- 1Department of Biomedical Engineering, University of Alabama at Birmingham, Birmingham, AL, United States
- 2Department of Biomedical Sciences, University of Alabama at Birmingham, Birmingham, AL, United States
- 3O’Neal Comprehensive Cancer Center, Heersink School of Medicine, University of Alabama at Birmingham, Birmingham, AL, United States
Ovarian cancer is the 5th most common and the deadliest gynecological cancer, with a 5-year survival rate of less than 50 percent. Most deaths due to ovarian cancer are caused by recurrent disease, which typically corresponds to an increase in chemoresistance of the tumor cells. However, little is known about how ovarian tumor chemoresponse changes and if such changes are regulated by the tumor microenvironment (TME). Moreover, the ovarian TME, including the tissue compositions and biomechanical features, is not well-characterized primarily due to a lack of optimal models. To more effectively characterize the TME of ovarian cancer, which may help develop innovative treatment strategies, appropriate models are desperately needed. The most utilized models include mouse models with both patient-derived xenografts and mouse or human tumor cell line derivatives, and more recently microphysiological systems (MPS). While mouse models provide high levels of physiological complexity, there is virtually no control over the TME components after tumor initiation or implantation. On the other hand, MPS or organoid models permit high levels of control of initial composition but lack many features of in vivo models. Selection of appropriate components to create a TME model is paramount for generating a physiologically relevant in vitro and ex vivo systems. The importance of biomaterial or matrix selection in ovarian TME models lies in the role of these components to activate oncogenic signaling pathways either through receptor-ligand interactions or mechanotransduction. Recent studies suggest that off-target or post-target effects of chemotherapies may interfere with mechanotransductive pathways. In ovarian cancer, changes in fibrous proteins, adhesive glycoproteins, and glycosaminoglycans can remodel the mechanical environment, further altering mechanotransductive pathways. Therefore, the next-generation of ovarian tumor models should incorporate relevant biomaterials including hyaluronic acid (HA), collagens, fibrinogen, and fibronectin to investigate the link between matrix properties and mechanobiology with metastasis and chemoresistance.
1 Introduction
With a 5-year survival rate of less than 50%, ovarian cancer is the deadliest gynecological cancer and affects 20,000 individuals per year (Penny, 2020). Many cases of ovarian cancer are diagnosed at later stages, when the disease has metastasized to either the peritoneum,omentum, or distant organs (Lengyel, 2010). Moreover, many patients suffer from recurrent disease that is resistant to chemotherapies (Lengyel, 2010). A significant limitation to improving anti-cancer therapies for these patients is lack of preclinical models that accurately mimic the complicated stromal and mechanical features present in and around the growing ovarian tumor. While mouse models have offered insights to ovarian cancer signaling and development of new therapies, there is limited ability to investigate the specific role of material and biomechanical properties that drive ovarian cancer growth, metastasis, and response to therapies (Martinez et al., 2021). Recent work has suggested that biomechanical stimulation enhances ovarian cancer growth (Martinez et al., 2021). Additionally, there is evidence to suggest that off- or post-target effects of common chemotherapies used in ovarian cancer treatment may be linked to mechanotransduction pathways (Novak et al., 2020; Martinez et al., 2021). The changes to the tumor microenvironment (TME) during ovarian cancer growth include deposition of ECM proteins that further promote proliferation or metastatic events as well as dramatic changes in biomechanical forces including tension, shear, and compression (Figure 1) (Henke et al., 1996; Shen et al., 2012; Yousif, 2014). Deeper understanding of how the TME composition and mechanical properties change during ovarian cancer progression is needed to develop effective novel anti-cancer therapies. Despite this, TME and its effects on cancer progression are not well characterized. To more effectively characterize the TME of ovarian cancer, and develop the next-generation of treatments, an appropriate model should be chosen. In addition to traditional in vitro models, this review will discuss two main types of ovarian cancer models, mouse models and 3D tumor models (Ibrahim et al., 2022; Tsang et al., 2022). As models have advanced, the ability to control the matrix material has dramatically improved and yielded novel insights about tumor growth. This review will focus on discussing several common matrix proteins shown to regulate ovarian cancer growth, mechanical forces in the TME that drive remodeling, and the signaling pathways involved, and changes to tumor cell chemoresponse (Figure 2). Additionally, we will compare in vitro, and in vivo models currently used to investigate the biomechanical TME as it relates to ovarian tumor progression.
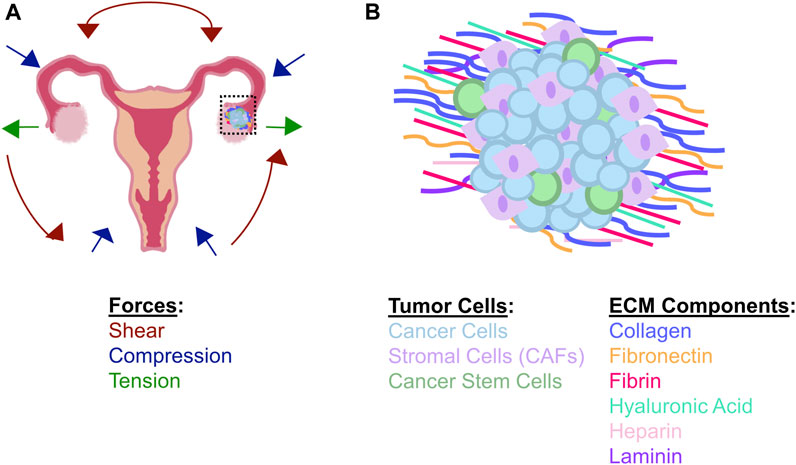
FIGURE 1. Matrix and cellular components found in the ovarian TME (A) Schematic showing changes in biomechanical forces present in the ovarian TME during disease progression. Shear forces are increased due to accumulation of ascites fluid; these forces are responsible for shearing ovarian cancer cells off of primary tumors (inset) and disseminating circulating tumor cells throughout the peritoneal cavity, leading to metastasis. Compressive forces are increased in the ovarian TME as the tumor cells continue to proliferate and additional ECM proteins are synthesized and deposited near the growing mass. Tension is increased around the tumor periphery due to the increased ECM inside the tumor as well as the increase in tumor size. Tensile forces may also be imparted by stromal cells in the TME, including CAFs, which demonstrate enhanced contractility to generate micro-strains in the matrix (B) The local TME is composed of both multiple cell types and numerous ECM materials during ovarian tumor progression. In addition to tumor cells and CAFS, some tumor cells will take on stem-like properties; these cells have been implicated as regulators of metastasis, dormancy, and chemoresistance. Various ECM proteins become dysregulated during tumor progression, including the stiffening of the tumor matrix through deposition and organization of fibrous components.
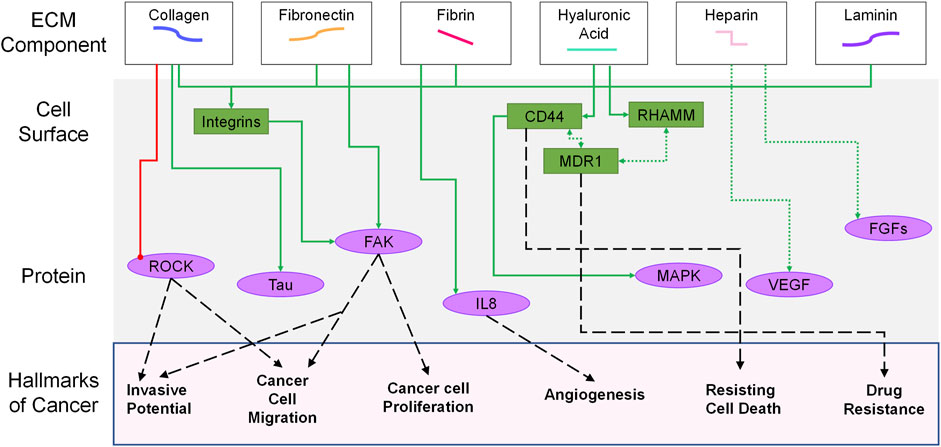
FIGURE 2. Overview of signaling pathways involved in ovarian tumor progression based on different ECM components. At the top of the image, the matrix proteins are shown tied to the cell surface receptors (green) that they interact with. Intracellular signaling pathways (purple) connect the surface proteins to phenotypic changes associated with classic hallmarks of cancer (bottom of figure) (Hanahan and Weinberg, 2011). For clarity, interactions between pathways are not highlighted (e.g.,—MAPK, ROCK, FAK). Solid green arrows indicate increases in signaling; dashed green arrows represent co-receptor associations. The red line is an inhibited pathway. Black dashed lines are used to tie hallmarks of cancer to specific cellular features associated with the behaviors.
2 Matrix components in the ovarian TME
To develop innovative in vitro models of the ovarian TME, a crucial consideration is the selection of appropriate matrix components that provide both physiological relevance and ability to easily interrogate cellular behaviors. The composition of the ovarian TME, in terms of matrix proteins and mechanical properties are significant regulators of disease progression (Mendoza-Martinez et al., 2021). Previous work has been done to highlight how individual components of the TME can activate oncogenic signaling pathways either through receptor ligand interactions or mechanotransduction (Horowitz et al., 2020). This review will highlight how the matrix proteins of the ovarian TME are directly responsible for promoting specific cellular behaviors. This will include a discussion of the prominent matrix components or features in the TME, inclusion of which could provide improved in vitro models to elucidate cancer cell behaviors. Materials present in the ovarian TME that should be considered when creating a models include fibrous proteins, adhesive glycoproteins, and glycosaminoglycans. Fibrous proteins, such as collagen, can contribute to an increase in TME stiffness, which is a common characteristic of cancer progression (Fogg et al., 2020). Adhesive glycoproteins are prevalent in the TME of several types of solid tumors. Glycosaminoglycans found in the ovarian TME can act as signaling molecules that are upstream of oncogenic signaling pathways (Wei et al., 2020). The changes in the types and amounts of matrix proteins present during ovarian cancer progression causes significant changes in the microenvironment stiffness and observed mechanical forces, and in turn, this dynamically-remodeling TME alters cancer cell signaling (Bregenzer et al., 2019). Furthermore, inclusion of mechanical factors that may impact cancer cell signaling, such as increased pressures or shears that mimic ascites build up would enhance the physiological relevance of the models while uncovering mechanobiological regulation of ovarian tumor growth. This review will discuss recent advancements in in vitro models that unite material and mechanical features to generate highly complex ex vivo TME systems for investigating cancer progression.
