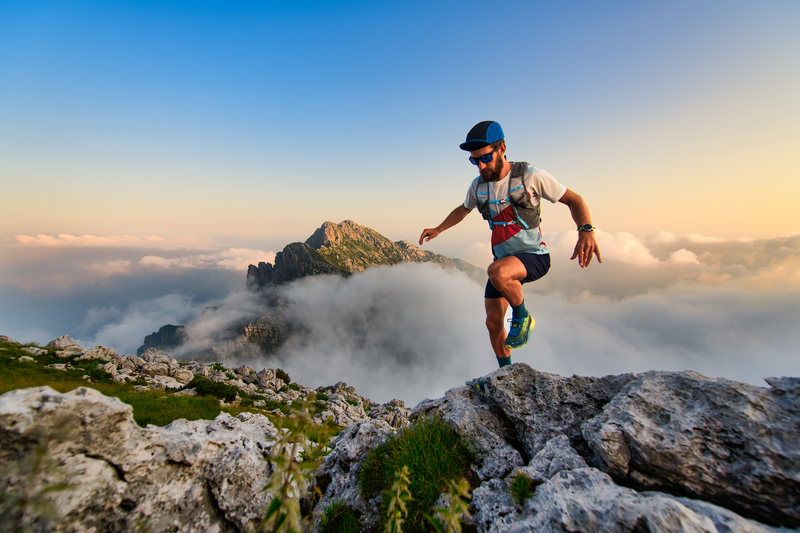
94% of researchers rate our articles as excellent or good
Learn more about the work of our research integrity team to safeguard the quality of each article we publish.
Find out more
ORIGINAL RESEARCH article
Front. Mater.
Sec. Structural Materials
Volume 12 - 2025 | doi: 10.3389/fmats.2025.1560419
This article is part of the Research Topic Joining and Welding of New and Dissimilar Materials - Volume III View all articles
The final, formatted version of the article will be published soon.
You have multiple emails registered with Frontiers:
Please enter your email address:
If you already have an account, please login
You don't have a Frontiers account ? You can register here
Joining and welding processes for dissimilar materials present unique challenges due to the need for precise monitoring and analysis of complex physical and chemical interactions. These processes are influenced by variations in material behavior, dynamic changes in process parameters, and environmental factors, making real-time action recognition a critical tool for ensuring consistent quality, efficiency, and reliability. Traditional methods for analyzing such processes often fail to effectively capture the multi-scale spatiotemporal dependencies and adapt to the inherent variability of these operations. To address these limitations, we propose a novel deep learning-based framework specifically designed for action recognition in joining and welding tasks involving dissimilar materials. Our proposed model, the Multi-Scale Spatiotemporal Attention Network (MS-STAN), leverages advanced hierarchical feature extraction techniques and attention mechanisms to capture fine-grained spatiotemporal patterns across varying scales, while simultaneously suppressing irrelevant or noisy regions within the input data. The framework integrates adaptive frame sampling and lightweight temporal modeling to ensure computational efficiency, making it practical for real-time applications without sacrificing accuracy. Domainspecific knowledge is embedded into the framework, enhancing its interpretability and improving its ability to generalize across diverse joining and welding scenarios. Experimental results highlight the model's superior performance in recognizing critical process actions, offering a promising solution for robust real-time monitoring, quality assurance, and optimization of joining and welding workflows. This work paves the way for more intelligent and adaptive manufacturing systems.
Keywords: Action recognition, Dissimilar materials, Joining and welding, Spatiotemporal modeling, deep learning
Received: 14 Jan 2025; Accepted: 19 Feb 2025.
Copyright: © 2025 Zou. This is an open-access article distributed under the terms of the Creative Commons Attribution License (CC BY). The use, distribution or reproduction in other forums is permitted, provided the original author(s) or licensor are credited and that the original publication in this journal is cited, in accordance with accepted academic practice. No use, distribution or reproduction is permitted which does not comply with these terms.
* Correspondence:
Yiming Zou, Qingdao University of Science and Technology, Qingdao, China
Disclaimer: All claims expressed in this article are solely those of the authors and do not necessarily represent those of their affiliated organizations, or those of the publisher, the editors and the reviewers. Any product that may be evaluated in this article or claim that may be made by its manufacturer is not guaranteed or endorsed by the publisher.
Research integrity at Frontiers
Learn more about the work of our research integrity team to safeguard the quality of each article we publish.