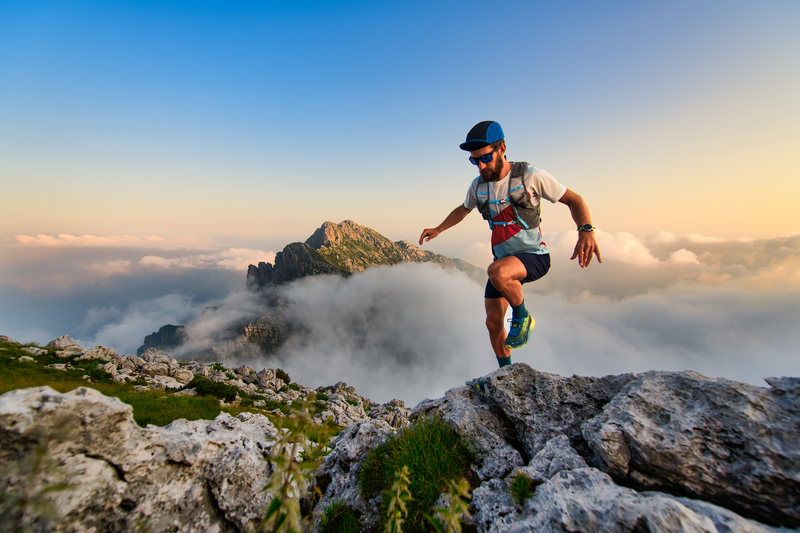
95% of researchers rate our articles as excellent or good
Learn more about the work of our research integrity team to safeguard the quality of each article we publish.
Find out more
ORIGINAL RESEARCH article
Front. Mech. Eng. , 18 October 2021
Sec. Micro- and Nanoelectromechanical Systems
Volume 7 - 2021 | https://doi.org/10.3389/fmech.2021.674860
This article is part of the Research Topic Advances in Brain Mechanics View all 12 articles
It is well known that the mechanical behavior of arterial walls plays an important role in the pathogenesis of vascular diseases. Most studies existing in the literature focus on the mechanical interactions between the blood flow and wall’s deformations. However, in the brain, the smaller vessels experience not only oscillatory forces due to the pulsatile blood flow but also structural and morphological changes controlled by the surrounding brain cells. In this study, the mechanical deformation of the cerebral arterial wall caused by the pulsatile blood flow and the dynamics of the neuronal nitric oxide (NO) is investigated. NO is a small diffusive gaseous molecule produced by the endothelial cells and neurons, which is involved in the regulation of cerebral blood flow and pressure. The cerebral vessel is assumed to be a hollow axial symmetric cylinder whose wall thickness is much smaller than the cylinder’s radius and longitudinal length is much less than the propagating wavelength. The wall is an isotropic, homogeneous linear viscoelastic material described by an NO-modulated variable-order fractional Maxwell model. A fractional telegraph equation is obtained for the axial component of the displacement. Patterns of wall’s deformation are investigated through numerical simulations. The results suggest that a significantly decreased inactivation of the neuronal NO may cause a reduction in the shear stress at the blood-vessel interface, which could lead to a decrease in the production of shear-induced endothelial NO and neurovascular disease.
Cerebral vasculature plays a critical role in brain’s metabolism and neurovascular conditions. The literature abounds with studies of cerebral blood flow and its interactions with the vasculature and brain cells [reviews of models and computer simulations can be found in chapter 4 of Drapaca and Sivaloganathan (2019)]. Recent advancements in technology have allowed researchers to gain invaluable knowledge about the intricate chemo-mechanical connections among neurons, glial, vascular, and blood cells. It is now acknowledged that the neurons and glial cells control the cerebral blood flow through the chemo-mechanical activation of the cells within the vascular wall (Attwell et al., 2010).
One of the many particles that facilitate the chemo-mechanical communications among the brain cells, blood, and the vascular wall is the nitric oxide (NO). A small diffusive gaseous molecule, the cerebral NO is mainly produced by shear-induced mechanotransduction at the blood-vessel interface (Sriram et al., 2016) and by synthesis reactions within the endothelial cells of the blood vessels and neurons (Forstermann and Sessa, 2012). NO diffuses and is removed from brain through some specialized chemical processes (Palacios-Callender et al., 2007; Unitt et al., 2010; Santos et al., 2011; Santos et al., 2012; Helms et al., 2016). In its role as a neuro-glial-vascular messenger, NO controls the cerebral blood flow and the release of neurotransmitters (Huang, 1999; Iadecola, 2004; Attwell et al., 2010; Contestabile et al., 2012; Iadecola, 2017). The regulation of the blood flow in brain is achieved through vasomotor mechanisms in which both the neuronal and endothelial NO are involved (Cockcroft, 2005; Metea and Newman, 2006; Attwell et al., 2010; Atochin and Huang, 2011; Petzold and Murthy, 2011; Contestabile et al., 2012; Lourenco et al., 2014; Haselden et al., 2020). However, throughout the entire cardiovascular system, the endothelial NO usually acts as a vasodilator (Attwell et al., 2010; Schuler et al., 2014). While the cerebral NO activity causes the local vasodilatation of downstream cerebral vessels at the neuro-glial-vascular unit site, the NO diffusion within the vascular wall relaxes the smooth muscle cells leading to the so-called remote vasodilation due to the propagation of muscle’s relaxation to the upstream arteries via the intercellular communications among endothelial and smooth muscle cells (Freed and Gutterman, 2017) facilitated by vascular gap junctions (Iadecola, 2004; Iadecola, 2017). Impaired cerebral NO production and/or decay can signal the presence of a neurovascular disease (Parker and Parks, 1995; Maurer et al., 2000; Wilkinson et al., 2004; Unitt et al., 2010; Santos et al., 2011; Haselden et al., 2020).
