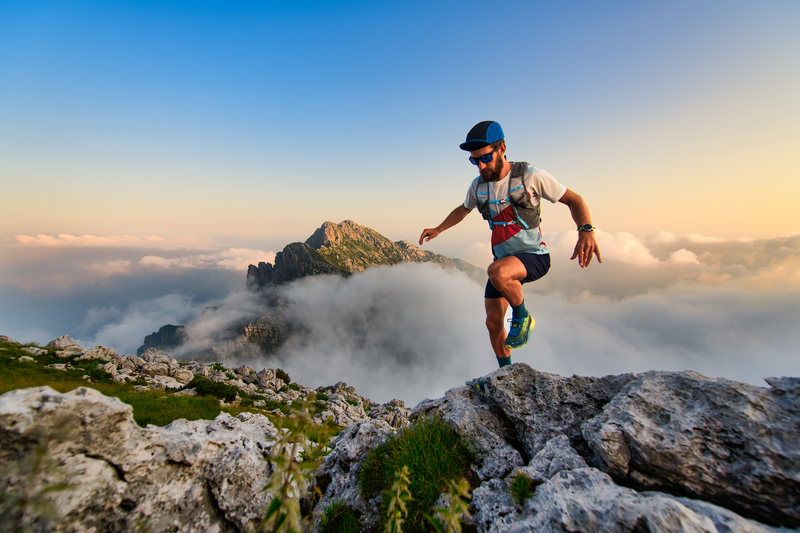
95% of researchers rate our articles as excellent or good
Learn more about the work of our research integrity team to safeguard the quality of each article we publish.
Find out more
ORIGINAL RESEARCH article
Front. Mech. Eng. , 09 January 2023
Sec. Mechatronics
Volume 8 - 2022 | https://doi.org/10.3389/fmech.2022.1075795
Introduction: There is evidence that increasing therapy dose after stroke might promote recovery. Unfortunately, in clinical practice, therapy dose is limited by financial and organizational constraints. Simple robotic devices could be used without supervision in the clinic or at home to increase dose without requiring additional resources. For this purpose, we developed HandyBot, a portable three-degrees-of-freedom end-effector haptic device to perform sensorimotor task-oriented therapy of hand function (i.e., grasping, forearm pronosupination, wrist flexion-extension) in different environments.
Methods: We present the mechatronic design of the device and its technical evaluation in terms of workspace, dynamics (i.e., max end-effector velocity, acceleration and force), sensing (i.e., position, velocity and force resolution) and haptic performance (i.e., transparency, maximum stable impedance range, rigid contact rendering accuracy). In addition, its feasibility and usability (in terms of System Usability Scale (SUS)) were assessed in a single-session experiment with four subjects with chronic stroke that tested the HandyBot therapy platform (i.e., haptic device with a graphical/physical user interface and a set of therapy exercises) while simulating unsupervised use (i.e., the subject used the device independently while a therapist was only observing the session).
Results: HandyBot showed hardware and control performances comparable to other less portable therapy devices for hand function (e.g., 94% accuracy in stiffness rendering, low apparent mass of 0.2 kg in transparency mode), making it a suitable platform for the implementation of sensorimotor therapy exercises. HandyBot showed good platform usability in terms of SUS (i.e., above 75 out of 100 for device and graphical user interfaces, above 65 out of 100 for the exercises) when tested in simulated unsupervised settings. These tests underlined minor design improvements that should be considered to allow using such a device in uncontrolled settings.
Discussion: HandyBot is a novel robot for hand rehabilitation after stroke that revealed high-quality hardware and haptic performance. HandyBot was usable for stroke patients at first exposure for (simulated) unsupervised robot-assisted sensorimotor therapy of hand function. This therapy approach combined with this novel portable robotic device has the potential to help increase therapy dose and decrease therapy-associated costs (e.g., therapist time to therapy time ratio) in different environments.
Almost two-third of stroke survivors suffer from long-term upper limb impairment and are permanently disabled in the execution of activities of daily living (ADL) (Broeks et al., 1999; Adamson et al., 2004). There is evidence that increasing therapy dose (i.e., number of exercise task repetitions and total therapy time) might promote an increase in upper limb sensorimotor recovery, even long time after the stroke (Schneider et al., 2016; Ward et al., 2019; Qiu et al., 2020). Unfortunately, in clinical practice, the therapy dose that can be achieved is often constrained by financial and organizational limitations (Schneider et al., 2016; Ward et al., 2019). As a result, patients after stroke typically receive suboptimal amounts of upper limb therapy both during inpatients rehabilitation programs in the clinic, and back home after discharge, where time to perform exercises would be more available but adequate support to engage in quality therapy is lacking (Lambercy et al., 2021).
Robotic devices could be viable tools to support high-dose functional therapies that are relevant for ADL (Hung et al., 2017). These devices offer motivating task-oriented therapies that allow therapy outcomes similar to dose-matched conventional therapies (Lo et al., 2010; Klamroth-Marganska et al., 2014; Mehrholz et al., 2015; Rodgers et al., 2019; Ranzani et al., 2020) and, in the case of haptic devices, realistic/accurate sensorimotor interactions that could support upper limb recovery (Perfetti and Grimaldi, 1979). However, the use of robotic devices is currently mainly limited to short therapy sessions in the clinics under constant supervision of specialized therapists, which limits their potential as a vector to increase therapy dose. One way to achieve this objective could be to use such technology within unsupervised (i.e., therapy performed without external supervision or intervention) robot-assisted therapy programs that could start progressively in the clinic (Büsching et al., 2018; Budhota et al., 2021) and continue at home after discharge (Chen et al., 2019; Hyakutake et al., 2019; McCabe et al., 2019; Radder et al., 2019; Lambercy et al., 2021). Unfortunately, to be usable by neurological subjects in different environments, current robotic devices should be rethought to guarantee simplicity of use, adaptability to different users and ergonomics, affordability and scalability, safety and portability (e.g., that could be easily transported in a suitcase) (Story, 2010; Lu et al., 2011; Hung et al., 2017). However, meeting these requirements is a challenge (Chen et al., 2019). As a result, only few robotic devices for upper limb rehabilitation have been proposed and tested for use in different environments (e.g., home (Lemmens et al., 2014; Sivan et al., 2014; Wolf et al., 2015; Hyakutake et al., 2019; McCabe et al., 2019)), often without an in-depth evaluation of their technical performance. Furthermore, little is known about their usability, which should be assessed already in early development stages to optimize the device design based on the user needs (Meyer et al., 2021), as well as increase acceptance and user compliance to a therapy plan (Lee et al., 2005; Lu et al., 2011; Just et al., 2018; Meyer et al., 2019; Ranzani et al., 2021).
In this paper, we present the mechatronic design and technical evaluation of HandyBot (Figure 1), a novel portable haptic device developed to perform task-oriented therapy of hand and wrist function without supervision in different environments (e.g., clinic, home). This work builds on our previous research and the knowledge gained from two haptic end-effector devices developed and clinically validated to support rehabilitation of hand function, the HapticKnob (HK, (Lambercy et al., 2007)) and the ReHapticKnob (RHK, (Metzger et al., 2011)). These devices train hand opening-closing (i.e., grasping, GR) and forearm pronosupination (PS) through sensorimotor and cognitive functionally-relevant therapy tasks, which are particularly important for hand rehabilitation (Metzger et al., 2014b). The tasks can be reproduced with high haptic accuracy through active instrumented pads that get in touch with the fingers, and are visualized in virtual reality through a computer screen. This robot-assisted therapy approach already proved their efficacy in terms of motor impairment reduction in supervised clinical settings (Lambercy et al., 2011; Ranzani et al., 2020) and was recently redesigned to allow to perform unsupervised therapy in controlled clinical settings (Ranzani et al., 2019; Ranzani et al., 2021; Devittori et al., 2022). However, the essential step necessary for using this technology outside of the controlled settings of the clinic could not yet be achieved due to the device size, cost and technology complexity.
FIGURE 1. A subject performing a therapy exercise (WristGrasp) on the HandyBot. HandyBot is a portable table-top end-effector robot for the assessment and therapy of grasping, forearm pronosupination and wrist flexion-extension tasks. Virtual tasks are haptically reproduced through two pads that get in contact with the fingers of the user, and visually rendered in a virtual reality environment presented on a computer screen. The user can start therapy by logging in to the personal account via a fingerprint reader or inserting a password through a pushbutton keyboard, which also serves as an input interface to interact with the graphical user interface. Two easily reachable emergency stop buttons are embedded in the actuation box of the device, which includes the actuators as well as electronics and safety components.
The current work has two objectives. The primary goal of this paper is to report on the mechatronic design and development of HandyBot and evaluate in depth its technical characteristics in terms of workspace, sensing, dynamics and haptic performance to guarantee good rendering of therapy exercises focusing on sensorimotor tasks that are key to hand rehabilitation (Metzger et al., 2014a). The secondary goal is to explore the feasibility of using HandyBot as a therapy device and evaluate its usability in a single-session experiment with subjects after chronic stroke, which are the target population for unsupervised use of the device. This work is expected to provide technology developers with key aspects to consider for the design of simple and scalable multi-DOF end-effector rehabilitation technology targeting unsupervised use and how such approach can be perceived by stroke patients.
