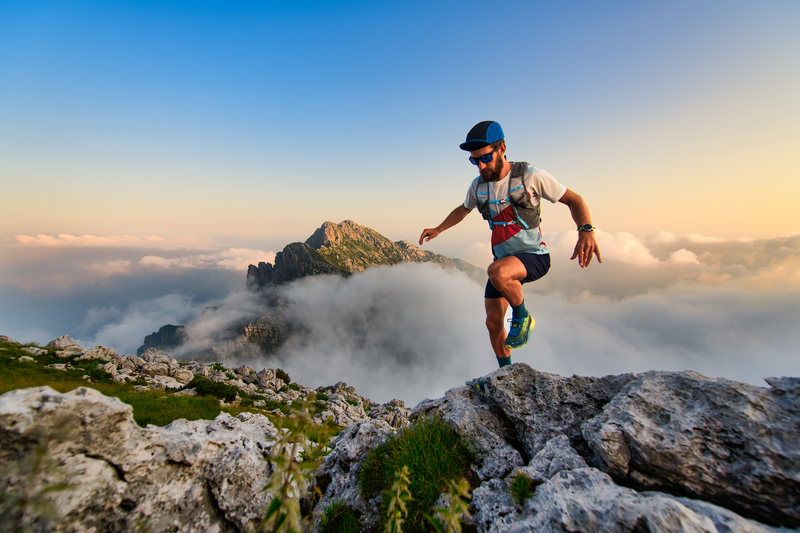
94% of researchers rate our articles as excellent or good
Learn more about the work of our research integrity team to safeguard the quality of each article we publish.
Find out more
EDITORIAL article
Front. Mater.
Sec. Smart Materials
Volume 12 - 2025 | doi: 10.3389/fmats.2025.1568640
This article is part of the Research Topic Biological-Inspired Artificial Intelligent Systems: State and Perspectives View all 5 articles
The final, formatted version of the article will be published soon.
You have multiple emails registered with Frontiers:
Please enter your email address:
If you already have an account, please login
You don't have a Frontiers account ? You can register here
Starting with the basics, the term "Artificial Intelligence" is somewhat misleading. A marked distinction between "natural" and "artificial" intelligence could be considered inappropriate, the difference lies rather in the data processing strategies and architectures implemented by the biological and by non-living systems. To date, we have seen non-living systems performing computation, but it is rare to witness them exhibiting intelligence in the way we understand it. Defining intelligence itself is indeed an even greater intellectual challenge. In 1921 a Symposium has been organized (Colvin et al., 1921) about "Intelligence and its measurements". It consists of a group of fourteen psychologists who addressed three questions posed by the editors of the Journal of Educational Psychology: 1) What is intelligence? 2) How can it best be measured by group tests? 3) What are the most crucial next steps in research? There was a very great disagreement concerning the concept of intelligence, resulting in fourteen different definitions. We suppose that we are not far from that situation of the last century.Whatever we define it as, intelligence certainly always needs a support to run. In living beings it is even more than a support, it is a body (Pfeifer et al., 2007). The way in which the body shapes our intelligence is deep and complex, and it is often not considered enough in the designing of data processing devices. It has nothing to do with the interplay between the software and the hardware on which AI programs currently run on the market. It counts on a self-organized complex system mutually interacting at different scales, composed by a redundant, adaptive and fault-tolerant architecture. The difference between the effective implementation of these two realms (artificial/computing silicon-based and biological neuronal system) marks the gap between data processing concepts and strategies, as well as the difference in their corresponding energy demands, which evolution has managed to minimize so effectively.This collection of research articles highlights a detailed overview of the strategies supporting AI algorithms on one hand (Xu et al., 2024), and on the other hand it illustrates the opportunities presented by neuromorphic hardware that can at least partially mimic the behavior of biological units, such as synapses (Maldonado et al., 2023).An insight is presented here as a common thread across different papers, focusing on the role and utilization of stochasticity in improving device performance, as well as the advantages brought by its non-linearity. The latter is another biological-inspired feature (Borghi et al., 2024), whose significance in data processing tasks is explored in a various ways (Cipollini et al., 2024).Non-linearity is involved in biological systems at the level of dendritic trees (Häusser et al., 2000), as in spatial distribution of ionic channels and synapses (Mäki-Marttunen and Mäki-Marttunen, 2022) and it does not find a correspondence in the perceptron (Rosenblatt, 1958), the reference model of neuron used in AI algorithms, where the weights associated to the inputs are input-independent. The model of Receptron (Paroli et al., 2023) proposed here, accounts for this non-linearity of the system's response to inputs (Martini et al., 2024). It is implemented in a neuromorphic device used for the specific computational task of classifying Boolean functions. Furthermore, the training processes used by AI algorithms on artificial neural networks, primarily based on back-propagation, are not comparable to the reprogrammability and adaptability of these neuromorphic devices, which closely mimic biological neural networks.Together, this Research Topic provides a general overview of current AI architectures, especially those used in image processing, along with examples of neuromorphic hardware units, both at synaptic and ensemble of neuronal networks levels. It highlights opportunities for the development of neuromorphic data processing devices and architectures that leverage on nonlinearity, stochasticity and self-adaptability of physical systems.
Keywords: neuromorphic computing, reprogrammable threshold logic gate, artificial neural network, Memristor, resistive switching device
Received: 30 Jan 2025; Accepted: 10 Feb 2025.
Copyright: © 2025 Borghi and Vassanelli. This is an open-access article distributed under the terms of the Creative Commons Attribution License (CC BY). The use, distribution or reproduction in other forums is permitted, provided the original author(s) or licensor are credited and that the original publication in this journal is cited, in accordance with accepted academic practice. No use, distribution or reproduction is permitted which does not comply with these terms.
* Correspondence:
Francesca Borghi, University of Milan, Milan, Italy
Disclaimer: All claims expressed in this article are solely those of the authors and do not necessarily represent those of their affiliated organizations, or those of the publisher, the editors and the reviewers. Any product that may be evaluated in this article or claim that may be made by its manufacturer is not guaranteed or endorsed by the publisher.
Research integrity at Frontiers
Learn more about the work of our research integrity team to safeguard the quality of each article we publish.