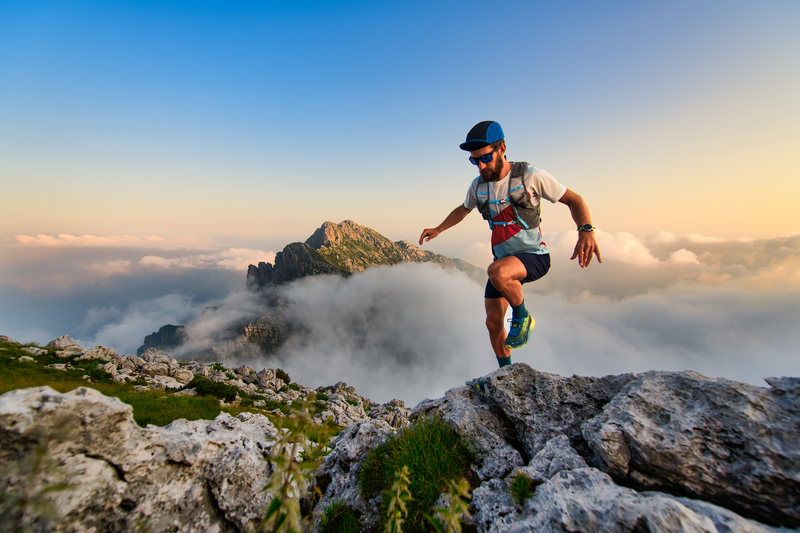
94% of researchers rate our articles as excellent or good
Learn more about the work of our research integrity team to safeguard the quality of each article we publish.
Find out more
ORIGINAL RESEARCH article
Front. Mech. Eng. , 25 November 2022
Sec. Digital Manufacturing
Volume 8 - 2022 | https://doi.org/10.3389/fmech.2022.1003456
The system is designed with the basics of fundamental units termed dimensional analysis (DA). The fundamental units are modeled to figure out some quantitative measures without the knowledge of the system behavior. Subsequently, the dimension analysis-based modeling helps to develop the functional relation of input parameters for the set objectives. The generalized model is validated with the output of experiments with an agreement to adopt the model within a certain range of error. Single-point incremental forming (SPIF) is an innovative sheet metal forming technique in which the metal sheets are shaped as desired without using dedicated dies. The SPIF investigations and declared results are desperately waiting for its industrial acceptability, but the optimization of the process is absent. The current study is to develop the functional relation of input parameters of SPIF through dimensional analysis. The investigation included statistical, ANN, and DA results for R in SPIF. Statistically, the step-down size (Δz; p = 0.005), area of tool end (A; p = 0.048), and wall angle (θ; p = 0.014) are found significant. The modified R-values are lower than the true and ANN modeled R, and its mean error is noted as 6.136. The functional relation confirmed that the step-down size and area of tool end are prominent factors for surface roughness and its influences on output are 150% and 100%, respectively.
The dimensional unit is a fundamental vector that defines the physical significance of basic factors quantitatively. Dimensional analysis (DA) is a technique to reduce complex physical complexities to their simplest forms before any experimental investigation or quantitative analysis (Bridgman, 1931). Its ubiquitous use is in the engineering and science discipline. Some application of dimensional analysis includes heat and mass transfer, engineering mechanics, structural design, astrophysics, aerodynamics, explosions, chemical reactions (Sedov, 1959; Kurth, 1972; Baker et al., 1973; Taylor, 1974; Lokarnik, 1991; Barenblatt, 1996), nuclear reactors (Sonin and Huber, 1978), biology (McMahon and Bonner, 1983), and economics (de Jong, 1967). DA is an important tool to correlate the model with experimental results for unknown behavior of dependent and independent variables. Sometimes, the dimensionless variables are framed to predict the prototype behavior from the measurements on the model. The dimensional model meets with parameters and variables. It also helps to discern between dependent and independent variables such as in mechanical systems, the positions of different parts as function of time. A physical model based on the dimensional analysis in which researchers listed the dependent and independent parameters that significantly affect the system. The list of independent parameters is the basis of the dimensional matrix. In the second step, we derive the null space basis of the dimensional matrix. Finally, the significance of the null space basis to each vector corresponds to a non-dimensional variable that is arranged to an optimal form of the basis for developing the physical model (Price, 2003; Kumar et al., 2013).