Our approach was to develop HandyBot as a portable and more easily scalable haptic device that could offer the same type of sensorimotor therapy as the HK and the RHK, with the long-term objective of independent training in the home environment. To ensure the compatibility with therapy exercises previously clinically validated (Metzger et al., 2014b; Ranzani et al., 2019; Ranzani et al., 2021) and guarantee training conditions similar to the interaction with real objects, the same movements (i.e., grasping and forearm pronosupination) should be trained through an end-effector approach. Moreover, given its relevance in ADL tasks (Nelson et al., 1994; Reissner et al., 2019) and its important synergies with grasping functions (Pezent et al., 2017), it was decided to add a third degree of freedom (DOF) to train wrist flexion-extension (FE). Three DOF allow to train hand therapy tasks, while maintaining device simplicity and usability without supervision. Very few robotic devices support these three DOFs, and they either do not allow their combined training (Khor et al., 2014; Tong et al., 2015), which is essential for typical ADL or rehabilitation exercises, or they are embedded in complex and/or expensive multi-DOF therapy platforms (i.e., haptic device with a graphical/physical user interface and a set of therapy exercises) (Loureiro and Harwin, 2007; Pezent et al., 2017; Just et al., 2018). To make a powered device suitable not only for inpatient rehabilitation, but also for home rehabilitation and potential independent use (e.g., on a pay-per-use policy directed by the clinic), its cost should be reduced compared to upper limb robot-assisted therapy devices costing tens of thousands euros (Loureiro and Harwin, 2007; Metzger et al., 2011; Huang et al., 2018; Just et al., 2018). At the same time, ease of use and portability (i.e., ability to be transported in a suitcase) should be ensured (Lu et al., 2011; Khor et al., 2014), user comfort and ergonomics should be optimized (Hung et al., 2017) and safety during use and interaction with the device should be guaranteed (Story, 2010). As learned during a previous study with the RHK (Ranzani et al., 2020), the parts interacting with the user (e.g., finger pads, straps, supports) should be adaptable in size/positioning and prevent hand slippage, while arm supports should be positioned not too far from the hand fixations to reduce pressure marks on the fingers. Thumb motion during grasping would require simultaneous flexion-extension and adduction-abduction for a natural grasping (Bützer et al., 2020). Based on data from previous studies with the RHK (Ranzani et al., 2020) and biomechanical considerations, the maximum hand aperture between thumb and middle finger during typical therapy exercises should be 110mm, the maximum pronation (or supination) of the forearm is 90° and the maximum flexion (or extension) of the wrist is smaller than 80° (Norkin and White, 2016). The maximum force at the fingertip (i.e., thumb or four fingers) should be 50 N to successfully simulate typical object manipulations in ADL and therapy tasks. The maximum PS and FE torques relevant to simulate daily tasks are typically below 1.2 Nm (Williams et al., 2001; Lambercy et al., 2007). Respecting a tradeoff between electromechanical components quality and cost, accurate haptic renderings (ranging from low impedances, i.e., transparency (Metzger et al., 2012), to high impedances) should be reproduced under a stable closed-loop behavior in particular in the grasping DOF, where they are perceived by the hand with maximum sensitivity (Skedung et al., 2013).
Several design concepts and existing design solutions (Bouri et al., 2013; Tong et al., 2015) have been evaluated for the development of our 3-DOF robot HandyBot before selecting a cable-based design that could maximize structural stiffness and compactness, while providing suitable ranges of motion and forces, and a complete decoupling between the three DOF (Figure 2). The first rotational DOF is the forearm pronosupination θPS, which is controlled by a geared motor MPS-GPS rotating an aluminum L-shape structure (yellow). Two motors Mj drive the rotational DOF for wrist flexion-extension (j = FE, blue) and the linear DOF for grasping (j = GR, green) through a series of capstan drive stages with 0.69 mm tungsten cables (section 7 x 7 x 7 x 0.025 mm, Baird Industries). As recommended in literature (Nef et al., 2007; Beira et al., 2010), a transmission based on capstan drives (i.e., cable transmission) was selected since it allows to reduce friction and backlash, while maintaining high structural stiffness and allowing for the rendering of a wide range of control impedances. To pass through the coaxial joints Ji avoiding cable cross-over conflicts, coaxial pulleys Pj,i (in the form of coaxial tubes of different lengths) are used, as proposed by (Beira et al., 2018). To avoid cable slippage, each tungsten cable is constrained to the driver/load pulleys via ball beads (3 mm) and drives inside grooves on the pulley surfaces. The pulley PFE,2 directly drives the flexion-extension plate (light blue) generating a rotation θFE around the L-shape structure. The rotation of its coaxial pulley PGR,2 is transformed in the simultaneous linear motion xGR of custom made aluminum carriages mounted on linear guides (Misumi miniature guides SELB8), one (in green) moving horizontally the finger pad and two (in lighter green) driving symmetrically the (left- or right-hand) thumb pad with an inclination of 15°. This allows for the simultaneous flexion-extension and adduction-abduction of the thumb during grasping (Bützer et al., 2020). To change the left/right hand side, the finger pad can be rotated around its axis, while the thumb pad can be connected to the respective inclined slider. The pads and the palm support (Figure 1) include Velcro straps that maintain hand and fingers in place during active movements, and can be rapidly exchanged and/or manufactured on a 3D printer in different hand sizes for men, or for women. Additionally, to ensure user comfort, the palm support (Figure 1) can slide to different fixed positions depending on the hand size and shape.
FIGURE 2. Simplified model of the HandyBot. The geared motor MPS-GPS rotates the ball bearing supported yellow L-shape structure, which allows to perform forearm pronosupination with an angle θPS. Two motors MFE and MGR drive the wrist flexion-extension (light blue plate, angle θFE) and the grasping DOF (thumb and finger pads in light green and green, displacement xGR) through a series of capstan drives with tungsten cables. To allow the transmission of the power through the coaxial joints Ji, coaxial pulleys PFE,i and PGR,i (concentric tubes of different lengths) are used for each DOF. Linear guides allow the transformation of the rotational displacement of the idler pulley PGR,2 to a symmetric displacement xGR of both the thumb and finger pads. The position of each DOF is measured through rotational encoders E on the motor shafts, and redundant potentiometers (pink) for safety and calibration. The force exerted on the finger pad FGR is measured through a 1-DOF load cell embedded in the pad support. Note: The figure shows the right-hand configuration. The thumb pad could be connected to the 3rd unplugged linear slider to allow left-hand use of the device.
Positions of the end-effector are measured over time (t) in joint space with incremental encoders Ej attached to the motor shafts, which measure relative angles δθj,0 (j = PS, FE, GR). The position of the end-effector in task space [θPS(t), θFE(t), xGR) (t)] can be described with respect to joint space coordinates, and the initial end-effector absolute position in task space [θPS(0), θFE (0), xGR (0)], which serves to calibrate the encoders. θFE (0) and xGR (0) are measured by pre-calibrated soft-potentiometers positioned at the end-effector to guarantee redundant safety in position reading in the degrees of freedom with cable transmission (i.e., a mismatch in encoder and potentiometer reading shuts down the robot, since it may be associated with a failure in the cable transmission). In PS, where a geared transmission is used, θPS(0) should be instead always 0° at robot start-up. Once powered off, the end-effector tends to passively go back to this position, which is also indicated with a visual indicator on the robot. As a result, end-effector positions can be described by three linear equations.
The derivatives of these equations result in the following forward kinematics:
where iPS is the gear ratio of the gear GPS and dj,i are the diameters of the pulleys Pj,i. The determinant of the Jacobian matrix is not equal to zero, thus the system has no singularities for any given state. The motion is only constrained by the mechanical range limits of the device.
The architecture of HandyBot is shown in Figure 3. A portable reconfigurable I/O device with an embedded processor running LabVIEW Real-Time 2018 (National Instruments myRIO-1900) performs the low-level control of HandyBot. The low-level control (frequency 1 kHz) reads the sensors, performs safety routines and data saving, and sends commands to the actuators through a state machine including position and impedance (feedforward for PS and FE, with force feedback for GR (Hogan and Buerger, 2005; Metzger et al., 2012)) control modes. MyRIO can be connected via USB2.0 to any portable laptop (e.g., ACER Aspire VN7-792G, Intel Core i7-6700HQ, 32 GB RAM), which performs the high-level control of HandyBot in Unity 2018.2.18f1 at 60 Hz. The high-level control regulates the therapy exercises and interactive graphical/physical interfaces with the user, and communicates via UDP with the low-level control. The Unity software architecture is the same as the one used for the RHK and consists of a main graphical user interface, which controls the execution of selected therapy exercises planned within a therapy session. It includes two sections, one for the user and one for the therapist to customize the therapy parameters, for example, before the first therapy session. For more details, please refer to (Ranzani et al., 2021).
