Quantitative Second Harmonic Generation Imaging of Cartilage Damage. Cell Tissue Bank 9, 299–307. doi:10.1007/s10561-008-9070-7
Brown, E., Brunker, J., and Bohndiek, S. E. (2019). Photoacoustic Imaging as a Tool to Probe the Tumour Microenvironment. Dis. Model. Mech. 12, dmm039636. doi:10.1242/dmm.039636
Buchanan, J. K., Zhang, Y., Holmes, G., Covington, A. D., and Prabakar, S. (2019). Role of X‐Ray Scattering Techniques in Understanding the Collagen Structure of Leather. ChemistrySelect 4, 14091–14102. doi:10.1002/SLCT.201902908
Buckley, M. R., Sarver, J. J., Freedman, B. R., and Soslowsky, L. J. (2013). The Dynamics of Collagen Uncrimping and Lateral Contraction in Tendon and the Effect of Ionic Concentration. J. Biomech. 46, 2242–2249. doi:10.1016/J.JBIOMECH.2013.06.029
Butcher, D. T., Alliston, T., and Weaver, V. M. (2009). A Tense Situation: Forcing Tumour Progression. Nat. Rev. Cancer 9, 108–122. doi:10.1038/NRC2544
Campbell, I. C., Sherwood, J. M., Overby, D. R., Hannon, B. G., Read, A. T., Raykin, J., et al. (2018). “Quantification of Scleral Biomechanics and Collagen Fiber Alignment,” in Glaucoma (New York, NY: Humana Press), 135–159. doi:10.1007/978-1-4939-7407-8_13
Campbell, K. R., and Campagnola, P. J. (2017). Wavelength-Dependent Second Harmonic Generation Circular Dichroism for Differentiation of Col I and Col III Isoforms in Stromal Models of Ovarian Cancer Based on Intrinsic Chirality Differences. J. Phys. Chem. B 121, 1749–1757. doi:10.1021/ACS.JPCB.6B06822
Campisi, J., and d’Adda di Fagagna, F. (2007). Cellular Senescence: when Bad Things Happen to Good Cells. Nat. Rev. Mol. Cell Biol. 8, 729–740. doi:10.1038/nrm2233
Canty, E. G., and Kadler, K. E. (2005). Procollagen Trafficking, Processing and Fibrillogenesis. J. Cell Sci. 118, 1341–1353. doi:10.1242/jcs.01731
Chakraborty, N., Wang, M., Solocinski, J., Kim, W., and Argento, A. (2016). Imaging of Scleral Collagen Deformation Using Combined Confocal Raman Microspectroscopy and Polarized Light Microscopy Techniques. PLoS One 11, e0165520. doi:10.1371/JOURNAL.PONE.0165520
Chen, J., Kim, J., Shao, W., Schlecht, S. H., Baek, S. Y., Jones, A. K., et al. (2019). An Anterior Cruciate Ligament Failure Mechanism. Am. J. Sports Med. 47, 2067–2076. doi:10.1177/0363546519854450
Condeelis, J., and Pollard, J. W. (2006). Macrophages: Obligate Partners for Tumor Cell Migration, Invasion, and Metastasis. Cell 124, 263–266. doi:10.1016/J.CELL.2006.01.007
Converse, M. I., Walther, R. G., Ingram, J. T., Li, Y., Yu, S. M., and Monson, K. L. (2018). Detection and Characterization of Molecular-Level Collagen Damage in Overstretched Cerebral Arteries. Acta Biomater. 67, 307–318. doi:10.1016/J.ACTBIO.2017.11.052
Coudrillier, B., Pijanka, J. K., Jefferys, J. L., Goel, A., Quigley, H. A., Boote, C., et al. (2015). Glaucoma-Related Changes in the Mechanical Properties and Collagen Micro-Architecture of the Human Sclera. PLoS One 10, e0131396. doi:10.1371/JOURNAL.PONE.0131396
Cox, G., Kable, E., Jones, A., Fraser, I., Manconi, F., and Gorrell, M. D. (2003). 3-Dimensional Imaging of Collagen Using Second Harmonic Generation. J. Struct. Biol. 141, 53–62. doi:10.1016/S1047-8477(02)00576-2
Cox, T. R., and Erler, J. T. (2011). Remodeling and Homeostasis of the Extracellular Matrix: Implications for Fibrotic Diseases and Cancer. Dis. Model. Mech. 4, 165–178. doi:10.1242/DMM.004077
Cui, S.-J., Fu, Y., Liu, Y., Kou, X.-X., Zhang, J.-N., Gan, Y.-H., et al. (2019). Chronic Inflammation Deteriorates Structure and Function of Collagen Fibril in Rat Temporomandibular Joint Disc. Int. J. Oral Sci. 11, 2. doi:10.1038/s41368-018-0036-8
D’Alonzo, M., Bozkurt, A., Alessi-Fox, C., Gill, M., Brooks, D. H., Rajadhyaksha, M., et al. (2021). Semantic Segmentation of Reflectance Confocal Microscopy Mosaics of Pigmented Lesions Using Weak Labels. Sci. Rep. 11, 3679. doi:10.1038/s41598-021-82969-9
Dayan, D., Hiss, Y., Hirshberg, A., Bubis, J. J., and Wolman, M. (1989). Are the Polarization Colors of Picrosirius Red-Stained Collagen Determined Only by the Diameter of the Fibers? Histochemistry 93, 27–29. doi:10.1007/BF00266843
Dong, Y., He, H., Sheng, W., Wu, J., and Ma, H. (2017). A Quantitative and Non-Contact Technique to Characterise Microstructural Variations of Skin Tissues during Photo-Damaging Process Based on Mueller Matrix Polarimetry. Sci. Rep. 7, 14702. doi:10.1038/s41598-017-14804-z
Dremin, V. V., Anin, D., Sieryi, O., Borovkova, M. A., Näpänkangas, J., Meglinski, I. V., et al. (2020). “Imaging of Early Stage Breast Cancer with Circularly Polarized Light,” in Tissue Optics and Photonics, Strasbourg, France (Bellingham, Washington: International Society for Optics and Photonics), 1136304. doi:10.1117/12.2554166
