- 1Department of Chemistry and Bioscience, Aalborg University, Aalborg, Denmark
- 2Department of Materials Science and Engineering, The Pennsylvania State University, University Park, PA, United States
Predicting the atomic-scale structure of multicomponent glasses from their composition and thermal history would greatly accelerate the discovery of new engineering and functional glasses. A statistical mechanics-based approach has recently been applied to predict the composition-structure evolution in binary oxide glasses by determining the relative entropic and enthalpic contributions to the bonding preferences. In this work, we first establish the network modifier-former interaction parameters in sodium silicate and sodium borate glasses to predict the structural evolution in sodium borosilicate glasses. Due to the significant variations in the experimentally determined structural speciation in borosilicate glasses, we perform classical molecular dynamics (MD) simulations to establish and validate our structural model. We also show that the statistical mechanical model naturally accounts for the difference in structural speciation from MD simulations and NMR experiments, which in turn arises from the difference in cooling rate and thus thermal history of the glasses. Finally, we demonstrate the predictive capability of the model by accurately accounting for the structural evolution in potassium borosilicate glasses without using any adjustable model parameters. This is possible, because all the interaction parameters are already established in the potassium silicate, potassium borate, and sodium borosilicate glasses, respectively.
Introduction
A multitude of applications of oxide glasses exist, including in the fields of construction materials (Almutawa et al., 2013), electronic substrates (Rahman and Padavettan, 2012), medical technology (Day et al., 2011), etc. There is thus a need to continually develop new glass compositions with physical and chemical properties tuned for each specific application (Naumis, 2005; Mauro, 2014; Mauro et al., 2016) while fulfilling a number of criteria related to their production, including glass-forming ability, cost, emissions, toxicity, refractory compatibility, etc. To accelerate the pace of glass composition development, correlations between the atomic-scale structure of glasses and their macroscopic properties need to be established, for example based on topological constraint theory (Mauro, 2011; Micoulaut, 2016; Smedskjaer et al., 2017; Bauchy, 2019).
Oxide glasses are composed of network formers (such as Si, B, or P), which form the structural backbone and are linked together through bridging oxygen (BO). Network modifiers (such as Na or Ca) break the backbone by forming non-bridging oxygen (NBO) or stabilizing negatively charged network formers. Such short range order (SRO) rearrangements have been intensively investigated using various analytical tools, including solid state nuclear magnetic resonance (NMR) spectroscopy (Youngman, 2018), neutron diffraction (Fischer et al., 2006), and Raman spectroscopy (Neuville et al., 2014). Despite the significant advances within these technologies, the SRO analysis of glasses remains a tedious task due to their non-crystalline nature (Mauro, 2014) and the fact that they feature wide bond angle and length distributions. The SRO of glasses can be accurately characterized in simple compositions with only two to maybe three oxide components, whereas the accuracy drops for multicomponent compositions (Eckert, 2018). Moreover, predicting the structural descriptors (such as average coordination number of network formers) in multicomponent glasses is often impossible with the current models available. Recently, a statistical mechanics based model (Mauro, 2013) has been applied to determine relative enthalpy barriers for modifiers to associate with the various network former units in binary modifier-former glasses (Bødker et al., 2018, 2019). In this work, we attempt to transfer the enthalpy barriers established for binary alkali borate and alkali silicate glasses to predict the structural evolution in ternary alkali borosilicate glasses.
Borosilicate glasses are among the most utilized glasses, e.g., as thermal shock resistant glassware (Lima and Monteiro, 2001) and nuclear waste immobilization (Plodinec, 2000), and their composition-structure relations have thus been intensively investigated (Martens and Müller-Warmuth, 2000). Addition of modifier cations can increase the connectivity of the glass by charge-stabilizing tetrahedral boron (Lelong et al., 2017), but also reduce it by depolymerizing silicon structural units, and at high modifier concentrations also boron. The network formers' competition for modifier cations depends on the Si/B ratio, as described by Bray (1985) and Araujo (1980). However, to our knowledge, a comprehensive composition-structure model, accounting for the quantitative boron and silicon speciation as function of Si/B ratio and modifier content and type(s), has not yet been developed. Knowledge of the evolution of structural units with modifier concentration and Si/B ratio is needed for predicting the properties of borosilicate glasses directly from their composition (for a fixed thermal and pressure history; Smedskjaer et al., 2011).
To gain additional information about the structure of borosilicate glasses, molecular dynamics (MD) simulations have also been conducted based on recent force field developments (Deng and Du, 2018; Wang et al., 2018). The timescales in MD simulations are typically reduced to the nanosecond scale (Micoulaut, 2016), resulting in typical quenching rates in the range of 1–10 K/ps. This leads to a much higher fictive temperature (Tf) in simulated glasses compared to experimental glasses. As a result, the structures of the MD-generated glasses have been frozen in at a higher temperature than the experimental counterparts, giving rise to distributions of structural units that are more entropically dominated (Tomozawa et al., 2005). Despite this disadvantage of the MD simulations, they provide additional structural details, including the complete atomic configuration (Massobrio et al., 2015). Moreover, structural analysis of the experimentally synthesized glasses also comes with some disadvantages, such as uncertainties related to the actual chemical composition (especially if it is not analyzed), inhomogeneity or phase separation, partial crystallization, and thermal history differences. In addition, there are uncertainties related to the data analysis, such as deconvolution of NMR spectra. For alkali borosilicate glasses, we have found that the reported structures (determined by NMR spectroscopy) differ significantly from study to study and even within the same studies for the nominally same glass compositions. For example, the fraction of Si with four BOs in Na2O-B2O3-2SiO2 has been found to be 0 and 57% by Bhasin et al. (1998) and Nanba et al. (2004), respectively, while the fraction of four-fold coordinated boron in Na2O-B2O3-1.33SiO2 has been reported to be both 44 and 62% in the same study (Martens and Müller-Warmuth, 2000).
To overcome these problems in this study, we first show how the statistical mechanics model of SRO structure established based on experimental NMR data can be linked to that based on MD simulations data. With this link established, we will then show that we can predict the structure of MD-simulated ternary sodium borosilicate glasses by first establishing relative enthalpic and entropic bonding preferences in binary sodium borates and silicates. To do so, we only need one additional parameter, namely, the modifiers' relative preference for associating with a silicate structural group relative to a borate structural group. This newly established parameter is then used to predict the structural evolution in potassium borosilicate glasses without any free parameters. These iterations will be based on structural data obtained by NMR experiments and MD simulations, including results from literature (Maekawa et al., 1991; Bhasin et al., 1998; Du and Cormack, 2004; Adkins and Cormack, 2011; Schuch et al., 2011; Deng and Du, 2018) for sodium borate, sodium silicate, and sodium borosilicate glasses as well as new MD simulations performed in this study for potassium borosilicate glasses.