2.1 Fibrous proteins
Fibrous proteins, including collagens and laminins, are a vital part of the ovarian TME providing both strength and overall structure to the matrix during all stages of the disease. The majority of fibrous proteins in the ovarian TME are from the collagen family, which includes fibrillar, non-fibrillar, and fibrillar-associated collagens (Cho et al., 2015). The most prevalent fibrous proteins in the ovarian ECM are the fibrillar collagens and the most abundant and important of the fibrillar collagen are type I, III, IV, and XI (Banzato et al., 2008; Carter and Raggio, 2009; Grässel and Bauer, 2013; Cho et al., 2015). The structure of these collagen proteins comprised of three alpha chains organized into a triple helix. These helices are arranged in parallel strands in a normal microenvironment interacting with other ECM components. However, during tumor progression, more collagen fibrils are produced leading to localized disorganization and ultimately “push” into the surrounding tissue. The protrusion of nascently deposited collagen into healthy tissues is thought to be an important linkage that enhances invasive behaviors, migratory characteristics, and chemoresistance of tumor cells (Cho et al., 2015). Studies have shown that collagen type I, specifically, has demonstrated to increase ovarian tumor cell invasive behavior due to increasing directionality within the tumor, potentially through ROCK inhibition (Alkmin et al., 2020). This collagen subtype has also been linked to upregulation of the protein tau which can increase chemoresistance to paclitaxel within the tumor (Ahmed et al., 2005; Shen et al., 2012; Cho et al., 2015). Increased collagen type III crosslinking in the ovarian TME has been linked to more progressed disease states (Kauppila et al., 1996). In addition, studies looking at IHC data derived from patient samples suggest that ovarian ECM collagen type IV decreases at the onset of tumorigenesis but then increases as the cancer develops and begins metastasis (Capo-Chichi et al., 2002). On the other hand, increases in levels of collagen type XI serve as a biomarker for tumor onset and potential recurrence (Cheon et al., 2014; Raglow and Thomas, 2015). Each of these specific collagen subtypes play a different role in promoting ovarian tumor growth or as markers of disease progression, highlighting the inherit complexity of the TME involved with regulating cancer cell growth, metastasis, or response to therapies.
In noncancerous ovaries, over 50% of all ovarian cell types express at least one gene associated with laminin production, with most cells expressing at least three different laminin genes, making laminins a key component to consider in the development of novel in vitro or ex vivo ovarian models (Ricciardelli and Rodgers, 2006; Hao et al., 2020). As a part of the basal lamina, laminin acts as an adhesion site for normal and cancerous ovarian cells through beta-1 integrin signaling (Capo-Chichi et al., 2002; Ahmed et al., 2005; He et al., 2015; Ajeti et al., 2017). In early ovarian cancer stages, the loss of laminin may lead to changes in tumor cell proliferation; however, as the disease progresses laminin expression increases and this may promote shedding into the peritoneal space and subsequent metastatic invasion of the peritoneum (Yang et al., 2002; Bar et al., 2004). This is further demonstrated by increased laminin observed in ascitic fluid of ovarian cancer patients and in metastases but not in serum levels or primary tumor sites (Byers et al., 1995; Capo-Chichi et al., 2002). Therefore, inclusion of laminin in appropriate ovarian TME models should consider not only laminin types and levels but also the stage of disease to be modeled. Current examples of such models and divergent ovarian tumor cell behaviors observed will be described in detail in a later section of this review.
2.2 Adhesive Glycoproteins
Adhesive glycoproteins, such as fibrinogen and fibronectin, are characterized by being able to interact with both integrins and the ECM surrounding the cells. Increases in fibrin and fibronectin in the ovarian TME is associated with several hallmarks of cancer including the following: tumor promoting inflammation, inducing angiogenesis, sustaining proliferative signaling, activating invasion and metastasis (Hanahan and Weinberg, 2011). By linking integrins and the ECM, these glycoproteins further play a role in cancer cell migration behaviors. Fibronectin is secreted by normal fibroblasts and cancer associated fibroblasts (CAFs). In the ovarian TME, it is theorized that CAFs supply the majority of fibronectin (Rick et al., 2019). In healthy, non-cancerous tissues fibronectin creates a fibrous matrix that acts as a scaffold and plays a role in cell signaling for differentiation, migration, and vascular wound healing through thrombosis (Obara et al., 1988; Jarnagin et al., 1994; Ni et al., 2003; Singh et al., 2010). Fibronectin plays a role in matrix assembly by binding to other fibronectin and forming fibronectin fibrils (Singh et al., 2010). These fibrils can elongate and cause tension between cells, this tension can alter the mechanical environment in the ovarian TME (Friedland et al., 2009). A hallmark of CAFs includes the overproduction and remodeling of matrix proteins compared to normal, quiescent fibroblasts (Alcoser et al., 2015; Sewell-Loftin et al., 2017). Ultimately, the CAFs create a stiffer TME compared to noncancerous tissues (Deng et al., 2022). Integrin interactions play a vital role in promotion and inhibition of fibronectin assembly. Increases in α5β1integrin has been shown to be able increase fibronectin in mouse ovary and may play a role in TME matrix remodeling (Giancotti et al., 1990). It has been suggested that CAF-secreted fibronectin and the resulting fibronectin fiber orientation leads cancer cells into an organized and directional migration during metastasis (Erdogan et al., 2017). The increased fibronectin in the TME compared to a healthy ovarian ECM can lead to cancer metastasis through various oncolytic pathways, angiogenesis, and proliferation. These oncolytic pathways include FAK which is associated with migration and invasion (Yousif, 2014). In murine models, knocking out fibronectin production led to a decrease in the cancer cell ability to proliferate, attach, and metastasize (Kenny et al., 2014). Fibrin is another adhesive glycoprotein that promotes proliferation and migration in ovarian tumor cells (Henke et al., 1996). Fibrin also plays a role in angiogenesis through various mechanisms including IL-8 signaling, integrin binding, and through fibrin degradation products (Yang et al., 1993; Bach et al., 1998; Lalla et al., 2001; Martinez et al., 2001). It has been shown that fibrin in the TME can be used for cancer cell adhesion and plays a role in cancer metastasis (Shahid et al., 2019).
2.3 Glycosaminoglycans
Glycosaminoglycans (GAGs) are a family of negatively charged polysaccharides found in the ECM and on cell surfaces throughout the body in both normal and diseased tissues. GAGs play a role in wound healing, cellular communication, and cellular adhesion (Rabenstein, 2002; Volpi, 2006; Chalkiadaki et al., 2009). There are four main categories of GAGs: hyaluronic acid (HA), heparan sulfate, chondroitin sulfate, and keratin sulfate, all of which may be found in the ovarian TME (Whitham et al., 1999; Davies et al., 2004; Morla, 2019; Biskup et al., 2021). During ovarian tumor progression, GAGs have been shown to be involved in cellular adhesion, angiogenesis, proliferation, drug resistance, and metastasis (Sasisekharan et al., 2002; Chen et al., 2009; Vallen et al., 2014). HA is the only non-sulfonated GAG and is commonly found in the ovarian ECM. Previous studies have shown that an increase in HA in the ovarian TME is associated with a worse prognosis (Oliveira-Ferrer et al., 2022). The main role of HA is keeping the tissue hydrated (Morla, 2019). It also has a role in cell signaling as the ligand for cell surface receptor, CD44, which is a cancer stem cell marker and is associated with cancer cell proliferation and migration through MAPK cell signaling pathway. HA can be separated into 2 categories, high molecular weight (greater than 1 MDa) and low molecular weight (less than 1 MDa), with different molecular weight HAs having divergent regulatory behaviors in tumor progression and cellular behaviors (Turley et al., 2002). High molecular weight HA has been shown to have anti-inflammatory and immunosuppressive properties (Prevo et al., 2001) while on the other hand low molecular weight HA has been linked to an inflammatory response (Noble et al., 1996; Rowley et al., 2020). The higher the molecular weight of HA, the more affinity the molecule has for CD44 (Lesley et al., 2000). HA signals for cell motility through the Receptor for Hyaluronan-Mediated Motility (RHAMM). Motility is activated by interactions with actin filaments and microtubules (Zaman et al., 2005). RHAMM signaling is also upstream of ERK and Src cell signaling cascades. RHAMM can be found on tumor cell surfaces and the ERK and Src signaling cascades are commonly upregulated in ovarian cancer (Wiener et al., 2003; Jiang et al., 2019). It has been suggested that RHAMM and CD44 may co-localize for HA signaling (Carvalho et al., 2021). CD44 also colocalizes with multiple drug resistant protein 1 (MDR1) making it also associated with chemotherapy drug resistance (Chen et al., 2009). High molecular weight HA also plays a role in metabolism by diminishing oxidate stress and absorbing Reactive Oxygen Species (ROS) (Pauloin et al., 2008). Through CD44 signaling, HA can regulate reduction/oxidation states and ROS generation (Ye et al., 2012). This relates to altered metabolism that can be observed in ovarian cancer cells (Nantasupha et al., 2021). There is also evidence that high molecular weight HA is able to “coat” a cell surface with CD44 which acts as a protective layer to prevent the activation of apoptosis by “masking” cell death receptors (Bourguignon et al., 1997). In angiogenesis, low molecular weight HA is proangiogenic by promoting endothelial cell proliferation and migration (Toole, 2004). High molecular weight HA prevents endothelial cell proliferation, migration, and even inhibits sprout formation, making it antiangiogenic (Toole, 2004). Low molecular weight HA in the ovarian ECM can increase some pro-inflammatory signaling, which are important in oncogenesis (Grivennikov and Karin, 2010; Rowley et al., 2020). Additionally, work by Chopra et al. has demonstrated that HA can interact with integrin signaling on soft gels (∼300 Pa) to alter mechanotransduction pathways and promote altered behaviors in cells including enhanced cell spreading and actin cytoskeletal remodeling associated that are typically only associated with stiffer substrates (>30 kPa) in a manner independent of YAP signaling (Chopra et al., 2012; Chopra et al., 2014). These studies suggest that the increased expression of HA in the ovarian TME may promote increased invasive behaviors through cooperative signaling with integrin pathways and further highlight how ovarian ECM components drive disease progression via mechanical and signaling cues.
Heparin sulfate (HS) is another type of glycosaminoglycan that undergoes sulfination before being secreted by the cells into the ECM. HS can be found on the surface of ovarian tumor cells and has been shown to exhibit both paradoxical protumor and antitumor effects (Liu et al., 2002; Yoneda et al., 2012). One determining factor of whether HS is protumorigenic or antitumorigenic is whether it is a soluble factor or bound to the cell surface. When on the cell surface, HS allows for the cell to adhere to the capillary lining during metastasis through the bloodstream (Sasisekharan et al., 2002). This adhesion is the first step of extravasation, where the tumor cells will be able to transverse out of the capillary and into the surrounding tissues to create a metastatic site. HS interacts with several growth factors including fibroblast growth factors 1 and 2 and vascular endothelial growth factor (VEGF) and HS expression on a cell surface will alter several signaling cascades related to these growth factors to cause cancer cell proliferation (Mulloy and Linhardt, 2001; Robinson and Stringer, 2001; Mundhenke et al., 2002; Ostrovsky et al., 2002; Sasisekharan et al., 2002). On the other hand, the anticoagulation effects of HS are what make it antitumorigenic. HS present in the TME can interrupt fibrin capsule formation, permitting increased infiltration of immune cells and better penetration of anti-cancer therapies; therefore, HS can be given to ovarian cancer patients as an anti-cancer therapy (Kakkar and Williamson, 1999; Ma et al., 2020). HS has also been shown to be associated with an increased response of macrophages to tumor cells and it can disrupt cellular adhesion by blocking selectin (Koopmann et al., 1999; Varki and Varki, 2002). Inclusion of perlecan, a HS proteoglycan as a component of Matrigel, can therefore alter how ovarian cancer cells behave in TME models.
