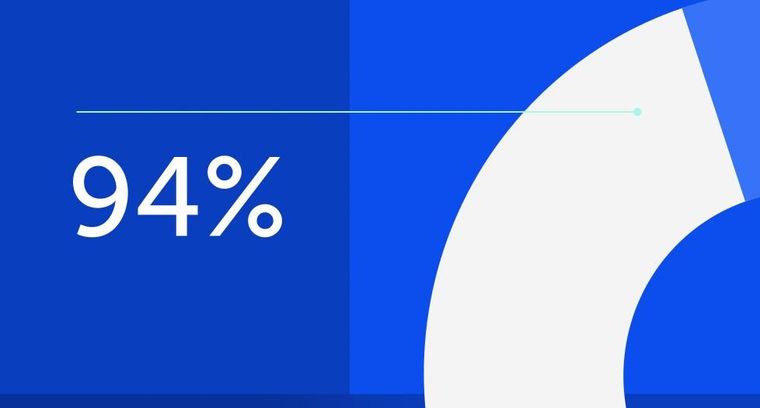
94% of researchers rate our articles as excellent or good
Learn more about the work of our research integrity team to safeguard the quality of each article we publish.
Find out more
EDITORIAL article
Front. Mar. Sci., 09 April 2025
Sec. Ocean Observation
Volume 12 - 2025 | https://doi.org/10.3389/fmars.2025.1588067
This article is part of the Research TopicDemonstrating Observation Impacts for the Ocean and Coupled PredictionView all 18 articles
Editorial on the Research Topic
Demonstrating observation impacts for the ocean and coupled prediction
Global ocean forecasting emerged as a true operational service under the decade-long project called the Global Ocean Data Assimilation Experiment (GODAE, Smith, 2000). Since the beginning of GODAE, ocean observations were recognised as the foundation of operational oceanography. Under GODAE, several groups developed capabilities in ocean data assimilation (Bell et al., 2009) and built operational ocean and seasonal forecast systems (e.g., Dombrowsky et al., 2009). GODAE also played a role in the establishment of important programs in ocean observing – including Argo (Roemmich and Gilson, 2009; Roemmich et al., 2019), which began as a joint project of CLIVAR and GODAE (Freeland et al., 2010); and GHRSST (Group for High-Resolution Sea Surface Temperature HRSST program, Donlon et al., 2009; Martin et al., 2012), which began as a GODAE pilot project. Following the success of GODAE, the ocean forecasting community continued to work together to improve ocean forecasting systems under GODAE OceanView (Bell et al., 2015), and now under OceanPredict (Davidson et al., 2019).
Research on optimal ocean observation strategies has a long history (e.g., Munk and Wunsch, 1982), with numerical assessment of the impact of observations on ocean forecasts beginning long before GODAE began (e.g., Miller, 1990). In 2007, at a GODAE workshop in Paris, a new GODAE task team was established - called the Observing System Evaluation task team (OSEval-TT, Oke et al., 2009a). The OSEval-TT continued under GODAE OceanView and OceanPredict, publishing several community papers that demonstrate the value of ocean observations for ocean forecasting (e.g., Oke et al., 2015a, b; Fujii et al., 2019; Martin et al., 2022) and seasonal prediction (e.g., Fujii et al., 2015b). Aside from those community papers, OSEval-TT members and groups applied ocean analysis, reanalysis, and forecast systems to quantify the impacts of ocean observations for various ocean services (e.g., Le Traon et al., 2019). Those studies included demonstrations of the complementarity of observations from different platforms (e.g., Oke and Schiller, 2007; Lea et al., 2014; Gasparin et al., 2023); the value of ocean observations in coastal regions (e.g., Oke et al., 2009b; Kerry et al., 2018); the foundational importance of satellite altimeter data (e.g., Remy et al., 2013; Verrier et al., 2017; Hamon et al., 2019; Benkiran et al., 2021); the impact of Argo data on data-assimilating systems (e.g., Balmaseda et al., 2007; Zhang et al., 2017); the usefulness of data from mooring arrays (e.g., Fujii et al., 2015a); and the impact of ocean observations for coupled prediction (e.g., Halliwell et al., 2017; King et al., 2020). These studies have provided evidence of the importance of ocean observations for ocean services that have helped argue the case for sustaining the global ocean observing system.