Although the NO-modulated vasodilation contributes to the mechanical deformation of the vascular walls of intracerebral vessels, existing mathematical models and corresponding numerical simulations of blood flow interacting with the deformable vascular wall do not account for it since their focus is the mechanics of big arteries in the presence of the pulsatile blood flow. The arteries are usually modeled as anisotropic, incompressible, nonlinear elastic materials whose constitutive stress-strain relationships may also incorporate collagen fibers’ orientations and waviness, and/or the activation of smooth muscle cells (see Ebrahimi, 2009; Holzapfel and Ogden, 2010; Kim and Wagenseil, 2014; Espinosa et al., 2018 and references within). For instance, muscle activation has been modeled using 1) the (original or modified) Hill model (Hill, 1938), 2) an elastic strain-energy function dependent on the concentration of free intracellular calcium (Rachev and Hayashi, 1999), 3) a strain-energy function dependent on the chemical kinetics of the smooth muscle (Stalhand et al., 2008), and 4) a lumped Hodgkin-Huxley-like electrical circuit of the smooth muscle cell membrane coupled with a fluid compartment model describing the mass balances of considered ions and a contractile kinematics model regulated by intracellular calcium (Yang et al., 2003). Given the viscoelastic behavior of constituent cells (Kasza et al., 2007) of the blood vessels (and biological tissues, in general), the vascular wall has also been modeled as a viscoelastic material (Toth et al., 1998; Orosz et al., 1999; Holzapfel et al., 2002; Hodis and Zamir, 2008; Ebrahimi, 2009). Since these models do not incorporate the NO influence on the vascular wall, they are not able to predict neurovascular pathologies in which NO plays a critical role. Furthermore, the coupling of most of the above models with the cerebral NO dynamics will probably increase the already large number of model parameters that are practically impossible to find in a living brain with minimally invasive procedures using present-day technologies.
The aim of this study is to propose a novel mechanical model for cerebral arterioles that accounts for changes in the wall’s mechanical behavior due to the NO-activated vascular cells and has few parameters. Orosz et al. (1999) used stress-relaxation measurements in cerebral arteries of human cadavers to show that four- and five-element generalized Maxwell viscoelastic models provide more accurate descriptions of the vascular wall mechanics than the two-element Maxwell viscoelastic model. One way to obtain a linear viscoelastic constitutive law with fewer parameters that perform as well as (or better) a spring-dashpot model with many elements is to use an integral formulation instead of the differential operator representation commonly associated with rheological (spring-dashpot) models. Integral constitutive laws admit equivalent differential formulations only for certain expressions of their relaxation functions (Gurtin and Sternberg, 1965; Drapaca et al., 2007). It was observed experimentally that relaxation functions represented as power functions of negative fractional exponents accurately describe the fading memory of many viscoelastic materials, including polymers and soft biological tissues (Nutting, 1921; Gemant, 1935; Gemant, 1936; Scott Blair and Coppen, 1939; Scott Blair and Coppen, 1942; Nutting, 1943; Guttinger, 1966; Caputo and Mainardi, 1971; Bagley and Torvik, 1983a; Bagley and Torvik, 1983b; Koeller, 1984; Torvik and Bagley, 1984; Suki et al., 1994; Mainardi, 2010). Integral constitutive laws with decaying fraction power-law relaxation functions have equivalent differential representations like those used in classic rheological models where integer-order derivatives were replaced by fractional-order derivatives (convolutions between decaying fraction power functions and integer-order derivatives). These fractional viscoelastic models are causal at zero time (Bagley and Torvik, 1983b; Torvik and Bagley, 1984) and can be derived from molecular theories that incorporate the molecular complexity of various polymers (Bagley and Torvik, 1983b; Suki et al., 1994). In this context, the fractional order of the strain history models the “contribution of the long-chain molecules to the macroscopic stress” (Suki et al., 1994). Also, Suki et al. (1994) noticed the structural similarities between the lung tissue and some polymers and showed that a fractional viscoelastic model can successfully predict the viscoelastic behavior of lung tissue. Given that lungs are highly vascularized, it is reasonable to assume that a fractional viscoelastic model could be employed in studies of blood vessels mechanics.
Therefore, this study proposes a new NO-modulated variable-order fractional Maxwell viscoelastic model for the cerebral vascular wall and investigates its predictions through numerical simulations. The suggested constitutive equation is a generalization of the (constant order) fractional Maxwell viscoelastic model (see, for instance, Mainardi, 2010). The classic (first-order) Maxwell viscoelastic model has been previously used in the literature to model vascular walls (see, for instance, Hodis and Zamir, 2008), and therefore, this is the model which was chosen to be generalized here. According to Lorenzo and Hartley (2002), variable-order fractional operators are suitable in modeling, for instance, the fading memory characteristic of viscoelastic materials and the order memory which records the order in which memories are recalled [a recent review of applications of variable-order fractional operators can be found in Patnaik et al. (2020)]. In the model proposed here, the neuronal NO dynamics controls the order memory. With only three parameters, the proposed model can account for vessel’s mechanics and the fact that the order memory of chemo-mechanical events is essential to the proper functionality of the constituent cells of the vascular wall. Lastly, the vascular wall is assumed to be isotropic and homogeneous.