Previously, the DA model is proposed in various manufacturing processes. In cold forging, the effect of the shear friction factor (mfd) at the counter punch interface in force requirement is deduced dimensionally (Jung and Im, 2013). Furthermore, the influence of lubricant in cold forging is derived through DA and Π- Buckingham pi-theorem (Pawelski, 1992). Grinding force is estimated in the grinding of K1045 steel through DA and response surface methodology (RSM). The functional relation declared that the depth of cut (d) and the velocity of work (Vw) are the significant factors whereas the spindle speed and the width of cut are reciprocal to them (Alauddin et al., 2007). The length to diameter (L/D) ratio along with convective heat transfer of airs coefficients (05–25 W/m2K) is estimated for the full journal bearing by using dimensionless parameters with the application of the Buckingham pi-theorem. The developed model represented the convective heat transfer coefficient (h) and power consumption (p) as the significant parameters for L/D of full journal bearing (Reddy and Reddy, 2014). The results of DA and ANN for local fault in rolling contact found a very good agreement with the experimental findings (Jamadar and Vakharia, 2016).
Single-point incremental forming (SPIF) is a tremendous forming technique in which the metal sheet is shaped without the use of dedicated dies. Huge SPIF results are reported for its optimization such as formability, surface roughness, springback effect, and tool path but no adequate optimized condition is granted for SPIF due to variation in the material properties as well as undefined process parameters for the SPIF process for particular blank (metal sheet). The forming style of SPIF is mostly suitable for the formation of customized products, rapid prototyping, and/or small batch size production (Park and Kim, 2003; Jeswiet et al., 2005a; AmbrogioDuflou et al., 2007; Oraon and Sharma, 2010). The influences of the sheet thickness, tool diameter, and the wall angle on the induced axial force in the incremental sheet metal forming (ISMF) of steel grades DC04, DC05, and aluminum grades AA1050-O and AA3003-O. The authors concluded the rate of deformation; that is, step-down size and feed rate of the tool is the influential input parameters in SPIF and the tool diameters are responsible for the magnitude of induced forces (Jeswiet et al., 2005b; Ambrogio et al., 2007; Petek et al., 2010). An approximation formula was developed for the computation of axial and radial forces based on a membrane analysis. Furthermore, a similar analysis is performed with the use of a refined material model (Iseki, 2001; Pohlak et al., 2007). Petek et al. analyzed the effect of tool rotation in forming behavior without the lubricated condition. They reported cracks which occurred in the specimen due to excess tool rotation (Petek et al., 2009). The SPIF parameters on thinning of work (strain distribution) and the punch load (induced forces) are estimated statistically and by using genetic algorithm (GA). The finite element method (FEM) result confirmed that the forming forces in steady state SPIF is found to have the greatest error of 6% (Aerens et al., 2010; Bahloul et al., 2014). In continuing research, a medical implant (femoral condylar surface of the knee) of titanium alloy is developed successfully (Oleksik et al., 2010). Hamilton and Jeswiet (2010) studied the influences of the process parameters on the external surface roughness termed as ‘Orange peel effect’ in SPIF. Furthermore, the surface roughness of SPIF parts made of carbon steel (DC01), stainless steel (304), and aluminum (A1050) is measured through various measurement techniques such as grey relational analysis (GRA) and analysis of variance (ANOVA). The authors reported that the surface roughness and wall thickness depends on the step-down size and its contribution on these are 64.19% and 17.23%, respectively (Radu and Cristea, 2013; Patel et al., 2015; Uttarwar et al., 2015; Shah and Chaudhary, 2016). Further SPIF research claimed that the wall angle, step-down size, spindle speed, and tool diameter contributed 69%, 27%, 3%, and 1%, respectively, to the surface roughness (Khatal et al., 2016). An artificial neural network (ANN) is a computer-based numeric solution for predicting the outputs. Sometimes the functional relationship is derived through ANN without a definite knowledge of the parametric behavior of the dependent and independent variables (Kecman, 2001). In SPIF, the outputs such as vertical force component (Fz) and average surface roughness (Ra) are derived through artificial intelligence tools. The authors reported that the coefficient of regression for training, testing, and validation for Fz as 0.960, 0.704, and 0.938, respectively, whereas the overall R2for Ra was noted as 09474 with MAE of 1.068% (Ambrogio et al., 2011; Varthini et al., 2014; Oraon and Sharma, 2018a; Oraon and Sharma, 2018b).