Eekhoff, J. D., Fang, F., and Lake, S. P. (2018). Multiscale Mechanical Effects of Native Collagen Cross-Linking in Tendon. Connect. Tissue Res. 59, 410–422. doi:10.1080/03008207.2018.1449837
Eleswarapu, S. V., Responte, D. J., and Athanasiou, K. A. (2011). Tensile Properties, Collagen Content, and Crosslinks in Connective Tissues of the Immature Knee Joint. PLoS One 6, e26178. doi:10.1371/JOURNAL.PONE.0026178
Elsayad, K., Werner, S., Gallemí, M., Kong, J., Sánchez Guajardo, E. R., Zhang, L., et al. (2016). Mapping the Subcellular Mechanical Properties of Live Cells in Tissues with Fluorescence Emission-Brillouin Imaging. Sci. Signal. 9, rs5. doi:10.1126/scisignal.aaf6326
Fessel, G., Li, Y., Diederich, V., Guizar-Sicairos, M., Schneider, P., Sell, D. R., et al. (2014). Advanced Glycation End-Products Reduce Collagen Molecular Sliding to Affect Collagen Fibril Damage Mechanisms but Not Stiffness. PLoS One 9, e110948. doi:10.1371/journal.pone.0110948
Gailhouste, L., Grand, Y. L., Odin, C., Guyader, D., Turlin, B., Ezan, F., et al. (2010). Fibrillar Collagen Scoring by Second Harmonic Microscopy: A New Tool in the Assessment of Liver Fibrosis. J. Hepatol. 52, 398–406. doi:10.1016/J.JHEP.2009.12.009
Garcia, M., and Gruev, V. (2017). Optical Characterization of Rigid Endoscopes and Polarization Calibration Methods. Opt. Express 25, 15713–15728. doi:10.1364/OE.25.015713
Garofalo, R., Cesari, E., Vinci, E., and Castagna, A. (2011). Role of Metalloproteinases in Rotator Cuff Tear. Sports Med. Arthrosc. 19, 207–212. doi:10.1097/JSA.0B013E318227B07B
Gaul, R. T., Nolan, D. R., and Lally, C. (2018). The Use of Small Angle Light Scattering in Assessing Strain Induced Collagen Degradation in Arterial Tissue Ex Vivo. J. Biomech. 81, 155–160. doi:10.1016/J.JBIOMECH.2018.10.006
Gelse, K., Pöschl, E., and Aigner, T. (2003). Collagens-structure, Function, and Biosynthesis. Adv. Drug Deliv. Rev. 55, 1531–1546. doi:10.1016/J.ADDR.2003.08.002
Ghosh, N., and Vitkin, A. I. (2011). Tissue Polarimetry: Concepts, Challenges, Applications, and Outlook. J. Biomed. Opt. 16, 110801. doi:10.1117/1.3652896
Ghosh, N., Wood, M., and Vitkin, A. (2010). “Polarized Light Assessment of Complex Turbid Media Such as Biological Tissues Using Mueller Matrix Decomposition,” in Handbook of Photonics for Biomedical Science (Boca Raton, FL: CRC Press), 253–282. doi:10.1201/9781439806296
Goth, W., Lesicko, J., Sacks, M. S., and Tunnell, J. W. (2016). Optical-Based Analysis of Soft Tissue Structures. Annu. Rev. Biomed. Eng. 18, 357–385. doi:10.1146/annurev-bioeng-071114-040625
Goth, W., Potter, S., Allen, A. C. B., Zoldan, J., Sacks, M. S., and Tunnell, J. W. (2019). Non-Destructive Reflectance Mapping of Collagen Fiber Alignment in Heart Valve Leaflets. Ann. Biomed. Eng. 47, 1250–1264. doi:10.1007/s10439-019-02233-0
Guan, G., Li, C., Ling, Y., Yang, Y., Vorstius, J. B., Keatch, R. P., et al. (2013). Quantitative Evaluation of Degenerated Tendon Model Using Combined Optical Coherence Elastography and Acoustic Radiation Force Method. J. Biomed. Opt. 18, 111417. doi:10.1117/1.JBO.18.11.111417
Gusachenko, I., Tran, V., Houssen, Y. G., Allain, J.-M., and Schanne-Klein, M.-C. (2012). Polarization-Resolved Second-Harmonic Generation in Tendon upon Mechanical Stretching. Biophysical J. 102, 2220–2229. doi:10.1016/J.BPJ.2012.03.068
He, C., He, H., Chang, J., Chen, B., Ma, H., and Booth, M. J. (2021). Polarisation Optics for Biomedical and Clinical Applications: A Review. Light Sci. Appl. 10, 2047–7538. doi:10.1038/s41377-021-00639-x
Heijblom, M., Klaase, J. M., van den Engh, F. M., van Leeuwen, T. G., Steenbergen, W., and Manohar, S. (2011). Imaging Tumor Vascularization for Detection and Diagnosis of Breast Cancer. Technol. Cancer Res. Treat. 10, 607–623. doi:10.7785/TCRT.2012.500227
Henao-Murillo, L., Ito, K., and van Donkelaar, C. C. (2018). Collagen Damage Location in Articular Cartilage Differs if Damage Is Caused by Excessive Loading Magnitude or Rate. Ann. Biomed. Eng. 46, 605–615. doi:10.1007/s10439-018-1986-x
Hielscher, A., Eick, A., Mourant, J., Shen, D., Freyer, J., and Bigio, I. (1997). Diffuse Backscattering Mueller Matricesof Highly Scattering media. Opt. Express 1, 441–453. doi:10.1364/OE.1.000441
Highberger, J. H., Gross, J., and Schmitt, F. O. (1951). The Interaction of Mucoprotein with Soluble Collagen; an Electron Microscope Study. Proc. Natl. Acad. Sci. 37, 286–291. doi:10.1073/pnas.37.5.286