Under OceanPredict, when the United Nations Decade of the Ocean for sustainable development began, the OSEval-TT established Synergistic Observing Network for Ocean Prediction (SynObs). SynObs seeks to “extract maximum benefit from combining various observation platform measurements, typically satellite and in situ observation data, or combinations of coastal and open ocean platforms for ocean/coastal predictions”. The flagship activity of SynObs is the coordinated multi-system OSEs/OSSEs. This Research Topic of Frontiers in Marine Science is also a ‘contribution’ to SynObs. This Research Topic invited research papers - summarized below - that demonstrate observation impacts for the ocean and coupled prediction. The Research Topic includes 17 research articles, mostly using Observing System Experiments (OSEs) or Observing System Simulation Experiments (OSSEs), with contributions from 86 authors.
Paul et al. present a method that identifies which assimilated observations yield a beneficial contribution to an analysis field produced using a ensemble data assimilation system. Using a regional system applied to the Bay of Bengal, the authors demonstrate improvement by assimilating only those observations that are identified as “beneficial”, with about 50% of available observations improving the analysis. Interestingly, they show that assimilating too many observations can degrade performance. A similar result is reported by Lorenc and Marriott (2014) for numerical weather predictions.
Sugiura et al. propose improving ocean data assimilation by using integral quantities like heat and freshwater content derived from temperature and salinity profiles. Unlike traditional methods that are prone to noise and biases, this approach seems to offer a more robust constraint. The authors demonstrate that these integral measures are particularly beneficial in data-sparse regions.
Argo is the foundational ocean observing platform for subsurface properties in the deep-ocean (Johnson et al., 2022). But Argo doesn’t routinely observe the continental shelves. A new initiative, called the Fishing Vessel Ocean Observing Network (FVON, Van Vranken et al., 2023), offers a complimentary platform. In this Research Topic, Kerry et al. use a 4D-Var system in the Tasman Sea to show how fishing vessel-collected subsurface temperature data can improve shelf-sea forecasts. Similarly, Hirose et al. demonstrate how data from low-cost sensors on Japanese fishing vessels enhance coastal forecasts. Both studies highlight the value of integrating non-traditional platforms to fill observational gaps and improve coastal ocean forecasts.
Edwards et al. review how ocean observations improve circulation models in coastal and marginal seas, where data are sparse. They highlight challenges like representativeness errors in model-data comparisons and discuss tools such as OSSEs, array modes, and Artificial Intelligence algorithms. Examples from global coastal models show that integrating in-situ and subsurface observations reduces errors and enhances forecast accuracy.
Aydogdu et al. demonstrate that assimilation of glider observations consistently improves state estimates of the circulation in the Western Mediterranean Sea, particularly eddies. They also demonstrate improvements to upper-layer chlorophyll estimates in the biogeochemical system by improving physical mixing.
Several papers in this Research Topic demonstrate the impact of surface velocities on ocean forecasts. This includes a study by Mirouze et al. and Waters et al., using the United Kingdom (UK) and French ocean forecast system, that share the same ocean model, but uses a different approach to data assimilation. Both studies show improvements to forecast surface currents when assimilating total surface velocity particularly in the equatorial regions. Waters et al. also show good improvement in western boundary current regions and in the Antarctic Circumpolar Current, but with minimal impact on subsurface temperature and salinity. Mirouze et al. report some degradation in subsurface temperature and salinity outside of the tropics, due to over-fitting of surface velocity observations. In a separate paper, Waters et al. presents an inter-comparison of the two above-mentioned systems. They show that using equivalent OSSEs, errors in surface velocities are reduced more in the UK system, compared to the French, with improvements evident to greater depths.
