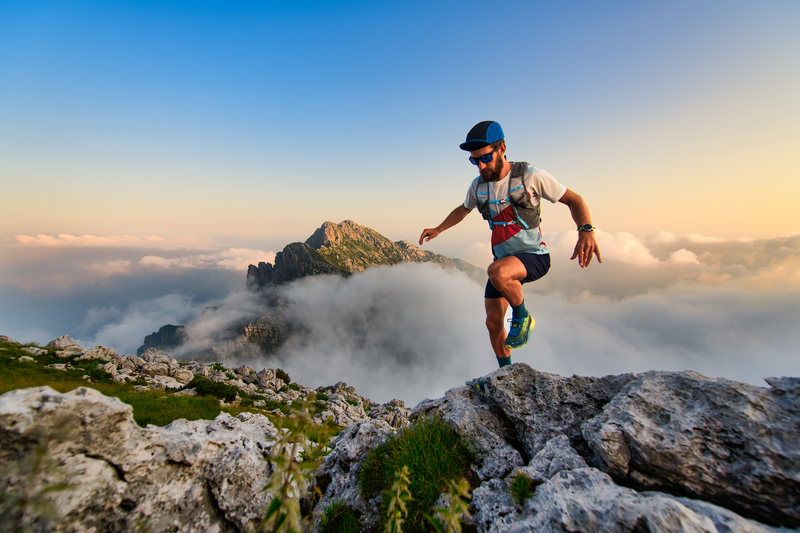
94% of researchers rate our articles as excellent or good
Learn more about the work of our research integrity team to safeguard the quality of each article we publish.
Find out more
ORIGINAL RESEARCH article
Front. Mater. , 03 October 2023
Sec. Metamaterials
Volume 10 - 2023 | https://doi.org/10.3389/fmats.2023.1289250
This article is part of the Research Topic Transformation Optics and its Frontier Branches View all 11 articles
Electromagnetic cloak or illusion, which can interfere with device detection and provide superior self-protection capabilities for animals or humans, has received much attention. The proposal of transformation optics provides a generalized strategy for realizing electromagnetic illusion. However, the complex parameter composition causes a substantial computational cost, which is not conducive to practical applications. To overcome these challenges, we report an intelligent illusory metasurface optimized by a genetic algorithm, which not only presents predefined illusory effects but also reduces the parameter space in physics. By designing a high-performance tunable metasurface, a high-fidelity inverse design is performed in simulation. Near-field and far field results show that the metasurface can generate virtual targets in different scenarios and realize electromagnetic illusion. This work is helpful in facilitating the practical application of electromagnetic illusion strategies.
Electromagnetic (EM) illusion technology is one of the most promising applications (Jiang et al., 2010; Jiang and Cui, 2011; Schittny et al., 2014; Xu et al., 2015; Yang et al., 2016; Huang et al., 2018; Cai et al., 2022; Liang et al., 2023), which can visually produce virtual objects that do not exist or transform an object into other forms, interfering with EM detection and ultimately achieving camouflage for active attack or passive defense. In traditional methods, optical illusions can be realized with the theory of transformation optics (Pendry et al., 2006; Schurig et al., 2006; Lai et al., 2009a; Lai et al., 2009b; Luo et al., 2009; Landy and Smith, 2013; Zheng et al., 2019), such as using complementary media to eliminate the original object and release the illusion of another object. However, this design is very complicated, and the extreme materials with anisotropy and inhomogeneity also make its practical application face a bottleneck. At the same time, a critical prerequisite for the realization of EM illusions is the acquisition of high-performance metamaterials/metasurfaces with the desired EM response.
Traditionally, this process of designing metasurfaces needs to be accomplished by time-consuming, inefficient, empirically guided numerical simulations or physically based methods; and theoretical methods will fail when the shape of the object is irregular (Chen et al., 2007; Li and Pendry, 2008; Hao et al., 2021; Shan et al., 2021; Hao et al., 2022). In recent years, intelligent optimization algorithms have emerged, strongly contributing to the study of metasurfaces (Goodfellow et al., 2016; Vaswani et al., 2017; Li et al., 2019; Qian et al., 2020; Shlezinger et al., 2021; Keeley et al., 2022; Ma et al., 2022; Shao et al., 2022; Lu et al., 2023; Zhong et al., 2023). Such as intelligent scattering and cloak (Qian et al., 2020), adaptive focusing (Lu et al., 2023), etc., which provide guidance for the inverse design of metasurfaces, and also provide the basis for realizing the EM illusion of false targets.