Hildebrand, K. A., Gallant-Behm, C. L., Kydd, A. S., and Hart, D. A. (2005). The Basics of Soft Tissue Healing and General Factors that Influence Such Healing. Sports Med. Arthrosc. 13, 136–144. doi:10.1097/01.JSA.0000173230.61276.F4
Hoy, R. C., D'Erminio, D. N., Krishnamoorthy, D., Natelson, D. M., Laudier, D. M., Illien‐Jünger, S., et al. (2020). Advanced Glycation End Products Cause RAGE ‐Dependent Annulus Fibrosus Collagen Disruption and Loss Identified Using In Situ Second Harmonic Generation Imaging in Mice Intervertebral Disk In Vivo and in Organ Culture Models. JOR Spine 3, e1126. doi:10.1002/JSP2.1126
Hwang, J., Huang, Y., Burwell, T. J., Peterson, N. C., Connor, J., Weiss, S. J., et al. (2017a). In Situ Imaging of Tissue Remodeling with Collagen Hybridizing Peptides. ACS Nano 11, 9825–9835. doi:10.1021/acsnano.7b03150
Hwang, J., San, B. H., Turner, N. J., White, L. J., Faulk, D. M., Badylak, S. F., et al. (2017b). Molecular Assessment of Collagen Denaturation in Decellularized Tissues Using a Collagen Hybridizing Peptide. Acta Biomater. 53, 268–278. doi:10.1016/J.ACTBIO.2017.01.079
Hysi, E., He, X., Fadhel, M. N., Zhang, T., Krizova, A., Ordon, M., et al. (2020). Photoacoustic Imaging of Kidney Fibrosis for Assessing Pretransplant Organ Quality. JCI Insight 5, e136995. doi:10.1172/JCI.INSIGHT.136995
Ignatieva, N. Y., Zakharkina, O. L., Andreeva, I. V., Sobol, E. N., Kamensky, V. A., Myakov, A. V., et al. (2007). IR Laser and Heat-Induced Changes in Annulus Fibrosus Collagen Structure. Photochem. Photobiol. 83, 675–685. doi:10.1111/J.1751-1097.2007.072.X
Iqbal, S. M. A., Deska-Gauthier, D., and Kreplak, L. (2019). Assessing Collagen Fibrils Molecular Damage after a Single Stretch-Release Cycle. Soft Matter 15, 6237–6246. doi:10.1039/C9SM00832B
Jacques, S. L. (2013). Optical Properties of Biological Tissues: A Review. Phys. Med. Biol. 58, R37–R61. doi:10.1088/0031-9155/58/11/R37
Jiao, S., Yao, G., and Wang, L. V. (2000). Depth-resolved Two-Dimensional Stokes Vectors of Backscattered Light and Mueller Matrices of Biological Tissue Measured with Optical Coherence Tomography. Appl. Opt. 39, 6318–6324. doi:10.1364/AO.39.006318
Kafian-Attari, I., Nippolainen, E., Semenov, D., Hauta-Kasari, M., Töyräs, J., and Afara, I. O. (2020). Tissue Optical Properties Combined with Machine Learning Enables Estimation of Articular Cartilage Composition and Functional Integrity. Biomed. Opt. Express 11, 6480. doi:10.1364/BOE.402929
Karamanos, N. K., Theocharis, A. D., Neill, T., and Iozzo, R. V. (2019). Matrix Modeling and Remodeling: A Biological Interplay Regulating Tissue Homeostasis and Diseases. Matrix Biol. 75-76, 1–11. doi:10.1016/J.MATBIO.2018.08.007
Keane, T. J., Horejs, C.-M., and Stevens, M. M. (2018). Scarring vs. Functional Healing: Matrix-Based Strategies to Regulate Tissue Repair. Adv. Drug Deliv. Rev. 129, 407–419. doi:10.1016/J.ADDR.2018.02.002
Kennedy, B. F., Wijesinghe, P., and Sampson, D. D. (2017). The Emergence of Optical Elastography in Biomedicine. Nat. Photon 11, 215–221. doi:10.1038/nphoton.2017.6
Kim, B.-M., Eichler, J. r., Reiser, K. M., Rubenchik, A. M., and Da Silva, L. B. (2000). Collagen Structure and Nonlinear Susceptibility: Effects of Heat, Glycation, and Enzymatic Cleavage on Second Harmonic Signal Intensity. Lasers Surg. Med. 27, 329–335. doi:10.1002/1096-9101(2000)27:4<329::aid-lsm5>3.0.co;2-c
Kirby, M. A., Heuerman, A. C., and Yellon, S. M. (2018). Utility of Optical Density of Picrosirius Red Birefringence for Analysis of Cross-Linked Collagen in Remodeling of the Peripartum Cervix for Parturition. Integr. Gynecol. Obstet. J. 1 (2(, 1–9. doi:10.31038/IGOJ.2018107
Kirkpatrick, N. D., Hoying, J. B., Botting, S. K., Weiss, J. A., and Utzinger, U. (2006). In Vitro model for Endogenous Optical Signatures of Collagen. J. Biomed. Opt. 11, 054021. doi:10.1117/1.2360516
Kondratko-Mittnacht, J., Lakes, R., and Vanderby, R. (2015). Shear Loads Induce Cellular Damage in Tendon Fascicles. J. Biomech. 48, 3299–3305. doi:10.1016/J.JBIOMECH.2015.06.006
Kunnen, B., Macdonald, C., Doronin, A., Jacques, S., Eccles, M., and Meglinski, I. (2015). Application of Circularly Polarized Light for Non-invasive Diagnosis of Cancerous Tissues and Turbid Tissue-like Scattering media. J. Biophoton 8, 317–323. doi:10.1002/JBIO.201400104
Lake, S. P., Liu, Q., Xing, M., Iannucci, L. E., Wang, Z., and Zhao, C. (2020). “Tendon and Ligament Tissue Engineering,” in Principles of Tissue Engineering. Editors R. Lanza, R. Langer, and J. P. Vacanti (Cambridge, MA: Academic Press), 989–1005. doi:10.1016/B978-0-12-818422-6.00056-3