Molecular Dynamics Simulations
Glass Preparation
Twelve glasses in the family of RK2O-B2O3-KSiO2 (see Table S1) were simulated by MD using LAMMPS (Plimpton, 1995). A combined potential of the Coulomb and Buckingham potential was used in combination with a potential spline for low values of separation to avoid the Buckingham catastrophe. We refer to the work of Deng and Du for details on the potential and potential spline (Deng and Du, 2018). The force field parameters and quenching procedure have also been taken from that study (Deng and Du, 2018). This includes using varying values for the B-O interaction according to the values of R and K. Cut-offs for all short-range interactions were 11 Å while long range Coulombic interactions were computed directly below 11 Å and using the Particle-Particle-Particle-Mesh (PPPM) method with a relative accuracy of 10−5 above 11 Å. A timestep of 1 fs was used for all simulations. Initially ~3,000 atoms were placed randomly in a simulation box with a density ~2% lower than the experimental value to allow for more realistic dynamics in the melt, while avoiding any unrealistic overlaps. This was followed by a potential energy minimization and 60 ps of equilibration in the NVT ensemble at 300 K. The temperature was then set to 6,000 K, allowing the system to equilibrate for 100 ps, followed by a step function to 5,000 K and another isothermal equilibration for 100 ps, both in the NVT ensemble. The system was then quenched from 5,000 to 300 K at 5 K/ps in the NVT ensemble, followed by structural relaxation at 300 K for 60 ps at zero pressure in the NPT ensemble. Finally, this was followed by another 60 ps of structural relaxation in the NVT ensemble.
Structural and Thermal Analysis
The number of BOs and NBOs associated with each silicon species was evaluated by first counting the number of oxygens within the first coordination shell of every boron and oxygen atom. In addition to providing the degree of connectivity around the Si atoms, it also yields the coordination number of boron as a function of composition. The potential used in the simulations has already been verified elsewhere (Deng and Du, 2018). This analysis was followed by counting the number of boron and silicon within the first coordination shell of all oxygens, hence categorizing each oxygen as either bridging or non-bridging. These two pieces of information were then combined to yield the number of NBOs associated with every boron and silicon atom in each simulation. All structural characteristics were averages of 10 structures from the final NVT equilibration.
Fictive temperatures (Tf) were found by employing the method of Liu et al. (2018). This method uses local ground-state enthalpy as a function of temperature to estimate Tf, giving very well-defined transitions compared to common methods of temperature-enthalpy plots as the method only considers the enthalpy of the atomic configuration at each temperature, leaving out contributions of atom dynamics.
Statistical Mechanical Structure Model
The statistical mechanical model used herein to predict the modifier-former associations in mixed network former glasses was first proposed by Mauro (2013) and later implemented and validated on binary oxide glasses by Bødker et al. (2018, 2019). The model is based on the assumption that the probability for the initially added network modifier to interact with a certain network former atom depends on the relative statistical entropy (i.e., the fraction of microstates consistent with the macrostate of each network former) and the relative enthalpic barrier for the modifier to break the bonds associated with each network former. Here, the microstates refer to the SRO structural units as each of these correspond to a specific potential energy, while the macrostate is the sum of structural units consistent with a given network former. Using Na2O-B2O3-SiO2 as an example, the probability for sodium to associate with a borate unit depends on the statistical entropy of boron (i.e., the fraction of boron to silicon content) and the enthalpic energy barrier for this interaction, relative to the enthalpic barrier of the sodium-silicon interaction. This is analogous to calculating the probabilities for drawing a red marble from an urn with both red and blue marbles with different sizes. If we know the relative fraction of the red marbles, i.e., the statistical entropy, and their relative size compared to blue marbles (analogous to the enthalpy barrier in the glass system), we can calculate the probability to draw a red marble over a blue using a non-central (weighted) distribution function (Mauro and Smedskjaer, 2014). In the glass system, we assume that the energy barrier for a modifier to interact with the network former is constant regardless of the composition. The statistical entropy of each network former species does, however, change upon interactions with a modifier ion, since a drawn species is not replaced. This results in a hypergeometric distribution that describes the evolution of network former species as a function of the modifier concentration. As the entropy of the system changes with composition, so does the probability for the modifier-former interaction, requiring a numerical solution to predict the structural evolution of network former species.
To establish the model (Mauro, 2013), we first consider the Boltzmann distribution function (Schwabl, 2006) that describes the probability (i.e., the weighting factor) for a system to be in a given state as a function of the system's temperature and the energy of that state,
where pi is the probability of state i, k is the Boltzmann constant, T is the temperature of the system, εi is the total energy of state i, and M is the total number of states. Here, pi describes the probability for a network modifier to interact with a given network former species i, and consequently, εi becomes the free energy of this interaction, which may be described by entropic and enthalpic contributions,
Next, we introduce the statistical entropy of the system as,
where, Ωi is the number of microstates consistent with a given macrostate for species i,
We then obtain,
or,
The number of microstates consistent with the macrostate of species i divided by the total number of microstates consistent with the macrostate of the oxide glass will be the same as the relative fraction of species i divided by the total number of species. Since the fraction of a given structural species i in the glass changes with composition, we obtain
where ω represents a given modifier concentration, gi is the degeneracy (initial fraction) of species i, and ni,ω is the total fraction of species i that has already interacted at modifier concentration ω. ω represents an absolute quantity of modifier ions, but we have converted it to a relative concentration for easy comparison with the experimental data. When calculating the probability of an interaction with species i at concentration ω, we must use the fraction of species i at the previous concentration step (ω−1),
The double summation in the denominator is over all species M and the accumulated number of successful interactions of species i after j number of attempts (ni, j) up to but not including the current modifier concentration ω. For each concentration step, the fraction ni,ω of network former species i interacting with the modifier ion can be calculated from the probability of the interaction and its fraction at the previous concentration step ω−1. Then, that fraction is subtracted from the remaining amount of network forming species i at the next concentration, which is used to calculate the new probability and so on. Hence, the probability distribution function in Equation (8) is a type of non-central hypergeometric distribution function, where the relative enthalpy Hi values are the free parameters when fitting to experimental data. We define as the weighting factor wi for a modifier to interact with the structural group i, where T is assumed to be equal to Tf for T < Tf, since the structure is assumed to freeze in at the fictive temperature:
where,
The application of Equation (9) to predict the structure of binary oxide glasses has been described in detail elsewhere (Bødker et al., 2018, 2019). Here, we will use the M2O-B2O3-SiO2 system, where M is an alkali metal, to explain the numerical procedure when calculating the compositional dependence of structural units with the present model. The SRO structural units of interest in the silicate part of the borosilicate network are the Qn units, where n is the number of BO per tetrahedron (n = 0, 1, 2, 3, or 4), while those of interest in the borate part are the Bn units, again with n = 0, 1, 2, 3, or 4.