This study proposes an illusionary metasurface optimized using genetic algorithm (GA) (Lecun et al., 1998; Shakya, 2002; Pierro et al., 2004; Hinton et al., 2012; Lingaraj, 2016; Krizhevsky et al., 2017; Katoch et al., 2021) that can produce false targets in different scenarios. Firstly, the metasurface with high reflectivity and wide phase coverage is designed, and an adaptive regulation model of GA and metasurface is constructed. Different objective functions are selected according to the requirements in different environments, and the tunable metasurface satisfying the predefined objectives is reverse-designed after global optimization and iteration of the algorithm. Ultimately, illusory scattering that produces false vehicle targets is achieved using the metasurface in both the absence of objects and the presence of a single object. Despite the operating frequency being 5 GHz, the optimization method has no restriction on the frequency, and false scattering can be achieved at other arbitrary frequencies by simply modifying the appropriate objective function. This work eliminates the need for theoretical computation of complex parameters and allows iterative generation of spurious scattering given only the optimization objective. It has a wide range of promising applications in various aspects, such as metasurface inverse design and intelligent tuning.
Figure 1 illustrates the conceptual diagram of an EM illusion using the tunable metasurface to generate the virtual target. The intelligent metasurface consists of a series of tunable unit cells (as shown in the inset at the top right), and it is independently tunable in one-dimensional direction (y-direction) for each cell to produce a rich EM response. When an object exists on the metasurface (actual target), initial radar cross section (RCS) is generated under the effect of EM waves. The observer can recognize the target by judging the approximate shape of the object based on the RCS. If other types of far-field RCS can be generated, the detection device will be interfered with to realize EM illusion. As shown in Figure 1, using the target RCS as the objective function, after the global optimization of the GA and the modulation of the metasurface, the system finally produces the RCS that is generated only when two targets exist, i.e., the virtual target is generated.
A high-performance tunable reflection unit cell is designed to generate the false targets. Figure 2A shows the designed structure, consisting of a dielectric substrate and a metal with a relative permittivity
FIGURE 2. The unit cell and S-parameters. (A) Schematic diagram of the unit cell, where
The S-parameter characteristics are shown in Figures 2B, C. The horizontal coordinate represents the frequency change. It can be seen that the reflection phase varies continuously up to 330° at
The virtual target is then generated using GA and the metasurface, the progress is illustrated in Figure 3. The metasurface is composed of a set of tunable unit cells, the state is independently along the y-direction, and it is periodically arranged along the x-direction. The size of the metasurface is
where
FIGURE 3. The process for generating false targets in different scenarios using GA and metasurface. (A) Input data for the first scenario. (B) Genetic algorithm process. (C) The first illusion scene. (D) Input data or the second scenario. (E) The second illusion scene.
In the first case, we take the electric field generated by the metasurface as the input (Figure 3A) and take the single-vehicle scattering data as the target (Goal-1 in Figure 3B). After global optimization by the GA, we can eventually generate the scattering field similar to one vehicle target, thus confusing the observer, as shown in Figure 3C.
In the second case, the electric field generated by the metasurface and the vehicle together is used as the input (Figure 3D). Taking it as the goal data (Goal-2 of Figure 3B), the system can generate the electric field that similar to two vehicle targets after global optimization, thus creating a false target (as shown in Figure 3E). After being driven and guided by the GA, the various virtual target can be generated in different scenarios, interfering with the detection and realizing the EM illusion.
The results of generating one false vehicle target are shown in Figure 4. Figure 4A shows the results of the real part of the electric field of the vehicle model on a metal ground, where it can be seen that there is strong backward scattering. The vehicle model has a height of about 60 mm and a length of about 70 mm. The electric field is used as the target data. Using GA to optimize and adjust the metasurface, and the final iteration electric field is shown in Figure 4B. In Figure 4, the horizontal coordinate x denotes the length of the metasurface, and the vertical coordinate y denotes the distance from the metasurface, and all data are normalized. A comparison of Figs a and b shows that there is a high similarity, which means the metasurface produces a false target that approximates the vehicle model. The MAE loss is 0.15.
FIGURE 4. Metasurface generates the false vehicle target. (A) Scattering of the object. (B) Scattering of the metasurface. (C) The results of the RCS.