As in Hodis and Zamir (2008), it is further assumed that the cerebral vessel is a hollow axial symmetric cylinder whose wall thickness is much smaller than the cylinder’s radius, and longitudinal length is much less than the propagating wavelength. These assumptions together with the new constitutive equation led to a variable-order fractional telegraph equation for the unknown axial displacement of the vessel’s wall. By assuming that the blood-vessel interface was exposed to the pulsatile blood flow and the vessel-tissue interface was tethered (Hodis and Zamir, 2008), an initial-boundary value problem was obtained and solved using the separation of variables method and finite difference schemes. The assumption of a tethered vessel-tissue interface is supported by experiments performed using high-resolution ultrasonic scanning that shows significantly larger longitudinal displacements of the wall’s middle layers than the corresponding displacements of the wall’s outer layers (Persson et al., 2003; Cinthio et al., 2006). Lastly, the variable fractional order was taken to be proportional to the concentration of neuronal NO. Two cases of neuronal NO synthesis (NOS) were considered: 1) a stepwise activation of NO with drastically decreased NO inactivation and 2) a dynamic activation of NO (Hall and Garthwaite, 2006). Case 1 may not be physiological because the main neuronal NOS activation matches the short pulsatile Ca2+- calmodulin binding activation in dendritic spines (Hall and Garthwaite, 2006), and thus, this case may model impaired neuronal NO dynamics. Indeed, the temporal variation of NO resembles a stepwise function when the NO inactivation is drastically reduced by either brain ischemia (Santos et al., 2011) or the inhibition of cytochrome c oxidase (COX) (Palacios-Callender et al., 2007; Unitt et al., 2010). Brains of Alzheimer disease (AD) patients may suffer from a COX inhibition and thus an accumulation of NO (primarily in the temporal cortex and hippocampus) because a selective defect of COX causing a significant reduction in COX activity was found in AD brains (Parker and Parks, 1995; Maurer et al., 2000). Numerical simulations generated in MATLAB show smaller displacements within the vascular wall in the case of stepwise NOS than in the case of dynamic NOS. Also, the shear stress at the bloodvessel interface is smaller in the case of stepwise neuronal NOS. Since the production of shear-induced endothelial NO is proportional to the shear stress at the inner boundary of the vessel’s wall (Sriram et al., 2016), it follows that less endothelial NO will be produced in this case which could ultimately lead to neurovascular disease. Thus, the model could be used as a complementary clinical tool for early detection of disease and intervention (Alzheimer, for instance).
The study is structured as follows. The proposed mathematical model and the initial-boundary value problem under investigation are presented in Mathematical Model. The corresponding semi-analytic solution is given in Semi-Analytic Solution. Numerical simulations are shown in Results, which is followed by the last section containing a discussion of the results and final conclusions.
The vessel is modeled as a hollow horizontal axial symmetric circular cylinder of radius
for
FIGURE 1. A schematic of the geometric domain occupied by a vessel of radius
In this study, it is assumed that the mechanical behavior of the vessel’s wall is described by the constitutive equation of a variable-order fractional Maxwell linear viscoelastic material:
where, based on the model’s assumptions, the infinitesimal strain
The constitutive Eq. 3 has three physical parameters:
where
According to Ramirez and Coimbra (2010), Eq. 5 is desirable in modeling of physical processes since a physical interpretation of the variable fractional order
Equation 6 yields
From Eq. 5 and the zero initial conditions satisfied by
where
FIGURE 2. Plots of dimensionless material functions (A)
Replacing Eq. 4 in Eq. 3, applying operator
Differentiating Eq. 8 with respect to the spatial variable
Lastly, by replacing Eq. 9 in Eq. 2 the following equation is obtained:
Eq. 10 is a variable-order fractional telegraph equation (or two-term time-variable fractional diffusion-wave equation).