First, following our previous study of alkali borate glasses (Bødker et al., 2019), we consider the reaction mechanisms for B3 and B4 structural groups when interacting with an alkali modifier oxide (M2O),
These two reactions describe the boron anomaly, where a three-fold coordinated B3 structural unit may either form a four-fold coordinated B4 structural unit without NBOs or a three-fold coordinated B2 unit with one NBO. Previous studies (Uhlmann and Shaw, 1969; Yiannopoulos et al., 2001) have shown that Equation (11) dominates at low modifier concentration, while Equation (12) dominates at higher modifier concentration. To capture this so-called boron anomaly in this study, we introduce a critical concentration above which a parameter (αB4/B2) changes from 1 to 0, allowing only one of the two reactions to occur at any point. This is a structural simplification, but as it will be shown, it enables us to establish a simple model, yet predict the structural evolution with sufficient accuracy. An additional complication occurs when a network modifier interacts with a B4 unit,
As the modifier concentration increases, the B4 structural units will begin to become converted to B2 units, forming new NBOs on either boron or silicon units close to the initial B4 unit. Then the modifier cations interact with the newly created NBOs. Equation (9) is used to calculate the fraction of the introduced modifier at each concentration step that initiates the conversion of a B4 structural unit; hence the fraction of free modifier is also known and must be included in the interactions at each ω. Analogous to our work on alkali phosphate glasses13, the following depolymerization reactions occur for all silicate structural units [and similarly for the B2 and B1 structural units (Bødker et al., 2019)],
With all the possible modifier interactions established, the fractions of the structural units at concentration ω are calculated as,
That is, the fraction of B3 at modifier concentration step ω (B3ω) equals the fraction of B3 at the previous concentration step () minus the fraction that reacts at ω () and also minus the fraction of B4 that is converted to B2 multiplied by the probability for the B3 to react (). The latter is needed, since the extra modifier from a B4 group (Equation 13) will react according to the bonding preference at this composition. In Equation (16), the number of B4 units will increase proportionally to the fraction of B3 units drawn (Equation 11), but only until the critical modifier concentration, where αB4/B2 changes from 1 to 0. Additional examples are given for B2 and Q3 structural units as,
In Equation (17), the fraction of B2 units will increase when a B3 is drawn (Equation 12) only when αB4/B2 is 0 and always when B3 is drawn by the modifiers released by B4 (). Additionally, the fraction of B2 will increase when a B4 is converted and become reduced when the modifier draws a B2 either initially () or after being released from a B4 (). The fractions of Q4, Q3,… Q0, B1, and B0 will increase according to the probability of their n+1 species to be drawn, but at the same time decrease according to the probability for the modifier to interact with that unit as shown in the Q3 example in Equation (18). Further explanation of the modeling procedure is given elsewhere (Bødker et al., 2019). Typically, the fitting parameters of the model would be all the relative Hi values (or wi, if Tf is unknown), but in this study, the relative Hi values are first established in binary sodium borate glasses and sodium silicate glasses (as demonstrated in section Validating Structure Model for Alkali Silicate and Borate Glasses below). These Hi values are then transferred to predict the structural evolution in the sodium borosilicate glasses with only one free parameter, the conversion factor (wSi,B). This parameter is needed, since all enthalpy values are calculated relatively within each system, i.e., the HQ3 in the sodium silicate is relative to the HQ4 parameter, while the HB2 in sodium borate is relative to the HB3 parameter.
Modeling of the composition-structure evolution in the binary and ternary systems to obtain the Hi values was performed in the object-oriented programming language Python. Here, the Basinhopping optimization method (part of the SciPy package) is used (Jones et al., 2001), since it attempts to find the global minimum in the parameter space by repeating the optimization with different guessed values of the starting parameters and solving for the structure in a self-consistent fashion. Additionally, all simulations were repeated five to 10 times with different starting values.
Results and Discussion
Validating Structure Model for Alkali Silicate and Borate Glasses
The statistical mechanical model has previously been established in binary phosphate (Bødker et al., 2018) and borate glasses (Bødker et al., 2019), but not yet in binary silicate glasses. The modeling procedure for silicate glasses is the same as for binary phosphate glasses (Bødker et al., 2018). Here, we apply this approach to sodium silicates (Figure 1A) and potassium silicates (Figure 1B) based on 29Si MAS NMR data from literature (Schroeder et al., 1973; Maekawa et al., 1991). To obtain relative Hi values for these glasses, we extrapolated the Tg values found in literature (Table S2) (Schroeder et al., 1973; MacDonald et al., 1985; Belova et al., 2015) using simple regression to estimate a Tg value for each modifier concentration step (ω). As shown in Figure 1, the model accurately captures the evolution of structural Qn units with the modifier concentration using the fitted Hi values as reported in Table 1. Similarly, the modeling of the structure of sodium borate (Shelby, 1983; Schuch et al., 2011) and potassium borate (Zhong and Bray, 1989) systems based on 11B MAS NMR data is shown in Figures S1a,b, with the corresponding Tg values from literature in Table S2. Only the fraction of B4 is obtained from 11B MAS NMR so these fits have been made using Equations (15) and (16) in section Statistical Mechanical Structure Model. Due to the limited amount of experimental data, these Hi values are very sensitive to any experimental uncertainties, which is especially the case for H3 and H4 of the borate glasses in the high modifier regions.
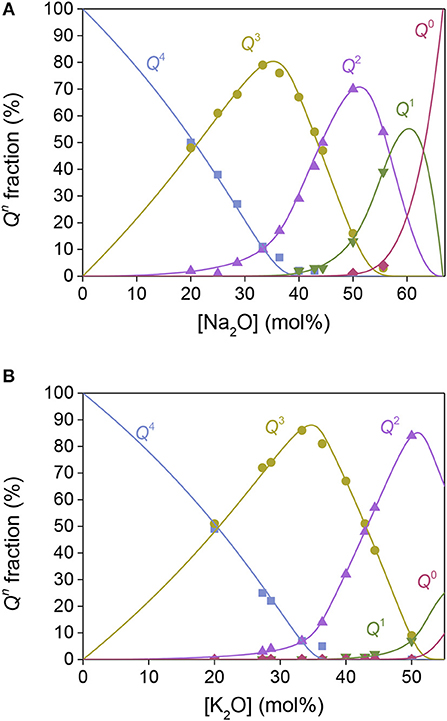
Figure 1. Composition dependence of the fraction of Qn structural units in (A) sodium silicate and (B) potassium silicate glasses. The closed symbols represent 29Si MAS NMR experimental data (from Maekawa et al., 1991 for sodium and Schroeder et al., 1973 and Maekawa et al., 1991 for potassium) and the solid lines represent the model predictions.
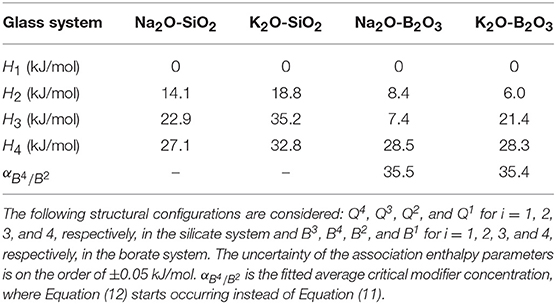
Table 1. Relative association enthalpies (Hi), where i corresponds to a given structural configuration, for the fitting of the current statistical mechanical model to experimental structure data.