Lake, S. P., Miller, K. S., Elliott, D. M., and Soslowsky, L. J. (2009). Effect of Fiber Distribution and Realignment on the Nonlinear and Inhomogeneous Mechanical Properties of Human Supraspinatus Tendon Under Longitudinal Tensile Loading. J. Orthop. Res. 27, 1596–1602. doi:10.1002/jor.20938
Lake, S. P., Miller, K. S., Elliott, D. M., and Soslowsky, L. J. (2010). Tensile Properties and Fiber Alignment of Human Supraspinatus Tendon in the Transverse Direction Demonstrate Inhomogeneity, Nonlinearity, and Regional Isotropy. J. Biomech. 43, 727–732. doi:10.1016/j.jbiomech.2009.10.017
Långsjö, T. K., Rieppo, J., Pelttari, A., Oksala, N., Kovanen, V., and Helminen, H. J. (2002). Collagenase-Induced Changes in Articular Cartilage as Detected by Electron-Microscopic Stereology, Quantitative Polarized Light Microscopy and Biochemical Assays. Cells Tissues Organs 172, 265–275. doi:10.1159/000067196
Laurence, D. W., Homburg, H., Yan, F., Tang, Q., Fung, K.-M., Bohnstedt, B. N., et al. (2021). A Pilot Study on Biaxial Mechanical, Collagen Microstructural, and Morphological Characterizations of a Resected Human Intracranial Aneurysm Tissue. Sci. Rep. 11, 1–15. doi:10.1038/s41598-021-82991-x
Le, Q.-T., Harris, J., Magliocco, A. M., Kong, C. S., Diaz, R., Shin, B., et al. (2009). Validation of Lysyl Oxidase as a Prognostic Marker for Metastasis and Survival in Head and Neck Squamous Cell Carcinoma: Radiation Therapy Oncology Group Trial 90-03. J. Clin. Oncol. 27, 4281–4286. doi:10.1200/JCO.2008.20.6003
Le, V.-H., Lee, S., Kim, B., Yoon, Y., Yoon, C. J., Chung, W. K., et al. (2015). Correlation Between Polarization Sensitive Optical Coherence Tomography and Second Harmonic Generation Microscopy in Skin. Biomed. Opt. Express 6, 2542–2551. doi:10.1364/BOE.6.002542
Lei, H., Johnson, L. A., Liu, S., Moons, D. S., Ma, T., Zhou, Q., et al. (2016). Characterizing Intestinal Inflammation and Fibrosis in Crohn’s Disease by Photoacoustic Imaging: Feasibility Study. Biomed. Opt. Express 7, 2837–2848. doi:10.1364/BOE.7.002837
Li, L., Zhao, Q., and Kong, W. (2018). Extracellular Matrix Remodeling and Cardiac Fibrosis. Matrix Biol. 68-69, 490–506. doi:10.1016/J.MATBIO.2018.01.013
Liang, X., Oldenburg, A. L., Crecea, V., Chaney, E. J., and Boppart, S. A. (2008). Optical Micro-scale Mapping of Dynamic Biomechanical Tissue Properties. Opt. Express 16, 11052–11065. doi:10.1364/OE.16.011052
Lin, L., and Wang, L. V. (2021). “Photoacoustic Imaging,” in Optical Imaging in Human Disease and Biological Research. Editors X. Wei, and B. Gu (Singapore: Springer), 147–175. doi:10.1007/978-981-15-7627-0_8
Lutz, V., Sattler, M., Gallinat, S., Wenck, H., Poertner, R., and Fischer, F. (2012). Impact of Collagen Crosslinking on the Second Harmonic Generation Signal and the Fluorescence Lifetime of Collagen Autofluorescence. Skin Res. Technol. 18, 168–179. doi:10.1111/J.1600-0846.2011.00549.X
Maitland, D. J., and Walsh, J. T. (1997). Quantitative Measurements of Linear Birefringence During Heating of Native Collagen. Lasers Surg. Med. 20, 310–318. doi:10.1002/(sici)1096-9101(1997)20:3<310:aid-lsm10>3.0.co;2-h
Mamalis, A., Ho, D., and Jagdeo, J. (2015). Optical Coherence Tomography Imaging of Normal, Chronologically Aged, Photoaged and Photodamaged Skin. Dermatol. Surg. 41, 993–1005. doi:10.1097/DSS.0000000000000457
Marcu, L., French, P. M. W., and Son, D. S. (2015). Fluorescence Lifetime Spectroscopy and Imaging. Boca Raton, FL: CRC Press. doi:10.1201/b17018
Marshall, L., Tarakanova, A., Szarek, P., and Pierce, D. M. (2020). Cartilage and Collagen Mechanics under Large-Strain Shear within In Vivo and at Supraphysiogical Temperatures. J. Mech. Behav. Biomed. Mater. 103, 103595. doi:10.1016/J.JMBBM.2019.103595
Martin, C., and Sun, W. (2015). Fatigue Damage of Collagenous Tissues: Experiment, Modeling and Simulation Studies. J. Long. Term. Eff. Med. Implants 25, 55–73. doi:10.1615/JLongTermEffMedImplants.2015011749
Marturano, J. E., Xylas, J. F., Sridharan, G. V., Georgakoudi, I., and Kuo, C. K. (2014). Lysyl Oxidase-Mediated Collagen Crosslinks May be Assessed as Markers of Functional Properties of Tendon Tissue Formation. Acta Biomater. 10, 1370–1379. doi:10.1016/J.ACTBIO.2013.11.024
Mayne, R., and Burgeson, R. (1987). Structure and Function of Collagen Types. Orlando, FL: Elsevier. doi:10.1016/B978-0-12-481280-2.X5001-6
Mcleod, C. M., and Mauck, R. L. (2016). High Fidelity Visualization of Cell-To-Cell Variation and Temporal Dynamics in Nascent Extracellular Matrix Formation. Sci. Rep. 6, 1–12. doi:10.1038/srep38852