In this Research Topic, Balmaseda et al. demonstrate that assimilation of sea-level, together with sub-surface temperature and salinity, significantly enhances the accuracy of seasonal predictions for key variables like sea surface temperature (SST), upper ocean heat content, and subsurface temperature distributions. The study also demonstrates that the inclusion of ocean observations leads to better initialization of coupled ocean-atmosphere models, yielding improved forecast accuracy in regions with strong climate variability, such as the tropical Pacific.
The impact of Argo observations on sub-seasonal predictions is demonstrated in this Research Topic by Balan-Salorjini et al. The authors also show how problems with coupled initialization degrade the potential value of Argo. Continued development of coupled data assimilation and coupled initialization is desperately needed.
Balmaseda et al. further demonstrate the significant and positive impact of observations from Argo floats, ship-based measurements, and moored buoys on the accuracy of seasonal forecasts. This study also shows how assimilation of sub-surface observations helps reduce initialization errors in the ocean state, thereby improving the predictability of key atmospheric and oceanic processes.
The paper by Smith et al., in this Research Topic, use a Canadian ocean/ice forecast system to show the positive impacts of assimilating Absolute Dynamic Topography (ADT). The greatest benefits appear to be under sea ice, with improved representation of Arctic surface circulation features like the Beaufort Gyre and Transpolar Drift.
Liu et al. assess the assimilation of synthetic and real SWOT data in a regional ocean-ice prediction system, showing it improves ocean prediction in regions with complex currents and bathymetry. SWOT assimilation enhances mesoscale features like eddies and boundary currents, with real observations improving sea surface height and aligning better with in situ and satellite data.
Rahman et al. evaluate SST estimates from various ocean reanalysis products in the North Indian Ocean. Products with better observational data and assimilation techniques offer more accurate SST, though discrepancies remain due to model resolution and sparse observations.
Ishikawa et al. examine how quality control (QC) of Argo data (Wong et al., 2022) affects global ocean data assimilation. Improved QC enhances data consistency, boosting reanalysis accuracy and model performance, which is vital for climate forecasting.
Finally, Fujii et al. review the SynObs project, part of the UN Ocean Decade, which evaluates and improves global ocean observing systems through OSEs and OSSEs. OSEs assess the impact of existing observations, while OSSEs explore potential future systems. Early results show that combining satellite and in-situ data significantly improves forecasts. The study highlights the need for ongoing evaluation to enhance ocean monitoring and ensure coordinated global strategies.
The contributions in this Research Topic fall into three categories, including the impacts of observation selection and processing, observations over continental shelves, and observations over the deep ocean. Using many different data-assimilating systems and many different approaches to evaluate the impact of observations on ocean analysis and forecasts and coupled forecasts. These studies span time scales from days to decades and spatial scales from sub-mesoscale eddies and regional models to the global ocean. While individual system studies show the value of observations in specific cases, coordinated efforts like SynObs highlight their broader importance. The flagship SynObs activity, with multi-system OSEs and OSSEs, reduces uncertainties and clearly demonstrates the essential role of ocean observations in operational oceanography and seasonal prediction. Without sustained observations, the quality and reliability of ocean analyses, forecasts, and even seasonal climate predictions would deteriorate. A lack of accurate, well-calibrated ocean observations would weaken the constraints on these systems, reducing their value for decision-making and scientific understanding.
PO: Conceptualization, Writing – original draft. YF: Conceptualization, Writing – review & editing. ER: Conceptualization, Writing – review & editing.
The authors of this paper sincerely thank the supporters of SynObs, and particularly the authors who contributed to this Research Topic.
The authors declare that the research was conducted in the absence of any commercial or financial relationships that could be construed as a potential conflict of interest.