The initial and boundary conditions are as follows:
It is assumed that the variable fractional order
whose approximate analytic solution for zero initial condition is (Mehala and Rajendran, 2014) as follows:
The equation of the second model is as follows:
whose approximate analytic solution for zero initial condition is (Mehala and Rajendran, 2014) as follows:
Here,
TABLE 1. List of parameters with corresponding values and units. Due to a lack of experimental data, the value of
FIGURE 3. Plots of NO concentrations versus the dimensionless time
The aim of the study is to find a semi-analytic solution to the initial-boundary value problem (10–12) for two variable orders given by scaled Eqs 13, 14. The first step is to formulate a corresponding non-dimensional problem. By introducing the dimensionless quantities:
the initial-boundary value problem (10–12) transforms into the following dimensionless form:
In Eq. 15,
A classic approach of solving initial-boundary value problems for partial differential equations is used. Look for a solution to problem (15–17) of the form:
such that
By substituting Eq. 20 in problem (15–17), the following initial-boundary value problem for
The first step in solving problem (21–23) is to look for a solution to the corresponding homogeneous equation:
The method of separation of variables suggests looking for a solution of the form
Thus, the eigenfunctions satisfying problem (25) are
Substituting Eq. 26 in problem (21, 22) and using the half-range Fourier sine series expansions of the functions
Let
By replacing expression (29) in problem (27, 28), the following problem for the unknown function
where
Thus, the last step is finding a solution to problem (30, 31). If the fractional order is a constant
Let
where
The semi-analytic solution to problem (15–17) is then obtained by replacing the discrete solution (35) for
The shear stress distribution within the vessel’s wall is obtained from the dimensionless form of Eq. 2 by integration (Hodis and Zamir, 2008):
where the following non-dimensionalization was used for the shear stress:
Numerical scheme Eq. 35 was implemented in MATLAB, and plots of the distributions of dimensionless displacements within the vessel’s wall (Eq. 32) and the dimensionless shear stress at the blood-vessel interface (Eq. 37) were generated for two expressions of the variable fractional order: the stepwise NOS and the dynamic NOS. The values of the parameters used in the numerical simulations are given in Table 1. Numerical simulations used a step size
Figure 4 shows temporal profiles of the dimensionless axial displacement
FIGURE 4. Plots of the dimensionless axial displacement
Figures 5, 6 show spatio-temporal variations of the dimensionless axial displacement
FIGURE 5. Spatio-temporal variations of the dimensionless axial displacement
FIGURE 6. Spatio-temporal variations of the dimensionless axial displacement
FIGURE 7. Plots of the dimensionless axial displacement
To better understand the differences noticed between the oscillatory patterns corresponding to the two cases of stepwise NOS and dynamic NOS, the dimensionless shear stress at the blood-vessel interface was calculated using Eq. 37 and MATLAB’s built-in function gradient. The results of this comparison are shown in Figure 8. The amplitude of the shear stress is bigger in the case of dynamic NOS which was expected given that the amplitude of the axial displacement is also bigger in this case. By varying the scaling factor of order
FIGURE 8. Plots of the dimensionless shear stress at the bloodvessel interface
The main contribution of this study is modeling the wall of a cerebral blood vessel using an NO-modulated variable-order fractional Maxwell viscoelastic model. The variable fractional order is assumed to be proportional to the neuronal NO dynamics, and thus, the order memory introduced by this choice guides the pattern of the fading memory of this viscoelastic material. Two cases of NOS are considered: a stepwise activation of NO and a dynamic activation of NO. Following the approach of Hodis and Zamir (2008), a variable-order fractional telegraph equation for the axial displacement of the wall was obtained which was solved under the assumptions that the outer boundary of the vascular wall was tethered, and the inner boundary of the wall was exposed to the pulsatile blood flow. Numerical simulations were created in MATLAB using numerical scheme (Eq. 35) and Garrappa’s function MT_FDE_PI12_PC (Garrappa, 2018) for constant fractional orders. The function MT_FDE_PI12_PC was used to validate scheme (Eq. 35) in the case of constant fractional orders. The main finding of these simulations is that a significantly decreased inactivation of the neuronal NO causes a reduction in the shear stress at the blood-vessel interface which could lead to a decrease in the production of shear-induced endothelial NO and ultimately to neurovascular disease.
Testing the computer code for various values of the parameters
Emerging imaging techniques could help validate the proposed model in animal models and thus make the model relevant to clinical applications. For instance, a multimodal in vivo magnetic resonance (MR)/electron paramagnetic resonance (EPR) spectroscopy/fluorometry could be used to visualize NO production and spatio-temporal distribution (Sharma et al., 2014). Also, intravascular optical coherence tomography could be used for the in vivo real-time estimation of the vascular stiffness (Potlov et al., 2020). By combining these imaging techniques and the high-resolution ultrasonic scanning of Persson et al. (2003) and Cinthio et al. (2006) for the visualization of wall’s longitudinal displacements, the cerebral NO dynamics and vascular wall mechanics may be investigated simultaneously. This approach can be used to estimate
Until the above-mentioned imaging techniques are adapted and approved for clinical applications, animal models can be used to find healthy physiological ranges for the model’s parameters and investigate the potential of using these parameters as biomarkers. For example, animal models of cerebral ischemia have shown that the decreasing amount of endothelial NO will act as a protective agent for a few minutes after the injury, while the amount of neuronal NO will increase causing neuronal injury (Huang, 1999; Wei et al., 1999). If the production of shear-induced endothelial NO mediated by the blood flow ceases to happen following ischemia, then the stepwise concentration of neuronal NO is a consequence of the significantly decreased NO inactivation caused by ischemia (Santos et al., 2011) and thus, according to the prediction made by the proposed model, may contribute to the reduction of the localized endothelial NO produced by the lower shear stress in the vessel wall at its inner boundary. Thus, the model’s prediction may explain the interplay between the endothelial and neuronal NO seen in cerebral ischemia. Lastly, model’s parameters estimated using the multimodal in vivo imaging techniques mentioned above could suggest the presence of cerebral ischemia and thus be used as a complementary diagnostic tool.
Improving the mathematical model may provide more sensitive biomarkers and helps avoiding the numerical stiffness issue mentioned earlier. According to Iadecola (2004), the NO-modulated local vasodilation of the intracerebral arterioles and capillaries propagates upstream in the vascular network which causes an increase of blood flow in the upstream arteries that leads to increased shear stress at the blood-vessel interface and thus an increase in the amount of shear-induced endothelial NO and a further flow-mediated vasodilation. The proposed model represents the blood flow as an inner boundary condition, and thus, this mechanism of global production of shear-induced endothelial NO is not accounted for. Thus, the decrease in the local production of the shear-induced endothelial NO due to a stepwise neuronal NOS predicted by the model proposed in this study might not be significant enough to cause adverse effects if the cerebral blood flow is intact. Coupling the deformation of the vessel’s wall and the blood flow and incorporating in the model the mechanism described above should provide a better prediction of the amount of shear-induced endothelial NO and the possible role that the neuronal NO dynamics may play in this process. Introducing more detailed information about the complex, multi-layered structure of the vascular wall could also enhance the accuracy of model’s predictions. Lastly, the full three-dimensional fluid-structure interaction problem should be formulated and solved since the simplifying assumptions made by Hodis and Zamir (2008) and implemented here that reduced the model to a one-dimensional problem might not be valid for cerebral arterioles given the overly complex geometry of the cerebral vascular network (Reina-De La Torre et al., 1998). The parameters of this enhanced mathematical model could be found either from in vitro/in situ measurements or from in vivo, real-time observations using medical imaging techniques.