FIGURE 3. System architecture of HandyBot. HandyBot is interfaced with the main supply via a power inlet with switch. All active components are separated from the mains through medically certified AC/DC power converters. Two emergency stop buttons (in red) operate two power contactors via a safety relay, and redundantly cut the power to the motors while maintaining the power for the real time system (myRIO), which performs the low-level control of the platform, and the sensors. MyRIO is connected via USB to the user laptop, which performs the high-level control of the platform and provides interactive graphical (e.g., virtual reality) and physical (e.g., fingerprint reader, pushbutton keyboard) user interfaces.
The actuators were dimensioned to meet the force/torque specifications. All motors are brushed DC motors (RE40, GB, 150W, Maxon Motor) controlled in current mode by servo controllers (Escon 50/5, Maxon Motor). The motor MPS has a gearbox GPS with gear ratio 21:1, while the gear ratios achieved through the pulleys in the FE and GR DOF are approximately 2:1 and 3:1 (35:17 and 54:17), respectively. These small gear ratios (i.e., smaller than 30:1) lend the system a medium backdrivability, which guarantees a tradeoff between system transparency and safety in case of power shut down (Perret and Vercruysse, 2014).
Robot positions are measured through optical incremental encoders (HEDL 5540, 500 counts per turn for PS; MR Type L, 1,024 counts per turn for FE and GR, Maxon Motor) mounted on the motor shafts. For redundant measurement of the end-effector position (e.g., in case of failure of the cable transmission) and initial calibration, one rotational soft potentiometer is mounted between the L-shape structure and the wrist flexion-extension plate (Rotary ThinPot, 351 travel, 3% linearity, Spectra Symbol) and one linear soft potentiometer (ThinPot, 100 mm travel, 1% linearity, Spectra Symbol) is placed on the side of the linear guides on which the finger pad is mounted. A 1DOF load cell (Thin-Beam load cell, weight capacity 178N, Omega) is mounted between the finger pad and the aluminum carriage supporting it. This enables precise measurement of forces applied by/to the fingers during grasping movements, which can be reasonably expected to match thumb forces during symmetric grasping (Lambercy et al., 2014).
In order to operate HandyBot, the user can directly login into the graphical user interface, in particular into his/her therapy plan, with an optical fingerprint reader (Digital Persona 4,500, HID) (Figure 1). An intuitive colored pushbutton keyboard (Xin-Mo 1 player controller interface, Arcade World UK) allows the user to autonomously navigate in his/her therapy page, select and perform therapy exercises, similarly to the RHK therapy platform previously investigated (Ranzani et al., 2021). Both interfaces are connected with the laptop via USB2.0.
To fulfill safety norms required for electronic medical devices, the following safety features were implemented:
• To fulfill the European Standard safety requirement for medical electrical equipment (Norm IEC 60601-1:2005:AMD1:2012), HandyBot is interfaced with the mains via a medically certified power entry module with switch (IEC appliance inlet C14 with filter, fuseholder 2-pole, line switch 2-pole, Schurter). All active parts are disconnected from the mains using medically certified isolated power supplies (VMS 550W, 365W and 100W, CUI). All the components in contact with the user do not carry mains voltage and have leakage currents below the limits imposed by the norm. All metallic parts are connected to each other and to the earth conductor of the isolating transformer.
• In case of emergency during interaction with the robot, the user can press either of the two emergency stop buttons located on top of the robot (Figure 1 and Figure 3 in red). This activates a safety relay (PNOZ s1, Pilz), which operates two power contactors connected in series to redundantly cut the power supply to the servo controllers without cutting the power to the sensors. This setup fulfills the highest safety level of the European Norm EN ISO 13849–1 on safety of machinery.
• To prevent any harm to the user, maximum positions, velocities and forces are limited by the software, and the range of motion (ROM) of each DOF is constrained by mechanical stops. Additionally, if a mismatch between the redundant positions sensors is detected (e.g., bigger than 10 mm or 20°), the power to the electronic system is cut.
Furthermore, the design of the device complies with the Council Directive for medical devices (93/42/EEC:2007), respects labeling and symbols for medical devices (ISO 15223:2015) and has acceptable residual risks for the user during its operation according to an in-depth risk analysis (DIN EN ISO 14971:2018).
The general performance of HandyBot was assessed through workspace (i.e., ROM), sensing and dynamics performance measures, in order to provide a direct comparison with the HK and RHK (Metzger et al., 2011), as well as with other state of the art upper limb rehabilitation robots. The sensing measures directly affect the control performance and stability, and include:
• Encoder position resolution: minimal displacement/rotation of the end-effector that can be captured by the encoder.
• Velocity resolution: minimal detectable displacement (i.e., position resolution) divided by the sampling interval of 0.001 s.
• Maximum measurable force and force resolution: reflect the ranges provided by the sensor manufacturer. The resolution is calculated based on the force amplifier and the analogue to digital conversion of the signal.
The following dynamics measures reflect mechanical and actuation properties of the device:
• Maximum velocity and acceleration: estimated using offline lowpass filtered (20 Hz) position measurements from the encoders when giving a maximum current step (for velocity estimation) or a maximum current impulse (10 ms long, for acceleration estimate) to the motors (Hayward and Astley, 1996).
• Static friction: computed by increasing the motor current by small steps until a movement (i.e., 2 and 0.2 mm for rotational and translational DOF, respectively) of the end-effector was detected.
• Maximum and continuous end-effector force: calculated based on the stall and continuous torques provided in the motor datasheet and transformed from joint to task space.
Additional measures allow to evaluate the haptic performance of the device when using impedance control during human-robot interactions.
• Transparency planes describe the lower apparent impedance of the device (Tagliamonte et al., 2011). The transparency plane visually indicates, through its flatness, whether the haptic display is transparent or resists active movements of the user. To construct the transparency plane on the grasping DOF, the end-effector was moved by hand at different velocities during transparency rendering, while interaction force FGR and position xGR were recorded with the corresponding force sensor and encoder. Raw position data were then differentiated and filtered with a zero-phase lowpass filter with a cut-off frequency of 15 Hz to obtain velocity
• where mapp and bapp are the apparent inertia and damping felt by the user during human-robot interaction. The linearity of the transparency plane model can be validated if the trajectory points lie close to the fitted plane (i.e., the residuals of the multiple linear regression fit are small).
• Fidelity of rigid contact analyzes the ability of the device to render a sharp transition from transparency to high-impedance renderings (e.g., virtual wall), which can be often used to display virtual objects. The transition is implemented through a virtual spring-damper element that varies with the position xGR:
• During this test, one of the highest combinations of stiffness kd and damping bd that can be stably rendered was identified, while the resulting controlled stiffness at the end effector kctrl was calculated using force and encoder signals. The ability of the device to render a rigid contact was quantified as controlled stiffness fidelity (i.e., ratio between kctrl and kd).
• KB plots, as described by Colgate and Brown (Colgate and Brown, 1994), display the curve of stable kd and bd combinations that are at the edge with an unstable behavior of the system when a human interacts with a virtual wall described by (6). For instance, the stability limit can be identified when increasing the stiffness kd for a fixed damping bd until a stable impact with the virtual wall is not possible anymore. The area underneath the curve can be seen as an estimate of the Z-width (Colgate and Brown, 1994) and represents the stable parameter combinations.
The performance measures were computed for each DOF, excluding maximum measurable force, force resolution, transparency planes, rendering of rigid contact and KB plots, which were only computed for GR, as it is the only DOF equipped with a force sensor and requiring the highest haptic fidelity (Skedung et al., 2013). To enable a fair technical comparison between devices, device size, cost and weight were also considered. For more details on the performance measures evaluation, please refer to (Metzger et al., 2011; Metzger et al., 2012).
To achieve the goal of unsupervised robot-assisted therapy, the robotic platform should meet a wide range of human factors and mechatronics requirements. In addition, its usability should be assessed early during development to ensure positive user experience and compliance to a therapy program, as well as identify necessary design improvements (Shah and Robinson, 2007; Power et al., 2018; Meyer et al., 2019). The user interface and therapy exercises for unsupervised use developed in Unity for the RHK were positively evaluated in our previous work (Metzger et al., 2014b) and (Ranzani et al., 2021), and were therefore selected as a starting point for HandyBot. To verify the usability of HandyBot with minimal to no external supervision, a single-session experiment was performed with four subjects in the chronic stage after stroke (>6 months). This typically corresponds to the target population for unsupervised exercises in home settings for which HandyBot has been designed. Participants took part in a single test session, which consisted of a supervised and a simulated unsupervised part conducted in laboratory settings, as shown in Figure 4.
FIGURE 4. Study protocol. Abbreviations: FMA-UE—Fugl-Meyer of the Upper Extremity; mRS - modified Rankin Scale; aROM—active Range of Motion; SUS—System Usability Scale.