Reconciling Structure Data From Experiments and MD Simulations
Simulated quenching of liquid to glasses using MD requires the use of high cooling rates, in turn yielding higher Tf values for MD glasses compared to experimental glasses. In other words, the structure freezes in at a higher temperature and becomes more entropically controlled. Assuming that the enthalpic contributions to the modifier-former interactions are the same for experimental and MD simulated glasses, the present statistical mechanical model should be able to predict the structural evolution in a MD simulated glass system based on the difference in fictive temperature. Figure 2A shows the Qn speciation in a 35Na2O-65SiO2 glass as a function of Tf, including model predictions, experimental data from 29Si NMR spectroscopy (Maekawa et al., 1991), and simulations data from a previous MD study (Adkins and Cormack, 2011). The good agreement between model and data confirms the hypothesis that the present model can be used to describe the MD simulated structure of oxide glasses based on experimentally obtained NMR data (or vice versa) with only Tf as the free parameter. Likewise, Figure 2B shows the fraction of four-fold coordinated boron (B4) in a 40Li2O-60B2O3 glass as a function of Tf, including model predictions from our previous study (Bødker et al., 2019) based on the fractions of superstructural units, experimental data from 10B NMR spectroscopy (Feller et al., 1982). Since the fraction of B4 varies non-monotonically with the fictive temperature according to the model (Bødker et al., 2019), the values of B4 fraction from NMR and MD are almost identical, in agreement with previous studies (Xu et al., 1988; Ohtori et al., 2001).
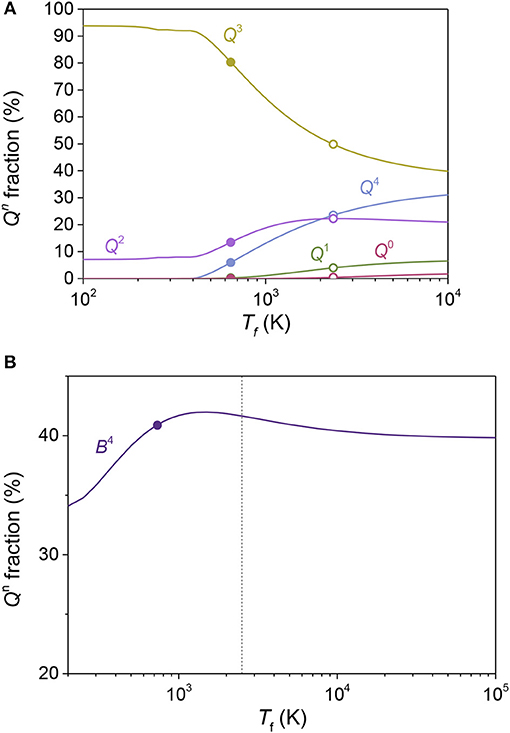
Figure 2. The fraction of structural units in (A) 35Na2O-65SiO2 and (B) 40Li2O-60B2O3 glasses as a function of fictive temperature (Tf). (A) The closed symbols represent 29Si MAS-NMR experimental data (Maekawa et al., 1991), the open symbols represent MD simulated data (Adkins and Cormack, 2011), and the solid lines represent the model predictions. (B) The closed symbol represent 10B NMR experimental data (Feller et al., 1982) and the dashed line is an estimate of Tf of the MD simulated glass. The solid line represents the model prediction.
In Figure 1A, the structural units in the Na2O-SiO2 system are plotted against the modifier concentration, where the solid symbols represent 29Si NMR data (Maekawa et al., 1991) and the lines as model predictions with known Tg values (MacDonald et al., 1985; Belova et al., 2015) with the resulting Hi parameters as reported in Table 1. Next, we use the obtained Hi values from the binary sodium silicate glasses (Table 1) to predict the structural evolution of the MD simulated Na2O-SiO2 glasses (Du and Cormack, 2004; Adkins and Cormack, 2011), with only an adjustable conversion factor from experimental Tg values to predicted Tf values (Figure 3). The excellent agreement suggests that we can predict MD simulated structures based on input from experimental structure data and the thermal history of the MD simulated glasses. With the link established between experimental and MD simulated glass structures, we are now able to utilize the proposed model to predict realistic glass structures of alkali borosilicates based only on knowledge of MD simulated glass structures. As it will be shown below, this becomes useful for multicomponent systems with composition fluctuations, phase separation, uncertain NMR deconvolutions, etc.
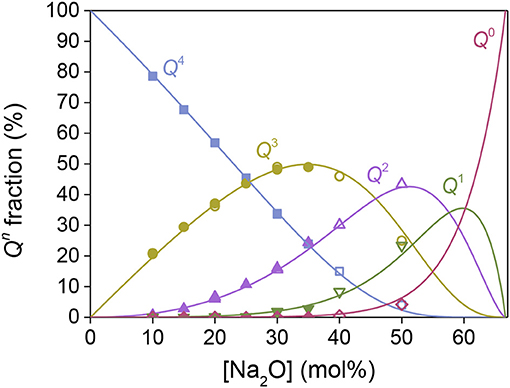
Figure 3. Composition dependence of the fraction of Qn structural units in sodium silicate glasses. The closed and open symbols represent MD simulated data obtained by Du and Cormack (2004) and Adkins and Cormack (2011), respectively. The solid lines represent model predictions, using the same bonding preferences parameter, but a different fictive temperature compared to Figure 1A.
Structure Model for Sodium Borosilicate Glasses
29Si NMR data of silica-containing glasses can be challenging to deconvolute, e.g., due to the bond angle distribution contributing to very broad and overlapping peaks (Mahler and Sebald, 1995). In binary alkali silicate glasses, the chemical composition of the glasses may be used to constrain the deconvolution by assuming neutral charge balance in the glass and that all modifiers interact with the structure, significantly improving the accuracy of the deconvolution. However, in borosilicate glasses, determination of the boron speciation typically only involves quantification of the boron coordination number with no distinction of symmetric (only BO) and asymmetric (one or more NBO) trigonal boron units. In this case, the chemical composition of the glasses cannot be used to constrain the 29Si NMR deconvolution, since the fraction of modifier associated with the boron species is unknown. Figure 4A shows the statistical mechanics-based model predictions compared to experimental data obtained by 11B and 29Si NMR spectroscopy techniques (Bhasin et al., 1998; Nanba et al., 2004) in the sodium borosilicate system. These predictions were made with only the relative Si/B weighting factor (wSi,B) as a free parameter. All Hi values and were transferred from the binary silicate and borate glasses, as reported in Table 1. The compositional evolution of Qn and Bn speciation are shown in Figures 4B,C, respectively, for glasses with K = 2. Model vs. experiments comparisons for other B/Si ratios (i.e., K value) are shown in Figure S2. Overall we find that although the major trends in Qn and Bn with composition are captured by the model (with only one adjustable parameter), there are relatively large deviations in the absolute values Qn and Bn between model and experiments.