The author(s) declare that no Generative AI was used in the creation of this manuscript.
All claims expressed in this article are solely those of the authors and do not necessarily represent those of their affiliated organizations, or those of the publisher, the editors and the reviewers. Any product that may be evaluated in this article, or claim that may be made by its manufacturer, is not guaranteed or endorsed by the publisher.
Balmaseda M., Anderson D., Vidard A. (2007). Impact of Argo on analyses of the global ocean. Geophysical Res. Lett. 34, L16605. doi: 10.1029/2007GL030452
Bell M. J., Lefebvre M., Le Traon P.-Y., Smith N., Wilmer-Becker K. (2009). GODAE: the global ocean data assimilation experiment. Oceanography 22, 14–21. doi: 10.5670/oceanog.2009.62
Bell M. J., Schiller A., Le Traon P.-Y., Smith N., Dombrowsky E., Wilmer-Becker K. (2015). An introduction to GODAE oceanView. J. Operational Oceanography 8, s2–s11. doi: 10.1080/1755876X.2015.1022041
Benkiran M., Ruggiero G., Greiner E., Le Traon P.-Y., Rémy E., Lellouche J. M., et al. (2021). Assessing the impact of the assimilation of SWOT observations in a global high-resolution analysis and forecasting system part 1: Methods. Front. Mar. Sci. 8, 691955. doi: 10.3389/fmars.2021.691955
Davidson F., Alvera-Azcarate A., Barth A., Brassington G. B., Chassignet E. P., Clementi E., et al. (2019). Synergies in operational oceanography: the intrinsic need for sustained ocean observations. Front. Mar. Sci. 6, 450. doi: 10.3389/fmars.2019.00450
Dombrowsky E., Bertino L., Brassington G. B., Chassignet E. P., Davidson F., Hurlburt H. E., et al. (2009). GODAE systems in operation. Oceanography 22, 80–95. doi: 10.5670/oceanog.2009.68
Donlon C. J., Casey K. S., Robinson I. S., Gentemann C. L., Reynolds R. W., Barton I., et al. (2009). The GODAE high-resolution sea surface temperature pilot project. Oceanography 22, 34–45. doi: 10.5670/oceanog.2009.64
Freeland H., Roemmich D., Garzoli S. L., Le Traon P.-Y., Ravichandran M., Riser S., et al. (2010). Argo - a decade of progress. Proc. OceanObs 9, 357–370. doi: 10.5270/OceanObs09
Fujii Y., Cummings J., Xue Y., Schiller A., Lee T., Balmaseda M. A., et al. (2015a). Evaluation of the Tropical Pacific Observing System from the ocean data assimilation perspective. Q. J. R. Meteorol. Soc. 141, 2481–2496. doi: 10.1002/qj.2015.141.issue-692
Fujii Y., Ogawa K., Brassington G. B., Ando K., Yasuda T., Kuragano T. (2015b). Evaluating the impacts of the tropical Pacific observing system on the ocean analysis fields in the global ocean data assimilation system for operational seasonal forecasts in JMA. J. Operational Oceanography 8, 25–39. doi: 10.1080/1755876X.2015.1014640
Fujii Y., Remy E., Zuo H., Oke P. R., Halliwell G. R., Gasparin F., et al. (2019). Observing system evaluation based on ocean data assimilation and prediction systems: on-going challenges and future vision for designing/supporting ocean observational networks. Front. Mar. Sci. 6, 417. doi: 10.3389/fmars.2019.00417
Gasparin F., Lellouche J.-M., Cravatte S. E., Ruggiero G., Rohith B., Le Traon P. Y., et al. (2023). On the control of spatial and temporal oceanic scales by existing and future observing systems: An observing system simulation experiment approach. Front. Mar. Sci. 10, 1021650. doi: 10.3389/fmars.2023.1021650
Halliwell G., Mehari M., Shay L., Kourafalou V., Kang H., Kim H.-S., et al. (2017). OSSE quantitative assessment of rapid-response prestorm ocean surveys to improve coupled tropical cyclone prediction. J. Geophysical Res.: Oceans 122, 5729–5748. doi: 10.1002/2017JC012760