3 Mechanical properties of the ovarian TME
A challenge in designing next-generation in vitro ovarian TME systems is the incomplete understanding of the mechanical environment to be mimicked by such systems. Overall, there is a gap in our knowledge for precise mechanical parameters at different disease stages (Bregenzer et al., 2019). Recent studies have characterized various ovarian tissues as well as serous and mucinous cancerous tissues using atomic force microscopy (AFM) (Ansardamavandi et al., 2020). While all samples demonstrated heterogeneous stiffness distributions, the data indicate that mature ovarian tumors with dysregulated ECM organization had significantly higher stiffnesses (∼14 kPa) compared to serous tumors (∼5 ka); moreover, the studies by Ansardamavandi et al. suggest that cellular regions, regardless of tumor subtype, are significantly less stiff compared to ECM or stromal regions. Therefore, two types of stiffness values matter in ovarian tumor models: the matrix or substrate and the cellular contents. Increased matrix stiffness correlates with increases in proliferation and metastatic behaviors in SKOV-3 cells but is also associated with decreased response to paclitaxel and cisplatin, potentially through multidrug resistance regulated by ATP binding cassette subfamily B members 1 and 4 (ABCB1 and ABCB4) (McKenzie et al., 2018; Fan et al., 2021). These studies included polyacrylamide (PA) gels ranging from 0.5 to 25 kPa to mimic lymph tissues, healthy peritoneum, and tumors. On the other hand, lower stiffness values are associated with increased stem-like properties or metastatic potential of ovarian cancer cells and organoids in both human (HEY8) and mouse (MOSE) models (Xu et al., 2012; Babahosseini et al., 2014). The decreases in cell stiffness are permissive for cells to migrate and move through stiff ECMs where the effective mesh size is small (Wisdom et al., 2018). Additionally, recent studies have suggested that increases in shear stresses, such as those caused by the accumulation and buildup of ascites fluid during ovarian tumor progression, can alter markers related to epithelial to mesenchymal transformation (EMT) and cellular cytoskeletal regulation to effectively decrease cell stiffness and increase metastatic behaviors (He et al., 2014; Ip et al., 2016; Hyler et al., 2018; Conrad et al., 2022). The increased shear stress may also decrease sensitivity to standard chemotherapies such as paclitaxel and cisplatin (Ip et al., 2016). The phenomenon of shear-induced mechanical and phenotypic shifts coupled with the physical forces of the ascites fluid act to simply dislodge ovarian tumor cells as well, further enhancing metastatic potential (Avraham-Chakim et al., 2013). Together, these studies suggest a complicated series of interactions between mechanical cues from the environment and biomechanical changes to cells that drive ovarian tumor progression and chemoresponsive. For a more thorough discussion of changes in mechanical features of the TME during disease progression and specifically on the ovarian cancer microenvironment, the authors recommend the following review articles (Jain et al., 2014; Bregenzer et al., 2019).
3.1 Ovarian ECM mechanosignaling
Mechanotransduction converts external forces from the ECM or other environmental cues into a biochemical response inside cells (Pagliari and Forte, 2018). Signaling molecules that work with mechanical forces are said to be mechanotransducers and these interactions are said to be mechanosensitive. The role of biomechanical forces and thus mechanotransduction on the progression of ovarian cancers is poorly understood, due to the complex in vivo mechanical environment that is difficult to study. As the composition and organization of the ECM changes during tumor progression, increases in local tensile, compressive, and shear forces can be found in the ovarian TME. As deposition and alignment of fibrous proteins like collagen increase, the result is an increased stiffness of the local ECM which leads to altered proliferation and migration behaviors during cancer progression (Cho et al., 2015; Campbell and Campagnola, 2017). As mentioned earlier, fibronectin fibrils can create tension in the ovarian TME that can be sensed by the signaling molecules found on the cancer cell surfaces. Additionally, the increases in collagen, fibronectin, and fibrin increases some pro-cancer cell signaling mechanotransduction pathways including Src and MAPK. Furthermore, as ovarian cancer progresses, peritoneal fluid begins to accumulate in the abdomen, called ascites, and can dramatically increase the intraperitoneal pressures and generate large shear stresses in the ovarian TME (Shield et al., 2009; Hyler et al., 2018). Studies have shown that increasing shear flow can increase the expression of cancer stem cell markers, including CD44 and Oct-4, compared to a static in vitro system (Li et al., 2017). Inside ascites, aggregates of cancer cells called spheroids form and remain in suspension. Ultimately, these spheroids will give rise to metastatic growths, as the ascites fluidic movement causes them to be delivered throughout the abdominal cavity (Li et al., 2017). This is the first step in metastasis, as the spheroids will attach to the omentum via CD44 before beginning to invade and proliferate (Motohara et al., 2019). Additionally, spheroids are characterized by being chemoresistant, and shear stress may play a role in driving this behavior; studies have shown that SKOV-3 spheroids exposed to higher levels of shear demonstrate less apoptosis and cell death in response to cisplatin and paclitaxel, two commonly used first line chemotherapies against ovarian cancer (Li et al., 2017). The increase in chemoresistance could be due to multi-drug resistant (MDR) proteins being upregulated in cancer cells isolated from ascites (Novak et al., 2018). The changes in biomechanical features of the TME, driven by changes in the ECM composition and organization, as well as ascitic fluid build-up, must be taken into consideration for the development of next-generation models of ovarian cancer progression. Without such efforts, novel therapeutic efforts will fail to address the changes in cancer cell signaling directly impacted by these biomechanical properties.
The role of biomechanical forces in driving ovarian cancer progression is not fully understood. The mechanisms that transduce these forces into biochemical signaling and altered cell behaviors is driven by aberrant mechanotransduction occurring through integrins, focal adhesions, and even cell surface receptors that are mechanosensitive. The ability of ovarian cells to sense the stiffness of the surrounding environment is particularly important for follicle development in healthy tissues. As an individual ages, the ovarian ECM is continuously reorganized and becomes stiffer due to an increase in collagen and a decrease in HA (Pagliari and Forte, 2018; Amargant et al., 2020). Altered matrix composition and/or organization during ovarian tumor growth can elicit differential signaling through a variety of mechanotransduction pathways (Figure 2) that result in changes to ovarian cancer cell behaviors including migration, invasion, differentiation, drug resistance, and altered metabolism (Ahmed et al., 2005; Ricciardelli and Rodgers, 2006; Yousif, 2014; Sun et al., 2016; Zhao et al., 2019; Sewell-Loftin et al., 2020). For instance, increases in collagen in the ovarian TME are associated with increased signaling through discoidan domain receptor 2 (DDR2) to drive ovarian phenotypic shift that permits invasion through mesothelium (Grither et al., 2018). As invasion through the mesothelial layer is an important first step in metastatic processes, ovarian cancer spheroids have also been shown to require altered contractility driven through myosin to “break through” the mesothelial cells (Iwanicki et al., 2011). Increases in osteopontin in ascites fluid can signal through CD44, altering the MAPK and PI3K/Akt pathways to drive ovarian cancer metastasis (Qian et al., 2021). These represent only a few examples of how altered matrix composition may drive ovarian tumor growth through mechanotransduction pathways. The next sections in this review will discuss currently used in vivo and in vitro models of ovarian cancer and compare how TME composition and mechanical properties are investigated or controlled for in studies.
4 Mouse models of ovarian cancer progression
The gold standard for preclinical models of cancer are murine models, with recent history seeing advances in transgenic technologies that permit exploration of tumor regulation at multiple levels to elucidate genetic and epigenetic regulators (Morin and Weeraratna, 2016; Fan et al., 2022). Mouse models have the distinct advantage of being able to observe a full physiological environment with the effects that the rest of the body, including stromal cells, blood vessels, and biomechanical forces, have on the TME and cancer progression (Maniati et al., 2020). The matrix composition and mechanical forces of a TME are naturally embedded into a mouse model. While some mouse models of ovarian cancer include immunocompetent animals, which can help uncover how immune cells are involved in cancer growth or response to therapies, other studies use immunocompromised or humanized models where the immune system may not be fully functional (Grabosch et al., 2019; Iyer et al., 2021). The advantages of immunocompromised or humanized models still include a full in vivo biomechanical environment with multiple stromal components, as well as the ability to study human tumor cells. However, the lack of fully functional immune system can confound the understanding of how tumor cells respond to therapeutic intervention (Hu et al., 2002; Magnotti and Marasco, 2018). This is seen in research, where it is common for false positives to be observed in cancer pharmaceutical studies (Vandamme, 2014). Additional drawbacks of mouse models include price and ethical concerns.
4.1 Comparing in vivo ovarian cancer models
While the bulk of this review is focused on the use of materials in in vitro models of ovarian tumor progression, many studies incorporate matrix components associated with human disease when studying ovarian growth in mouse models. We will briefly summarize the types of mouse models most frequently used (Table 1). The main categories for mouse model studies of ovarian cancer are related to cell source and location of primary tumor site. For cell sources, allograft models include ID8 cells or mouse ovarian surface epithelial (MOSE) cells. Xenograft models use immunocompromised mice injected with one of many of the cell lines previously mentioned in this review (SKOV-3, HEY1, OVCAR, etc.) or may be derived from clinical samples, in which case it is referred to as a patient-derived xenograft (Magnotti and Marasco, 2018; Tudrej et al., 2019). Classification of in vivo models is further broken down by site of injection, including subcutaneous, intraperitoneal, and orthotopic injection sites (Magnotti and Marasco, 2018; Tudrej et al., 2019; Atiya et al., 2021; Karakashev and Zhang, 2021). Subcutaneous injections can generate tumors that grow underneath the skin, usually on the dorsal side of the animal. Intraperitoneal models are injected into the peritoneal cavity, which is more anatomically relevant than the subcutaneous model due to proximity to the mice ovaries; this model can somewhat recapitulate the ascites fluid buildup, depending on injection volume, and has been shown to demonstrate changes in collagen organization in the TME as well as alterations in ovarian cancer adhesion behaviors (Hu et al., 2002; Asem et al., 2020). These studies have also provided clinical relevance in showing delivery of chemotherapies via intraperitoneal injection can alter cancer cell response and reduce ascites volume (Zhao et al., 2019). Orthotopic ovarian models are injected into the ovarian bursa, making it the most anatomically relevant of the PDX models; however, this model is the most technically difficult to perform due to the surgical nature of the implantation (Grabosch et al., 2019). The orthotopic model also spontaneously generates metastatic growth that mimics human disease (Khanna and Hunter, 2005; Grabosch et al., 2019; Atiya et al., 2021). The choice of cell type and location of primary tumor can be used to tailor the type of ovarian TME model generated, based on what type of phenotypic behaviors or outcomes one is trying to observe. Furthermore, these systems can be paired with biomaterial studies to provide a higher level of control over initial TME properties. For instance, Zheng et al., investigated the role of matrix components in a subcutaneous injection model using SKOV-3 in immunodeficient mice with results showing that including collagen I with injected cells, but not collagen IV or laminin, increases tumor growth (Zheng et al., 2015). The advancement of PDX models has further driven TME investigations for ovarian cancer progression.