In the supervised part (in blue), a therapist assessed the subject’s baseline ability level through a set of conventional assessments (Fugl-Meyer of the Upper Extremity (FMA-UE) (Fugl-Meyer et al., 1975), modified Rankin Scale (mRS) (Rankin, 1957)) and a robotic assessment of the subject’s active ROM (aROM), which was used to customize the difficulty level of the therapy exercises. After that, the therapist instructed the subject on how to perform two exercises (i.e., Tunnel and WristGrasp), and actively guided the subject in a preliminary execution of the exercises. In the Tunnel exercise, the subject had to coordinate GR and PS to navigate inside a virtual tunnel during 1-min blocks, while avoiding obstacles and reacting to viscous perturbations (for more details please refer to (Ranzani et al., 2021)). The WristGrasp exercise was a novel sensorimotor exercise, developed to take advantage of the new features of HandyBot and train wrist FE in coordination with GR (Figure 1), which would be relevant for ADL (Pezent et al., 2017). In this exercise, the subject had to grasp a glass sphere and release it onto an invisible pedestal, located at random wrist flexion-extension positions within the subject active ROM. The location of the pedestal could only be identified through haptic cues (i.e., changes in wrist FE force field around the target position). One exercise block lasted 3 min. Both exercises have different levels of difficulty, which are adapted after each block based on performance (similarly to (Metzger et al., 2014b)). The exercises do not assist the patient (e.g., via assist-as-needed) and are rendered haptically via impedance control (with force feedback in GR and feedforward in PS and wrist FE).
In the unsupervised part of the experiment (in green), each subject had to independently use the therapy platform to perform the Tunnel exercise (i.e., 10 blocks) and the WristGrasp exercise (i.e., 3 blocks). During this time, the therapist sat at the back of the room, silently observed the subject’s actions and intervened only in case of risk. In particular, the subject had to independently position the fingers on the finger/thumb pads, log in to the graphical user interface, find and start the appropriate therapy exercises from a graphical list, perform both exercises and log out from the personal therapy account. At the end of the experiment, the subject answered four System Usability Scale (SUS) questionnaires, which are the main outcome measures: two on the exercises, one on the graphical user interface, and one on the HandyBot device with its physical interfaces (e.g., finger pads, palm support). It is important to assess the usability of these components separately, to better understand the user experience and which aspects of the therapy platform may require improvements. The SUS assesses the overall usability of the system under investigation. Two items of the SUS refer specifically to the “learnability” of a system (i.e., “I think that I would need the support of a technical person to be able to use this system”, “I needed to learn a lot of things before I could get going with this system”) and were considered of high importance for an unsupervised usage scenario (Lewis and Sauro, 2009). Ideally, the total SUS score calculated from its ten items should be greater than 50 out of 100, indicating an overall usability between “OK” and “best imaginable” (Bangor et al., 2009), and the learnability subscore should be greater than ten out of 20. The experimental protocol was approved by the ETH Zurich Ethics Commission, Switzerland (2020-N-16). Given the small sample size tested, the results of the experiment are reported as median with first and third quartile (i.e., median (quartile 1 - quartile 3)).
HandyBot resulted in a compact, easy to transport, table-top design with an actuation metal box (i.e., including actuation, electronics and safety components, 445 × 305 × 135 mm) and an end-effector (150 × 300 × 200 mm) (Figure 1). The performance of HandyBot in terms of workspace, dynamics and sensing is reported in Table 1, together with overall device size, cost and weight. In addition, these metrics are compared with the previously developed HK and RHK to show the similarity in performance despite the scalability and compactness of the new device. Based on the data reported in the literature, some of the performance metrics can also be compared with three other portable robot-assisted therapy devices, namely the ReachMAN (Yeong et al., 2009), the CR2-Haptic (Khor et al., 2014) and the OpenWrist (Pezent et al., 2017), which train (singularly and/or simultaneously) at least two of the DOF trained by HandyBot. In these devices, the ROM is 25–90 mm in GR (Yeong et al., 2009), between ±85° and ±180° in PS (Yeong et al., 2009; Khor et al., 2014; Pezent et al., 2017), and between ±70° and ±135° in FE (Khor et al., 2014; Pezent et al., 2017), generating maximum end-effector forces/torques up to 10.8 N, 3.5 Nm and 3.6 Nm, respectively. Their static friction is below 2 N in GR (Yeong et al., 2009), and below 0.4 Nm and 0.11 Nm in PS (Yeong et al., 2009; Khor et al., 2014; Pezent et al., 2017) and FE (Khor et al., 2014; Pezent et al., 2017), respectively.
TABLE 1. Performance measures of HandyBot and comparison with HapticKnob (Lambercy et al., 2007) and ReHapticKnob (Metzger et al., 2011).
On the grasping DOF, transparency planes were identified for the uncontrolled device and when the device is controlled to render transparency (i.e., minimal impedance) via impedance control with force feedback (Figure 5). The uncontrolled device showed an apparent mass of 0.65 kg and an apparent damping of 24.24 Ns/m with average residuals above 1 N, while the impedance control with force feedback during transparency rendering reduced the apparent mass by 69.14% (to 0.20 kg), the apparent damping by 88.54% (to 2.78 Ns/m) and the residuals below 0.7 N. The dynamic human-robot interaction movements achieved during the transparency rendering test reached large velocity and acceleration values close to or above the maximum velocity and acceleration of the actuated HandyBot (Figure 5c). This indicates that the control allows the user, with little additional effort, to push the system above its limits, and that, despite reaching their saturation, the motors are still supporting transparency rendering.
FIGURE 5. Transparency planes. User experienced transparency (apparent dynamics) during physical human-robot interaction in the grasping degree of freedom when HandyBot is uncontrolled (A), and when it is controlled via impedance control with force feedback (B) and perturbed with motion patterns shown in (C). Recorded force-motion trajectories (blue dots) are plotted over velocity and acceleration to indicate the damping and inertia components of the apparent impedance. A linear fit (yellow plane) and the identified model parameters of the apparent damping bapp and mass mapp allow a qualitative and quantitative comparison between the performance of the different control approaches. The plane size represents the range of maximum actuated velocity and acceleration reachable by HandyBot. The averaged (AVG) residuals indicate the fitting accuracy of the transparency planes.
A combination of kd = 25 kN/m and bd = 0.02 kN*s/m was selected to test the control fidelity in rendering a rigid contact, since this kd-bd combination represents one of the highest impedances that HandyBot can stably render (as shown by the blue square in Figure 6B). The virtual wall transition is shown in Figure 6A in comparison with the RHK (Metzger et al., 2012) and the commercially available Phantom Premium 1.5 (Massie and Salisbury, 1994), which were also tested with the kd-bd combination yielding their best haptic performance in terms of wall rendering. Similarly to these devices, HandyBot can render transparency with resistances <1 N but, as shown in Table 2, can reach a controlled stiffness accuracy of 94% compared to the accuracies of 92% and 80% of the RHK and Phantom Premium 1.5, respectively. Figure 6B shows the KB plots for HandyBot and RHK in the grasping DOF (Metzger et al., 2012). The maximum stable rendered stiffness
FIGURE 6. Rendering of a virtual wall and KB plots with HandyBot, ReHapticKnob and Phantom Premium 1.5. (A) Comparison between the interaction with a virtual wall (unidirectional stiff spring-damper combination) rendered with HandyBot (blue), ReHapticKnob (black (Metzger et al., 2012) and with the commercially available Phantom Premium 1.5 (orange (Massie and Salisbury, 1994). (B) Stable desired spring-damper combinations renderable as rigid contact (described in (6) via impedance control with force feedback by HandyBot (Kf = 5) or ReHapticKnob (Kf = 10) in grasping. The area underneath the curve (Z-width estimate) describes all the possible stable parameter combinations. The blue square indicates the desired virtual wall parameters tested in HandyBot to evaluate its rendering performance (see Table 2).
TABLE 2. Performance parameters of virtual wall rendering with HandyBot, ReHapticKnob and Phantom Premium 1.5. Desired and achieved parameters for a virtual wall (shown in Figure 6.A) rendered in the grasping DOF of HandyBot, ReHapticKnob (Metzger et al., 2012) and Phantom Premium 1.5 (Massie and Salisbury, 1994).
Four subjects (1 female, 3 male) in the chronic stage after an ischemic (3) or hemorrhagic (1) stroke were eligible and agreed to participate in the feasibility and usability evaluation experiment. As shown in Table 3, most subjects had mild to moderate (Woytowicz et al., 2017) upper-limb impairment with a FMA-UE of 48.5 (41.3–55.5) out of 66 points and a mRS of 2.0 (1.8–2.3). During the simulated unsupervised part of the experiment, the subjects could independently position their hand in the finger/thumb pads and operate the device to start and perform the appropriate therapy exercises. No serious adverse event related to the use of the robot, nor any event that would put at risk the safety of the user was observed. Subject three (FMA-UE of 39 out of 66, maximum active ROM index to thumb 38 mm) required external help in both exercises as he could not autonomously open the hand due to high hand muscle tone. Regarding the hardware, minor usability limitations were identified for all users. The 3D printed palm support and its strap fixation were too weak to maintain the hand and arm in place with respect to the device, which has a perceivable residual inertia (after impedance control) in the PS DOF. The issue with the straps led to difficulties for the users in maintaining their anatomical axes (i.e., mainly the forearm pronosupination axis) aligned with the respective robot axes. Moreover, supporting wrist FE forces were at times not sufficient to maintain the hand in place during rapid pronosupination rotations in the Tunnel exercise, in which gravity directly acted on the wrist FE DOF. Regarding the software, the subjects had difficulties in understanding the rules of the WristGrasp exercise mainly due to difficulties in perceiving haptic cues at the level of the wrist or coordinating grasping forces to not break the sphere while moving. HandyBot was ranked with a SUS score between OK and excellent (76.3 (58.1–91.3) out of 100) and a learnability subscore of 11.3 (9.4–13.8) out of 20, while the graphical user interface was ranked with a SUS score between good and excellent (85.0 (73.8–91.3) out of 100) and a learnability subscore of 13.8 (7.5–18.1) out of 20. The Tunnel and WristGrasp exercises were ranked with SUS scores between good and excellent (78.8 (70.0–83.8) out of 100) and between OK and good (67.5 (61.3–74.4) out of 100), and learnability subscores of 13.8 (8.8–17.5) and 12.50 (9.4–16.3) out of 20, respectively.