Figure 4C shows the comparison results of the far-field RCS, where the red solid line is the RCS produced by the target (Figure 4A) and the blue solid line is the RCS produced by the metasurface (Figure 4B). The RCS was defined as:
where
where
Figure 5 shows the results of the metasurface generating a virtual target in another scenario. In Figure 5A, a vehicle target made of PEC is placed above a metallic ground and generates scattering, labeling the object as target one. In Figure 5, the horizontal coordinate x denotes the length of the metasurface and the vertical coordinate y denotes the distance from the metasurface. Two identical vehicles (target one and target two) were placed above the PEC, and their near-field scattering is shown in Figure 5B. The effect of the metal object results in inhomogeneity across the real part of the electric field. Using the data in Figure 5B as the goal function, and the final iteration electric field is shown in Figure 5C. The comparative results of Figures 5B, C reveal the consistency of the electric field, at which point the MAE loss is 0.18.
FIGURE 5. Metasurface generates the false vehicle target in another scenario. (A) Scattering of the target one. (B) Scattering of targets one and two. (C) Scattering from metasurface and target one. (D,E) Comparison of RCS in different scenarios.
Figures 5D, E show the far-field RCS results. In Figure 5D, the blue solid line is the RCS generated by target one (i.e., Figure 5A). The red solid line is the RCS generated by target one and target two together (i.e., Figure 5B), and the correlation of the curves is only 20%. After global optimization, the far-field RCS generated by the metasurface and target one has a high similarity with the two targets, as shown in Figure 5E, and the correlation coefficient is 97.3%.
In conclusion, we implement a joint GA and metasurface to realize an EM phantom for false target generation, and this joint modulation model is validated to be effective in different scenarios. High-performance and reliable tunable reflective hypersurface structures are designed, which can achieve a continuous phase change of about 330° at the operating frequency while the amplitude is better than −2 db. The metasurface arrays designed with the assistance of genetic algorithms can generate scattering from vehicle targets in both scenarios with no objects or in the presence of a single object, and both near-field and far-field data validate the effectiveness of the simulations. Compared to traditional deep learning algorithms, GA can achieve global optimization with unlabeled data and explore more parsing solutions to the problem. In scenarios with more targets, only the optimization objective function is modified to achieve false scattering for different scenarios. This work provides new ideas for EM metasurface inverse design and can be used to decode richer structures.
Data can be obtained from the corresponding author (HL).
RZ: Investigation, Validation, Writing–original draft. TC: Writing–review and editing, Supervision. KW: Writing–review and editing, Supervision. HW: Investigation, Methodology, Supervision, Writing–review and editing. HL: Conceptualization, Methodology, Writing–review and editing.
The authors declare financial support was received for the research, authorship, and/or publication of this article. This work was sponsored by the Natural Science Foundation of Zhejiang Province under Grant No. LQ21F050002.
The authors declare that the research was conducted in the absence of any commercial or financial relationships that could be construed as a potential conflict of interest.
The reviewer CT declared a shared affiliation with the author HL to the handling editor at time of review.
All claims expressed in this article are solely those of the authors and do not necessarily represent those of their affiliated organizations, or those of the publisher, the editors and the reviewers. Any product that may be evaluated in this article, or claim that may be made by its manufacturer, is not guaranteed or endorsed by the publisher.