Meador, W. D., Sugerman, G. P., Tepole, A. B., and Rausch, M. K. (2022). Biaxial Mechanics of Thermally Denaturing Skin - Part 1: Experiments. Acta Biomater. 140, 412–420. doi:10.1016/J.ACTBIO.2021.09.033
Megens, R. T. A., Reitsma, S., Prinzen, L., Oude Egbrink, M. G. A., Engels, W., Leenders, P. J. A., et al. (2010). In Vivo high-resolution Structural Imaging of Large Arteries in Small Rodents Using Two-Photon Laser Scanning Microscopy. J. Biomed. Opt. 15, 011108. doi:10.1117/1.3281672
Mienaltowski, M. J., and Birk, D. E. (2014). “Structure, Physiology, and Biochemistry of Collagens,” in Progress in Heritable Soft Connective Tissue Diseases. Advances in Experimental Medicine and Biology. Editors J. Halper (Dordrecht: Springer), Vol. 802, 5–29. doi:10.1007/978-94-007-7893-1_2
Miler, I., Rabasovic, M. D., Aleksic, M., Krmpot, A. J., Kalezic, A., Jankovic, A., et al. (2021). Polarization‐resolved SHG Imaging as a Fast Screening Method for Collagen Alterations During Aging: Comparison with Light and Electron Microscopy. J. Biophoton. 14, e202000362. doi:10.1002/JBIO.202000362
Miyazawa, A., Makita, S., Li, E., Yamazaki, K., Kobayashi, M., Sakai, S., et al. (2019). Polarization-sensitive Optical Coherence Elastography. Biomed. Opt. Express 10, 5162–5181. doi:10.1364/BOE.10.005162
Mourant, J. R., Freyer, J. P., Hielscher, A. H., Eick, A. A., Shen, D., and Johnson, T. M. (1998). Mechanisms of Light Scattering from Biological Cells Relevant to Noninvasive Optical-Tissue Diagnostics. Appl. Opt. 37, 3586–3593. doi:10.1364/AO.37.003586
Mourant, J. R., Powers, T. M., Bocklage, T. J., Greene, H. M., Dorin, M. H., Waxman, A. G., et al. (2009). In Vivo Light Scattering for the Detection of Cancerous and Precancerous Lesions of the Cervix. Appl. Opt. 48, D26–D35. doi:10.1364/AO.48.000D26
Muanenda, Y., Oton, C. J., and Di Pasquale, F. (2019). Application of Raman and Brillouin Scattering Phenomena in Distributed Optical Fiber Sensing. Front. Phys. 7, 155. doi:10.3389/fphy.2019.00155
Naylor, E. J. (1953). The Structure of the Cornea as Revealed by Polarized Light. J. Cell Sci. s3-94, 83–88. doi:10.1242/jcs.s3-94.25.83
Nishizawa, N., Al‐Qadi, B., and Kuchimaru, T. (2021). Angular Optimization for Cancer Identification with Circularly Polarized Light. J. Biophotonics 14, e202000380. doi:10.1002/JBIO.202000380
Pham, H. T.-T., Nguyen, A. L.-T., Vo, T.-V., Huynh, K.-C., and Phan, Q.-H. (2018). Optical Parameters of Human Blood Plasma, Collagen, and Calfskin Based on the Stokes-Mueller Technique. Appl. Opt. 57, 4353. doi:10.1364/ao.57.004353
Pierangelo, A., Nazac, A., Benali, A., Validire, P., Cohen, H., Novikova, T., et al. (2013). Polarimetric Imaging of Uterine Cervix: A Case Study. Opt. Express 21, 14120–14130. doi:10.1364/OE.21.014120
Poole, J. J. A., and Mostaço-Guidolin, L. B. (2021). Optical Microscopy and the Extracellular Matrix Structure: A Review. Cells 10, 1760. doi:10.3390/CELLS10071760
Prevedel, R., Diz-Muñoz, A., Ruocco, G., and Antonacci, G. (2019). Brillouin Microscopy: An Emerging Tool for Mechanobiology. Nat. Methods 16, 969–977. doi:10.1038/s41592-019-0543-3
Qi, J., and Elson, D. S. (2016). A High Definition Mueller Polarimetric Endoscope for Tissue Characterisation. Sci. Rep. 6, 1–11. doi:10.1038/srep25953
Qi, J., and Elson, D. S. (2017). Mueller Polarimetric Imaging for Surgical and Diagnostic Applications: A Review. J. Biophotonics 10, 950–982. doi:10.1002/jbio.201600152
Qu, L., Li, Y., Xie, P., Liu, L., Wang, Y., Wu, J., et al. (2022). Cross-modal Coherent Registration of Whole Mouse Brains. Nat. Methods 19, 111–118. doi:10.1038/s41592-021-01334-w
Quinn, K. P., and Winkelstein, B. A. (2008). Altered Collagen Fiber Kinematics Define the Onset of Localized Ligament Damage during Loading. J. Appl. Physiol. 105, 1881–1888. doi:10.1152/japplphysiol.90792.2008
Rabotyagova, O. S., Cebe, P., and Kaplan, D. L. (2008). Collagen Structural Hierarchy and Susceptibility to Degradation by Ultraviolet Radiation. Mater. Sci. Eng. C 28, 1420–1429. doi:10.1016/J.MSEC.2008.03.012
Ramshaw, J. A. M., Shah, N. K., and Brodsky, B. (1998). Gly-X-Y Tripeptide Frequencies in Collagen: a Context for Host-Guest Triple-Helical Peptides. J. Struct. Biol. 122, 86–91. doi:10.1006/JSBI.1998.3977
Ricard-Blum, S., Baffet, G., and Théret, N. (2018). Molecular and Tissue Alterations of Collagens in Fibrosis. Matrix Biol. 68–69, 122–149. doi:10.1016/J.MATBIO.2018.02.004
Rich, L., and Whittaker, P. (2017). Collagen and Picrosirius Red Staining: a Polarized Light Assessment of Fibrillar Hue and Spatial Distribution. J. Morphol. Sci. 22, 97–104.