This paper presents the mechatronic design, as well as performance evaluation of HandyBot, a novel portable end-effector haptic device optimized for unsupervised robot-assisted therapy of hand function after stroke. HandyBot builds on the sensorimotor robot-assisted therapy concept developed on two earlier haptic devices, HapticKnob (HK) and ReHapticKnob (RHK), whose efficacy was successfully validated in supervised clinical environments when used by subjects after stroke (Lambercy et al., 2011; Ranzani et al., 2020). HandyBot strives to provide a similar therapy platform (i.e., end-effector haptic device with user interface and sensorimotor therapy exercises) than the one previously validated on the RHK (Ranzani et al., 2021), and to extend its use to different environments (e.g., start in the clinic and continue at home), to further promote unsupervised use. This promises to complement conventional therapies, increase therapy dose of quality rehabilitation and subject autonomy while decreasing reliance on hospital stays (Lambercy et al., 2021).
HandyBot is noticeably more compact and portable than the HK and the RHK, and still allows to actively train grasping and forearm pronosupination, and an additional movement (i.e., wrist flexion extension), following the same validated sensorimotor therapy concept (Lambercy et al., 2011; Metzger et al., 2014b).
Excluding wearable devices (e.g., hand gloves, exoskeletons), only few powered portable devices focus on the training of hand function (Hesse et al., 2008; Khor et al., 2014; Tong et al., 2015; Pezent et al., 2017) and allow to actively assist/resist the patient movements and/or to reproduce sensorimotor therapy tasks. Compared to these portable devices and its non-portable predecessors, HandyBot is the first portable haptic device that trains simultaneously PS, FE and GR and that could be used in unsupervised settings. It maintains similar performance in terms of workspace, dynamics and sensing, despite achieving an important cost reduction (approximately -55%) with respect to earlier concepts, making the system more scalable in view of potential deployment in home settings.
The robot workspace is similar to state-of-the-art rehabilitation devices for PS and FE and slightly smaller for GR, both in minimal and maximal hand aperture (i.e., 10–110 mm thumb to index) (Yeong et al., 2009; Khor et al., 2014; Pezent et al., 2017). This allows simulating fine object manipulation while respecting biomechanical and therapy requirements (Norkin and White, 2016; Ranzani et al., 2020). Maximum achievable movement/force dynamics and sensor resolution are in the same order of magnitude of other devices except for PS, which achieves slightly lower accelerations and has an increased static friction, which can be attributed to the high weight and inertia of the metal L-shape structure necessary to align the robotic wrist FE axis with the user anatomical axis. Maximum generated grasping forces are in line with other rehabilitation devices (Lambercy et al., 2007; Yeong et al., 2009) and correspond to the force levels needed in therapy exercises and in ADL (Lambercy et al., 2007). While PS can achieve torques and, potentially, maximum impedances higher than average (Yeong et al., 2009; Khor et al., 2014; Pezent et al., 2017), the FE DOF achieves maximum torques that, after overcoming the robot inertia, only allow to assist/resist/perturb the user movements, but cannot passively hold the limb of the user in different positions particularly against gravity (i.e., when the user is in extreme pronation or supination positions).
Through a single low-cost force sensor, HandyBot allows to maintain good haptic control performance in terms of rigid contact rendering fidelity, and span between maximum achievable impedances and transparencies, particularly at the level of hand grasping, which is characterized by the finest sensorimotor control (Radman, 2013; Röijezon et al., 2017). The transparency rendering performance is better than the RHK, achieving a quarter of the apparent mass (i.e., 0.2 kg compared to 0.8 kg in RHK). However, smaller maximum impedances, but still sufficient for the available therapy exercises, are reached (Metzger et al., 2012; Metzger et al., 2014b).
The differences in impedance rendering between HandyBot and RHK could be explained by the lower quality of the low-cost components of HandyBot (e.g., force sensor in GR), which may negatively affect the control performance, and by the nature of their mechanical transmissions (i.e., transmission type, gear ratio). A geared transmission (e.g., gearboxes and/or timing belts, as in the case of RHK and PS in HandyBot) allows to stably render a wide range of impedances, but significantly increases size, weight and inertia of end-effector designs, proportionally to the number of DOF. Additionally, it can reduce transparency mainly due to backlash and/or high gear ratios. A cable transmission (GR and FE in HandyBot) has instead the potential to reduce the size, weight and inertia of the end-effector, and to improve transmission transparency. Moreover, tungsten wires allow to maintain a stable level of cable tensioning over time, without the need to re-tension them (Beira et al., 2010). Furthermore, in GR, HandyBot has approximately a 3:1 gear ratio, which significantly reduces the maximum range of renderable impedances compared to the 12:1 gear ratio of the RHK with the same actuator.
We achieved promising preliminary usability results with our therapy platform, which promise that HandyBot can be usable without supervision and learnable during a first exposure. After a supervised instruction phase, we simulated unsupervised therapy conditions, but we allowed the therapist to intervene only in case help was strictly needed (e.g., in adverse conditions), as done in unsupervised trainings in clinical settings (Lemmens et al., 2014; Sivan et al., 2014; Hyakutake et al., 2019). Throughout the test, the therapist intervention was only required for one subject (subject three) that had an increase in hand muscle tone during the experiment, but whose muscle tone and hand active ROM were already altered at baseline. Robotic assessments incorporated into the therapy exercises could allow to monitor hand muscle tone throughout the therapy, to avoid negative consequences such as pain that could affect recovery (Ranzani et al., 2019). The device respects safety norms for medical devices, as well as ergonomics and adaptability design requirements, and did not show safety-related problems. Minimization of issues requiring external intervention and safety are fundamental for the use of the therapy platform, particularly in the home environment, where supervision is not always available or would require additional communication channels (e.g., telerehabilitation (Wolf et al., 2015)). The preliminary usability results of HandyBot, graphical user interface and Tunnel exercise are between good and excellent (i.e., approximately between 70 and 90 out of 100), which is aligned with the usability results achieved when using the RHK with minimal supervision (Ranzani et al., 2021), meaning that the change of hardware did not affect the user experience during therapy. The WristGrasp exercise obtained lower but positive usability scores (i.e., above “OK”), probably associated with the difficulty of the exercise, which requires good sensorimotor functions to hold the glass sphere without breaking it or to identify target wrist flexion-extension positions based on haptic cues. The usability results are positively aligned with the few other studies that evaluated the usability of technology-assisted therapy platforms (Sivan et al., 2014; Chen et al., 2015; Pei et al., 2017), although only one of these assessed the SUS with an average score of 71.8 out of 100 (Pei et al., 2017).
Our evaluation allowed to identify important design improvements that will be addressed before testing the device in real unsupervised conditions (e.g., home). Hand/arm supports should be optimized to allow precise control of the limb positioning (e.g., avoid compensatory movements) and prevent misalignments between anatomical and robot joints, which could obtrude the movements of the subject in positions that are at the limit of the robot workspace. Completely eliminating these issues is a challenge, particularly in an end-effector device when patients try to control multiple DOF simultaneously. Therefore, exercises that train a maximum of 2 DOF simultaneously should be considered with an end-effector approach. Furthermore, as recommended in literature (Perfetti and Grimaldi, 1979), to avoid visual compensation in solving sensorimotor therapy tasks, the hand of the subject should be covered during therapy.
Our preliminary usability results should be interpreted with respect to the small sample size tested, although this size can be considered sufficient to identify major usability challenges of the platform in early stages of development (Virzi, 1992). However, the results should be further validated over a longer time horizon in a larger population and in real unsupervised conditions (e.g., in the home environment). This could allow to verify the feasibility and efficacy of this therapy approach, whether subjects could learn to use the system and progressively improve their performance level, and if their motivation to use the device would change. Furthermore, in future studies, it could be interesting to investigate how a device like HandyBot could be complemented by different haptic feedback modalities (e.g., vibrotactile feedback (Scalera et al., 2018; Yunus et al., 2020)).