Although SPIF is being under consideration for its optimization through experiments, mathematics, statistics, and simulations, it is trying to develop the functional relation with the help of its input parameters. The present model is derived for the first time with the selective inputs which are significantly affecting the output of the SPIF.
The experiments are conducted on the DT-110 (Mikron Tool Pvt. Ltd., Singapore) in which aluminum grade AA3003-O (blank) is clamped firmly in the fixture. A customized hemispherical end SPIF tool is developed by grooving the face of a 40C5 steel rod (50 mm long and 7 mm diameter) and inserting stainless steel bearing ball of 06 mm diameter (See Figure 1).
The input parameters such as the step-down size/step depth (∆z), forming speeds (rotation speed (R) and tool linear transverse speed (f), thickness of the metal sheet (T), wall angle (θ), and the density of lubrication (D) are taken. The incremental forming started from the outer layer of the square pyramid of base size 35 mm2. Taguchi design of experiment (DoE) is selected for experiments in which two levels of input parameters are set as per L8 orthogonal array as shown in Table 1. The numeric value of high- and low-level input parameters are taken after extensive literature review and pivot experiments.
An interesting observation is reported in SPIF, that is, the strain distribution (thickness of metal sheet) at the corners of the square pyramid is quite different from the conical pyramid (Iseki, 2001; Pohlak et al., 2007; Petek et al., 2009). Since the SPIF process is under investigation, the effect of input parameters is not well defined. Dimensional accuracy with a good surface finish is the prime concern of the customer, therefore the average surface roughness (R) (which reflects the average surface finish of the formed part) is considered as the output response for DA modeling. The atomic force microscopy (AFM) technique is adopted in R measurement. The forming surface generatrix is considered due to incremental depth rather than tool feed and its rotational speed. A sample piece of 5 mm2 is cut from the SPIF part for AF microscopy (See Figure 2). An optical probe scanned 65,536 grits in a single pass. The microscopy results include two- and three-dimensional surface profiles along with average surface roughness (R), peak-to-peak (R), ten-point height (R), and root mean square (R) values.
The significant input parameter for R is calculated with 95% confidence by using the statistical tool MINITAB version 17.0.1. The significance of the input variable on the output factor (R) is confirmed by the value of P. If p ≤ 0.05, the ANOVA results for R are tabulated in Table 2. Statistically, the R-value is influenced by the step-down size (Δz; p = 0.005), area of tool end (A; = 0.048), and the wall angle (θ; p = 0.014) for AA3003-O material.
Furthermore, the artificial intelligence (AI) technique is utilized to predict the R-value. The input parameters taken in the SPIF experiments and measured surface roughness are considered as inputs in ANN modeling. The modeling is done through MATLAB R2010a in which feed-forward back propagation (FFBP) is adopted for the drawing network 6-10-1 (see Figure 3).
Sigmoid transfer function and linear activation function (Purelin) are taken at the hidden and output layer, respectively. The measured Ra is segregated into 60%, 20%, and 20% for training (Exp. No. 1, 3, 4, and 7), testing (Exp. No. 2 and 6), and validation (Exp. No. 5 and 8). Feed-forward back propagation (FFBP) network is trained in the range of 1–15 neurons under the Levenberg–Marquardt (LM) algorithm in which LEARNGDM adaption rate and TRAINLM training function has functioned and finally compared the best-suited coefficient of regression (R-value) for the ANN model.