Rivenson, Y., Wang, H., Wei, Z., de Haan, K., Zhang, Y., Wu, Y., et al. (2019). Virtual Histological Staining of Unlabelled Tissue-Autofluorescence Images via Deep Learning. Nat. Biomed. Eng. 3, 466–477. doi:10.1038/s41551-019-0362-y
Roa, C., Du Le, V. N., Mahendroo, M., Saytashev, I., and Ramella-Roman, J. C. (2021). Auto-Detection of Cervical Collagen and Elastin in Mueller Matrix Polarimetry Microscopic Images Using K-NN and Semantic Segmentation Classification. Biomed. Opt. Express 12, 2236. doi:10.1364/BOE.420079
Robitaille, M. C., Zareian, R., DiMarzio, C. A., Wan, K.-T., and Ruberti, J. W. (2011). Small-Angle Light Scattering to Detect Strain-Directed Collagen Degradation in Native Tissue. Interf. Focus. 1, 767–776. doi:10.1098/RSFS.2011.0039
Ruini, C., Schlingmann, S., Jonke, Ž., Avci, P., Padrón-Laso, V., Neumeier, F., et al. (2021). Machine Learning Based Prediction of Squamous Cell Carcinoma in Ex Vivo Confocal Laser Scanning Microscopy. Cancers 13, 5522. doi:10.3390/cancers13215522
Rytky, S. J. O., Huang, L., Tanska, P., Tiulpin, A., Panfilov, E., Herzog, W., et al. (2021). Automated Analysis of Rabbit Knee Calcified Cartilage Morphology Using Micro‐Computed Tomography and Deep Learning. J. Anat. 239, 251–263. doi:10.1111/joa.13435
Saini, K., Cho, S., Dooling, L. J., and Discher, D. E. (2020). Tension in Fibrils Suppresses Their Enzymatic Degradation - A Molecular Mechanism for ‘Use it or Lose it’. Matrix Biol. 85-86, 34–46. doi:10.1016/J.MATBIO.2019.06.001
Saitou, T., Kiyomatsu, H., and Imamura, T. (2018). Quantitative Morphometry for Osteochondral Tissues Using Second Harmonic Generation Microscopy and Image Texture Information. Sci. Rep. 8, 1–14. doi:10.1038/s41598-018-21005-9
Scarcelli, G., and Yun, S. H. (2008). Confocal Brillouin Microscopy for Three-Dimensional Mechanical Imaging. Nat. Photon 2, 39–43. doi:10.1038/nphoton.2007.250
Scherer, R. L., McIntyre, J. O., and Matrisian, L. M. (2008). Imaging Matrix Metalloproteinases in Cancer. Cancer Metastasis Rev. 27, 679–690. doi:10.1007/s10555-008-9152-9
Schroeder, A. B., Karim, A., Ocotl, E., Dones, J. M., Chacko, J. V., Liu, A., et al. (2020). Optical Imaging of Collagen Fiber Damage to Assess Thermally Injured Human Skin. Wound Rep. Reg. 28, 848–855. doi:10.1111/wrr.12849
Sereysky, J. B., Andarawis-Puri, N., Jepsen, K. J., and Flatow, E. L. (2012). Structural and Mechanical Effects of In Vivo Fatigue Damage Induction on Murine Tendon. J. Orthop. Res. 30, 965–972. doi:10.1002/JOR.22012
Shah, N. S., Beebe, D. C., Lake, S. P., and Filas, B. A. (2016). On the Spatiotemporal Material Anisotropy of the Vitreous Body in Tension and Compression. Ann. Biomed. Eng. 44, 3084–3095. doi:10.1007/s10439-016-1589-3
Shavlokhova, V., Sandhu, S., Flechtenmacher, C., Koveshazi, I., Neumeier, F., Padrón-Laso, V., et al. (2021). Deep Learning on Oral Squamous Cell Carcinoma Ex Vivo Fluorescent Confocal Microscopy Data: A Feasibility Study. Jcm 10, 5326. doi:10.3390/jcm10225326
Shih, C.-C., Oakley, D. M., Joens, M. S., Roth, R. A., and Fitzpatrick, J. A. J. (2018). Nonlinear Optical Imaging of Extracellular Matrix Proteins. Methods Cell Biol 143, 57–78. doi:10.1016/BS.MCB.2017.08.004
Shin, A., Park, J., and Demer, J. L. (2018). Opto-Mechanical Characterization of Sclera by Polarization Sensitive Optical Coherence Tomography. J. Biomech. 72, 173–179. doi:10.1016/J.JBIOMECH.2018.03.017
Shoulders, M. D., and Raines, R. T. (2009). Collagen Structure and Stability. Annu. Rev. Biochem. 78, 929–958. doi:10.1146/ANNUREV.BIOCHEM.77.032207.120833
Sizeland, K. H., Wells, H. C., Kirby, N. M., Hawley, A., Mudie, S. T., Ryan, T. M., et al. (2020). Bovine Meniscus Middle Zone Tissue: Measurement of Collagen Fibril Behavior During Compression. Int. J. Nanomedicine 15, 5289–5298. doi:10.2147/IJN.S261298
Skelley, N. W., Castile, R. M., Cannon, P. C., Weber, C. I., Brophy, R. H., and Lake, S. P. (2016). Regional Variation in the Mechanical and Microstructural Properties of the Human Anterior Cruciate Ligament. Am. J. Sports Med. 44, 2892–2899. doi:10.1177/0363546516654480
Skelley, N. W., Castile, R. M., York, T. E., Gruev, V., Lake, S. P., and Brophy, R. H. (2015). Differences in the Microstructural Properties of the Anteromedial and Posterolateral Bundles of the Anterior Cruciate Ligament. Am. J. Sports Med. 43, 928–936. doi:10.1177/0363546514566192
Smith, M. V., Castile, R. M., Brophy, R. H., Dewan, A., Bernholt, D., and Lake, S. P. (2019). Mechanical Properties and Microstructural Collagen Alignment of the Ulnar Collateral Ligament During Dynamic Loading. Am. J. Sports Med. 47, 151–157. doi:10.1177/0363546518812416
Solomon, M., Liu, Y., Berezin, M. Y., and Achilefu, S. (2011). Optical Imaging in Cancer Research: Basic Principles, Tumor Detection, and Therapeutic Monitoring. Med. Princ. Pract. 20, 397–415. doi:10.1159/000327655
Solon, L. F., Castile, R. M., Smith, M. V., and Lake, S. P. (2021). Mechanical Properties and Microstructural Organization of Common Ulnar Collateral Ligament Grafts: Palmaris Longus and Gracilis Tendons. J. Orthop. Res, 1–7. doi:10.1002/JOR.25209
Sprangers, S., and Everts, V. (2019). Molecular Pathways of Cell-Mediated Degradation of Fibrillar Collagen. Matrix Biol. 75-76, 190–200. doi:10.1016/J.MATBIO.2017.11.008