Our positive performance results in terms of haptic rendering and usability with the same unsupervised therapy framework as the RHK open the door to the use of our compact device, HandyBot, in different unsupervised settings (e.g., clinic or home) after an appropriate supervised learning period in the clinic. Our device, focusing on active training of hand function, could complement existing upper limb robot-assisted therapy devices that have been deployed for home rehabilitation (Akbari et al., 2021; Budhota et al., 2021). However, these devices should be selected carefully since, particularly among non-wearable devices, usability without supervision and portability are often lacking. This robot-assisted approach could help increase therapy dose for the patients and reduce limb non-use after discharge, decrease therapy-associated costs (e.g., therapist time during unsupervised use in the clinic) and progressively increase patient independence in daily-life settings.
Future investigations should verify the feasibility and usability of our portable therapy platform within a clinical trial, which starts in the clinic and continues at home without supervision. These tests will also help understanding for which type of patient population (e.g., impairment type and severity, stage after stroke) this kind of therapy is most suitable. To make the step into the home environment, clear protocols will have to be defined to decide when the patient is ready to perform such training at home and how family members and therapists should be instructed to assist the patient (when needed). Furthermore, cost models for the implementation of robot-assisted rehabilitation at home should be carefully investigated, focusing for example on the development of rental models or reimbursement schemes, as robotic technologies currently remain too expensive to guarantee their direct accessibility to all persons with stroke.
This paper presents the mechatronic design of HandyBot, a portable haptic device for sensorimotor training of hand grasping, forearm pronosupination and wrist flexion-extension developed as a platform to provide unsupervised hand rehabilitation. We further report its technical characterization and preliminary feasibility and usability evaluation with subject with chronic stroke in simulated unsupervised settings. The results show that HandyBot achieves good workspace, dynamics, sensing and haptic performance, while guaranteeing compactness and scalability with respect to cost reduction with respect to other comparable devices, which train only some of the degrees of freedom of HandyBot and/or appear to be not suitable for unsupervised settings (e.g., due to their size, cost or complexity). HandyBot was usable by subjects with chronic stroke at first exposure with minimal external supervision in a controlled environment.
From a technology perspective, our results pave the way to the use of portable robot-assisted therapy platforms to implement a technology-supported continuum of care. In this vision, subjects with stroke could potentially familiarize early with the use of such technology in the clinic with progressively reduced therapist supervision and later continue the training at home after hospital discharge (e.g., via a rental model, or direct purchase provided that the costs of such technologies could be further reduced). This approach could allow increasing the dose of quality rehabilitation for persons with stroke, with the potential for improved patient autonomy and independence. To make the step into the home environment, clear protocols will have to be defined to decide when the patient is ready to perform such training at home and how family members and therapists should be instructed to assist the patient (when needed).
The original contributions presented in the study are included in the article, further inquiries can be directed to the corresponding author.
The studies involving human participants were reviewed and approved by ETH Zurich Ethics Commission, Switzerland. The patients/participants provided their written informed consent to participate in this study. Written informed consent was obtained from the individual(s) for the publication of any potentially identifiable images or data included in this article.
RR designed and developed HandyBot with the help of CH, FT, OL, RG, performed the data analysis and wrote the manuscript. RR, MA, and EK developed the low-level control and evaluated the technical performance of HandyBot. MA and RR developed the graphical user interface and therapy exercises. RR, OL, and RG defined the study protocol. JH, GD, and RR coordinated the data collection and conducted the usability evaluations with patients. All authors reviewed the final manuscript.
This work has received funding from the European Union Horizon 2020 research and innovation programme under grant agreement No. 688857 (SoftPro), from the Swiss State Secretariat for Education, Research and Innovation (SERI; contract number 15.0283-1), and by the National Research Foundation, Prime Minister’s Office, Singapore under its Campus for Research Excellence and Technological Enterprise (CREATE) programme. Open access funding provided by ETH Zurich Florianne.
The authors would like to acknowledge Ricardo Beira for his inputs on the device mechanics, and the medical doctors and therapists of the Clinica Hildebrand Centro di Riabilitazione Brissago, who helped in defining the design requirements and testing of preliminary kinematic prototypes. Special thanks go to David Fisch, Jiri Danihelka and Cherelle Connor who participated in the development of the graphical user interface and exercises, as well as Kevin Sin, who evaluated the device compliance with respect to safety norms for medical devices.
CH and FT are part of Hankamp Rehab, a company selling rehabilitation technologies, but they declare, as all the other authors, to not have competing or financial interests in the device and work presented in this paper.
All claims expressed in this article are solely those of the authors and do not necessarily represent those of their affiliated organizations, or those of the publisher, the editors and the reviewers. Any product that may be evaluated in this article, or claim that may be made by its manufacturer, is not guaranteed or endorsed by the publisher.
Adamson, J., Beswick, A., and Ebrahim, S. (2004). Is stroke the most common cause of disability? J. stroke Cerebrovasc. Dis. 13 (4), 171–177. doi:10.1016/j.jstrokecerebrovasdis.2004.06.003
Akbari, A., Haghverd, F., and Behbahani, S. (2021). Robotic home-based rehabilitation systems design: From a literature review to a conceptual framework for community-based remote therapy during COVID-19 pandemic. Front. Robotics AI 8, 612331. doi:10.3389/frobt.2021.612331
Bangor, A., Kortum, P., and Miller, J. (2009). Determining what individual SUS scores mean: Adding an adjective rating scale. J. usability Stud. 4 (3), 114–123.
Beira, R., Clavel, R., and Bleuler, H. (2018). Mechanical manipulator for surgical instruments. Mountain View, CA: Google Patents.
Beira, R., Sengül, A., Hara, M., Schoeneich, P., and Bleuler, H. (2010). “Tendon-based transmission for surgical robotics: Systematic experimental friction modeling,” in International Conference on Applied Bionics and Biomechanics (Lausanne, Switzerland: ICABB-2010).
Bouri, M., Baur, C., Clavel, R., Newman, C., and Zedka, M. (2013). “Handreha”: A new hand and wrist haptic device for hemiplegic children,” in ACHI 2013, The Sixth International Conference on Advances in Computer-Human Interactions, 286–292.
Broeks, J., Lankhorst, G., Rumping, K., and Prevo, A. (1999). The long-term outcome of arm function after stroke: Results of a follow-up study. Disabil. rehabilitation 21 (8), 357–364. doi:10.1080/096382899297459
Budhota, A., Chua, K. S., Hussain, A., Kager, S., Cherpin, A., Contu, S., et al. (2021). Robotic assisted upper limb training post stroke: A randomized control trial using combinatory approach toward reducing workforce demands. Front. neurology 12, 622014. doi:10.3389/fneur.2021.622014
Büsching, I., Sehle, A., Stürner, J., and Liepert, J. (2018). Using an upper extremity exoskeleton for semi-autonomous exercise during inpatient neurological rehabilitation-a pilot study. J. neuroengineering rehabilitation 15 (1), 72. doi:10.1186/s12984-018-0415-6
Bützer, T., Lambercy, O., Arata, J., and Gassert, R. (2020). Fully wearable actuated soft exoskeleton for grasping assistance in everyday activities. Soft Robot. 8, 128–143. doi:10.1089/soro.2019.0135
Chen, M.-H., Huang, L.-L., and Wang, C.-H. (2015). Developing a digital game for stroke patients’ upper extremity rehabilitation–design, usability and effectiveness assessment. Procedia Manuf. 3, 6–12. doi:10.1016/j.promfg.2015.07.101
Chen, Y., Abel, K. T., Janecek, J. T., Chen, Y., Zheng, K., and Cramer, S. C. (2019). Home-based technologies for stroke rehabilitation: A systematic review. Int. J. Med. Inf. 123, 11–22. doi:10.1016/j.ijmedinf.2018.12.001
Colgate, J. E., and Brown, J. M. (1994). “Factors affecting the z-width of a haptic display,” in Proceedings of the 1994 IEEE International Conference on Robotics and Automation (IEEE), 3205–3210.
Devittori, G., Ranzani, R., Dinacci, D., Romiti, D., Califfi, A., Petrillo, C., et al. (2022). “Automatic and personalized adaptation of therapy parameters for unsupervised robot-assisted rehabilitation: A pilot evaluation,” in 2022 International Conference on Rehabilitation Robotics (ICORR) (IEEE), 1–6.
Fugl-Meyer, A. R., Jääskö, L., Leyman, I., Olsson, S., and Steglind, S. (1975). The post-stroke hemiplegic patient. 1. a method for evaluation of physical performance. Scand. J. rehabilitation Med. 7 (1), 13–31.
Hayward, V., and Astley, O. R. (1996). “Performance measures for haptic interfaces,” in Robotics research (Springer), 195–206.
Hesse, S., Kuhlmann, H., Wilk, J., Tomelleri, C., and Kirker, S. G. (2008). A new electromechanical trainer for sensorimotor rehabilitation of paralysed fingers: A case series in chronic and acute stroke patients. J. Neuroengineering rehabilitation 5 (1), 21. doi:10.1186/1743-0003-5-21
Hogan, N., and Buerger, S. (2005). Impedance and interaction control robotics and automation handbook ed TR kurfess. Boca Raton, FL: CRC Press.
