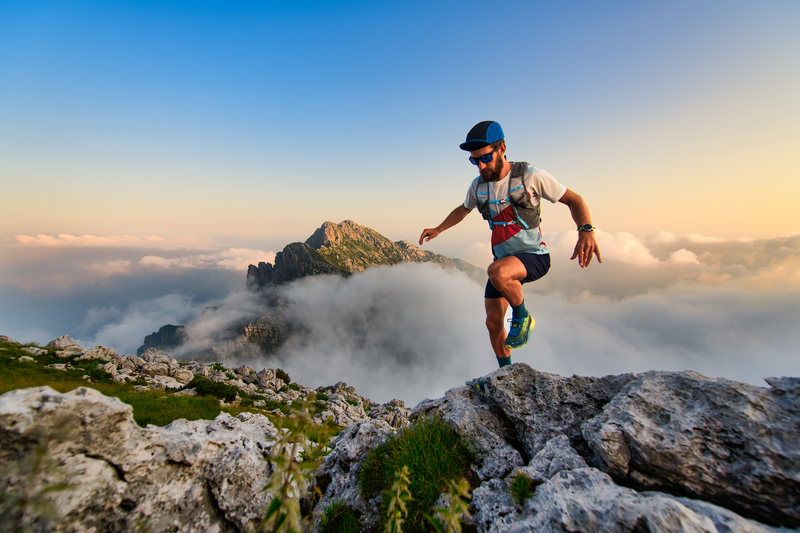
95% of researchers rate our articles as excellent or good
Learn more about the work of our research integrity team to safeguard the quality of each article we publish.
Find out more
EDITORIAL article
Front. Mater. , 22 October 2024
Sec. Mechanics of Materials
Volume 11 - 2024 | https://doi.org/10.3389/fmats.2024.1473420
This article is part of the Research Topic Modeling and Experimentation of Imperfections in Materials View all 5 articles
Editorial on the Research Topic
Modeling and experimentation of imperfections in materials
Understanding imperfections in materials is essential for advancing materials science and engineering. These imperfections influence mechanical properties, failure mechanisms, and the development of innovative materials. Our Research Topic, “Modeling and Experimentation of Imperfections in Materials,” gathers four contributions that push the boundaries of our understanding in this domain. Alongside my esteemed co-editors, Chu Lun Alex Leung, Massoud Malaki, Enyu Guo, Yang Yang, and James M. Goff, I am pleased to present these innovative studies.
In the paper “Splitting Failure and Deformation Evolution of Red Argillaceous Siltstone Disc in High Humidity Environment,” Zhao et al. investigate the impact of high humidity on the mechanical behavior of red argillaceous siltstone discs. Through Brazilian tests on disc samples with varying water content, the authors reveal a negative correlation between water content and mechanical properties such as tensile strength and elastic modulus. The study emphasizes the significant influence of moisture on failure modes, highlighting increased local crushing and altered displacement patterns. This work underscores the importance of understanding environmental effects on geological materials, particularly in mining and civil engineering applications.
The study “Rock-like Material under Large Diameter SHPB Dynamic Splitting Tension: Meso-damage Mechanical Behavior and Stress Wave Propagation Model” by Yang et al. explores the dynamic mechanical behavior and stress wave propagation in rock-like materials under splitting tension using a large diameter split Hopkinson pressure bar (SHPB). Their research identifies three distinct stages of mechanical behavior: initial elastic-plastic deformation, pre-peak plastic damage, and post-peak brittle fracture failure. The study finds that the transmission stress peak advances with increased incident stress wave, significantly influenced by strain rates. By defining a dynamic splitting damage variable based on fracture energy, the authors provide a nuanced understanding of damage evolution in rock-like materials, which is crucial for applications requiring precise modeling of dynamic stress responses.
In “Identifying a Machine-Learning Structural Descriptor Linked to the Creep Behavior of Kob-Andersen Glasses,” Wu and Ruiz Pestana integrate machine learning with materials science to predict plastic rearrangements in Kob-Andersen glasses. Using molecular dynamics simulations and feature selection techniques, the researchers train a classification model to predict particle rearrangements. They emphasize the importance of medium-range order and structural heterogeneity in predicting plastic events. The model, which achieves high accuracy, identifies significant features related to distances and volumes within the glass structure. This study highlights the potential of machine learning to enhance our understanding and prediction of material behavior at the atomic scale.