Strupler, M., Pena, A.-M., Hernest, M., Tharaux, P.-L., Martin, J.-L., Beaurepaire, E., et al. (2007). Second Harmonic Imaging and Scoring of Collagen in Fibrotic Tissues. Opt. Express 15, 4054–4065. doi:10.1364/OE.15.004054
Sun, Y., Chen, W.-L., Lin, S.-J., Jee, S.-H., Chen, Y.-F., Lin, L.-C., et al. (2006). Investigating Mechanisms of Collagen Thermal Denaturation by High Resolution Second-Harmonic Generation Imaging. Biophys. J. 91, 2620–2625. doi:10.1529/BIOPHYSJ.106.085902
Szczesny, S. E., Aeppli, C., David, A., and Mauck, R. L. (2018). Fatigue Loading of Tendon Results in Collagen Kinking and Denaturation but Does Not Change Local Tissue Mechanics. J. Biomech. 71, 251–256. doi:10.1016/J.JBIOMECH.2018.02.014
Thankam, F. G., Dilisio, M. F., Gross, R. M., and Agrawal, D. K. (2018). Collagen I: a Kingpin for Rotator Cuff Tendon Pathology. Am. J. Transl. Res. 10, 3291–3309. Available at: /pmc/articles/PMC6291732/ (Accessed January 9, 2022).
Todorov, M. I., Paetzold, J. C., Schoppe, O., Tetteh, G., Shit, S., Efremov, V., et al. (2020). Machine Learning Analysis of Whole Mouse Brain Vasculature. Nat. Methods 17, 442–449. doi:10.1038/s41592-020-0792-1
Trout, R. M., Gnanatheepam, E., Gado, A., Reik, C., Ramella-Roman, J. C., Hunter, M., et al. (2022). Polarization Enhanced Laparoscope for Improved Visualization of Tissue Structural Changes Associated with Peritoneal Cancer Metastasis. Biomed. Opt. Express 13, 571–589. doi:10.1364/BOE.443926
Troyanova-Wood, M., Meng, Z., and Yakovlev, V. V. (2016). “Elasticity-Based Identification of Tumor Margins Using Brillouin Spectroscopy,” in Biophysics, Biology, and Biophotonics: The Crossroads, San Francisco, CA (Bellingham, Washington: SPIE), 72–77. doi:10.1117/12.2213268
Tsai, M.-T., Lee, C. Y., and Nguyen Hoang, T. (2018). “Evaluation of Photodamage with Polarization-Sensitive Optical Coherence Tomography,” in Biomedical Imaging and Sensing Conference, Yokahoma, Japan (Bellingham, Washington: SPIE), 25–27. doi:10.1117/12.2316799
Tuchin, V. V. (2015). Tissue Optics: Light Scattering Methods and Instruments for Medical Diagnosis. Editor V. V. Tuchin (Bellingham, Washington: SPIE). doi:10.1117/3.1003040
Tuchin, V. V. (2016). Polarized Light Interaction with Tissues. J. Biomed. Opt. 21, 071114. doi:10.1117/1.jbo.21.7.071114
Tuchin, V. V., Wang, L. V., and Zimnyakov, D. A. (2006). Optical Polarization in Biomedical Applications. Editors V. V. Tuchin, L. V. Wang, and D. A. Zimnyakov (Berlin Heidelberg: Springer). doi:10.1007/978-3-540-45321-5
Velnar, T., Bailey, T., and Smrkolj, V. (2009). The Wound Healing Process: An Overview of the Cellular and Molecular Mechanisms. J. Int. Med. Res. 37, 1528–1542. doi:10.1177/147323000903700531
Voleti, P. B., Buckley, M. R., and Soslowsky, L. J. (2012). Tendon Healing: Repair and Regeneration. Annu. Rev. Biomed. Eng. 14, 47–71. doi:10.1146/ANNUREV-BIOENG-071811-150122
Wahyudi, H., Reynolds, A. A., Li, Y., Owen, S. C., and Yu, S. M. (2016). Targeting Collagen for Diagnostic Imaging and Therapeutic Delivery. J. Controlled Release 240, 323–331. doi:10.1016/j.jconrel.2016.01.007
Wang, G., Sun, Y., Jiang, S., Wu, G., Liao, W., Chen, Y., et al. (2021). Machine Learning-Based Rapid Diagnosis of Human Borderline Ovarian Cancer on Second-Harmonic Generation Images. Biomed. Opt. Express 12, 5658. doi:10.1364/BOE.429918
Wang, H., Rivenson, Y., Jin, Y., Wei, Z., Gao, R., Günaydın, H., et al. (2019). Deep Learning Enables Cross-Modality Super-resolution in Fluorescence Microscopy. Nat. Methods 16, 103–110. doi:10.1038/s41592-018-0239-0
Wang, J.-Y., Mansfield, J. C., Brasselet, S., Vergari, C., Meakin, J. R., and Winlove, C. P. (2021). Micro-Mechanical Damage of Needle Puncture on Bovine Annulus Fibrosus Fibrils Studied Using Polarization-Resolved Second Harmonic Generation(P-SHG) Microscopy. J. Mech. Behav. Biomed. Mater. 118, 104458. doi:10.1016/J.JMBBM.2021.104458
Wang, L. V., and Wu, H.-I. (2009). Biomedical Optics: Principles and Imaging. Editors L. V. Wang, and H.-I. Wu (Hoboken, NJ: John Wiley & Sons). doi:10.1002/9780470177013
Wang, Q., Liu, W., Chen, X., Wang, X., Chen, G., and Zhu, X. (2021). Quantification of Scar Collagen Texture and Prediction of Scar Development via Second Harmonic Generation Images and a Generative Adversarial Network. Biomed. Opt. Express 12, 5305. doi:10.1364/BOE.431096
Wei, Y., and Li, Z. (2016). Measurement of D-Spacing of Crystalline Samples with SAXS. Measurement 93, 473–479. doi:10.1016/J.MEASUREMENT.2016.07.051
Wells, A., Nuschke, A., and Yates, C. C. (2016). Skin Tissue Repair: Matrix Microenvironmental Influences. Matrix Biol. 49, 25–36. doi:10.1016/J.MATBIO.2015.08.001
Wells, P. B., Thomsen, S., Jones, M. A., Baek, S., and Humphrey, J. D. (2005). Histological Evidence for the Role of Mechanical Stress in Modulating thermal Denaturation of Collagen. Biomech. Model. Mechanobiol. 4, 201–210. doi:10.1007/s10237-005-0002-1
Whelan, A., Williams, E., Nolan, D. R., Murphy, B., Gunning, P. S., O’Reilly, D., et al. (2021). Bovine Pericardium of High Fibre Dispersion Has High Fatigue Life and Increased Collagen Content; Potentially an Untapped Source of Heart Valve Leaflet Tissue. Ann. Biomed. Eng. 49, 1022–1032. doi:10.1007/s10439-020-02644-4
Whitslar, W. H. (1889). A Study of the Chemical Composition of the Dental Pulp. Am. J. Dent. Sci. 23, 350–355. Available at: https://www.ncbi.nlm.nih.gov/pmc/articles/PMC6064419/ (Accessed December 16, 2021).