Huang, X., Naghdy, F., Naghdy, G., Du, H., and Todd, C. (2018). The combined effects of adaptive control and virtual reality on robot-assisted fine hand motion rehabilitation in chronic stroke patients: A case study. J. Stroke Cerebrovasc. Dis. 27 (1), 221–228. doi:10.1016/j.jstrokecerebrovasdis.2017.08.027
Hung, Y.-H., Chen, P.-J., and Lin, W.-Z. (2017). “Design factors and opportunities of rehabilitation robots in upper-limb training after stroke,” in 14th International Conference on Ubiquitous Robots and Ambient Intelligence (URAI) (IEEE), 650–654.
Hyakutake, K., Morishita, T., Saita, K., Fukuda, H., Shiota, E., Higaki, Y., et al. (2019). Effects of home-based robotic therapy involving the single-joint hybrid assistive limb robotic suit in the chronic phase of stroke: A pilot study. BioMed Res. Int. 2019, 1–9. doi:10.1155/2019/5462694
Just, F., Gunz, D., Duarte, J., Simonetti, D., Riener, R., and Rauter, G. (2018). “Improving usability of rehabilitation robots: Hand module evaluation of the ARMin exoskeleton,” in International Symposium on Wearable Robotics (Springer), 80–84.
Khor, K., Chin, P., Hisyam, A., Yeong, C., Narayanan, A., and Su, E. (2014). “Development of CR2-haptic: A compact and portable rehabilitation robot for wrist and forearm training,” in IEEE Conference on Biomedical Engineering and Sciences (IECBES) (IEEE), 424–429.
Klamroth-Marganska, V., Blanco, J., Campen, K., Curt, A., Dietz, V., Ettlin, T., et al. (2014). Three-dimensional, task-specific robot therapy of the arm after stroke: A multicentre, parallel-group randomised trial. Lancet Neurology 13 (2), 159–166. doi:10.1016/s1474-4422(13)70305-3
Lambercy, O., Dovat, L., Gassert, R., Burdet, E., Teo, C. L., and Milner, T. (2007). A haptic knob for rehabilitation of hand function. IEEE Trans. Neural Syst. Rehabilitation Eng. 15 (3), 356–366. doi:10.1109/tnsre.2007.903913
Lambercy, O., Dovat, L., Yun, H., Wee, S. K., Kuah, C. W., Chua, K. S., et al. (2011). Effects of a robot-assisted training of grasp and pronation/supination in chronic stroke: A pilot study. J. neuroengineering rehabilitation 8 (1), 63. doi:10.1186/1743-0003-8-63
Lambercy, O., Lehner, R., Chua, K. S., Wee, S. K., Rajeswaran, D. K., Kuah, C. W. K., et al. (2021). Neurorehabilitation from a distance: Can intelligent technology support decentralized access to quality therapy? Front. Robotics AI 8, 612415. doi:10.3389/frobt.2021.612415
Lambercy, O., Metzger, J.-C., Santello, M., and Gassert, R. (2014). A method to study precision grip control in viscoelastic force fields using a robotic gripper. IEEE Trans. Biomed. Eng. 62 (1), 39–48. doi:10.1109/tbme.2014.2336095
Lee, M., Rittenhouse, M., and Abdullah, H. A. (2005). Design issues for therapeutic robot systems: Results from a survey of physiotherapists. J. Intelligent robotic Syst. 42 (3), 239–252. doi:10.1007/s10846-004-7194-y
Lemmens, R. J., Timmermans, A. A., Janssen-Potten, Y. J., Pulles, S. A., Geers, R. P., Bakx, W. G., et al. (2014). Accelerometry measuring the outcome of robot-supported upper limb training in chronic stroke: A randomized controlled trial. PloS one 9 (5), e96414. doi:10.1371/journal.pone.0096414
Lewis, J. R., and Sauro, J. (2009). “The factor structure of the system usability scale,” in International conference on human centered design (Springer), 94–103.
Lo, A. C., Guarino, P. D., Richards, L. G., Haselkorn, J. K., Wittenberg, G. F., Federman, D. G., et al. (2010). Robot-assisted therapy for long-term upper-limb impairment after stroke. N. Engl. J. Med. 362 (19), 1772–1783. doi:10.1056/nejmoa0911341
Loureiro, R. C., and Harwin, W. S. (2007). “Reach & grasp therapy: Design and control of a 9-DOF robotic neuro-rehabilitation system,” in IEEE 10th International Conference on Rehabilitation Robotics (IEEE), 757–763.
Lu, E. C., Wang, R. H., Hebert, D., Boger, J., Galea, M. P., and Mihailidis, A. (2011). The development of an upper limb stroke rehabilitation robot: Identification of clinical practices and design requirements through a survey of therapists. Disabil. rehabilitation Assistive Technol. 6 (5), 420–431. doi:10.3109/17483107.2010.544370
Massie, T. H., and Salisbury, J. K. (1994). “The phantom haptic interface: A device for probing virtual objects,” in Proceedings of the ASME winter annual meeting, symposium on haptic interfaces for virtual environment and teleoperator systems (Chicago, IL, 295–300.
McCabe, J. P., Henniger, D., Perkins, J., Skelly, M., Tatsuoka, C., and Pundik, S. (2019). Feasibility and clinical experience of implementing a myoelectric upper limb orthosis in the rehabilitation of chronic stroke patients: A clinical case series report. PloS one 14 (4), e0215311. doi:10.1371/journal.pone.0215311
Mehrholz, J., Pohl, M., Platz, T., Kugler, J., and Elsner, B. (2015). Electromechanical and robot-assisted arm training for improving activities of daily living, arm function, and arm muscle strength after stroke. Hoboken, NJ: The Cochrane Library.
Metzger, J.-C., Lambercy, O., Califfi, A., Conti, F. M., and Gassert, R. (2014a). Neurocognitive robot-assisted therapy of hand function. IEEE Trans. haptics 7 (2), 140–149. doi:10.1109/toh.2013.72
Metzger, J.-C., Lambercy, O., Califfi, A., Dinacci, D., Petrillo, C., Rossi, P., et al. (2014b). Assessment-driven selection and adaptation of exercise difficulty in robot-assisted therapy: A pilot study with a hand rehabilitation robot. J. neuroengineering rehabilitation 11 (1), 154. doi:10.1186/1743-0003-11-154
Metzger, J.-C., Lambercy, O., Chapuis, D., and Gassert, R. (2011). “Design and characterization of the ReHapticKnob, a robot for assessment and therapy of hand function,” in Intelligent Robots and Systems (IROS), 2011 IEEE/RSJ International Conference on (IEEE), 3074–3080.
Metzger, J.-C., Lambercy, O., and Gassert, R. (2012). “High-fidelity rendering of virtual objects with the ReHapticKnob-novel avenues in robot-assisted rehabilitation of hand function,” in Haptics Symposium (HAPTICS), 2012 IEEE (IEEE), 51–56.
Meyer, J. T., Gassert, R., and Lambercy, O. (2021). An analysis of usability evaluation practices and contexts of use in wearable robotics. J. neuroengineering rehabilitation 18 (1), 1–15. doi:10.1186/s12984-021-00963-8
Meyer, J. T., Schrade, S. O., Lambercy, O., and Gassert, R. (2019). “User-centered design and evaluation of physical interfaces for an exoskeleton for paraplegic users,” in IEEE 16th International Conference on Rehabilitation Robotics (ICORR) (IEEE), 1159–1166.
Nef, T., Mihelj, M., and Riener, R. (2007). ARMin: A robot for patient-cooperative arm therapy. Med. Biol. Eng. Comput. 45 (9), 887–900. doi:10.1007/s11517-007-0226-6
Nelson, D. L., Mitchell, M. A., Groszewski, P. G., Pennick, S. L., and Manske, P. R. (1994). “Wrist range of motion in activities of daily living,” in Advances in the biomechanics of the hand and wrist (Springer), 329–334.
Norkin, C. C., and White, D. J. (2016). Measurement of joint motion: A guide to goniometry. F. A. Davis.
Pei, Y.-C., Chen, J.-L., Wong, A. M., and Tseng, K. C. (2017). An evaluation of the design and usability of a novel robotic bilateral arm rehabilitation device for patients with stroke. Front. neurorobotics 11, 36. doi:10.3389/fnbot.2017.00036
Perfetti, C., and Grimaldi, L. (1979). Rieducazione motoria dell'emiplegico. Milano, Italy: Ghedimedia.
Perret, J., and Vercruysse, P. (2014). “Advantages of mechanical backdrivability for medical applications of force control,” in Workshop on Computer/Robot Assisted Surgery (CRAS).
Pezent, E., Rose, C. G., Deshpande, A. D., and O'Malley, M. K. (2017). “Design and characterization of the openwrist: A robotic wrist exoskeleton for coordinated hand-wrist rehabilitation,” in International Conference on Rehabilitation Robotics (ICORR) (IEEE), 720–725.