According to Buckingham’s π-theorem, “A set number of independent parameters (inherent degrees of freedom) may be reduced by the number k. The value of k and the forms of the similarity parameters emerge from dimensional analysis” (Buckingham, 1914). In the present study, the functional relationships among the set of independent input parameters that are responsible for the surface roughness in SPIF are derived by applying Buckingham’s π-theorem. The SPIF input parameter (independent) strongly influenced the dependent variable, that is, average surface roughness (R). In SPIF, the Ra-value is measured in terms of mean differences of the top height to extreme craters. Therefore, the dimensional unit is ”L” and can be written as R (μm) = [L]. The input parameters taken for developing the model are ∆z, A, f, ρ, and σ which symbolized the step-down size depth, area of tool end, feed rate of the tool, ultimate tensile strength (UTS) of the metal sheet, and the density of the metal sheet, respectively. The fundamental units involved in the physical model comprise mass (M), length (L), and time (T). On the other hand, selected input parameters are prominently responsible for the R -value and are taken as an independent variable to correlate the functional relationships among them. The independent parameters along with their dimensional units are listed as follows:
To develop the functional relation of input parameters for deriving the surface roughness of the SPIF formed part, the independent input parameters are taken as a function of the R -value. Therefore, the functional relationship of dependent and independent parameters can be written as
Step 1 The listing of dependent variables forms the dimensional matrix.
Step 2 find the null space, transforming the matrix to reduced row echelon form.
Step 3 interpret the reduced row echelon form.
The leading entry on the (ith, jth) position indicated that the unknown jth is estimated by using the ith equation. Those columns in the coefficient part of the matrix that does not contain leading entries 193 correspond to unknowns that will be arbitrary. The derivatives of the dimensional model are shown 194 in the following equations:
The aforementioned solutions transformed into vector form, so therefore the null space for the given process 202 parameters has a basis. The vector form of null space basis is shown in Eqs 7–9 formed by the 203 set
The non-dimensional variable has only one element that is easily constructed from the solution vector. Therefore, Eqs. 7–9 are simplified and can be written in the form of a non-208 dimensional functional relationship as shown in the following equations:
where C is the constant for variables surface roughness, C is the constant for step depth, and C is the constant for the area of tool end. Since all these constant depends upon the input variables which 214 affected the surface roughness of the finished part. Therefore,
where C is a constant and its value is determined later.
Applying the Buckingham Pie theorem for dimensional relation modeling,
Therefore, the functional relationship among these can be written as
Upon the set of independent input parameters for the Ra-value in the SPIF process, the step-down size (Δz) 222 and the area of tool end (A) are found significant with the magnitude of 100% and 150% 223, respectively. The small ∆z-value may increase the production run time whereas the area of contact 224 interface is responsible for the Ra-value. Regression analysis is the process to get an equation that 225 enables to match the expected outcomes to the experiment result. The R -values measured (true value) in the SPIF of aluminum grade AA3003-O are taken as the primary data set for calculating the dimensionless constant C (Aerens et al., 2010). The two consecutive lowest R -values of low ∆z are considered for 228 finding the dimensionless constant C. The regression analysis provides the best-fitted function Y = 0.924x + 56.30 which corresponds to the value of dimensionless constant C as 0.924. It is also observed that the exponential regression analysis is the best-suited technique to calculate the value of 231 C. The general trend line is calculated by plotting the R -value against the respective set of experiments as shown in Figure 1.
The functional relation of input parameters for surface roughness (R) of the SPIF process is conducted for the first time. During modeling, various process parameters like formability rate, temperate, hardness, 237 toughness, and thermal conductivity of the tool/blank material are not considered in the current study. At 238 lower step depth size, that is, 0.1 mm, the Ra-values are in the range of 50–70 nm which is increased by 239 approximately 50% (90–110 nm) at 0.7 mm step depth. It is also evident that the inputs utilized 240 in ANN target reasonably well. The coefficient of regression, that is, R2 for Ra of AA3003-O is recorded 241 as 0.962.