Finally, the paper “Structural and Mechanical Properties of Cu-SiCp Nanocomposites Fabricated by Accumulative Roll Bonding (ARB)” by Zhang et al. investigates the microstructural evolution and mechanical properties of Cu-SiC composites processed through accumulative roll bonding (ARB). Using X-ray diffraction (XRD) and the Rietveld method, the study analyzes texture parameters and identifies significant changes in sub-grain size, microstrain, and texture during the ARB process. The findings indicate that ARB cycles improve the composite’s mechanical properties by refining grain size and enhancing texture. This research provides critical insights into the development of high-strength, ductile materials for structural applications, showcasing the potential of ARB in fabricating advanced nanocomposites.
These papers collectively contribute to a deeper understanding of how imperfections and environmental factors influence material properties and behavior. By employing a range of experimental techniques and modeling approaches, they offer comprehensive insights into the mechanisms underlying material performance. The integration of machine learning further demonstrates the innovative approaches driving modern materials science.
We extend our gratitude to all the authors for their rigorous research and to the reviewers for their invaluable feedback. We hope this Research Topic will inspire further research and innovation in the field of material imperfections. It has been an honor to work alongside my co-editors Chu Lun Alex Leung, Massoud Malaki, Enyu Guo, Yang Yang, and James M. Goff, whose expertise and dedication have been instrumental in curating this impactful Research Topic.
WX: Writing–review and editing, Writing–original draft. CL: Writing–review and editing. MM: Writing–review and editing. EG: Writing–review and editing. YY: Writing–review and editing. JG: Writing–review and editing.
The author(s) declare financial support was received for the research, authorship, and/or publication of this article. WX is grateful for the support from NSF DMR 1900876 and DOE DE-SC0022244. CLAL is grateful for the support from the UKRI—EPSRC grants (references: EP/R511638/1, EP/W006774/1, EP/P006566/1, EP/W003333/1, EP/V061798/1, and EP/W037483/1) the IPG Photonics/Royal Academy of Engineering Senior Research Fellowship in SEARCH (ref: RCSRF2324-18-71).
The authors declare that the research was conducted in the absence of any commercial or financial relationships that could be construed as a potential conflict of interest.
All claims expressed in this article are solely those of the authors and do not necessarily represent those of their affiliated organizations, or those of the publisher, the editors and the reviewers. Any product that may be evaluated in this article, or claim that may be made by its manufacturer, is not guaranteed or endorsed by the publisher.
Keywords: imperfections, materials science, mechanical behavior, dynamic splitting, machine learning
Citation: Xu W, Leung CLA, Malaki M, Guo E, Yang Y and Goff JM (2024) Editorial: Modeling and experimentation of imperfections in materials. Front. Mater. 11:1473420. doi: 10.3389/fmats.2024.1473420
Received: 31 July 2024; Accepted: 15 October 2024;
Published: 22 October 2024.
Edited and reviewed by:
Bo Wang, Northwestern Polytechnical University, ChinaCopyright © 2024 Xu, Leung, Malaki, Guo, Yang and Goff. This is an open-access article distributed under the terms of the Creative Commons Attribution License (CC BY). The use, distribution or reproduction in other forums is permitted, provided the original author(s) and the copyright owner(s) are credited and that the original publication in this journal is cited, in accordance with accepted academic practice. No use, distribution or reproduction is permitted which does not comply with these terms.
*Correspondence: Wenwu Xu, d2Vud3UueHVAc2RzdS5lZHU=
Disclaimer: All claims expressed in this article are solely those of the authors and do not necessarily represent those of their affiliated organizations, or those of the publisher, the editors and the reviewers. Any product that may be evaluated in this article or claim that may be made by its manufacturer is not guaranteed or endorsed by the publisher.
Research integrity at Frontiers
Learn more about the work of our research integrity team to safeguard the quality of each article we publish.