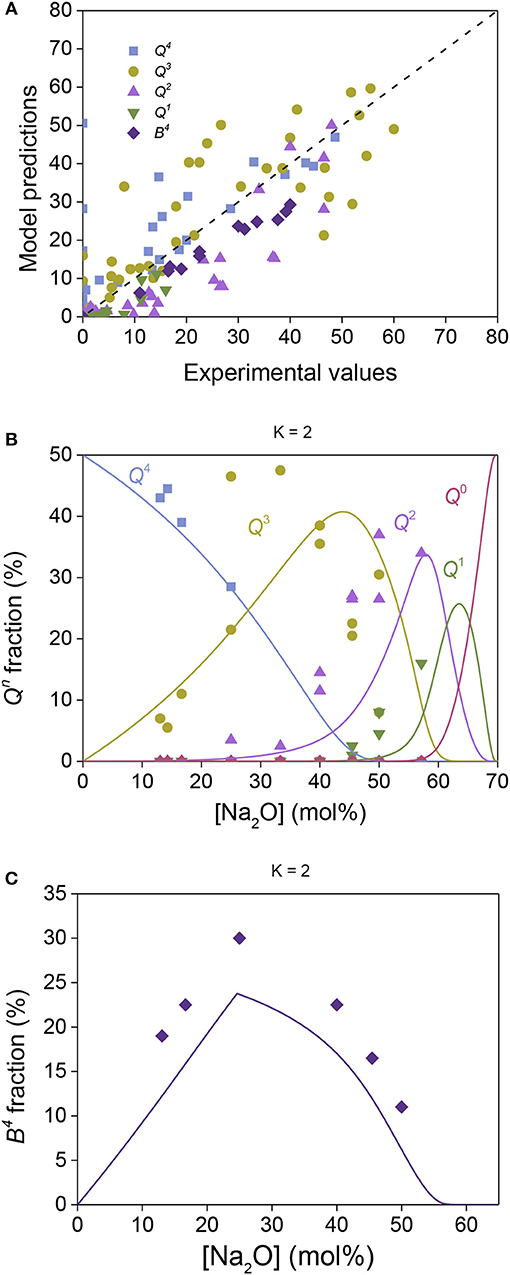
Figure 4. Sodium borosilicate structural data obtained by 29Si and 11B MAS NMR (Bhasin et al., 1998; Nanba et al., 2004) compared to model predictions. (A) All experimental data plotted against the model predictions, with the dashed line showing a one-to-one correlation. (B) Composition dependence of Qn fractions in sodium borosilicate glasses with K = 2, where the symbol represents experimental data by 29Si MAS NMR and the lines are model predictions. (C) Composition dependence of the fraction of four-fold coordinated boron (B4) in sodium borosilicate glasses with K = 2 as obtained by 11B MAS-NMR (symbols) and the model predictions (line).
To overcome the abovementioned experimental uncertainties associated with structure determination in borosilicate glasses, we next attempt to predict the composition dependence of silicon and boron speciation in MD simulated sodium borosilicate glasses, again based on the relative Hi values obtained separately in sodium silicate and sodium borate glasses (Table 1). Figure 5A shows the comparison of model predictions with MD simulated data (Deng and Du, 2018), obtained with only wSi,B as a fitting parameter found to be 0.16. The compositional evolution of Qn and Bn speciation are shown in Figures 5B,C, respectively, for glasses with K = 2, with additional comparisons given in Figure S3. Overall, we observe excellent agreement between the model predictions and MD data with only one adjustable parameter, supporting the assumption that the Hi values can be transferred from simple binary systems to multi-component systems containing the same interactions as in the binary glasses.
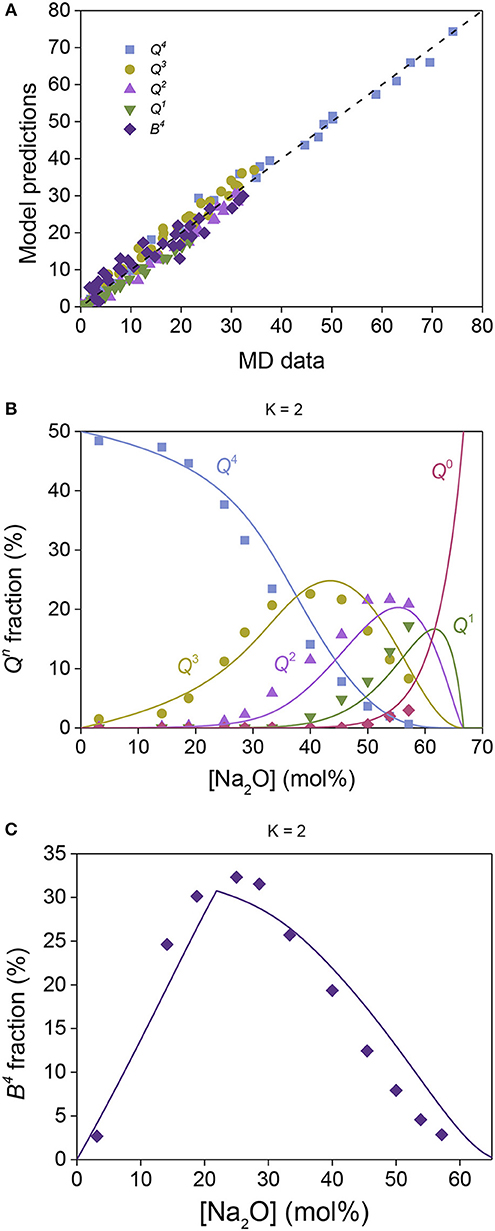
Figure 5. Sodium borosilicate structural data obtained by MD simulations (Deng and Du, 2018) compared to model predictions. (A) All experimental data plotted against the model predictions, where the dashed line shows the one-to-one correlation. (B) Qn fractions for silicate structural groups (symbols) and model predictions (lines) plotted against the overall modifier concentration for glasses with K = 2. (C) Fraction of four-fold coordinated boron as obtained by MD simulations (symbols) and the model predictions (line) against the overall modifier concentration for glasses with K = 2.
Structure Prediction of Potassium Borosilicate Glasses Without Free Parameters
To further test the validity and universality of the present model, the obtained wSi,B weighting factor from the sodium borosilicate glasses is transferred (along with the Hi values from Table 1) to predict the structural evolution in potassium borosilicate glasses. The structure data for these glasses are determined based on the MD simulations performed in this study (see section Molecular Dynamics Simulations). Figure 6A illustrates the general agreement between model predictions and MD data, while Figures 6B,C show the predicted Qn and Bn speciation, respectively, as a function of the modifier concentration for K = 2 (similar plots for K = 4 and 6 are shown in Figure S4). All the parameters used in the model have been obtained from different systems, namely, Hi and values from potassium silicate and potassium borate glasses (Table 1) and wSi,B = 0.16 from the sodium borosilicate glasses (section Structure model for sodium borosilicate glasses). In other words, no fitting is needed to obtain the model predictions. We note that a small amount of four-fold coordinated boron with one NBO is observed in the MD simulations (up to ~2% of the structure), but since this unit is not observed in the experimental data, it has been included as three-fold coordinated boron with two NBOs. This discrepancy is likely due to the use of the Buckingham potential and the higher fictive temperatures of the MD simulated glasses, resulting in a higher probability for energetically unfavorable structures.