Woessner, A. E., McGee, J. D., Jones, J. D., and Quinn, K. P. (2019). Characterizing Differences in the Collagen Fiber Organization of Skin Wounds Using Quantitative Polarized Light Imaging. Wound Rep. Reg. 27, 711–714. doi:10.1111/WRR.12758
Wood, M. F., Ghosh, N., Wallenburg, M. A., Li, S.-H., Weisel, R. D., Wilson, B. C., et al. (2010). Polarization Birefringence Measurements for Characterizing the Myocardium, Including Healthy, Infarcted, and Stem-Cell-Regenerated Tissues. J. Biomed. Opt. 15 (4), 047009. doi:10.1117/1.3469844
Wright, J. O., Skelley, N. W., Schur, R. P., Castile, R. M., Lake, S. P., and Brophy, R. H. (2016). Microstructural and Mechanical Properties of the Posterior Cruciate Ligament. J. Bone Jt. Surg. 98, 1656–1664. doi:10.2106/JBJS.16.00032
Wu, X., Pankow, M., Huang, H.-Y. S., and Peters, K. (2018). High-speed Polarization Imaging of Dynamic Collagen Fiber Realignment in Tendon-To-Bone Insertion Region. J. Biomed. Opt. 23, 1. doi:10.1117/1.JBO.23.11.116002
Xiao, D., Forys, B. J., Vanni, M. P., and Murphy, T. H. (2021). MesoNet Allows Automated Scaling and Segmentation of Mouse Mesoscale Cortical Maps Using Machine Learning. Nat. Commun. 12, 5992. doi:10.1038/s41467-021-26255-2
Yang, B., Lesicko, J., Sharma, M., Hill, M., Sacks, M. S., and Tunnell, J. W. (2015). Polarized Light Spatial Frequency Domain Imaging for Non-destructive Quantification of Soft Tissue Fibrous Structures. Biomed. Opt. Express 6, 1520–1533. doi:10.1364/BOE.6.001520
Yang, J.-M., Maslov, K., Yang, H.-C., Zhou, Q., Shung, K. K., and Wang, L. V. (2009). Photoacoustic Endoscopy. Opt. Lett. 34, 1591. doi:10.1364/OL.34.001591
York, T., Achilefu, S., Lake, S. P., Raman, B., Gruev, V., Powell, S. B., et al. (2014b). Bioinspired Polarization Imaging Sensors: From Circuits and Optics to Signal Processing Algorithms and Biomedical Applications. Proc. IEEE 102, 1450–1469. doi:10.1109/JPROC.2014.2342537
York, T., and Gruev, V. (2012). Characterization of a Visible Spectrum Division-Of-Focal-Plane Polarimeter. Appl. Opt. 51, 5392–5400. doi:10.1364/AO.51.005392
York, T., Kahan, L., Lake, S. P., and Gruev, V. (2014a). Real-Time High-Resolution Measurement of Collagen Alignment in Dynamically Loaded Soft Tissue. J. Biomed. Opt. 19, 1–6. doi:10.1117/1.JBO.19.6.066011
Zhang, S., Bassett, D. S., and Winkelstein, B. A. (2016). Stretch-Induced Network Reconfiguration of Collagen Fibres in the Human Facet Capsular Ligament. J. R. Soc. Interf. 13, 20150883. doi:10.1098/RSIF.2015.0883
Keywords: extracellular matrix, damage, remodeling, healing, biophotonics, biomedical optics
Citation: Iannucci LE, Dranoff CS, David MA and Lake SP (2022) Optical Imaging of Dynamic Collagen Processes in Health and Disease. Front. Mech. Eng 8:855271. doi: 10.3389/fmech.2022.855271
Received: 15 January 2022; Accepted: 22 February 2022;
Published: 18 March 2022.
Edited by:
Benjamin R. Freedman, Harvard University, United StatesReviewed by:
Adrian Buganza Tepole, Purdue University, United StatesAsa Barber, London South Bank University, United Kingdom
Copyright © 2022 Iannucci, Dranoff, David and Lake. This is an open-access article distributed under the terms of the Creative Commons Attribution License (CC BY). The use, distribution or reproduction in other forums is permitted, provided the original author(s) and the copyright owner(s) are credited and that the original publication in this journal is cited, in accordance with accepted academic practice. No use, distribution or reproduction is permitted which does not comply with these terms.
*Correspondence: Spencer P. Lake, bGFrZS5zQHd1c3RsLmVkdQ==