While the PDX model has the advantage of focusing on human tumor cells, the major drawback of these models is the lack of human ovarian stromal microenvironment, including differences in both ECM composition and biomechanical features. Also, human ovaries lack the bursa found surrounding mice ovaries making orthotopic models less anatomically relevant due to variability in the ability for cells to migrate and metastasis (Karakashev and Zhang, 2021). Additionally, all PDX models require fresh patient samples, and the tumor implantation is invasive and difficult to perform, with some patient samples failing to engraft after implantation (Atiya et al., 2021; Karakashev and Zhang, 2021). The lack of human stromal components could lead to differences in key signaling pathways that regulate tumor growth and limit the ability to predict clinical responses to new therapies currently in development (Atiya et al., 2021). One proposed solution to this is an advanced orthotopic mouse model where patient derived tumor cells as well as carcinoma-associated mesenchymal stem cells (CA-MSCs) are injected into the mouse; the addition of the CA-MSCs supports the formation of a more humanized stromal microenvironment within a relatively short timeframe (Atiya et al., 2021). Even with the CA-MSCs, the pro-cancer and anti-cancer effects of an immune system are missing.
A significant drawback of PDX models is that a majority of such models develop stromas with predominantly murine components, creating a discrepancy between cancerous and stromal features. The same study shows that PDX models conserve cellular proliferation markers between the host stroma and PDX stroma (Weroha et al., 2014). Another study comparing gene expression of ovarian tumors and matched xenografts showed that genes that differed between the two were associated with the immune system and the ECM (Liu et al., 2019). Specifically, genes associated with cell adhesion tended not to correlate between the host and the PDX model (Liu et al., 2019). This may be explained by significant differences in the TME composition of PDX models compared to human tumors (Liu et al., 2019). Recent studies have highlighted that while some correlation between transcriptomes of ovarian cancer patients and PDX models is present, there are changes to DNA mutations and stromal components as the tumor cells are passaged through the animal models (Ben-David et al., 2017; Maniati et al., 2020). Differences in the TME composition or organization between PDX models and the human microenvironment, which may lead to changes in adhesive molecular signaling, could alter overall tumor progression and prevent development of clinically relevant therapeutic strategies.
5 In vitro ovarian TME models
5.1 2D and 2.5D models of ovarian cancer
To improve control over the stromal compartments and the TME that regulate ovarian cancer growth, a wide variety of in vitro and ex vivo models have been developed with varying levels of complexity and control (Table 2). We will briefly discuss advantages and disadvantages of such models, while highlighting how specific studies and materials have elucidated some ovarian cancer behaviors. While traditional cell culture techniques and 2D monolayer studies in systems like the commercially-available FlexCell have clarified fundamental signaling in ovarian cancer, the advent of 2.5D systems has allowed for more functional studies. The most widely known system is the transwell assay, where changes in migration or invasive potential of cells can be measured as a function of matrix properties coated onto the insert. Additionally, these studies can be used to investigate how secreted factors, including extracellular vesicles, or conditioned media alters tumor cell behaviors (Chen et al., 2017; Wang et al., 2019; Zhang et al., 2019; Zhang et al., 2021). To more specifically study how matrix components alter ovarian cancer migration, the development of fiber-based gradient patterns of ECM molecules have been developed (He et al., 2015; Ajeti et al., 2017). In these studies, laminin fibers with concentration gradients were printed to act as a scaffold to investigate a variety of human ovarian cancer cell lines including SKOV-3, HEY1, and OVCA433. Results indicated that cytoskeletal organization was increased with increases in laminin content and that this generated not only higher levels of cell migration but also controlled migration directionality. To mimic the ascitic fluid environment, Bascetin et al. developed a macromolecular model where inert materials, including Ficoll or Dextran, were incubated in the media above SKOV-3 or IGROV1 cells; the inclusion of these large molecules mimicked a “crowded” ascites fluid environment and limited both adhesion and migration while decreasing ECM synthesis and deposition (Bascetin et al., 2021). Such studies demonstrate that the specific ovarian TME architecture and surrounding microenvironment are crucial regulators of disease progression.
5.2 3D models of the ovarian TME
To model the effects of mechanical strain on an in vitro ovarian TME model, various designs have been implemented. A Flexcell Compression Plus setup has been utilized to evaluate the effects of compression due to the buildup of ascites. In this model, hydrogels were used as the matrix and static compression ranging from 3.28 to 3.52 kPa was tested (Klymenko et al., 2018). This system was able to show changes in genes associated epithelial mesenchymal transition, including N-cadherin and E-cadherin (Klymenko et al., 2018). Another custom build system utilized a poly (dimethylsiloxane) (PDMS) bioreactor and a pressure chamber to deflect a hydrogel matrix. This system was able to have static and 0.05 Hz cyclic compression (Novak et al., 2020). Ovarian cancer cell proliferation increased with both the static compression and the cyclic compression, compared to the no strain controls (Novak et al., 2020). Cell death in response to multiple chemotherapy treatments also decreased with strain treatment, showing that the compression made the ovarian cancer cells more chemoresistant (Novak et al., 2020).
5.3 Microfluidic devise to model the ovarian TME
In the last decade, microfluidic devices have been explored as potential models of the ovarian TME due to their advanced capabilities for modeling different components of the microenvironment including features related to both matrix composition, cell populations, and shear flow from ascites build-up (Figure 3). An additional advantage of such MPS models is the optical transparency of materials used during construction to enable real-time imaging of changes in cell behaviors One such model system is made of PDMS on a sodium glass plate, creating a 2D model to study drug resistance in the presence of shear flow on monolayers of cells (Figure 3A). The design consists of two lines with three oval-shaped microtissue chambers in series on each line. Each line can be loaded independently with a different cell line so that two lines can be directly compared in an experiment with built-in triplicate samples (Flont et al., 2020). The specific shape and dimensions of the ovals are designed to mimic the maximum distance of cells to capillaries (∼200 μm) while providing sufficient flow to feed cells at any location in the chamber. Systems are fed via peristaltic pumps with flow rates of 10 μL/min. This design was utilized to evaluate the effects of combination therapy on malignant and non-malignant cells, A2780 and human ovarian fibroblasts, respectively. The first therapy consisted of a photosensitizer which is non-toxic to non-malignant cells, while the second therapy was the traditional chemotherapy doxorubicin. The results showed that the combination therapy was most effective at killing the cancer cells but not the non-malignant cells when a higher concentration of the photosensitizer was introduced to the cells (Flont et al., 2020).
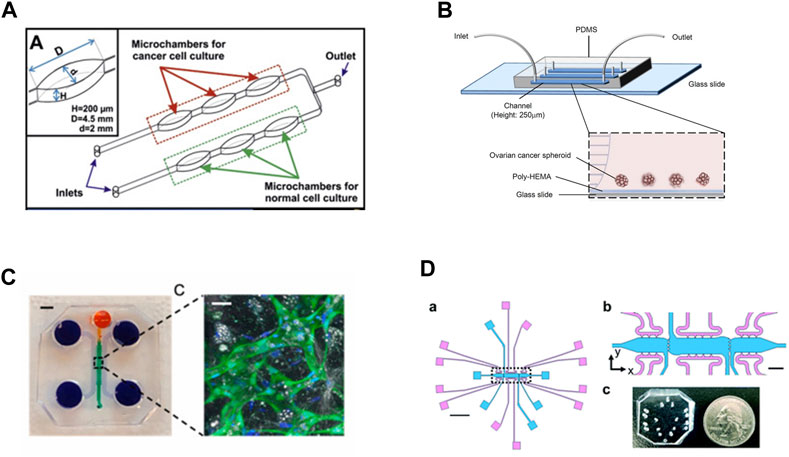
FIGURE 3. In vitro model systems of the ovarian TME (A) Microfluidic model consisting of three oval chambers in series, with two parallel flow lines. These systems were designed to test chemoresponsive of malignant and non-malignant cell lines, allowing for direct comparisons of treatments. Adapted from Flont et al., 2020, reprinted with permission (B) Microfluidic model to test chemoresponsive and stemness of ovarian tumor spheroids under shear flow. The system includes a poly-HEMA coated substrate to prevent spheroid adhesion, which better mimics circulating tumor clusters in ascites fluid. Adapted from Ip et al., 2016, reprinted under Creative Commons CC BY license (C) A 3D microfluidic system to mimic the omentum and peritoneal cavity by incorporating mesothelial cells seeded onto a self-assembled vascular network supported by additional stromal components. The system contains multiple fluidic lines for media and cell seeding surrounding the primary chamber where vessels are loaded. Scale bar for device = 3 mm; scale bar for vessel inset = 30 μm. Vessels are marked by GFP-tagged endothelial cells counterstained with DAPI. Adapted from Ibrahim et al., 2022; reprinted with permission (D) (a) Multi-microtissue model with independent tissue chambers (blue) and fluidic lines (pink). Scale bar in (a) = 2 mm. (b) Inset from (a) with clearer view of communication ports between microtissue regions. Scale bar = 200 μm. (C) PDMS device shown, not bonded to glass slide or substrate, with quarter for scale. Ports are punched using blunt-tipped needles to load pipette tips for gel/cell loading or media lines. Adapted from Sewell-Loftin, et al., 2020, reprinted under Created Commons CC BY license.
Expanding on the idea of shear flow effects in the TME, some models are focused specifically on recapitulating the ascites of the human ovarian TME (Tsang et al., 2022). This is achieved by mimicking the shear forces found in the ovarian TME and utilizing 3D tumor spheroids, where a glass slide coated with poly 2-hydroxyethylmethacrylate (poly-HEMA) is bonded to PDMS to create a fluidic channel (Figure 3B) (Ip et al., 2016; Li et al., 2017). Tumor spheroids better model the presentation of tumor cell clusters in the ascites fluid and may have better predictive value for understanding chemotherapy-induced apoptosis, making them more appropriate for a chemoresistance study (Shen et al., 2012; Ip et al., 2016). The poly-HEMA coating prevents cell attachment, thus providing a model of circulating tumor spheroids as they would occur in the ascites fluid. By controlling the flow rates, the cancer spheroids in the devices experience similar shear forces as found in the ascites. Spheroids composed of SKOV-3 cells had increased chemoresistance under 0.02 dyne/cm2 shear to both cisplatin and paclitaxel compared to non-flow controls. (Ip et al., 2016).
A distinct advantage of MPS include the ability to create a TME with multiple stromal cell types as well as a vascular network. For instance, the first ovarian TME microfluidic model that not only incorporates the human mesothelium and adipocyte stroma but also a functional vasculature network was recently developed (Ibrahim et al., 2022). The model is fabricated with PDMS poured over an acrylic mold and it is composed of four channels (Figure 3C). The first channel is for loading the fibrin gel and cellular components, while the other three channels are fluid channels for feeding. First endothelial cells and stroma can be loaded to form a blood vessel network which starts to assemble within 2 days. Then mesothelial cells are added through the top media channel to create a monolayer on top of the vasculature; cancer cells can then be added to the system seeded through the media line to represent migration or metastatic events. The advantage the mesothelial layer is that it represents a physiologically relevant structure in human ovarian cancer metastasis; before an ovarian cancer cell can metastasize, the cell must first invade through a mesothelial layer in the omentum (Motohara et al., 2019). While this model utilized fibrin as the matrix component, other matrix components were secreted by the cancer cells, including collagen VI and fibronectin. While only proof of concept studies have been completed, this model could be used for studying ovarian cancer invasion and metastasis (Ibrahim et al., 2022).