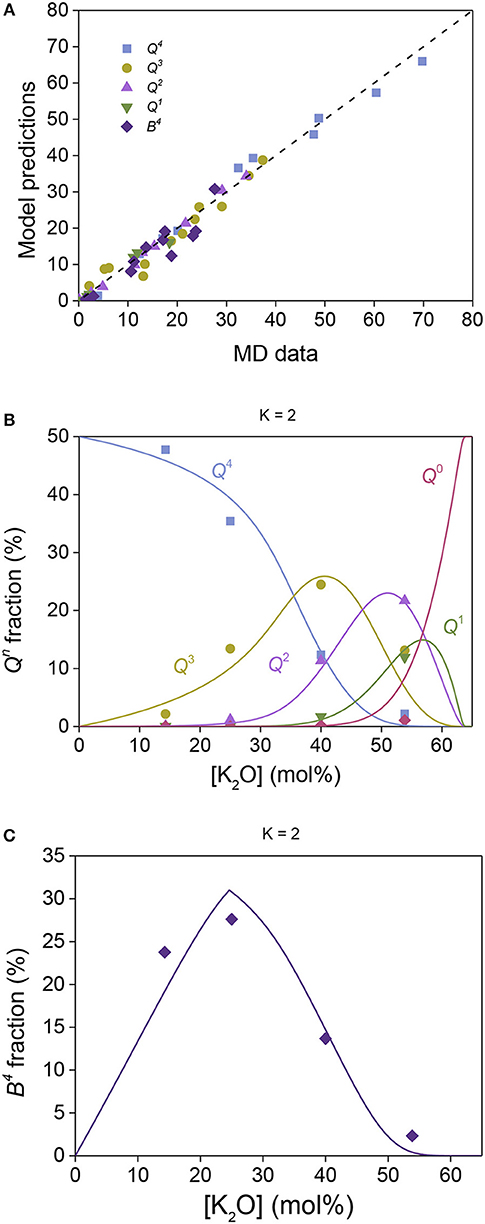
Figure 6. Potassium borosilicate structural data obtained by MD simulations compared to model predictions with zero free parameters. (A) All experimental data plotted against the model predictions, where the dashed line shows the one-to-one correlation. (B) Qn fractions for silicate structural groups (symbols) and model predictions (lines) plotted against the overall modifier concentration for glasses with K = 2. (C) Fraction of four-fold coordinated boron as obtained by MD simulations (symbols) and model predictions (line) plotted against the overall modifier concentration for glasses with K = 2.
To correct for the thermal history difference, the Tf values for the MD simulated glasses have been determined by extrapolating experimental data (Grandjean et al., 2008) the same way as shown in Figure 2A, i.e., all Tf values have been scaled with a constant relative to the experimental values. The predicted Tf values by this method are generally in good agreement with those determined by using the method of Liu et al. (2018) (see Figure S5).
Perspectives
Taking alkali borosilicate glasses as an example, we have found good agreement between the predicted values and those determined by MD simulations and also established a link between MD simulated structures in glasses and their melt-quenched counterparts. We expect this approach can be applied to predict the structure of various multicomponent glasses based on knowledge of the structure of the binary glasses that they are constituted by. This would greatly reduce the experimental or simulation time needed to predict the composition-structure evolution in multicomponent glasses. In this study, we included model predictions of glasses that contained two or three of the following four components: Na2O, K2O, B2O3, and SiO2. This required model simulations on four types of binary glasses, namely, Na2O-SiO2, K2O-SiO2, Na2O-B2O3, and K2O-B2O3. Building on these four simulations, we would in principle be able to predict seven different glass systems, such as K2O-Na2O-B2O3-SiO2, K2O-B2O3-SiO2, K2O-Na2O-SiO2, etc. However, to predict the composition-structure evolution in all these systems, the effect of mixing different types of modifiers on the structure should be accounted for. The probability distributions of interactions in mixed modifier systems have already been developed (Goyal and Mauro, 2018), but still need to be verified experimentally. Another limitation of the model is the Tf dependence of the predicted structural units. If the model is used to predict the structural evolution in compositions not yet established by MD or experiments, a model of thermal history dependence must be incorporated to iteratively estimate the structural evolution and evolution of Tf simultaneously.
As the number of oxide components increases, the number of required simulations would increase linearly, whereas the number of systems predicted from the simulations would increase exponentially. This makes the present approach a promising tool for screening the atomic scale structure of many multicomponent oxide-based glasses. Such prediction would especially be useful when it is coupled with a relevant structure-property model. Finally, we note that the present approach predicts the mean structures of a given composition. Recently, Kirchner et al. (2018) and Kirchner and Mauro (2019) proposed a statistical mechanics-based method to explore topological fluctuations in the glass structure and properties, which would be interesting to couple with the present composition-structure model for borosilicate glasses in future work.
Conclusions
We have shown that a structure model based on the Boltzmann distribution can be used to predict the structure of ternary borosilicate glasses by transferring model parameters from simpler glasses with some of the same components. We started by using experimental structure data for sodium silicate and sodium borate glasses to determine the relative enthalpy values for sodium to interact with each structural group within the network. Using these values, we then applied the model to predict the structural evolution in sodium borosilicate glass system with only one free parameter to account for the relative propensity for the sodium modifier to interact with the silicate and borate part of the network. This parameter is required since the determined enthalpy values are relative, not absolute. With the wSi,B parameter established for the sodium borosilicate system, we used the model to accurately predict the structural evolution in potassium borosilicate glasses without any free parameters, in this case building on transferred enthalpy values from experimental data of potassium silicate and potassium borate glasses. Finally, we have also shown that the statistical mechanical model is able to predict both experimental and MD structure data using only the fictive temperature as the scaling parameter.
Data Availability
The datasets generated for this study are available on request to the corresponding author.
Author Contributions
MS and MB designed the study. MB performed the statistical mechanical modeling and Python programming. SS performed the MD simulations. MB and MS wrote the manuscript with revisions from JM and SS. All authors participated in discussing the data.
Funding
This work was supported by the Independent Research Fund Denmark (grant no. 7017-00019). Computational resources were provided by the DeIC National HPC Center (ABACUS 2.0) at the University of Southern Denmark and funded by Aalborg University.
Conflict of Interest Statement
The authors declare that the research was conducted in the absence of any commercial or financial relationships that could be construed as a potential conflict of interest.