In conclusion, the study proposes a novel NO-modulated variable-order fractional Maxwell viscoelastic model of the cerebral arterioles and investigates the effects of the neuronal NOS on the mechanical behavior of the vessel’s wall. Numerical simulations show how neuronal NO dynamics influence the deformation and shear stress within the vascular wall. A generalization of this model to a three-dimensional geometry and the incorporation of the blood flow into the model should provide a better understanding of the coupling between NO dynamics and mechanical damage and their combined role in neurovascular diseases.
The original contributions presented in the study are included in the article/supplementary material; further inquiries can be directed to the corresponding author.
CD stated the problem under investigation, proposed the mathematical model and its semi-analytic solution, implemented code in MATLAB, generated results, and wrote the paper.
The authors declare that the research was conducted in the absence of any commercial or financial relationships that could be construed as a potential conflict of interest.
All claims expressed in this article are solely those of the authors and do not necessarily represent those of their affiliated organizations, or those of the publisher, the editors and the reviewers. Any product that may be evaluated in this article, or claim that may be made by its manufacturer, is not guaranteed or endorsed by the publisher.
Attwell, D., Buchan, A. M., Charpak, S., Lauritzen, M., MacVicar, B. A., and Newman, E. A. (2010). Glial and Neuronal Control of Brain Blood Flow. Nature 468, 232–243. doi:10.1038/nature09613
Bagley, R. L., and Torvik, P. J. (1983b). A Theoretical Basis for the Application of Fractional Calculus to Viscoelasticity. J. Rheology 27, 201–210. doi:10.1122/1.549724
Bagley, R. L., and Torvik, P. J. (1983a). Fractional Calculus - A Different Approach to the Analysis of Viscoelastically Damped Structures. AIAA J. 21, 741–748. doi:10.2514/3.8142
Bazhlekova, E., and Bazhlekov, I. (2017). Stokes' First Problem for Viscoelastic Fluids with a Fractional Maxwell Model. Fractal Fract 1, 7. doi:10.3390/fractalfract1010007
Blair, G. W. S., and Caffyn, J. (1942). The Classification of the Rheological Properties of Industrial Materials in the Light of Power-Law Relations between Stress, Strain and Time. J. Sci. Instrum. 19, 88–93. doi:10.1088/0950-7671/19/6/303
Caputo, M., and Mainardi, F. (1971). A New Dissipation Model Based on Memory Mechanism. Pageoph 91, 134–147. doi:10.1007/bf00879562
Cinthio, M., Ahlgren, Å. R., Bergkvist, J., Jansson, T., Persson, H. W., and Lindström, K. (2006). Longitudinal Movements and Resulting Shear Strain of the Arterial wall. Am. J. Physiology-Heart Circulatory Physiol. 291 (1), H394–H402. doi:10.1152/ajpheart.00988.2005
Cockcroft, J. (2005). Exploring Vascular Benefits of Endothelium-Derived Nitric Oxide. Am. J. Hypertens. 18, 177–183. doi:10.1016/j.amjhyper.2005.09.001
Contestabile, A., Monti, B., and Polazzi, E. (2012). Neuronal-glial Interactions Define the Role of Nitric Oxide in Neural Functional Processes, Curr. Neuropharmacology 10, no. 4, 303–310. doi:10.2174/157015912804143522
Drapaca, C. S., and Sivaloganathan, S. (2019). Mathematical Modelling and Biomechanics of the Brain. New York, USA: Fields Institute Monographs, Springer Science+Business Media, LLC. doi:10.1007/978-1-4939-9810-4
Drapaca, C. S., Sivaloganathan, S., and Tenti, G. (2007). Nonlinear Constitutive Laws in Viscoelasticity. Maths. Mech. Sol. 12, 475–501. doi:10.1177/1081286506062450
Ebrahimi, A. P. (2009). Mechanical Properties of normal and Diseased Cerebrovascular System. J. Vasc. Interv. Neurol. 2 (2), 155–162.
Forstermann, U., and Sessa, W. C. (2012). Nitric Oxide Synthases: Regulation and Function. Eur. Heart J. 33, 829–837. doi:10.1093/eurheartj/ehr304
Freed, J. K., and Gutterman, D. D. (2017). Communication Is Key: Mechanisms of Intercellular Signaling in Vasodilation. J. Cardiovasc. Pharmacol. 69 (5), 264–272. doi:10.1097/FJC.0000000000000463
Gabriela Espinosa, M., Catalin Staiculescu, M., Kim, J., Marin, E., and Wagenseil, J. E. (2018). Elastic Fibers and Large Artery Mechanics in Animal Models of Development and Disease. J. Biomech. Eng. 140 (2), 0208031–02080313. doi:10.1115/1.4038704
Garrappa, R. (2018). Numerical Solution of Fractional Differential Equations: A Survey and a Software Tutorial. Mathematics 6, 16. doi:10.3390/math6020016
Gemant, A. (1936). A Method of Analyzing Experimental Results Obtained from Elasto‐Viscous Bodies. Physics 7, 311–317. doi:10.1063/1.1745400
Gemant, A. (1935). Compressional Waves in media with Complex Viscosity. Physics 6, 363–365. doi:10.1063/1.1745278
Gorenflo, R., Kilbas, A. A., Mainardi, F., and Rogosin, S. V. (2014). Mittag-Leffler Functions, Related Topics and Applications. New York, USA: Springer-Verlag. doi:10.1007/978-3-662-43930-2_6
Gurtin, M., and Sternberg, E. (1965). On the Linear Theory of Viscoelasticity. Archive Rational Mech. Anal. 11, 291–356.