Upon the set of independent input parameters for the Ra-value in the SPIF process, the step-down size (Δz) 243 and the area of tool end (A) are found significant with the magnitude of 100% and 150% 244, respectively. The small ∆z-value may increase the production run time whereas the area of contact 245 interface is responsible for the Ra-value. The optimized arrangements of the non-dimensional 246 variables are made by presuming that the R -value is zero when significant input parameters are zero, that is,.∆z = A = zero. Thus, the step-down size (∆z) and the area of tool end (A) will never come in the denominator. Hence, Eq. 15 can be simplified and written as
Now, using the value of dimensionless constant C in the proposed model, the modified predicted 252 value of R and error in the model are tabulated in Table 3. This is noticed that the value of the best 253 function calculated Ra is lower than both the R, that is, experimental and ANN R for each experiment.
The general trend line is calculated by plotting Ra against the respective set of experiments as shown in Figure 4. The mean error is calculated by subtracting the best-fitted value from the experimental R in each experiment. The acceptable limit of mean error (6.136) is noted in the present model.
Figure 4 indicates that the best-fitted value of Ra is quite acceptable in comparison to both experimental Ra as well as Ann Ra. An exponential trend line is plotted against the best-fitted Ra which also shows that the developed model for Ra in SPIF has a functional relation between Δz and A as shown in Eq. 16, that is, Ra is majorly dependent on the step-depth size and the area of forming tool imposed during SPIF.
A mathematical model of surface roughness Ra is formulated by considering major input parameters 273 that directly influence the surface roughness of the SPIF product. Some independent parameters are 274 not considered in the present analysis. The functional relationship between the step-down size (∆z) and the area of tool end (A) is noticed in the dimensional analysis for surface roughness in SPIF. However, the influences of others 277 (independent parameters) are not observed but they may be significant for different dependents such as induced forces, tool wear, and tool path. The model is validated and found in a very good agreement with the low-step depth and the area of tool ends at the contact interface between the tool and the metal sheet 280 during SPIF. The error of prediction of the surface roughness using this model is 6.136. The model 281 can, thus, be used to predict the surface roughness in SPIF.
The original contributions presented in the study are included in the article/Supplementary Material; further inquiries can be directed to the corresponding author.
All authors listed have made a substantial, direct, and intellectual contribution to the work and approved it for publication.
The authors declare that the research was conducted in the absence of any commercial or financial relationships that could be construed as a potential conflict of interest.
All claims expressed in this article are solely those of the authors and do not necessarily represent those of their affiliated organizations, or those of the publisher, the editors, and the reviewers. Any product that may be evaluated in this article, or claim that may be made by its manufacturer, is not guaranteed or endorsed by the publisher.
Aerens, R., Eyckens, P., Bael, A. V., and Duflou, J. R. (2010). Force prediction for single point incremental forming deduced from experimental and FEM observations. Int. J. Adv. Manuf. Technol. 46, 9969–12982. doi:10.1007/s00170-009-2160-2
Alauddin, M., Zhang, L. C., and Hashmi, M. S. J. (2007). Grinding force modelling: Combining dimensional analysis with response surface methodology. Int. J. Manuf. Technol. Manag. 12 (1/2/3), 299–310. doi:10.1504/ijmtm.2007.014155
Ambrogio, G., Cozza, V., Filice, L., and Micari, F. (2007). An analytical model for improving precision in single point incremental forming. J. Mater. Process. Technol. 19, 192–395. doi:10.1016/j.jmatprotec.2007.03.079
Ambrogio, G., Filice, L., Guerriero, F., Guido, R., and Umbrello, D. (2011). Prediction of incremental sheet forming process performance by using a neural network approach. Int. J. Adv. Manuf. Technol. 54, 9921–12930. doi:10.1007/s00170-010-3011-x
AmbrogioDuflou, G. J. R., Filice, L., and Aerens, R. (2007). “Some considerations on force trends in Incremental Forming of different materials,” in Proc. of material forming, Zaragoza, Spain, April 18–April 20, 2007, 193–198. doi:10.1063/1.2729510
Bahloul, R., Arfa, H., and Belhadi, H. S. (2014). A study on optimal design of process parameters in single point incremental forming of sheet metal by combining Box-Behnken design of experiments, response surface methods and genetic algorithms. Int. J. Adv. Manuf. Technol. 74, 1163–4185. doi:10.1007/s00170-014-5975-4
Baker, W. E., Westine, P. S., and Dodge, F. T. (1973). Similarity methods in engineering dynamics. Rochelle Park, NJ: Hayden.