Piazza, C., Catalano, M. G., Bianchi, M., Ricciardi, E., Prattichizzo, D., Haddadin, E., et al. (2018). “The softpro project: Synergy-based open-source technologies for prosthetics and rehabilitation,” in International Symposium on Wearable Robotics (Cham: Springer), 370–374.
Power, V., de Eyto, A., Hartigan, B., Ortiz, J., and O’Sullivan, L. W. (2018). “Application of a user-centered design approach to the development of XoSoft–A lower body soft exoskeleton,” in International Symposium on Wearable Robotics (Springer), 44–48.
Qiu, Q., Cronce, A., Patel, J., Fluet, G. G., Mont, A. J., Merians, A. S., et al. (2020). Development of the Home based Virtual Rehabilitation System (HoVRS) to remotely deliver an intense and customized upper extremity training. J. neuroengineering rehabilitation 17 (1), 1–10. doi:10.1186/s12984-020-00789-w
Radder, B., Prange-Lasonder, G. B., Kottink, A. I., Holmberg, J., Sletta, K., van Dijk, M., et al. (2019). Home rehabilitation supported by a wearable soft-robotic device for improving hand function in older adults: A pilot randomized controlled trial. PloS one 14 (8), e0220544. doi:10.1371/journal.pone.0220544
Rankin, J. (1957). Cerebral vascular accidents in patients over the age of 60: II. Prognosis. Scott. Med. J. 2 (5), 200–215. doi:10.1177/003693305700200504
Ranzani, R., Eicher, L., Viggiano, F., Engelbrecht, B., Held, J. P. O., Lambercy, O., et al. (2021). Towards a platform for robot-assisted minimally-supervised therapy of hand function: Design and pilot usability evaluation. Front. Bioeng. Biotechnol. 9 (254), 652380. doi:10.3389/fbioe.2021.652380
Ranzani, R., Lambercy, O., Metzger, J.-C., Califfi, A., Regazzi, S., Dinacci, D., et al. (2020). Neurocognitive robot-assisted rehabilitation of hand function: A randomized control trial on motor recovery in subacute stroke. J. NeuroEngineering Rehabilitation 17 (1), 115. doi:10.1186/s12984-020-00746-7
Ranzani, R., Viggiano, F., Engelbrecht, B., Held, J. P., Lambercy, O., and Gassert, R. (2019). “Method for muscle tone monitoring during robot-assisted therapy of hand function: A proof of concept,” in IEEE 16th International Conference on Rehabilitation Robotics (ICORR) (IEEE), 957–962.
Reissner, L., Fischer, G., List, R., Giovanoli, P., and Calcagni, M. (2019). Assessment of hand function during activities of daily living using motion tracking cameras: A systematic review. Proc. Institution Mech. Eng. Part H J. Eng. Med. 233 (8), 764–783. doi:10.1177/0954411919851302
Rodgers, H., Bosomworth, H., Krebs, H. I., van Wijck, F., Howel, D., Wilson, N., et al. (2019). Robot assisted training for the upper limb after stroke (RATULS): A multicentre randomised controlled trial. Lancet 394 (10192), 51–62. doi:10.1016/s0140-6736(19)31055-4
Röijezon, U., Faleij, R., Karvelis, P., Georgoulas, G., and Nikolakopoulos, G. (2017). A new clinical test for sensorimotor function of the hand–development and preliminary validation. BMC Musculoskelet. Disord. 18 (1), 407. doi:10.1186/s12891-017-1764-1
Scalera, L., Seriani, S., Gallina, P., Di Luca, M., and Gasparetto, A. (2018). An experimental setup to test dual-joystick directional responses to vibrotactile stimuli. IEEE Trans. Haptics 11 (3), 378–387. doi:10.1109/toh.2018.2804391
Schneider, E. J., Lannin, N. A., Ada, L., and Schmidt, J. (2016). Increasing the amount of usual rehabilitation improves activity after stroke: A systematic review. J. Physiother. 62 (4), 182–187. doi:10.1016/j.jphys.2016.08.006
Shah, S. G. S., and Robinson, I. (2007). Benefits of and barriers to involving users in medical device technology development and evaluation. Int. J. Technol. Assess. health care 23 (1), 131–137. doi:10.1017/s0266462307051677
Sivan, M., Gallagher, J., Makower, S., Keeling, D., Bhakta, B., O’Connor, R. J., et al. (2014). Home-based computer assisted arm rehabilitation (hCAAR) robotic device for upper limb exercise after stroke: Results of a feasibility study in home setting. J. neuroengineering rehabilitation 11 (1), 163. doi:10.1186/1743-0003-11-163
Skedung, L., Arvidsson, M., Chung, J. Y., Stafford, C. M., Berglund, B., and Rutland, M. W. (2013). Feeling small: Exploring the tactile perception limits. Sci. Rep. 3 (1), 1–6. doi:10.1038/srep02617
Story, M. F. (2010). “Medical devices in home health care,” in The role of human factors in home health care: workshop summary. Editor S. Olson (Washington, DC: National Academies Press), 145–172.
Tagliamonte, N. L., Scorcia, M., Formica, D., Campolo, D., and Guglielmelli, E. (2011). Effects of impedance reduction of a robot for wrist rehabilitation on human motor strategies in healthy subjects during pointing tasks. Adv. Robot. 25 (5), 537–562. doi:10.1163/016918611x558270
Tong, L. Z., Ong, H. T., Tan, J. X., Lin, J., Burdet, E., Ge, S., et al. (2015). “Pediatric rehabilitation with the reachMAN's modular handle,” in : 2015 37th Annual International Conference of the IEEE Engineering in Medicine and Biology Society (EMBC) (IEEE), 3933–3936.
Virzi, R. A. (1992). Refining the test phase of usability evaluation: How many subjects is enough? Hum. factors 34 (4), 457–468. doi:10.1177/001872089203400407
Ward, N. S., Brander, F., and Kelly, K. (2019). Intensive upper limb neurorehabilitation in chronic stroke: Outcomes from the queen square programme. J. Neurol. Neurosurg. Psychiatry 90 (5), 498–506. doi:10.1136/jnnp-2018-319954
Williams, D. J., Krebs, H. I., and Hogan, N. (2001). “A robot for wrist rehabilitation,” in 2001 Conference Proceedings of the 23rd Annual International Conference of the IEEE Engineering in Medicine and Biology Society (IEEE), 1336–1339.
Wolf, S. L., Sahu, K., Bay, R. C., Buchanan, S., Reiss, A., Linder, S., et al. (2015). The HAAPI (home arm assistance progression initiative) trial: A novel robotics delivery approach in stroke rehabilitation. Neurorehabilitation neural repair 29 (10), 958–968. doi:10.1177/1545968315575612
Woytowicz, E. J., Rietschel, J. C., Goodman, R. N., Conroy, S. S., Sorkin, J. D., Whitall, J., et al. (2017). Determining levels of upper extremity movement impairment by applying a cluster analysis to the Fugl-Meyer assessment of the upper extremity in chronic stroke. Archives Phys. Med. rehabilitation 98 (3), 456–462. doi:10.1016/j.apmr.2016.06.023
Yeong, C. F., Melendez-Calderon, A., Gassert, R., and Burdet, E. (2009). “ReachMAN: A personal robot to train reaching and manipulation,” in 2009 IEEE/RSJ International Conference on Intelligent Robots and Systems (IEEE), 4080–4085.
Keywords: neurorehabilitation, robot-assisted therapy, stroke, hand, robotics, haptics, unsupervised therapy, self-directed therapy
Citation: Ranzani R, Albrecht M, Haarman CJW, Koh E, Devittori G, Held JPO, Tönis FJ, Gassert R and Lambercy O (2023) Design, characterization and preliminary usability testing of a portable robot for unsupervised therapy of hand function. Front. Mech. Eng 8:1075795. doi: 10.3389/fmech.2022.1075795
Received: 20 October 2022; Accepted: 16 December 2022;
Published: 09 January 2023.
Edited by:
Tony Szturm, University of Manitoba, CanadaReviewed by:
Julius Griškevičius, Vilnius Gediminas Technical University, LithuaniaCopyright © 2023 Ranzani, Albrecht, Haarman, Koh, Devittori, Held, Tönis, Gassert and Lambercy. This is an open-access article distributed under the terms of the Creative Commons Attribution License (CC BY). The use, distribution or reproduction in other forums is permitted, provided the original author(s) and the copyright owner(s) are credited and that the original publication in this journal is cited, in accordance with accepted academic practice. No use, distribution or reproduction is permitted which does not comply with these terms.
*Correspondence: Raffaele Ranzani, cmVsYWIucHVibGljYXRpb25zQGhlc3QuZXRoei5jaA==
Disclaimer: All claims expressed in this article are solely those of the authors and do not necessarily represent those of their affiliated organizations, or those of the publisher, the editors and the reviewers. Any product that may be evaluated in this article or claim that may be made by its manufacturer is not guaranteed or endorsed by the publisher.
Research integrity at Frontiers
Learn more about the work of our research integrity team to safeguard the quality of each article we publish.