Guttinger, W. Generalized Functions and Dispersion Relations in Physics. Fortschritte der Physik 14, 483–602. doi:10.1002/prop.19660140114
Hall, C. N., and Garthwaite, J. (2006). Inactivation of Nitric Oxide by Rat Cerebellar Slices. J. Physiol. 577 (2), 549–567. doi:10.1113/jphysiol.2006.118380
Haselden, W. D., Kedarasetti, R. T., and Drew, P. J. (2020). Spatial and Temporal Patterns of Nitric Oxide Diffusion and Degradation Drive Emergent Cerebrovascular Dynamics. Plos Comput. Biol. 16 (7), e1008069. doi:10.1371/journal.pcbi.1008069
Helms, C. C., Liu, X., and Kim-Shapiro, D. B. (2017). Recent Insights into Nitrite Signaling Processes in Blood. Biol. Chem. 398, 319–329. doi:10.1515/hsz-2016-0263
Hill, A. V. (1938). The Heat of Shortening and the Dynamic Constants of Muscle. Proc. R. Soc. Lond. B 126, 136–195. doi:10.1098/rspb.1938.0050
Hodis, S., and Zamir, M. (2008). Solutions of the Maxwell Viscoelastic Equations for Displacement and Stress Distributions within the Arterial wall. Phys. Rev. E Stat. Nonlin Soft Matter Phys. 78, 021914. doi:10.1103/PhysRevE.78.021914
Holzapfel, G. A., Gasser, T. C., and Stadler, M. (2002). A Structural Model for the Viscoelastic Behavior of Arterial walls: Continuum Formulation and Finite Element Analysis. Eur. J. Mech. - A/Solids 21, 441–463. doi:10.1016/s0997-7538(01)01206-2
Holzapfel, G. A., and Ogden, R. W. (2010). Constitutive Modelling of Arteries. Proc. R. Soc. A. 466, 1551–1597. doi:10.1098/rspa.2010.0058
Huang, P. L. (1999). Neuronal and Endothelial Nitric Oxide Synthase Gene Knockout Mice. Braz. J. Med. Biol. Res. 32, 1353–1359. doi:10.1590/s0100-879x1999001100005
Iadecola, C. (2004). Neurovascular Regulation in the normal Brain and in Alzheimer's Disease. Nat. Rev. Neurosci. 5, 347–360. doi:10.1038/nrn1387
Iadecola, C. (2017). The Neurovascular Unit Coming of Age: a Journey through Neurovascular Coupling in Health and Disease. Neuron 96, 17–42. doi:10.1016/j.neuron.2017.07.030
IT’IS Foundation (2021). Tissue Properties Database: Density. Available at: https://itis.swiss/virtual-population/tissue-properties/database/density/. Zurich: Zurich43.
Kasza, K. E., Rowat, A. C., Liu, J., Angelini, T. E., Brangwynne, C. P., Koenderink, G. H., et al. (2007). The Cell as a Material. Curr. Opin. Cel Biol. 19, 101–107. doi:10.1016/j.ceb.2006.12.002
Kim, J., and Wagenseil, J. E. (2014). Bio-chemo-mechanical Models of Vascular Mechanics. Ann. Biomed. Eng. 43, 1477–1487. doi:10.1007/s10439-014-1201-7
Koeller, R. C. (1984). Applications of Fractional Calculus to the Theory of Viscoelasticity. ASME J. Appl. Mech. 51, 299–307. doi:10.1115/1.3167616
Lorenzo, C. F., and Hartley, T. T. (2002). Variable Order and Distributed Order Fractional Operators. NASA/TM-2002-211376, New York, USA: Springer Link. doi:10.1023/A:1016586905654
Lourenço, C. F., Santos, R. M., Barbosa, R. M., Cadenas, E., Radi, R., and Laranjinha, J. (2014). Neurovascular Coupling in hippocampus Is Mediated via Diffusion by Neuronal-Derived Nitric Oxide. Free Radic. Biol. Med. 73, 421–429. doi:10.1016/j.freeradbiomed.2014.05.021
Luchko, Y., and Gorenflo, R. (1999). An Operational Method for Solving Fractional Differential Equations with the Caputo Derivatives. Acta Mathematica Vietnamica 24 (2), 207–233.
Mainardi, F. (2010). Fractional Calculus and Waves in Linear Viscoelasticity. London, UK: Imperial College Press.
Maurer, I., Zierz, S., and Moller, H.-J. (2000). A Selective Defect of Cytochrome C Oxidase Is Present in Brain of Alzheimer Disease Patients. Neurobiol. Aging 21, 455–462. doi:10.1016/s0197-4580(00)00112-3
Medical Physiology, 3rd Edition: Elastic Properties of Blood Vessels (2021). https://doctorlib.info/physiology/medical/104.html. Chicago: Doctorlib.