Hamon M., Greiner E., Le Traon P.-Y., Remy E. (2019). Impact of multiple altimeter data and mean dynamic topography in a global analysis and forecasting system. J. Atmospheric Oceanic Technol. 36, 1255–1266. doi: 10.1175/JTECH-D-18-0236.1
Johnson G. C., Hosoda S., Jayne S. R., Oke P. R., Riser S. C., Roemmich D., et al. (2022). Argo – Two decades: Global oceanography, revolutionized. Annu. Rev. Mar. Sci. 14, 379–403. doi: 10.1146/annurev-marine-022521-102008
Kerry C., Roughan M., Powell B. (2018). Observation impact in a regional reanalysis of the East Australian Current System. J. Geophysical Res.: Oceans 123, 7511–7528. doi: 10.1029/2017JC013685
King R. R., Lea D. J., Martin M. J., Mirouze I., Heming J. (2020). The impact of Argo observations in a global weakly coupled ocean–atmosphere data assimilation and short-range prediction system. Q. J. R. Meteorol. Soc. 146, 401–414. doi: 10.1002/qj.v146.726
Lea D., Martin M., Oke P. (2014). Demonstrating the complementarity of observations in an operational ocean forecasting system. Q. J. R. Meteorol. Soc. 140, 2037–2049. doi: 10.1002/qj.2014.140.issue-683
Le Traon P. Y., Reppucci A., Alvarez Fanjul E., Aouf L., Behrens A., Belmonte M., et al. (2019). From observation to information and users: The copernicus marine service perspective. Front. Mar. Sci. 6, 234. doi: 10.3389/fmars.2019.00234
Lorenc A. C., Marriott R. T. (2014). Forecast sensitivity to observations in the Met Office global numerical weather prediction system. Q. J. R. Meteorol. Soc. 140, 209–224. doi: 10.1002/qj.v140.678
Martin M., Dash P., Ignatov A., Banzon V., Beggs H., Brasnett B., et al. (2012). Group for High Resolution Sea Surface temperature (GHRSST) analysis fields inter-comparisons. Part 1: A GHRSST multi-product ensemble (GMPE). Deep Sea Res. Part II: Topical Stud. Oceanography 77, 21–30. doi: 10.1016/j.dsr2.2012.04.013
Martin M., Remy E., Tranchant B., King R., Greiner E., Donlon C. (2022). Observation impact statement on satellite sea surface salinity data from two operational global ocean forecasting systems. J. Operational Oceanography 15, 87–103. doi: 10.1080/1755876X.2020.1771815
Miller R. N. (1990). Tropical data assimilation experiments with simulated data: The impact of the tropical ocean and global atmosphere thermal array for the ocean. J. Geophysical Res.: Oceans 95, 11461–11482. doi: 10.1029/JC095iC07p11461
Munk W. H., Wunsch C. I. (1982). Observing the ocean in the 1990s. Philos. Trans. R. Soc. London Ser. A. Math. Phys. Sci. 307, 439–464. doi: 10.1098/rsta.1982.0120
Oke P. R., Balmaseda M. A., Benkiran M., Cummings J. A., Dombrowsky E., Fujii Y., et al. (2009a). Observing system evaluations: using GODAE systems. Oceanography 22, 144–153. doi: 10.5670/oceanog.2009.72
Oke P., Larnicol G., Fujii Y., Smith G., Lea D., Guinehut S., et al. (2015a). Assessing the impact of observations on ocean forecasts and reanalyses: Part 1, global studies. J. Operational Oceanography 8, 49–62. doi: 10.1080/1755876X.2015.1022067
Oke P., Larnicol G., Jones E., Kourafalou V., Sperrevik A., Carse F., et al. (2015b). Assessing the impact of observations on ocean forecasts and reanalyses: Part 2, regional applications. J. Operational Oceanography 8, 63–79. doi: 10.1080/1755876X.2015.1022080
Oke P. R., Sakov P., Schulz E. (2009b). A comparison of shelf observation platforms for assimilation in an eddy-resolving ocean model. Dynamics Atmospheres Oceans 48, 121–142. doi: 10.1016/j.dynatmoce.2009.04.002
Oke P. R., Schiller A. (2007). Impact of Argo, SST, and altimeter data on an eddy-resolving ocean reanalysis. Geophysical Res. Lett. 34, 1–7. doi: 10.1029/2007GL031549
Remy E., Drevillon M., Desportes C. (2013). Impact of the loss/addition of satellite altimetry on Mercator ocean operational products. Mercator. Newslett. 47,20–23.
