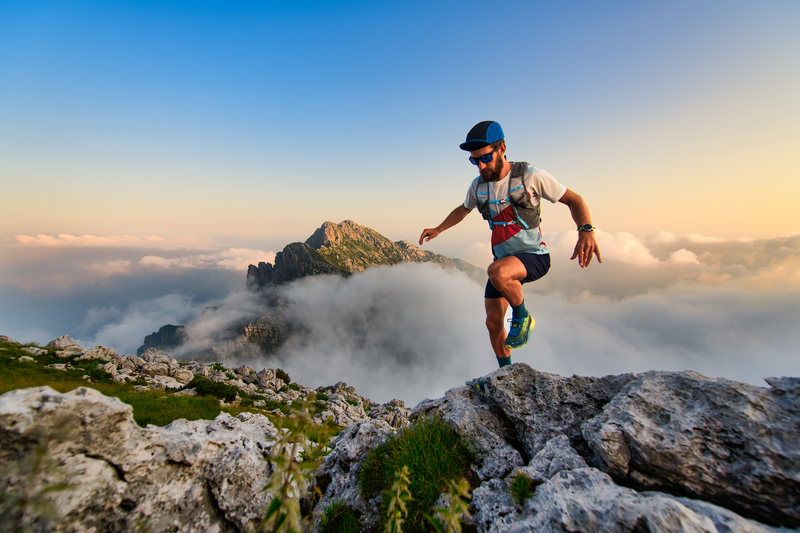
95% of researchers rate our articles as excellent or good
Learn more about the work of our research integrity team to safeguard the quality of each article we publish.
Find out more
ORIGINAL RESEARCH article
Front. Mar. Sci.
Sec. Ocean Observation
Volume 12 - 2025 | doi: 10.3389/fmars.2025.1576558
This article is part of the Research Topic Underwater Visual Signal Processing in the Data-Driven Era View all articles
The final, formatted version of the article will be published soon.
You have multiple emails registered with Frontiers:
Please enter your email address:
If you already have an account, please login
You don't have a Frontiers account ? You can register here
Nitrate is a critical limiting nutrient that significantly influences marine primary productivity and carbon sequestration. However, three-dimensional observation and reconstruction of oceanic nitrate remain constrained by the scarcity of in-situ data and limited spatial coverage. To address the challenge of limited observational labels hindering the development of global deep learning models for marine three-dimensional estimation, this study proposes a novel deep learning framework that utilizes underwater signals for label augmentation, thereby reducing the uncertainty in three-dimensional nitrate estimation. Initially, we employ a Bayesian neural network, utilizing multiple subsurface parameters from Biogeochemical-Argo (BGC-Argo) measurements to generate virtual nitrate labels with quantified uncertainty. These augmented labels are then assimilated into a U-Net-based model, greatly expanding the training dataset and further integrating sea surface environmental variables for comprehensive three-dimensional reconstruction. The proposed uncertainty-weighted loss function refines model training, balancing the quality and training impact of both observed and augmented labels. Quantitative evaluations using BGC-Argo and cruise measurement data demonstrate notable improvements in spatial and temporal generalization, with RMSE reductions of approximately 15% and 28%, respectively, particularly in under-sampled areas and complex upper ocean regions. This research framework offers a promising solution for oceanic three-dimensional data reconstruction in the absence of supervised data and has the potential to be coupled with various marine parameters and reconstruction models, providing deeper insights into the spatiotemporal variations of marine environments.
Keywords: Three-dimensional nitrate estimation, BGC-Argo, Mediterranean Sea, Bayesian neural network, Label augmentation, U-net
Received: 14 Feb 2025; Accepted: 17 Mar 2025.
Copyright: © 2025 Yu, Fan and Li. This is an open-access article distributed under the terms of the Creative Commons Attribution License (CC BY). The use, distribution or reproduction in other forums is permitted, provided the original author(s) or licensor are credited and that the original publication in this journal is cited, in accordance with accepted academic practice. No use, distribution or reproduction is permitted which does not comply with these terms.
* Correspondence:
Guodong Fan, Shandong Technology and Business University, Yantai, 264005, Shandong Province, China
Disclaimer: All claims expressed in this article are solely those of the authors and do not necessarily represent those of their affiliated organizations, or those of the publisher, the editors and the reviewers. Any product that may be evaluated in this article or claim that may be made by its manufacturer is not guaranteed or endorsed by the publisher.
Research integrity at Frontiers
Learn more about the work of our research integrity team to safeguard the quality of each article we publish.