Acknowledgments
We thank Sushmit Goyal (Corning Inc.), Randall E. Youngman (Corning Inc.), Jincheng Du (University of North Texas), and Mathieu Bauchy (University of California, Los Angeles) for valuable discussions.
Supplementary Material
The Supplementary Material for this article can be found online at: https://www.frontiersin.org/articles/10.3389/fmats.2019.00175/full#supplementary-material
References
Adkins, L., and Cormack, A. (2011). Large-scale simulations of sodium silicate glasses. J. Non Cryst. Solids 357, 2538–2541. doi: 10.1016/j.jnoncrysol.2011.03.012
Almutawa, F., Vandal, R., Wang, S. Q., and Lim, H. W. (2013). Current status of photoprotection by window glass, automobile glass, window films, and sunglasses. Photodermatol. Photoimmunol. Photomed. 29, 65–72. doi: 10.1111/phpp.12022
Araujo, R. J. (1980). Statistical mechanical model of boron coordination. J. Non Cryst. Solids 42, 209–230. doi: 10.1016/0022-3093(80)90023-X
Bauchy, M. (2019). Deciphering the atomic genome of glasses by topological constraint theory and molecular dynamics: a review. Comput. Mater. Sci. 159, 95–102. doi: 10.1016/j.commatsci.2018.12.004
Belova, E. V., Kolyagin, Y. A., and Uspenskaya, I. A. (2015). Structure and glass transition temperature of sodium-silicate glasses doped with iron. J. Non Cryst. Solids 423–424, 50–57. doi: 10.1016/j.jnoncrysol.2015.04.039
Bhasin, G., Bhatnagar, A., Bhowmik, S., Stehle, C., Affatigato, M., Feller, S., et al. (1998). Short range order in sodium borosilicate glasses obtained via deconvolution of 29Si MAS NMR spectra. Phys. Chem. Glass. 39, 269–274.
Bødker, M. S., Mauro, J. C., Goyal, S., Youngman, R. E., and Smedskjaer, M. M. (2018). Predicting Q-speciation in binary phosphate glasses using statistical mechanics. J. Phys. Chem. B 122, 7609–7615. doi: 10.1021/acs.jpcb.8b04604
Bødker, M. S., Mauro, J. C., Youngman, R. E., and Smedskjaer, M. M. (2019). Statistical mechanical modeling of borate glass structure and topology: prediction of superstructural units and glass transition temperature. J. Phys. Chem. B 123, 1206–1213. doi: 10.1021/acs.jpcb.8b11926
Bray, P. J. (1985). Structural models for borate glasses. J. Non Cryst. Solids 75, 29–36. doi: 10.1016/0022-3093(85)90198-X
Day, D. E., Tomsia, A. P., Jung, S. B., Sonny Bal, B., Fu, Q., Rahaman, M. N., et al. (2011). Bioactive glass in tissue engineering. Acta Biomater. 7, 2355–2373. doi: 10.1016/j.actbio.2011.03.016
Deng, L., and Du, J. (2018). Development of boron oxide potentials for computer simulations of multicomponent oxide glasses. J. Am. Ceram. Soc. 102, 2482–2505. doi: 10.1111/jace.16082
Du, J., and Cormack, A. N. (2004). The medium range structure of sodium silicate glasses: a molecular dynamics simulation. J. Non Cryst. Solids 349, 66–79. doi: 10.1016/j.jnoncrysol.2004.08.264
Eckert, H. (2018). Spying with spins on messy materials: 60 years of glass structure elucidation by NMR spectroscopy. Int. J. Appl. Glas. Sci. 9, 167–187. doi: 10.1111/ijag.12333
Feller, S. A., Dell, W. J., and Bray, P. J. (1982). 10B NMR studies of lithium borate glasses. J. Non Cryst. Solids 51, 21–30. doi: 10.1016/0022-3093(82)90186-7
Fischer, H. E., Barnes, A. C., and Salmon, P. S. (2006). Neutron and x-ray diffraction studies of liquids and glasses. Rep. Prog. Phys. 69, 233–299. doi: 10.1088/0034-4885/69/1/R05
Goyal, S., and Mauro, J. C. (2018). Statistical mechanical model of bonding in mixed modifier glasses. J. Am. Ceram. Soc. 101, 1906–1915. doi: 10.1111/jace.15364
Grandjean, A., Malki, M., Montouillout, V., Debruycker, F., and Massiot, D. (2008). Electrical conductivity and 11B NMR studies of sodium borosilicate glasses. J. Non Cryst. Solids 354, 1664–1670. doi: 10.1016/j.jnoncrysol.2007.10.007
Jones, E., Oliphant, T., and Peterson, P. (2001). SciPy: Open Source Scientific Tools for Python. Available online at: http://www.scipy.org/ (accessed May 15, 2019).
Kirchner, K. A., Kim, S. H., and Mauro, J. C. (2018). Statistical mechanics of topological fluctuations in glass-forming liquids. Phys. A Stat. Mech. Appl. 510, 787–801. doi: 10.1016/j.physa.2018.07.028
Kirchner, K. A., and Mauro, J. C. (2019). Statistical mechanical model of the self-organized intermediate phase in glass-forming systems with adaptable network topologies. Front. Mater. 6:11. doi: 10.3389/fmats.2019.00011
Lelong, G., Cormier, L., Hennet, L., Michel, F., Rueff, J. P., Ablett, J. M., et al. (2017). Lithium borate crystals and glasses: how similar are they? A non-resonant inelastic X-ray scattering study around the B and O K-edges. J. Non Cryst. Solids 472, 1–8. doi: 10.1016/j.jnoncrysol.2017.06.012
Lima, M. M., and Monteiro, R. (2001). Characterisation and thermal behaviour of a borosilicate glass. Thermochim. Acta 373, 69–74. doi: 10.1016/S0040-6031(01)00456-7
Liu, Z., Hu, Y., Li, X., Song, W., Goyal, S., Micoulaut, M., et al. (2018). Glass relaxation and hysteresis of the glass transition by molecular dynamics simulations. Phys. Rev. B 98:104205. doi: 10.1103/PhysRevB.98.104205
MacDonald, W. M., Anderson, A. C., and Schroeder, J. (1985). Low-temperature behavior of potassium and sodium silicate glasses. Phys. Rev. B 31, 1090–1101. doi: 10.1103/PhysRevB.31.1090
Maekawa, H., Maekawa, T., Kawamura, K., and Yokokawa, T. (1991). The structural groups of alkali silicate glasses determined from 29Si MAS-NMR. J. Non Cryst. Solids 127, 53–64. doi: 10.1016/0022-3093(91)90400-Z
Mahler, J., and Sebald, A. (1995). Deconvolution of 29Si magic-angle spinning nuclear magnetic resonance spectra of silicate glasses revisited - some critical comments. Solid State Nucl. Magn. Reson. 5, 63–78. doi: 10.1016/0926-2040(95)00027-N
Martens, R., and Müller-Warmuth, W. (2000). Structural groups and their mixing in borosilicate glasses of various compositions - an NMR study. J. Non Cryst. Solids 265, 167–175. doi: 10.1016/S0022-3093(99)00693-6
Massobrio, C., Du, J., Bernasconi, M., and Salmon, P. S. (2015). Molecular Dynamics Simulations of Disordered Materials. Cham: Springer International Publishing.