Cai, T., Zheng, B., Lou, J., Shen, L., Yang, Y., Tang, S., et al. (2022). Experimental realization of a superdispersion-enabled ultrabroadband terahertz cloak. Adv. Mat. 34 (38), 2205053. doi:10.1002/adma.202205053
Chen, H., Wu, B.-I., Zhang, B., and Kong, J. A. (2007). Electromagnetic wave interactions with a metamaterial cloak. Phys. Rev. Lett. 99 (6), 063903. doi:10.1103/physrevlett.99.063903
Hao, H., Ran, X., Tang, Y., Zheng, S., and Ruan, W. (2021). A single-layer focusing metasurface bassed on induced magnetism. Prog. Electromagn. Res. 172, 77–88. doi:10.2528/pier21111601
Hao, H., Tang, Y., Zheng, S., Ran, X., and Ruan, W. (2022). Design of circular polarization multiplexing beam splitter based on transmission metasurface. Prog. Electromagn. Res. M. 109, 125–136. doi:10.2528/pierm22010408
Hinton, G., Deng, L., Yu, D., Dahl, G. E., Mohamed, A., Jaitly, N., et al. (2012). Deep neural networks for acoustic modeling in speech recognition:the shared views of four research groups. IEEE Signal Proc. Mag. 29 (6), 82–97. doi:10.1109/msp.2012.2205597
Huang, C., Yang, J., Wu, X., Song, J., Pu, M., Wang, C., et al. (2018). Reconfigurable metasurface cloak for dynamical electromagnetic illusions. ACS Photonics 5 (5), 1718–1725. doi:10.1021/acsphotonics.7b01114
Jiang, W. X., and Cui, T. J. (2011). Radar illusion via metamaterials. Phys. Rev. E 83 (2), 026601. doi:10.1103/physreve.83.026601
Jiang, W. X., Ma, H. F., Cheng, Q., and Cui, T. J. (2010). Illusion media:generating virtual objects using realizable metamaterials. Appl. Phys. Lett. 96 (12), 121910. doi:10.1063/1.3371716
Katoch, S., Chauhan, S. S., and Kumar, V. (2021). A review on genetic algorithm:past, present, and future. Multimed. Tools Appl. 80 (5), 8091–8126. doi:10.1007/s11042-020-10139-6
Keeley, E., Joe, L., Colin, G., and Ian, J. (2022). Machine-learning-enabled recovery of prior information from experimental breast microwave imaging data. Prog. Electromagn. Res. 175, 1–11. doi:10.2528/pier22051601
Krizhevsky, A., Sutskever, I., and Hinton, G. E. (2017). ImageNet classification with deep convolutional neural networks. Commun. ACM 60 (6), 84–90. doi:10.1145/3065386
Lai, Y., Chen, H., Zhang, Z.-Q., and Chan, C. T. (2009b). Complementary media invisibility cloak that cloaks objects at a distance outside the cloaking shell. Phys. Rev. Lett. 102 (9), 093901. doi:10.1103/physrevlett.102.093901
Lai, Y., Ng, J., Chen, H., Han, D., Xiao, J., Zhang, Z.-Q., et al. (2009a). Illusion optics:the optical transformation of an object into another object. Phys. Rev. Lett. 102 (25), 253902. doi:10.1103/physrevlett.102.253902
Landy, N., and Smith, D. R. (2013). A full-parameter unidirectional metamaterial cloak for microwaves. Nat. Mat. 12 (1), 25–28. doi:10.1038/nmat3476
Lecun, Y., Bottou, L., Bengio, Y., and Haffner, P. (1998). Gradient-based learning applied to document recognition. Proc. IEEE 86 (11), 2278–2324. doi:10.1109/5.726791
Li, J., and Pendry, J. B. (2008). Hiding under the carpet: A new strategy for cloaking. Phys. Rev. Lett. 101 (20), 203901. doi:10.1103/physrevlett.101.203901
Li, L., Shuang, Y., Ma, Q., Li, H., Zhao, H., Wei, M., et al. (2019). Intelligent metasurface imager and recognizer. Light. Sci. Appl. 8 (1), 97. doi:10.1038/s41377-019-0209-z
Liang, Q., Li, Z., Xu, J., Duan, Y., Yang, Z., and Li, D. (2023). A 4D-printed electromagnetic cloaking and illusion function convertible metasurface. Adv. Mat. Technol. 2202020. doi:10.1002/admt.202202020
Lingaraj, H. (2016). A study on genetic algorithm and its applications. Int. J. Comput. Sci. Eng. 4, 139–143.
Lu, H., Zhao, J., Zheng, B., Qian, C., Cai, T., Li, E., et al. (2023). Eye accommodation-inspired neuro-metasurface focusing. Nat. Commun. 14 (1), 3301. doi:10.1038/s41467-023-39070-8
Luo, Y., Zhang, J., Chen, H., Ran, L., Wu, B.-I., and Kong, J. A. (2009). A rigorous analysis of plane-transformed invisibility cloaks. IEEE Trans. Antenn. Propag. 57 (12), 3926–3933. doi:10.1109/tap.2009.2027824
Ma, W., Xu, Y., Xiong, B., Deng, L., Peng, R. W., Wang, M., et al. (2022). Pushing the limits of functionality-multiplexing capability in metasurface design based on statistical machine learning. Adv. Mater 34 (16), 2110022. doi:10.1002/adma.202110022
Pendry, J. B., Schurig, D., and Smith, D. R. (2006). Controlling electromagnetic fields. Science 312 (5781), 1780–1782. doi:10.1126/science.1125907
Pierro, D.-F., Khu, S.-T., and Djordjevi, S. (2004). A new genetic algorithm to solve effectively highly multi- objective problems: POGA, report nr. Center for WaterSystems, University of Exeter., London, UK ,
Qian, C., Zheng, B., Shen, Y., Jing, L., Li, E., Shen, L., et al. (2020). Deep-learning-enabled self-adaptive microwave cloak without human intervention. Nat. Photonics 14 (6), 383–390. doi:10.1038/s41566-020-0604-2
Schittny, R., Kadic, M., Bückmann, T., and Wegener, M. (2014). Invisibility cloaking in a diffusive light scattering medium. Science 345 (6195), 427–429. doi:10.1126/science.1254524
Schurig, D., Mock, J. J., Justice, B. J., Cummer, S. A., Pendry, J. B., Starr, A. F., et al. (2006). Metamaterial electromagnetic cloak at microwave frequencies. Science 314 (5801), 977–980. doi:10.1126/science.1133628
Shakya, S. K. (October 2002). “Probabilistic model building genetic algorithm (pmbga): A survey,” in Proceedings of the 7th international conference, granada, Spain.