Other MPS have been developed that leverage multiple chambers and fluidic lines to provide independent control over interstitial fluid flows as well as control over the ECM composition of adjacent microtissues (Figure 3D) (Sewell-Loftin et al., 2020; Buckley et al., 2023). This model is composed PDMS bonded to glass slides with three separate microtissue chambers that can be loaded with cells and matrix independently of each other. There is a media feeding line of the top and bottom of each chamber, allowing for fine-tuned control over the direction and magnitude of fluid flow. Chambers are connected via four 20 μm ports that allow for mechanical and chemical crosstalk between the adjacent chambers, as well as permitting cell migration between chambers. The control over interstitial flow between the chambers also imparts control over convective diffusion of secreted factors between microtissue regions, thus allowing for limiting diffusion of paracrine factors from different adjacent regions. This system was used to test how tensile strain decreases sensitivity of SKOV-3 and OVCAR8 cells to paclitaxel treatment; briefly, ovarian cancer cells pre-treated with 72 h of tensile strain via a Flexcell system demonstrated decreased response to paclitaxel treatment in the 3D microtissue model. The advantage of such designs is that spatiotemporal control can be leveraged to create normal and cancerous tissue mimics with stromal components including fibroblasts, MSCs, and/or vasculature to study tumor growth, migration, and response to therapeutics. Overall, MPS offer a unique opportunity to investigate ovarian cancer progression in highly complex, biomimetic in vitro systems with much higher levels of control compared to traditional mouse models.
5.4 Comparing in vivo to ex vivo or in vitro models
The ultimate goal of studies described in this review is to elucidate the mechanisms that drive ovarian cancer disease progression and therapeutic response to develop the next-generation of anti-cancer treatments and improve patient outcomes. The in vivo and in vitro models provide their own advantages and disadvantages, which must be leveraged appropriately to ensure we uncover important regulators of ovarian tumor progression. A critical consideration then, is how to best model the complicated physiological TME in terms of biomechanical features including matrix composition and organization. Important elements for a model of an ovarian tumor microenvironment to consider are not only cellular components but also mechanical forces including tension, compression, and shear forces, and fibrous matrix components. The combination of studies using both advanced in vitro systems and validation in relevant preclinical mouse models may drive the next innovations in ovarian cancer therapeutic development.
6 Discussion
In conclusion, both matrix components and biomechanical forces in the ovarian TME play a vital role in cancer progression but the precise mechanisms are poorly understood due to limitations in our current models. To further understand the disease, appropriate TME models must be developed and utilized. An ovarian TME model should account for the fibrous proteins, adhesive glycoproteins, glycosaminoglycans, and the mechanical forces that are found in the natural ovarian TME. Fibrous proteins, including collagen, are important because its presence is increased in the extracellular matrix of the disease state. This increases the TME stiffness and collagen signaling, and its downstream effects. Adhesive glycoproteins like fibrin and fibrinogen play a vital role in interacting with ovarian cancer cells and can increase cancer cell adhesion and proliferation as well as aid in metastasis. Glycosaminoglycans play an interesting role in the ovarian TME because they can be both pro-tumor and anti-tumor depending on their molecular weight and presentation. High molecular weight hyaluronic acid can play a protumor role as a ligand for its receptor, CD44. The CD44 receptor is known to be associated with cancer cell proliferation, metastasis, and chemoresistance. Cell surface bound heparan sulfate can be pro-angiogenic and lead to cellular proliferation while soluble heparan sulfate can disrupt the fibrin capsule and increase the antitumor immune response. Because of these interactions, these ECM components play an important role in ovarian cancer progression and an accurate model should account for their effects. Accurately capturing this complex interplay between matrix components that regulate ovarian cancer cell behaviors is a daunting challenge for the generation of appropriate models. Current 3D models being utilized to study ovarian TME and cancer progression include PDX mouse models and MPS. PDX mouse models have the advantages of having a natural microenvironment already incorporated. Unfortunately, a mouse ovarian TME does not fully equate to that of a human and interactions between the TME and the cancer cells can be lost. While MPS-based studies have uncovered significant findings for ovarian tumor growth due to their high levels of customization and control, there are still limitations including difficulties with device design and fabrication and working with small volumes. Overall, materials and model choices should be considered and the options that best represent the human native ovarian TME should be chosen. The next-generation of in vitro or ex vivo models of the ovarian TME should combine the high levels of physiological complexity of murine models with the adaptability of MPS to uncover specific mechanisms that drive tumor growth, metastasis, and response to chemotherapies. A model that utilizes human tumor cells and stromal components, including immune cells, embedded in a matrix that mimics the native ovarian ECM would elucidate the biochemical and biomechanical crosstalk that regulates tumor progression and ultimately assist in the development of novel, innovative therapies to improve patient outcomes.
Author contributions
Writing—original draft preparation: MK and AC Writing—reviewing and editing: MK and MS-L. Drafting and critical revision of the work: MS-L. All authors contributed to the article and approved the submitted version.
Funding
The authors wish to thank the following funding sources: R00-CA230202 (National Cancer Institute, MS-L), IMPACT Award (O’Neal Comprehensive Cancer Center, MS-L), the Fine Family Philanthropic Grant (MS-L), and T32-EB023872 (National Heart, Lung, and Blood Institute, MK).
Conflict of interest
MS-L receives compensation for consulting services from CerFlux, Incorporated.
The remaining authors declare that the research was conducted in the absence of any commercial or financial relationships that could be construed as a potential conflict of interest.
Publisher’s note
All claims expressed in this article are solely those of the authors and do not necessarily represent those of their affiliated organizations, or those of the publisher, the editors and the reviewers. Any product that may be evaluated in this article, or claim that may be made by its manufacturer, is not guaranteed or endorsed by the publisher.
References
Ahmed, N., Riley, C., Rice, G., and Quinn, M. (2005). Role of integrin receptors for fibronectin, collagen and laminin in the regulation of ovarian carcinoma functions in response to a matrix microenvironment. Clin. Exp. Metastasis 22, 391–402. doi:10.1007/s10585-005-1262-y
Ajeti, V., Lara-Santiago, J., Alkmin, S., and Campagnola, P. J. (2017). Ovarian and breast cancer migration dynamics on laminin and fibronectin bidirectional gradient fibers fabricated via multiphoton excited photochemistry. Cell Mol. Bioeng. 10, 295–311. doi:10.1007/s12195-017-0492-9
Alcoser, T. A., Bordeleau, F., Carey, S. P., Lampi, M. C., Kowal, D. R., Somasegar, S., et al. (2015). Probing the biophysical properties of primary breast tumor-derived fibroblasts. Cell Mol. Bioeng. 8, 76–85. doi:10.1007/s12195-014-0360-9
Alkmin, S., Brodziski, R., Simon, H., Hinton, D., Goldsmith, R. H., Patankar, M., et al. (2020). Role of collagen fiber morphology on ovarian cancer cell migration using image-based models of the extracellular matrix. Cancers (Basel) 12, 1390. doi:10.3390/cancers12061390
Amargant, F., Manuel, S. L., Tu, Q., Parkes, W. S., Rivas, F., Zhou, L. T., et al. (2020). Ovarian stiffness increases with age in the mammalian ovary and depends on collagen and hyaluronan matrices. Aging Cell 19, e13259. doi:10.1111/acel.13259
Ansardamavandi, A., Tafazzoli-Shadpour, M., Omidvar, R., and Nili, F. (2020). <p>An AFM-based nanomechanical study of ovarian tissues with pathological conditions</p>. Int. J. Nanomedicine 15, 4333–4350. doi:10.2147/ijn.s254342
Asem, M., Young, A., Oyama, C., Clauredelazerda, A., Liu, Y., Ravosa, M. J., et al. (2020). Ascites-induced compression alters the peritoneal microenvironment and promotes metastatic success in ovarian cancer. Sci. Rep. 10, 11913. doi:10.1038/s41598-020-68639-2
Atiya, H. I., Orellana, T. J., Wield, A., Frisbie, L., and Coffman, L. G. (2021). An orthotopic mouse model of ovarian cancer using human stroma to promote metastasis. J. Vis. Exp. 169. doi:10.3791/62382
Avraham-Chakim, L., Elad, D., Zaretsky, U., Kloog, Y., Jaffa, A., and Grisaru, D. (2013). Fluid-flow induced wall shear stress and epithelial ovarian cancer peritoneal spreading. PLoS One 8, e60965. doi:10.1371/journal.pone.0060965
Babahosseini, H., Ketene, A. N., Schmelz, E. M., Roberts, P. C., and Agah, M. (2014). Biomechanical profile of cancer stem-like/tumor-initiating cells derived from a progressive ovarian cancer model. Nanomedicine 10, 1013–1019. doi:10.1016/j.nano.2013.12.009
Bach, T. L., Barsigian, C., Yaen, C. H., and Martinez, J. (1998). Endothelial cell VE-cadherin functions as a receptor for the β15–42 sequence of fibrin. J. Biol. Chem. 273, 30719–30728. doi:10.1074/jbc.273.46.30719
Banzato, A., Bobisse, S., Rondina, M., Renier, D., Bettella, F., Esposito, G., et al. (2008). A paclitaxel-hyaluronan bioconjugate targeting ovarian cancer affords a potent in vivo therapeutic activity. Clin. Cancer Res. 14, 3598–3606. doi:10.1158/1078-0432.ccr-07-2019
Bar, J. K., Grelewski, P., Popiela, A., Noga, L., and Rabczynski, J. (2004). Type IV collagen and CD44v6 expression in benign, malignant primary and metastatic ovarian tumors: Correlation with ki-67 and p53 immunoreactivity. Gynecol. Oncol. 95, 23–31. doi:10.1016/j.ygyno.2004.06.046
Bascetin, R., Laurent-Issartel, C., Blanc-Fournier, C., Vendrely, C., Kellouche, S., Carreiras, F., et al. (2021). A biomimetic model of 3D fluid extracellular macromolecular crowding microenvironment fine-tunes ovarian cancer cells dissemination phenotype. Biomaterials 269, 120610. doi:10.1016/j.biomaterials.2020.120610
Ben-David, U., Ha, G., Tseng, Y. Y., Greenwald, N. F., Oh, C., Shih, J., et al. (2017). Patient-derived xenografts undergo mouse-specific tumor evolution. Nat. Genet. 49, 1567–1575. doi:10.1038/ng.3967
Biskup, K., Stellmach, C., Braicu, E. I., Sehouli, J., and Blanchard, V. (2021). Chondroitin sulfate disaccharides, a serum marker for primary serous epithelial ovarian cancer. Diagn. (Basel) 11, 1143. doi:10.3390/diagnostics11071143