Mauro, J. C. (2013). Statistics of modifier distributions in mixed network glasses. J. Chem. Phys. 138:12A522. doi: 10.1063/1.4773356
Mauro, J. C. (2014). Grand challenges in glass science. Front. Mater. 1:20. doi: 10.3389/fmats.2014.00020
Mauro, J. C., and Smedskjaer, M. M. (2014). Statistical mechanics of glass. J. Non Cryst. Solids 396–397, 41–53. doi: 10.1016/j.jnoncrysol.2014.04.009
Mauro, J. C., Tandia, A., Vargheese, K. D., Mauro, Y. Z., and Smedskjaer, M. M. (2016). Accelerating the design of functional glasses through modeling. Chem. Mater. 28, 4267–4277. doi: 10.1021/acs.chemmater.6b01054
Micoulaut, M. (2016). Concepts and applications of rigidity in non-crystalline solids: a review on new developments and directions. Adv. Phys. X 6149, 1–29. doi: 10.1080/23746149.2016.1161498
Nanba, T., Nishimura, M., and Miura, Y. (2004). A theoretical interpretation of the chemical shift of 29Si NMR peaks in alkali borosilicate glasses. Geochim. Cosmochim. Acta 68, 5103–5111. doi: 10.1016/j.gca.2004.05.042
Naumis, G. G. (2005). Energy landscape and rigidity. Phys. Rev. E Stat. Nonlin. Soft Matter Phys. 71:026114. doi: 10.1103/PhysRevE.71.026114
Neuville, D. R., de Ligny, D., and Henderson, G. S. (2014). Advances in Raman spectroscopy applied to earth and material sciences. Rev. Mineral. Geochem. 78, 509–541. doi: 10.2138/rmg.2013.78.13
Ohtori, N., Takase, K., Akiyama, I., Suzuki, Y., Handa, K., Sakai, I., et al. (2001). Short-range structure of alkaline-earth borate glasses by pulsed neutron diffraction and molecular dynamics simulation. J. Non Cryst. Solids 293–295, 136–145. doi: 10.1016/S0022-3093(01)00662-7
Plimpton, S. (1995). Fast parallel algorithms for short-range molecular dynamics. J. Comput. Phys. 117, 1–19. doi: 10.1006/jcph.1995.1039
Plodinec, M. J. (2000). Borosilicate glasses for nuclear waste imobilisation. Glas. Technol. 41, 186–192.
Rahman, I. A., and Padavettan, V. (2012). Synthesis of silica nanoparticles by sol-gel: size-dependent properties, surface modification, and applications in silica-polymer nanocomposites—a review. J. Nanomater. 2012:132424. doi: 10.1155/2012/132424
Schroeder, J., Mohr, R., Macedo, P., and Montrose, C. (1973). Rayleigh and Brillouin scattering in K2O-SiO2 glasses. J. Am. Ceram. Soc. 10, 510–514. doi: 10.1111/j.1151-2916.1973.tb12399.x
Schuch, M., Trott, C., and Maass, P. (2011). Network forming units in alkali borate and borophosphate glasses and the mixed glass former effect. RSC Adv. 1, 1370–1382. doi: 10.1039/c1ra00583a
Shelby, J. E. (1983). Thermal expansion of alkali borate glasses. J. Am. Ceram. Soc. 66, 225–227. doi: 10.1111/j.1151-2916.1983.tb10023.x
Smedskjaer, M. M., Hermansen, C., and Youngman, R. E. (2017). Topological engineering of glasses using temperature-dependent constraints. MRS Bull. 42, 29–33. doi: 10.1557/mrs.2016.299
Smedskjaer, M. M., Mauro, J. C., Youngman, R. E., Hogue, C. L., Potuzak, M., and Yue, Y. (2011). Topological principles of borosilicate glass chemistry. J. Phys. Chem. B 115, 12930–12946. doi: 10.1021/jp208796b
Tomozawa, M., Hong, J. W., and Ryu, S. R. (2005). Infrared (IR) investigation of the structural changes of silica glasses with fictive temperature. J. Non Cryst. Solids 351, 1054–1060. doi: 10.1016/j.jnoncrysol.2005.01.017
Uhlmann, D. R., and Shaw, R. R. (1969). The thermal expansion of alkali borate glasses and the boric oxide anomaly. J. Non Cryst. Solids 1, 347–359. doi: 10.1016/0022-3093(69)90018-0
Wang, M., Anoop Krishnan, N. M., Wang, B., Smedskjaer, M. M., Mauro, J. C., and Bauchy, M. (2018). A new transferable interatomic potential for molecular dynamics simulations of borosilicate glasses. J. Non Cryst. Solids 498, 294–304. doi: 10.1016/j.jnoncrysol.2018.04.063
Xu, Q., Kawamura, K., and Yokokawa, T. (1988). Molecular dynamics calculations for boron oxide and sodium borate glasses. J. Non Cryst. Solids 104, 261–272. doi: 10.1016/0022-3093(88)90397-3
Yiannopoulos, Y. D., Chryssikos, G. D., and Kamitsos, E. I. (2001). Structure and properties of alkaline earth borate glasses. Phys. Chem. Glas. 42, 164–172.
Youngman, R. (2018). NMR spectroscopy in glass science: a review of the elements. Materials 11, E476. doi: 10.3390/ma11040476
Keywords: glass, borosilicates, modeling, statistical mechanics, structure
Citation: Bødker MS, Sørensen SS, Mauro JC and Smedskjaer MM (2019) Predicting Composition-Structure Relations in Alkali Borosilicate Glasses Using Statistical Mechanics. Front. Mater. 6:175. doi: 10.3389/fmats.2019.00175
Received: 22 May 2019; Accepted: 08 July 2019;
Published: 26 July 2019.
Edited by:
Matthieu Micoulaut, Sorbonne Universités, FranceReviewed by:
Jean-Marc Delaye, Commissariat à l'Energie Atomique et aux Energies Alternatives (CEA), FranceRoman Golovchak, Austin Peay State University, United States
Copyright © 2019 Bødker, Sørensen, Mauro and Smedskjaer. This is an open-access article distributed under the terms of the Creative Commons Attribution License (CC BY). The use, distribution or reproduction in other forums is permitted, provided the original author(s) and the copyright owner(s) are credited and that the original publication in this journal is cited, in accordance with accepted academic practice. No use, distribution or reproduction is permitted which does not comply with these terms.
*Correspondence: Morten M. Smedskjaer, bW9zQGJpby5hYXUuZGs=