Shan, T., Li, M., Xu, S., and Yang, F. (2021). Phase synthesis of beam-scanning reflectarray antenna based on deep learning technique. Prog. Electromagn. Res. 172, 41–49. doi:10.2528/pier21091307
Shao, S., Fan, M., Yu, C., Li, Y., Xu, X., Wang, H., et al. (2022). Machine learning-assisted sensing techniques for integrated communications and sensing in WLANs:current status and future directions. Prog. Electromagn. Res. 175, 45–79. doi:10.2528/pier22042903
Shlezinger, N., Alexandropoulos, G. C., Imani, M. F., Eldar, Y. C., and Smith, D. R. (2021). Dynamic metasurface antennas for 6G extreme massive MIMO communications. IEEE Wirel. Commun. 28 (2), 106–113. doi:10.1109/mwc.001.2000267
Vaswani, A., Shazeer, N., Parmar, N., Uszkoreit, J., Jones, L., Gomez, A. N., et al. (January 2017). “Attention is all you need,” in Proceedings of the neural information processing systems (Long Beach, CA, USA: ).
Xu, S., Xu, H., Gao, H., Jiang, Y., Yu, F., Joannopoulos, J. D., et al. (2015). Broadband surface-wave transformation cloak. Proc. Nat. Acad. Sci. 112 (25), 7635–7638. doi:10.1073/pnas.1508777112
Yang, Y., Jing, L., Zheng, B., Hao, R., Yin, W., Li, E., et al. (2016). Full-polarization 3D metasurface cloak with preserved amplitude and phase. Adv. Mat. 28 (32), 6866–6871. doi:10.1002/adma.201600625
Zheng, B., Yang, Y., Shao, Z., Yan, Q., Shen, N.-H., Shen, L., et al. (2019). Experimental realization of an extreme-parameter omnidirectional cloak. Research 2019 (8282641), 8282641. doi:10.34133/2019/8282641
Keywords: tunable metasurface, electromagnetic illusion, genetic algorithm, global optimization, inverse design
Citation: Zhu R, Chen T, Wang K, Wu H and Lu H (2023) Metasurface-enabled electromagnetic illusion with generic algorithm. Front. Mater. 10:1289250. doi: 10.3389/fmats.2023.1289250
Received: 05 September 2023; Accepted: 22 September 2023;
Published: 03 October 2023.
Edited by:
Shuomin Zhong, Ningbo University, ChinaReviewed by:
Yunbo Li, Southeast University, ChinaCopyright © 2023 Zhu, Chen, Wang, Wu and Lu. This is an open-access article distributed under the terms of the Creative Commons Attribution License (CC BY). The use, distribution or reproduction in other forums is permitted, provided the original author(s) and the copyright owner(s) are credited and that the original publication in this journal is cited, in accordance with accepted academic practice. No use, distribution or reproduction is permitted which does not comply with these terms.
*Correspondence: Hao Wu, MTIxMzAwMDFAemp1LmVkdS5jbg==; Huan Lu, bHVodWFuMTIzQHpqdS5lZHUuY24=
Disclaimer: All claims expressed in this article are solely those of the authors and do not necessarily represent those of their affiliated organizations, or those of the publisher, the editors and the reviewers. Any product that may be evaluated in this article or claim that may be made by its manufacturer is not guaranteed or endorsed by the publisher.
Research integrity at Frontiers
Learn more about the work of our research integrity team to safeguard the quality of each article we publish.