Mehala, N., and Rajendran, L. (2014). Analytical Solutions of Nonlinear Differential Equations in the Mathematical Model for Inactivation of Nitric Oxide by Rat Cerebellar Slices. Ajac 05, 908–919. doi:10.4236/ajac.2014.514099
Metea, M. R., and Newman, E. A. (2006). Glial Cells Dilate and Constrict Blood Vessels: A Mechanism of Neurovascular Coupling. J. Neurosci. 26 (11), 2862–2870. doi:10.1523/jneurosci.4048-05.2006
Moghaddam, B. P., and Machado, J. A. T. (2017). Extended Algorithms for Approximating Variable Order Fractional Derivatives with Applications. J. Sci. Comput. 71, 1351–1374. doi:10.1007/s10915-016-0343-1
N. Atochin, D., and L. Huang, P. (2011). Role of Endothelial Nitric Oxide in Cerebrovascular Regulation. Cpb 12 (9), 1334–1342. doi:10.2174/138920111798280974
Nutting, P. G. (1921). A Study of Elastic Viscous Deformation. Proc. Amer. Soc. Test. Mater. 21, 1162–1171. doi:10.2307/3407697
Nutting, P. G. (1943). A General Stress-Strain-Time Formula. J. Franklin Inst. 235, 513–524. doi:10.1016/s0016-0032(43)91483-8
Orosz, M., Molnarka, G., Toth, M., Nadasy, G. L., and Monos, E. (1999). Viscoelastic Behavior of Vascular wall Simulated by Generalized Maxwell Models – a Comparative Study. Med. Sci. Monit. 5 (3), 549–555.
Palacios-Callender, M., Hollis, V., Mitchison, M., Frakich, N., Unitt, D., and Moncada, S. (2007). Cytochrome C Oxidase Regulates Endogenous Nitric Oxide Availability in Respiring Cells: a Possible Explanation for Hypoxic Vasodilation. Proc. Natl. Acad. Sci. 104 (47), 18508–18513. doi:10.1073/pnas.0709440104
Parker, W. D., and Parks, J. K. (1995). Cytochrome C Oxidase in Alzheimer's Disease Brain. Neurology 45, 482–486. doi:10.1212/wnl.45.3.482
Patnaik, S., Hollkamp, J. P., and Semperlotti, F. (2020). Applications of Variable-Order Fractional Operators: a Review, Proc. R. Soc. A. 476, 20190498. doi:10.1098/rspa.2019.0498
Persson, M., Rydén Ahlgren, Å., Jansson, T., Eriksson, A., Persson, H. W., and Lindström, K. (2003). A New Non-invasive Ultrasonic Method for Simultaneous Measurements of Longitudinal and Radial Arterial wall Movements: First In Vivo Trial. Clin. Physiol. Funct. Imaging 23 (5), 247–251. doi:10.1046/j.1475-097x.2003.00504.x
Petzold, G. C., and Murthy, V. N. (2011). Role of Astrocytes in Neurovascular Coupling. Neuron 71, 782–797. doi:10.1016/j.neuron.2011.08.009
Potlov, A. Y., Proskurin, S. G., and Frolov, S. V. (2020). Young’s Modulus Evaluation for the Blood Vessel walls Using Intravascular Optical Coherence Tomography, 2020 International Conference on e-Health and Bioengineering (EHB), Iasi, Romania, Oct. 2020 1–4. doi:10.1109/EHB50910.2020.9280242
Rachev, A., and Hayashi, K. (1999). Theoretical Study of the Effects of Vascular Smooth Muscle Contraction on Strain and Stress Distributions in Arteries. Ann. Biomed. Eng. 27, 459–468. doi:10.1114/1.191
Ramirez, L. E. S., and Coimbra, C. F. M. (2010). On the Selection and Meaning of Variable Order Operators for Dynamic Modeling. Int. J. Differential Equations 2010, 1–16. doi:10.1155/2010/846107
Reina-De La Torre, F., Rodriguez-Baeza, A., and Sahuquillo-Barris, J. (1998). Morphological Characteristics and Distribution Pattern of the Arterial Vessels in Human Cerebral Cortex: a Scanning Electron Microscope Study. Anat. Rec. 251, 87–96. doi:10.1002/(sici)1097-0185(199805)251:1<87::aid-ar14>3.0.co;2-7
Samko, S. G., and Ross, B. (1993). Integration and Differentiation to a Variable Fractional Order. Integral Transforms Spec. Functions 1 (4), 277–300. doi:10.1080/10652469308819027
Santos, R. M., Lourenço, C. F., Ledo, A., Barbosa, R. M., and Laranjinha, J. (2012). Nitric Oxide Inactivation Mechanisms in the Brain: Role in Bioenergetics and Neurodegeneration, Int. J. Cel Biol., 2012, 1, 13. doi:10.1155/2012/391914
Santos, R. M., Lourenço, C. F., Pomerleau, F., Huettl, P., Gerhardt, G. A., Laranjinha, J., et al. (2011). Brain Nitric Oxide Inactivation Is Governed by the Vasculature. Antioxid. Redox Signaling 14 (6), 1011–1021. doi:10.1089/ars.2010.3297
Schuler, D., Sansone, R., Freudenberger, T., Rodriguez-Mateos, A., Weber, G., Momma, T. Y., et al. (2014). Measurement of Endothelium-dependent Vasodilation in Mice-Brief Report, Atvb 34, no. 12, 2651–2657. doi:10.1161/ATVBAHA.114.304699