Bourguignon, L. Y., Zhu, H., Chu, A., Iida, N., Zhang, L., and Hung, M. C. (1997). Interaction between the adhesion receptor, CD44, and the oncogene product, p185, promotes human ovarian tumor cell activation. J. Biol. Chem. 272, 27913–27918. doi:10.1074/jbc.272.44.27913
Bregenzer, M. E., Horst, E. N., Mehta, P., Novak, C. M., Repetto, T., and Mehta, G. (2019). The role of cancer stem cells and mechanical forces in ovarian cancer metastasis. Cancers (Basel) 11, 1008. doi:10.3390/cancers11071008
Buckley, M., Tidwell, M., Miller, B., Huskin, G., Berry, J., and Sewell-Loftin, M. K. (2023). Mechanical activation and expression of HSP27 in epithelial ovarian cancer. Res. Square 2023. doi:10.21203/rs.3.rs-2444182/v1
Byers, L. J., Osborne, J. L., Carson, L. F., Carter, J. R., Haney, A. F., Weinberg, J. B., et al. (1995). Increased levels of laminin in ascitic fluid of patients with ovarian cancer. Cancer Lett. 88, 67–72. doi:10.1016/0304-3835(94)03625-s
Campbell, K. R., and Campagnola, P. J. (2017). Assessing local stromal alterations in human ovarian cancer subtypes via second harmonic generation microscopy and analysis. J. Biomed. Opt. 22, 1–7. doi:10.1117/1.jbo.22.11.116008
Capo-Chichi, C. D., Smith, E. R., Yang, D. H., Roland, I. H., Vanderveer, L., Cohen, C., et al. (2002). Dynamic alterations of the extracellular environment of ovarian surface epithelial cells in premalignant transformation, tumorigenicity, and metastasis. Cancer 95, 1802–1815. doi:10.1002/cncr.10870
Carter, E. M., and Raggio, C. L. (2009). Genetic and orthopedic aspects of collagen disorders. Curr. Opin. Pediatr. 21, 46–54. doi:10.1097/mop.0b013e32832185c5
Carvalho, A. M., Soares Da Costa, D., Paulo, P. M. R., Reis, R. L., and Pashkuleva, I. (2021). Co-localization and crosstalk between CD44 and RHAMM depend on hyaluronan presentation. Acta Biomater. 119, 114–124. doi:10.1016/j.actbio.2020.10.024
Chalkiadaki, G., Nikitovic, D., Berdiaki, A., Sifaki, M., Krasagakis, K., Katonis, P., et al. (2009). Fibroblast growth factor-2 modulates melanoma adhesion and migration through a syndecan-4-dependent mechanism. Int. J. Biochem. Cell Biol. 41, 1323–1331. doi:10.1016/j.biocel.2008.11.008
Chen, H., Hao, J., Wang, L., and Li, Y. (2009). Coexpression of invasive markers (uPA, CD44) and multiple drug-resistance proteins (MDR1, MRP2) is correlated with epithelial ovarian cancer progression. Br. J. Cancer 101, 432–440. doi:10.1038/sj.bjc.6605185
Chen, L., Yao, Y., Sun, L., Zhou, J., Miao, M., Luo, S., et al. (2017). Snail driving alternative splicing of CD44 by ESRP1 enhances invasion and migration in epithelial ovarian cancer. Cell Physiol. Biochem. 43, 2489–2504. doi:10.1159/000484458
Cheon, D. J., Tong, Y., Sim, M. S., Dering, J., Berel, D., Cui, X., et al. (2014). A collagen-remodeling gene signature regulated by TGF-β signaling is associated with metastasis and poor survival in serous ovarian cancer. Clin. Cancer Res. 20, 711–723. doi:10.1158/1078-0432.ccr-13-1256
Cho, A., Howell, V. M., and Colvin, E. K. (2015). The extracellular matrix in epithelial ovarian cancer - a piece of a puzzle. Front. Oncol. 5, 245. doi:10.3389/fonc.2015.00245
Chopra, A., Lin, V., Mccollough, A., Atzet, S., Prestwich, G. D., Wechsler, A. S., et al. (2012). Reprogramming cardiomyocyte mechanosensing by crosstalk between integrins and hyaluronic acid receptors. J. Biomech. 45, 824–831. doi:10.1016/j.jbiomech.2011.11.023
Chopra, A., Murray, M. E., Byfield, F. J., Mendez, M. G., Halleluyan, R., Restle, D. J., et al. (2014). Augmentation of integrin-mediated mechanotransduction by hyaluronic acid. Biomaterials 35, 71–82. doi:10.1016/j.biomaterials.2013.09.066
Conrad, C., Moore, K., Polacheck, W., Rizvi, I., and Scarcelli, G. (2022). Mechanical modulation of ovarian cancer tumor nodules under flow. IEEE Trans. Biomed. Eng. 69, 294–301. doi:10.1109/tbme.2021.3092641
Davies, E. J., Blackhall, F. H., Shanks, J. H., David, G., Mcgown, A. T., Swindell, R., et al. (2004). Distribution and clinical significance of heparan sulfate proteoglycans in ovarian cancer. Clin. Cancer Res. 10, 5178–5186. doi:10.1158/1078-0432.ccr-03-0103
Deng, B., Zhao, Z., Kong, W., Han, C., Shen, X., and Zhou, C. (2022). Biological role of matrix stiffness in tumor growth and treatment. J. Transl. Med. 20, 540. doi:10.1186/s12967-022-03768-y
Erdogan, B., Ao, M., White, L. M., Means, A. L., Brewer, B. M., Yang, L., et al. (2017). Cancer-associated fibroblasts promote directional cancer cell migration by aligning fibronectin. J. Cell Biol. 216, 3799–3816. doi:10.1083/jcb.201704053
Fan, Y., Sun, Q., Li, X., Feng, J., Ao, Z., Li, X., et al. (2021). Substrate stiffness modulates the growth, phenotype, and chemoresistance of ovarian cancer cells. Front. Cell Dev. Biol. 9, 718834. doi:10.3389/fcell.2021.718834
Fan, Y., Wang, J., Fang, Z., Pierce, S. R., West, L., Staley, A., et al. (2022). Anti-tumor and anti-invasive effects of ONC201 on ovarian cancer cells and a transgenic mouse model of serous ovarian cancer. Front. Oncol. 12, 789450. doi:10.3389/fonc.2022.789450
Flont, M., Jastrzębska, E., and Brzózka, Z. (2020). Synergistic effect of the combination therapy on ovarian cancer cells under microfluidic conditions. Anal. Chim. Acta 1100, 138–148. doi:10.1016/j.aca.2019.11.047
Fogg, K. C., Renner, C. M., Christian, H., Walker, A., Marty-Santos, L., Khan, A., et al. (2020). Ovarian cells have increased proliferation in response to heparin-binding epidermal growth factor as collagen density increases. Tissue Eng. Part A 26, 747–758. doi:10.1089/ten.tea.2020.0001
Friedland, J. C., Lee, M. H., and Boettiger, D. (2009). Mechanically activated integrin switch controls α 5 β 1 function. Science 323, 642–644. doi:10.1126/science.1168441
Giancotti, F. R., Dorsett, B. H., Qian, H. N., Cronin, W. J., Barber, H. R., and Ioachim, H. L. (1990). Ovarian cancer-associated antibodies recovered from ascites: Their use for the isolation of ovarian cancer-associated antigen to produce monoclonal antibodies. Gynecol. Oncol. 37, 24–28. doi:10.1016/0090-8258(90)90301-z
Grabosch, S., Bulatovic, M., Zeng, F., Ma, T., Zhang, L., Ross, M., et al. (2019). Cisplatin-induced immune modulation in ovarian cancer mouse models with distinct inflammation profiles. Oncogene 38, 2380–2393. doi:10.1038/s41388-018-0581-9
Grässel, S., and Bauer, R. J. (2013). Collagen XVI in health and disease. Matrix Biol. 32, 64–73. doi:10.1016/j.matbio.2012.11.001
Grither, W. R., Divine, L. M., Meller, E. H., Wilke, D. J., Desai, R. A., Loza, A. J., et al. (2018). TWIST1 induces expression of discoidin domain receptor 2 to promote ovarian cancer metastasis. Oncogene 37, 1714–1729. doi:10.1038/s41388-017-0043-9
Grivennikov, S. I., and Karin, M. (2010). Inflammation and oncogenesis: A vicious connection. Curr. Opin. Genet. Dev. 20, 65–71. doi:10.1016/j.gde.2009.11.004
Hanahan, D., and Weinberg, R. A. (2011). Hallmarks of cancer: The next generation. Cell 144, 646–674. doi:10.1016/j.cell.2011.02.013
Hao, J., Tuck, A. R., Prakash, C. R., Damdimopoulos, A., Sjodin, M. O. D., Lindberg, J., et al. (2020). Culture of human ovarian tissue in xeno-free conditions using laminin components of the human ovarian extracellular matrix. J. Assist. Reprod. Genet. 37, 2137–2150. doi:10.1007/s10815-020-01886-4
He, Q. Z., Luo, X. Z., Wang, K., Zhou, Q., Ao, H., Yang, Y., et al. (2014). Isolation and characterization of cancer stem cells from high-grade serous ovarian carcinomas. Cell Physiol. Biochem. 33, 173–184. doi:10.1159/000356660
He, R. Y., Ajeti, V., Chen, S. J., Brewer, M. A., and Campagnola, P. J. (2015). Ovarian cancer cell adhesion/migration dynamics on micro-structured laminin gradients fabricated by multiphoton excited photochemistry. Bioeng. (Basel) 2, 139–159. doi:10.3390/bioengineering2030139
Henke, C. A., Roongta, U., Mickelson, D. J., Knutson, J. R., and Mccarthy, J. B. (1996). CD44-related chondroitin sulfate proteoglycan, a cell surface receptor implicated with tumor cell invasion, mediates endothelial cell migration on fibrinogen and invasion into a fibrin matrix. J. Clin. Invest. 97, 2541–2552. doi:10.1172/jci118702
Horowitz, M., Esakov, E., Rose, P., and Reizes, O. (2020). Signaling within the epithelial ovarian cancer tumor microenvironment: The challenge of tumor heterogeneity. Ann. Transl. Med. 8, 905. doi:10.21037/atm-2019-cm-08
Hu, L., Hofmann, J., Zaloudek, C., Ferrara, N., Hamilton, T., and Jaffe, R. B. (2002). Vascular endothelial growth factor immunoneutralization plus Paclitaxel markedly reduces tumor burden and ascites in athymic mouse model of ovarian cancer. Am. J. Pathol. 161, 1917–1924. doi:10.1016/s0002-9440(10)64467-7
Hyler, A. R., Baudoin, N. C., Brown, M. S., Stremler, M. A., Cimini, D., Davalos, R. V., et al. (2018). Fluid shear stress impacts ovarian cancer cell viability, subcellular organization, and promotes genomic instability. PLoS One 13, e0194170. doi:10.1371/journal.pone.0194170
Ibrahim, L. I., Hajal, C., Offeddu, G. S., Gillrie, M. R., and Kamm, R. D. (2022). Omentum-on-a-chip: A multicellular, vascularized microfluidic model of the human peritoneum for the study of ovarian cancer metastases. Biomaterials 288, 121728. doi:10.1016/j.biomaterials.2022.121728
Ip, C. K., Li, S. S., Tang, M. Y., Sy, S. K., Ren, Y., Shum, H. C., et al. (2016). Stemness and chemoresistance in epithelial ovarian carcinoma cells under shear stress. Sci. Rep. 6, 26788. doi:10.1038/srep26788
Iwanicki, M. P., Davidowitz, R. A., Ng, M. R., Besser, A., Muranen, T., Merritt, M., et al. (2011). Ovarian cancer spheroids use myosin-generated force to clear the mesothelium. Cancer Discov. 1, 144–157. doi:10.1158/2159-8274.cd-11-0010
Iyer, S., Zhang, S., Yucel, S., Horn, H., Smith, S. G., Reinhardt, F., et al. (2021). Genetically defined syngeneic mouse models of ovarian cancer as tools for the discovery of combination immunotherapy. Cancer Discov. 11, 384–407. doi:10.1158/2159-8290.cd-20-0818
Jain, R. K., Martin, J. D., and Stylianopoulos, T. (2014). The role of mechanical forces in tumor growth and therapy. Annu. Rev. Biomed. Eng. 16, 321–346. doi:10.1146/annurev-bioeng-071813-105259
Jarnagin, W. R., Rockey, D. C., Koteliansky, V. E., Wang, S. S., and Bissell, D. M. (1994). Expression of variant fibronectins in wound healing: Cellular source and biological activity of the EIIIA segment in rat hepatic fibrogenesis. J. Cell Biol. 127, 2037–2048. doi:10.1083/jcb.127.6.2037
Jiang, X. L., Gao, J. C., Jiang, L., Zhang, P. X., Kang, T. J., Sun, Q., et al. (2019). Expression and significance of MAPK/ERK in the specimens and cells of epithelial ovarian cancer. Zhonghua Fu Chan Ke Za Zhi 54, 541–547. doi:10.3760/cma.j.issn.0529-567x.2019.08.007
Kakkar, A. K., and Williamson, R. C. (1999). Antithrombotic therapy in cancer. BMJ 318, 1571–1572. doi:10.1136/bmj.318.7198.1571
Karakashev, S., and Zhang, R. G. (2021). Mouse models of epithelial ovarian cancer for preclinical studies. Zool. Res. 42, 153–160. doi:10.24272/j.issn.2095-8137.2020.382
Kauppila, S., Saarela, J., Stenbäck, F., Risteli, J., Kauppila, A., and Risteli, L. (1996). Expression of mRNAs for type I and type III procollagens in serous ovarian cystadenomas and cystadenocarcinomas. Am. J. Pathol. 148, 539–548.