Roemmich D., Alford M. H., Claustre H., Johnson K. S., King B., Moum J., et al. (2019). On the future of argo: A global, full-depth, multi-disciplinary array. Front. Mar. Sci. 6, 439. doi: 10.3389/fmars.2019.00439
Roemmich D., Gilson J. (2009). The 2004-2008 mean and annual cycle of temperature, salinity, and steric height in the global ocean from the Argo Program. Prog. Oceanography 82,81–100. doi: 10.1016/j.pocean.2009.03.004
Smith N. (2000). The global ocean data assimilation experiment. Adv. Space Res. 25, 1089–1098. doi: 10.1016/S0273-1177(99)00868-6
Van Vranken C., Jakoboski J., Carroll J. W., Cusack C., Gorringe P., Hirose N., et al. (2023). Towards a global Fishing Vessel Ocean Observing Network (FVON): state of the art and future directions. Front. Mar. Sci. 10, 1176814. doi: 10.3389/fmars.2023.1176814
Verrier S., Le Traon P.-Y., Remy E. (2017). Assessing the impact of multiple altimeter missions and argo in a global eddy-permitting data assimilation system. Ocean Sci. 13, 1077–1092. doi: 10.5194/os-13-1077-2017
Wong A. P., Gilson J., Cabanes C. (2022). Argo salinity: bias and uncertainty evaluation. Earth System Sci. Data Discussions 2022, 1–21. doi: 10.5194/essd-2022-323
Keywords: ocean observation, OSE (observing system experiment), OSSE (observing system simulation experiment), data assimilation, observation impacts, OceanPredict, SynObs
Citation: Oke PR, Fujii Y and Remy E (2025) Editorial: Demonstrating observation impacts for the ocean and coupled prediction. Front. Mar. Sci. 12:1588067. doi: 10.3389/fmars.2025.1588067
Received: 05 March 2025; Accepted: 19 March 2025;
Published: 09 April 2025.
Edited and Reviewed by:
Johannes Karstensen, Helmholtz Association of German Research Centres (HZ), GermanyCopyright © 2025 Oke, Fujii and Remy. This is an open-access article distributed under the terms of the Creative Commons Attribution License (CC BY). The use, distribution or reproduction in other forums is permitted, provided the original author(s) and the copyright owner(s) are credited and that the original publication in this journal is cited, in accordance with accepted academic practice. No use, distribution or reproduction is permitted which does not comply with these terms.
*Correspondence: Peter R. Oke, cGV0ZXIub2tlQGNzaXJvLmF1
Disclaimer: All claims expressed in this article are solely those of the authors and do not necessarily represent those of their affiliated organizations, or those of the publisher, the editors and the reviewers. Any product that may be evaluated in this article or claim that may be made by its manufacturer is not guaranteed or endorsed by the publisher.
Research integrity at Frontiers
Learn more about the work of our research integrity team to safeguard the quality of each article we publish.