Barenblatt, G. I. (1996). Scaling, self-similarity, and intermediate asymptotics. Cambridge, U.K.: Cambridge Univ. Press.
Buckingham, E. (1914). On physically similar systems; illustrations of the use of dimensional equations. Phys. Rev. 4, 345–376. doi:10.1103/physrev.4.345
Hamilton, K., and Jeswiet, J. (2010). Single point incremental forming at high feed rates and rotational speeds: Surface and structural consequences. CIRP Ann. 59, 311–314. doi:10.1016/j.cirp.2010.03.016
Iseki, H. (2001). An approximate deformation analysis and FEM analysis for the incremental bulging of sheet metal using a spherical roller. J. Mat. Process. Technol. 111, 150–154. doi:10.1016/S0924-0136(01)00500-3
Jamadar, I. M., and Vakharia, D. P. (2016). A novel approach integrating dimensional analysis and neural networks for the detection of localized faults in roller bearings. Measurement 94, 177–185. doi:10.1016/j.measurement.2016.07.086
Jeswiet, J., Duflou, J. R., and Szekeres, A. (2005). Forces in single point and two point incremental forming. Adv. Mat. Res. 6, 8449–8456. doi:10.4028/www.scientific.net/AMR.6-8.449
Jeswiet, J., Micari, F., Bramley, A., Duflou, J., and Allwood, J. (2005). Asymmetric single point incremental forming of sheet metal. CIRP Ann. 54 (2), 88–114. doi:10.1016/S0007-8506(07)60021-3
Jung, K. H., and Im, Y. T. (2013). Determination of a dimensionless equation for shear friction factor in cold forging. J. Tribol. 135, 3. doi:10.1115/1.4023856
Khatal, G. D., Borkar, B. R., and Ghadmode, M. M. (2016). Analytical study of SPIF process. Int. J. Adv. Res. Innovative Ideas Educ. 2 (4), 359–363.
Kumar, S., Sharma, V., Choudhary, A. K. S., Chattopadhyaya, S., and Hloch, S. (2013). Determination of layer thickness in direct metal deposition using dimensional analysis. Int. J. Adv. Manuf. Technol. 67, 2681–2687. doi:10.1007/s00170-012-4683-1
Oleksik, V., Pascu, A., Deac, R., Fleaca, R., Bologa, O., and Racz, G. (2010). Experimental study on the surface quality of the medical implants obtained by single point incremental forming. Int. J. Mat. Form. 3, 1935–1938. doi:10.1007/s12289-0100922-x
Oraon, M., and Sharma, V. (2018). Predicting Forces in single point incremental forming by using artificial neural network. Int. J. Eng. Trans. A Basics 31, 88. doi:10.5829/ije.2018.31.01a.13
Oraon, M., and Sharma, V. (2018). Prediction of surface roughness in single point incremental forming of AA3003-O alloy using artificial neural network. Int. J. Mater. Eng. Innovation 9, 1–19. doi:10.1504/IJMATEI.2018.092181
Oraon, M., and Sharma, V. (2010). Sheet metal micro forming: Future research potentials. Int. J. Prod. Industrial Eng. 1, 131–135.
Park, J. J., and Kim, Y. H. (2003). Fundamental studies on the incremental sheet metal forming technique. J. Mater. Process. Technol. 140, 447–453. doi:10.1016/S09240136(03)00768-4
Patel, J. R., Samvatsar, K. S., Prajapati, H. P., and Rangrej, S. S. (2015). Optimization of process parameters for reducing surface roughness produced during single point incremental forming process. Int. J. Recent Technol. Mech. Electr. Eng. 2 (9), 19–23.