Scott Blair, G. M., and Coppen, F. M. V. (1939). The Subjective Judgement of the Elastic and Plastic Properties of Soft Bodies; the "differential Thresholds" for Viscosities and Compression Moduli. Proc. R. Soc. Lond. B 128, 109–125. doi:10.1098/rspb.1939.0046
Sharma, R., Seo, J.-W., and Kwon, S. (2014). In VivoImaging of Nitric Oxide by Magnetic Resonance Imaging Techniques. J. Nanomater. 2014, 1–13. doi:10.1155/2014/523646
Sriram, K., Laughlin, J. G., Rangamani, P., and Tartakovsky, D. M. (2016). Shear-induced Nitric Oxide Production by Endothelial Cells. Biophysical J. 111, 208–221. doi:10.1016/j.bpj.2016.05.034
Stålhand, J., Klarbring, A., and Holzapfel, G. A. (2008). Smooth Muscle Contraction: Mechanochemical Formulation for Homogeneous Finite Strains. Prog. Biophys. Mol. Biol. 96, 465–481. doi:10.1016/j.pbiomolbio.2007.07.025
Suki, B., Barabasi, A. L., and Lutchen, K. R. (1994). Lung Tissue Viscoelasticity: a Mathematical Framework and its Molecular Basis. J. Appl. Physiol. 76 (6), 2749–2759. doi:10.1152/jappl.1994.76.6.2749
Terpolilli, N. A., Feiler, S., Dienel, A., Müller, F., Heumos, N., Friedrich, B., et al. (2016). Nitric Oxide Inhalation Reduces Brain Damage, Prevents Mortality, and Improves Neurological Outcome after Subarachnoid Hemorrhage by Resolving Early Pial Microvasospasms. J. Cereb. Blood Flow Metab. 36 (12), 2096–2107. doi:10.1177/0271678X15605848
Torvik, P. J., and Bagley, R. L. (1984). On the Appearance of the Fractional Derivative in the Behavior of Real Materials. ASME J. Appl. Mech. 51, 294–298. doi:10.1115/1.3167615
Tóth, M., Nádasy, G. L., Nyáry, I., Kerényi, T., Orosz, M., Molnárka, G., et al. (1998). Sterically Inhomogenous Viscoelastic Behavior of Human Saccular Cerebral Aneurysms. J. Vasc. Res. 35 (5), 345–355. doi:10.1159/000025604
Unitt, D. C., Hollis, V. S., Palacios-Callender, M., Frakich, N., and Moncada, S. (2010). Inactivation of Nitric Oxide by Cytochrome C Oxidase under Steady-State Oxygen Conditions. Biochim. Biophys. Acta (Bba) - Bioenerg. 1797, 371–377. doi:10.1016/j.bbabio.2009.12.002
Wei, G., Dawson, V. L., and Zweier, J. L. (1999). Role of Neuronal and Endothelial Nitric Oxide Synthase in Nitric Oxide Generation in the Brain Following Cerebral Ischemia. Biochim. Biophys. Acta (Bba) - Mol. Basis Dis. 1455, 23–34. doi:10.1016/s0925-4439(99)00051-4
West, B. J., Bologna, M., and Grigolini, P. (2003). Physics of Fractal Operators. USA: Springer-Verlag New York.
Wilkinson, I. B., Franklin, S. S., and Cockcroft, J. R. (2004). Nitric Oxide and the Regulation of Large Artery Stiffness. Hypertension 44, 112–116. doi:10.1161/01.HYP.0000138068.03893.40
Yang, J., Clark, J. W., Bryan, R. M., and Robertson, C. (2003). The Myogenic Response in Isolated Rat Cerebrovascular Arteries: Smooth Muscle Cell Model. Med. Eng. Phys. 25, 691–709. doi:10.1016/S1350-4533(03)00100-0
Keywords: variable-order fractional Maxwell viscoelastic model, cerebral nitric oxide dynamics, vascular wall deformation, variable-order fractional telegraph equation, separation of variables method
Citation: Drapaca CS (2021) A Nitric Oxide–Modulated Variable-Order Fractional Maxwell Viscoelastic Model of Cerebral Vascular Walls. Front. Mech. Eng 7:674860. doi: 10.3389/fmech.2021.674860
Received: 02 March 2021; Accepted: 10 September 2021;
Published: 18 October 2021.
Edited by:
Silvia Budday, University of Erlangen Nuremberg, GermanyReviewed by:
João Laranjinha, University of Coimbra, PortugalCopyright © 2021 Drapaca. This is an open-access article distributed under the terms of the Creative Commons Attribution License (CC BY). The use, distribution or reproduction in other forums is permitted, provided the original author(s) and the copyright owner(s) are credited and that the original publication in this journal is cited, in accordance with accepted academic practice. No use, distribution or reproduction is permitted which does not comply with these terms.
*Correspondence: Corina S. Drapaca, Y3NkMTJAcHN1LmVkdQ==
Disclaimer: All claims expressed in this article are solely those of the authors and do not necessarily represent those of their affiliated organizations, or those of the publisher, the editors and the reviewers. Any product that may be evaluated in this article or claim that may be made by its manufacturer is not guaranteed or endorsed by the publisher.
Research integrity at Frontiers
Learn more about the work of our research integrity team to safeguard the quality of each article we publish.