Kenny, H. A., Chiang, C. Y., White, E. A., Schryver, E. M., Habis, M., Romero, I. L., et al. (2014). Mesothelial cells promote early ovarian cancer metastasis through fibronectin secretion. J. Clin. Invest. 124, 4614–4628. doi:10.1172/jci74778
Khanna, C., and Hunter, K. (2005). Modeling metastasis in vivo. Carcinogenesis 26, 513–523. doi:10.1093/carcin/bgh261
Klymenko, Y., Wates, R. B., Weiss-Bilka, H., Lombard, R., Liu, Y., Campbell, L., et al. (2018). Modeling the effect of ascites-induced compression on ovarian cancer multicellular aggregates. Dis. Model Mech. 11, dmm034199. doi:10.1242/dmm.034199
Koopmann, W., Ediriwickrema, C., and Krangel, M. S. (1999). Structure and function of the glycosaminoglycan binding site of chemokine macrophage-inflammatory protein-1β. J. Immunol. 163, 2120–2127. doi:10.4049/jimmunol.163.4.2120
Lalla, R. V., Goralnick, S. J., Tanzer, M. L., and Kreutzer, D. L. (2001). Fibrin induces IL-8 expression from human oral squamous cell carcinoma cells. Oral Oncol. 37, 234–242. doi:10.1016/s1368-8375(00)00090-7
Lengyel, E. (2010). Ovarian cancer development and metastasis. Am. J. Pathol. 177, 1053–1064. doi:10.2353/ajpath.2010.100105
Lesley, J., Hascall, V. C., Tammi, M., and Hyman, R. (2000). Hyaluronan binding by cell surface CD44. J. Biol. Chem. 275, 26967–26975. doi:10.1016/s0021-9258(19)61467-5
Li, S. S., Ip, C. K., Tang, M. Y., Sy, S. K., Yung, S., Chan, T. M., et al. (2017). Modeling ovarian cancer multicellular spheroid behavior in a dynamic 3D peritoneal microdevice. J. Vis. Exp. 2017, 55337. doi:10.3791/55337
Liu, D., Shriver, Z., Qi, Y., Venkataraman, G., and Sasisekharan, R. (2002). Dynamic regulation of tumor growth and metastasis by heparan sulfate glycosaminoglycans. Semin. Thromb. Hemost. 28, 67–78. doi:10.1055/s-2002-20565
Liu, Y., Chanana, P., Davila, J. I., Hou, X., Zanfagnin, V., Mcgehee, C. D., et al. (2019). Gene expression differences between matched pairs of ovarian cancer patient tumors and patient-derived xenografts. Sci. Rep. 9, 6314. doi:10.1038/s41598-019-42680-2
Ma, S. N., Mao, Z. X., Wu, Y., Liang, M. X., Wang, D. D., Chen, X., et al. (2020). The anti-cancer properties of heparin and its derivatives: A review and prospect. Cell Adh Migr. 14, 118–128. doi:10.1080/19336918.2020.1767489
Magnotti, E., and Marasco, W. A. (2018). The latest animal models of ovarian cancer for novel drug discovery. Expert Opin. Drug Discov. 13, 249–257. doi:10.1080/17460441.2018.1426567
Maniati, E., Berlato, C., Gopinathan, G., Heath, O., Kotantaki, P., Lakhani, A., et al. (2020). Mouse ovarian cancer models recapitulate the human tumor microenvironment and patient response to treatment. Cell Rep. 30, 525–540 e7. doi:10.1016/j.celrep.2019.12.034
Martinez, A., Buckley, M., Scalise, C. B., Katre, A. A., Dholakia, J. J., Crossman, D., et al. (2021). Understanding the effect of mechanical forces on ovarian cancer progression. Gynecol. Oncol. 162, 154–162. doi:10.1016/j.ygyno.2021.04.003
Martinez, J., Ferber, A., Bach, T. L., and Yaen, C. H. (2001). Interaction of fibrin with VE-cadherin. Ann. N. Y. Acad. Sci. 936, 386–405. doi:10.1111/j.1749-6632.2001.tb03524.x
Mckenzie, A. J., Hicks, S. R., Svec, K. V., Naughton, H., Edmunds, Z. L., and Howe, A. K. (2018). The mechanical microenvironment regulates ovarian cancer cell morphology, migration, and spheroid disaggregation. Sci. Rep. 8, 7228. doi:10.1038/s41598-018-25589-0
Mendoza-Martinez, A. K., Loessner, D., Mata, A., and Azevedo, H. S. (2021). Modeling the tumor microenvironment of ovarian cancer: The application of self-assembling biomaterials. Cancers (Basel) 13, 5745. doi:10.3390/cancers13225745
Morin, P. J., and Weeraratna, A. T. (2016). Genetically-defined ovarian cancer mouse models. J. Pathol. 238, 180–184. doi:10.1002/path.4663
Morla, S. (2019). Glycosaminoglycans and glycosaminoglycan mimetics in cancer and inflammation. Int. J. Mol. Sci. 20, 1963. doi:10.3390/ijms20081963
Motohara, T., Masuda, K., Morotti, M., Zheng, Y., El-Sahhar, S., Chong, K. Y., et al. (2019). An evolving story of the metastatic voyage of ovarian cancer cells: Cellular and molecular orchestration of the adipose-rich metastatic microenvironment. Oncogene 38, 2885–2898. doi:10.1038/s41388-018-0637-x
Mulloy, B., and Linhardt, R. J. (2001). Order out of complexity--protein structures that interact with heparin. Curr. Opin. Struct. Biol. 11, 623–628. doi:10.1016/s0959-440x(00)00257-8
Mundhenke, C., Meyer, K., Drew, S., and Friedl, A. (2002). Heparan sulfate proteoglycans as regulators of fibroblast growth factor-2 receptor binding in breast carcinomas. Am. J. Pathol. 160, 185–194. doi:10.1016/s0002-9440(10)64362-3
Nantasupha, C., Thonusin, C., Charoenkwan, K., Chattipakorn, S., and Chattipakorn, N. (2021). Metabolic reprogramming in epithelial ovarian cancer. Am. J. Transl. Res. 13, 9950–9973.