Pawelski, O. (1992). Ways and limits of the theory of similarity in application to problems of physics and metal forming. J. Mater. Process. Technol. 34 (1-4), 19–30. doi:10.1016/0924-0136(92)90086-8
Petek, A., Kuzman, K., and Kopac, J. (2009). Deformations and forces analysis of single point incremental sheet metal forming. Archives Material Sci. enggineering 35 (2), 107116.
Petek, A., kuzman, k., and Kopec, J. (2010). Forces and deformations analysis of incremental sheet metal forming. Int. J. Adv. Manuf. Technol. 46, 9969–12982. doi:10.1016/j.mspro.2014.07.130
Pohlak, M., Majak, J., and Kuttner, R. (2007). Manufacturability and limitations in incremental sheet forming. Proc. Est. Acad. Sci. Eng. 13 (2), 129–139.
Price, J. F. (2003). Dimensional analysis of models and data sets. Am. J. Phys. 71, 437–447. doi:10.1119/1.1533057
Radu, M. C., and Cristea, I. (2013). Processing metal sheets by SPIF and analysis of parts quality. Mater. Manuf. Process. 28, 287–293. doi:10.1080/10426914.2012.746702
Reddy, G. M., and Reddy, V. D. (2014). Theoretical investigations on dimensional analysis of ball bearing parameters by using Buckingham pi-theorem. Procedia Eng. 97, 1305–1311. doi:10.1016/j.proeng.2014.12.410
Shah, H., and Chaudhary, S. (2016). Optimization of process parameters for incremental sheet metal forming process. Int. J. Technol. Res. Eng. 3 (7), 1432–1435.
Sonin, A. A., and Huber, P. W. (1978). On the scaling laws for air clearing in water-type pressure suppression systems. J. Heat. Transf. 100, 601–604. doi:10.1115/1.3450863
Uttarwar, P. B., Raini, S. K., and Malwad, D. S. (2015). Optimization of process parameter on surface roughness (Ra) and wall thickness on SPIF using taguchi method. Int. Res. J. Eng. Technol. 2 (9), 781–784.
Varthini, R., Gandhinathan, R., Pandivelan, C., and Jeevanantham, A. K. (2014). Modelling and optimization of process parameters of the single point incremental forming of aluminium 5052 alloy sheet using genetic algorithm-back Propagation neural network. Int. J. Mech. Prod. Eng. 2 (5), 55–62.
SPIF Single-point incremental forming
∆z Step-down size
ISMF Incremental sheet metal forming
A Ultimate tensile strength of work (metal sheet)
GA Genetic algorithm
f Feed rate of tool
GRA Grey relational analysis
ρ Density of lubricant
ANOVA Analysis of variance
R Surface roughness of the formed part
FEM Finite element method
C Dimensionless constant
ANN Artificial neural network
Keywords: SPIF, dimensional analysis, input parameters, surface roughness, modeling
Citation: Oraon M and Sharma V (2022) Deriving the functional relation of input parameters in single-point incremental forming through dimensional analysis. Front. Mech. Eng 8:1003456. doi: 10.3389/fmech.2022.1003456
Received: 26 July 2022; Accepted: 26 October 2022;
Published: 25 November 2022.
Edited by:
Shankar Sehgal, Panjab University, IndiaReviewed by:
Amit Bansal, I. K. Gujral Punjab Technical University, IndiaCopyright © 2022 Oraon and Sharma. This is an open-access article distributed under the terms of the Creative Commons Attribution License (CC BY). The use, distribution or reproduction in other forums is permitted, provided the original author(s) and the copyright owner(s) are credited and that the original publication in this journal is cited, in accordance with accepted academic practice. No use, distribution or reproduction is permitted which does not comply with these terms.
*Correspondence: Manish Oraon, bW9yYW9uQGJpdG1lc3JhLmFjLmlu
Disclaimer: All claims expressed in this article are solely those of the authors and do not necessarily represent those of their affiliated organizations, or those of the publisher, the editors and the reviewers. Any product that may be evaluated in this article or claim that may be made by its manufacturer is not guaranteed or endorsed by the publisher.
Research integrity at Frontiers
Learn more about the work of our research integrity team to safeguard the quality of each article we publish.