Ni, H., Yuen, P. S., Papalia, J. M., Trevithick, J. E., Sakai, T., Fässler, R., et al. (2003). Plasma fibronectin promotes thrombus growth and stability in injured arterioles. Proc. Natl. Acad. Sci. U. S. A. 100, 2415–2419. doi:10.1073/pnas.2628067100
Noble, P. W., Mckee, C. M., Cowman, M., and Shin, H. S. (1996). Hyaluronan fragments activate an NF-kappa B/I-kappa B alpha autoregulatory loop in murine macrophages. J. Exp. Med. 183, 2373–2378. doi:10.1084/jem.183.5.2373
Novak, C., Horst, E., and Mehta, G. (2018). Review: Mechanotransduction in ovarian cancer: Shearing into the unknown. Apl. Bioeng. 2, 031701. doi:10.1063/1.5024386
Novak, C. M., Horst, E. N., Lin, E., and Mehta, G. (2020). Compressive stimulation enhances ovarian cancer proliferation, invasion, chemoresistance, and mechanotransduction via CDC42 in a 3D bioreactor. Cancers (Basel). 12, 1521. doi:10.3390/cancers12061521
Obara, M., Kang, M. S., and Yamada, K. M. (1988). Site-directed mutagenesis of the cell-binding domain of human fibronectin: Separable, synergistic sites mediate adhesive function. Cell 53, 649–657. doi:10.1016/0092-8674(88)90580-6
Oliveira-Ferrer, L., Schmalfeldt, B., Dietl, J., Bartmann, C., Schumacher, U., and Stürken, C. (2022). Ovarian cancer-cell pericellular hyaluronan deposition negatively impacts prognosis of ovarian cancer patients. Biomedicines 10, 2944. doi:10.3390/biomedicines10112944
Ostrovsky, O., Berman, B., Gallagher, J., Mulloy, B., Fernig, D. G., Delehedde, M., et al. (2002). Differential effects of heparin saccharides on the formation of specific fibroblast growth factor (FGF) and FGF receptor complexes. J. Biol. Chem. 277, 2444–2453. doi:10.1074/jbc.m108540200
Pagliari, S., Forte, G., Vinarský, V., and Forte, G. (2018). Cellular mechanotransduction: From tension to function. Front. Physiol. [Online] 9, 824. doi:10.3389/fphys.2018.00824
Pauloin, T., Dutot, M., Warnet, J. M., and Rat, P. (2008). In vitro modulation of preservative toxicity: High molecular weight hyaluronan decreases apoptosis and oxidative stress induced by benzalkonium chloride. Eur. J. Pharm. Sci. 34, 263–273. doi:10.1016/j.ejps.2008.04.006
Prevo, R., Banerji, S., Ferguson, D. J., Clasper, S., and Jackson, D. G. (2001). Mouse LYVE-1 is an endocytic receptor for hyaluronan in lymphatic endothelium. J. Biol. Chem. 276, 19420–19430. doi:10.1074/jbc.m011004200
Qian, J., Lesavage, B. L., Hubka, K. M., Ma, C., Natarajan, S., Eggold, J. T., et al. (2021). Cancer-associated mesothelial cells promote ovarian cancer chemoresistance through paracrine osteopontin signaling. J. Clin. Invest. 131, e146186. doi:10.1172/jci146186
Rabenstein, D. L. (2002). Heparin and heparan sulfate: Structure and function. Nat. Prod. Rep. 19, 312–331. doi:10.1039/b100916h
Raglow, Z., and Thomas, S. M. (2015). Tumor matrix protein collagen XIα1 in cancer. Cancer Lett. 357, 448–453. doi:10.1016/j.canlet.2014.12.011
Ricciardelli, C., and Rodgers, R. J. (2006). Extracellular matrix of ovarian tumors. Semin. Reprod. Med. 24, 270–282. doi:10.1055/s-2006-948556
Rick, J. W., Chandra, A., Dalle Ore, C., Nguyen, A. T., Yagnik, G., and Aghi, M. K. (2019). Fibronectin in malignancy: Cancer-specific alterations, protumoral effects, and therapeutic implications. Semin. Oncol. 46, 284–290. doi:10.1053/j.seminoncol.2019.08.002
Robinson, C. J., and Stringer, S. E. (2001). The splice variants of vascular endothelial growth factor (VEGF) and their receptors. J. Cell Sci. 114, 853–865. doi:10.1242/jcs.114.5.853
Rowley, J. E., Amargant, F., Zhou, L. T., Galligos, A., Simon, L. E., Pritchard, M. T., et al. (2020). Low molecular weight hyaluronan induces an inflammatory response in ovarian stromal cells and impairs gamete development in vitro. Int. J. Mol. Sci. 21, 1036. doi:10.3390/ijms21031036
Sasisekharan, R., Shriver, Z., Venkataraman, G., and Narayanasami, U. (2002). Roles of heparan-sulphate glycosaminoglycans in cancer. Nat. Rev. Cancer 2, 521–528. doi:10.1038/nrc842
Sewell-Loftin, M. K., Bayer, S. V. H., Crist, E., Hughes, T., Joison, S. M., Longmore, G. D., et al. (2017). Cancer-associated fibroblasts support vascular growth through mechanical force. Sci. Rep. 7, 12574. doi:10.1038/s41598-017-13006-x
Sewell-Loftin, M. K., Katz, J. B., George, S. C., and Longmore, G. D. (2020). Micro-strains in the extracellular matrix induce angiogenesis. Lab. Chip 20, 2776–2787. doi:10.1039/d0lc00145g
Shahid, S., Iman, A., Matti, U., Rachid, K., Assaf, A., Eveno, C., et al. (2019). Fibrin deposit on the peritoneal surface serves as a niche for cancer expansion in carcinomatosis patients. Neoplasia 21, 1091–1101. doi:10.1016/j.neo.2019.08.006
Shen, Y., Shen, R., Ge, L., Zhu, Q., and Li, F. (2012). Fibrillar type I collagen matrices enhance metastasis/invasion of ovarian epithelial cancer via β1 integrin and PTEN signals. Int. J. Gynecol. Cancer 22, 1316–1324. doi:10.1097/igc.0b013e318263ef34
Shield, K., Ackland, M. L., Ahmed, N., and Rice, G. E. (2009). Multicellular spheroids in ovarian cancer metastases: Biology and pathology. Gynecol. Oncol. 113, 143–148. doi:10.1016/j.ygyno.2008.11.032
Singh, P., Carraher, C., and Schwarzbauer, J. E. (2010). Assembly of fibronectin extracellular matrix. Annu. Rev. Cell Dev. Biol. 26, 397–419. doi:10.1146/annurev-cellbio-100109-104020
Sun, Z., Guo, S. S., and Fässler, R. (2016). Integrin-mediated mechanotransduction. J. Cell Biol. 215, 445–456. doi:10.1083/jcb.201609037
Toole, B. P. (2004). Hyaluronan: From extracellular glue to pericellular cue. Nat. Rev. Cancer 4, 528–539. doi:10.1038/nrc1391
Tsang, S., Hassan, A., To, S., and Wong, A. (2022). Experimental models for ovarian cancer research. Exp. Cell Res. 416, 113150. doi:10.1016/j.yexcr.2022.113150
Tudrej, P., Kujawa, K. A., Cortez, A. J., and Lisowska, K. M. (2019). Characteristics of in vivo model systems for ovarian cancer studies. Diagnostics 9. 120. doi:10.3390/diagnostics9030120
Turley, E. A., Noble, P. W., and Bourguignon, L. Y. (2002). Signaling properties of hyaluronan receptors. J. Biol. Chem. 277, 4589–4592. doi:10.1074/jbc.r100038200
Vallen, M. J., Van Der Steen, S. C., Van Tilborg, A. A., Massuger, L. F., and Van Kuppevelt, T. H. (2014). Sulfated sugars in the extracellular matrix orchestrate ovarian cancer development: 'when sweet turns sour. Gynecol. Oncol. 135, 371–381. doi:10.1016/j.ygyno.2014.08.023
Vandamme, T. F. (2014). Use of rodents as models of human diseases. J. Pharm. Bioallied Sci. 6, 2–9. doi:10.4103/0975-7406.124301
Varki, N. M., and Varki, A. (2002). Heparin inhibition of selectin-mediated interactions during the hematogenous phase of carcinoma metastasis: Rationale for clinical studies in humans. Semin. Thromb. Hemost. 28, 53–66. doi:10.1055/s-2002-20564
Volpi, N. (2006). Therapeutic applications of glycosaminoglycans. Curr. Med. Chem. 13, 1799–1810. doi:10.2174/092986706777452470
Wang, Y., Yang, X., Yuan, M., Xian, S., Zhang, L., Yang, D., et al. (2019). Promotion of ovarian cancer cell invasion, migration and colony formation by the miR-21/Wnt/CD44v6 pathway. Oncol. Rep. 42, 91–102. doi:10.3892/or.2019.7153
Wei, J., Hu, M., Huang, K., Lin, S., and Du, H. (2020). Roles of proteoglycans and glycosaminoglycans in cancer development and progression. Int. J. Mol. Sci. 21, 5983. doi:10.3390/ijms21175983
Weroha, S. J., Becker, M. A., Enderica-Gonzalez, S., Harrington, S. C., Oberg, A. L., Maurer, M. J., et al. (2014). Tumorgrafts as in vivo surrogates for women with ovarian cancer. Clin. Cancer Res. 20, 1288–1297. doi:10.1158/1078-0432.ccr-13-2611
Whitham, K. M., Hadley, J. L., Morris, H. G., Andrew, S. M., Nieduszynski, I. A., and Brown, G. M. (1999). An improved method for the structural profiling of keratan sulfates: Analysis of keratan sulfates from brain and ovarian tumors. Glycobiology 9, 285–291. doi:10.1093/glycob/9.3.285
Wiener, J. R., Windham, T. C., Estrella, V. C., Parikh, N. U., Thall, P. F., Deavers, M. T., et al. (2003). Activated SRC protein tyrosine kinase is overexpressed in late-stage human ovarian cancers. Gynecol. Oncol. 88, 73–79. doi:10.1006/gyno.2002.6851
Wisdom, K. M., Adebowale, K., Chang, J., Lee, J. Y., Nam, S., Desai, R., et al. (2018). Matrix mechanical plasticity regulates cancer cell migration through confining microenvironments. Nat. Commun. 9, 4144. doi:10.1038/s41467-018-06641-z
Xu, W., Mezencev, R., Kim, B., Wang, L., Mcdonald, J., and Sulchek, T. (2012). Cell stiffness is a biomarker of the metastatic potential of ovarian cancer cells. PLoS One 7, e46609. doi:10.1371/journal.pone.0046609
Yang, D. H., Smith, E. R., Cohen, C., Wu, H., Patriotis, C., Godwin, A. K., et al. (2002). Molecular events associated with dysplastic morphologic transformation and initiation of ovarian tumorigenicity. Cancer 94, 2380–2392. doi:10.1002/cncr.10497
Yang, J. T., Rayburn, H., and Hynes, R. O. (1993). Embryonic mesodermal defects in alpha 5 integrin-deficient mice. Development 119, 1093–1105. doi:10.1242/dev.119.4.1093
Ye, J., Zhang, H., Wu, H., Wang, C., Shi, X., Xie, J., et al. (2012). Cytoprotective effect of hyaluronic acid and hydroxypropyl methylcellulose against DNA damage induced by thimerosal in Chang conjunctival cells. Graefes Arch. Clin. Exp. Ophthalmol. 250, 1459–1466. doi:10.1007/s00417-012-2087-4
Yoneda, A., Lendorf, M. E., Couchman, J. R., and Multhaupt, H. A. (2012). Breast and ovarian cancers: A survey and possible roles for the cell surface heparan sulfate proteoglycans. J. Histochem Cytochem 60, 9–21. doi:10.1369/0022155411428469
Yousif, N. G. (2014). Fibronectin promotes migration and invasion of ovarian cancer cells through up-regulation of FAK-PI3K/Akt pathway. Cell Biol. Int. 38, 85–91. doi:10.1002/cbin.10184
Zaman, A., Cui, Z., Foley, J. P., Zhao, H., Grimm, P. C., Delisser, H. M., et al. (2005). Expression and role of the hyaluronan receptor RHAMM in inflammation after bleomycin injury. Am. J. Respir. Cell Mol. Biol. 33, 447–454. doi:10.1165/rcmb.2004-0333oc
Zhang, J., Yuan, B., Zhang, H., and Li, H. (2019). Human epithelial ovarian cancer cells expressing CD105, CD44 and CD106 surface markers exhibit increased invasive capacity and drug resistance. Oncol. Lett. 17, 5351–5360. doi:10.3892/ol.2019.10221
Zhang, X., Sheng, Y., Li, B., Wang, Q., Liu, X., and Han, J. (2021). Ovarian cancer derived PKR1 positive exosomes promote angiogenesis by promoting migration and tube formation in vitro. Cell Biochem. Funct. 39, 308–316. doi:10.1002/cbf.3583
Zhao, Y., Cao, J., Melamed, A., Worley, M., Gockley, A., Jones, D., et al. (2019). Losartan treatment enhances chemotherapy efficacy and reduces ascites in ovarian cancer models by normalizing the tumor stroma. Proc. Natl. Acad. Sci. U. S. A. 116, 2210–2219. doi:10.1073/pnas.1818357116
Keywords: ovarian cancer, tumor microenvironment, matrix properties, microphysiological systems, mechanotransduction
Citation: Kramer M, Criswell A and Sewell-Loftin MK (2023) Biomaterial considerations for ovarian cancer models. Front. Mater. 10:1223276. doi: 10.3389/fmats.2023.1223276
Received: 15 May 2023; Accepted: 17 July 2023;
Published: 03 August 2023.
Edited by:
Jason Coombes, King’s College London, United KingdomReviewed by:
Chase Cornelison, University of Massachusetts Amherst, United StatesValeria Panzetta, University of Naples Federico II, Italy
Copyright © 2023 Kramer, Criswell and Sewell-Loftin. This is an open-access article distributed under the terms of the Creative Commons Attribution License (CC BY). The use, distribution or reproduction in other forums is permitted, provided the original author(s) and the copyright owner(s) are credited and that the original publication in this journal is cited, in accordance with accepted academic practice. No use, distribution or reproduction is permitted which does not comply with these terms.
*Correspondence: Mary Kathryn Sewell-Loftin, mksewellloftin@uab.edu