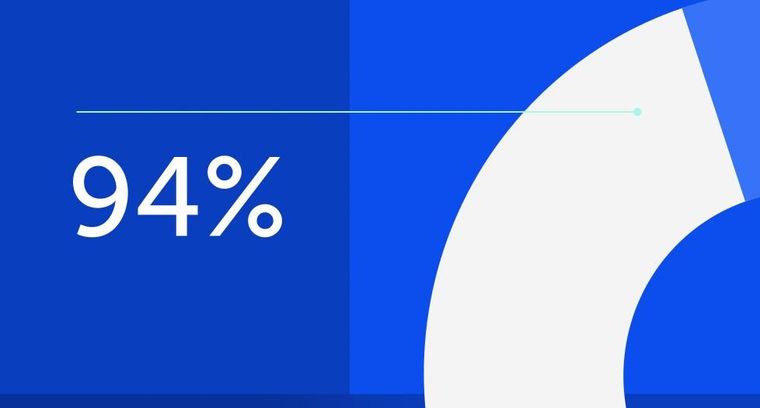
94% of researchers rate our articles as excellent or good
Learn more about the work of our research integrity team to safeguard the quality of each article we publish.
Find out more
ORIGINAL RESEARCH article
Front. Mar. Sci.
Sec. Ocean Observation
Volume 12 - 2025 | doi: 10.3389/fmars.2025.1560238
This article is part of the Research TopicRemote Sensing Applications in Oceanography with Deep LearningView all 12 articles
The final, formatted version of the article will be published soon.
Select one of your emails
You have multiple emails registered with Frontiers:
Notify me on publication
Please enter your email address:
If you already have an account, please login
You don't have a Frontiers account ? You can register here
Wave height prediction (WHP) is a crucial component of ocean observations. However, due to the strong spatiotemporal correlations and multi-source heterogeneity of ocean observing data, traditional models face inherent limitations in feature extraction and multidimensional information processing, making high-precision prediction a challenging task. To address this issue, this paper proposes a gated recurrent unit (GRU)-based model enhanced with an attention mechanism and multi-verse optimization (MVO), referred to as MVO-GRU-Att, to improve the accuracy and generalization capability of WHP. First, a spatiotemporal feature enhancement strategy is implemented by extracting representative spatiotemporal sequence samples from multi-site buoy sensor data, enabling the model to effectively capture the dynamic characteristics of ocean wave variations. Next, an attention-enhanced GRU optimization is introduced by integrating an attention mechanism (Att) into the GRU network, enhancing the model's ability to focus on critical features and improving its long-sequence modeling capabilities. Finally, hyperparameter optimization with MVO is applied, where the MVO algorithm is utilized to fine-tune GRU hyperparameters, thereby improving the model's generalization performance and adaptability to diverse ocean environments, ultimately enhancing prediction accuracy. To validate the effectiveness of the proposed model, we trained and evaluated it on data from multiple observation stations and conducted error analysis by comparing the predicted results with actual measurements. Experimental results demonstrate that, compared to five traditional methods, the MVO-GRU-Att model achieves a significant reduction in average error metrics (RMSE, MAE, MAPE) by 3.63%-38.01%, while increasing the similarity index R to 98.59%. These findings highlight the model's strong generalization ability across different marine environments and underscore its potential in advancing marine environmental monitoring and early warning systems, providing a more robust and adaptable approach for high-precision wave height prediction.
Keywords: Ocean observation, Wave height prediction, spatiotemporal sequence prediction, deep learning, Gated recurrent unit neural network
Received: 14 Jan 2025; Accepted: 18 Apr 2025.
Copyright: © 2025 Dong, Li, Zheng, Luo, Zhang and Yang. This is an open-access article distributed under the terms of the Creative Commons Attribution License (CC BY). The use, distribution or reproduction in other forums is permitted, provided the original author(s) or licensor are credited and that the original publication in this journal is cited, in accordance with accepted academic practice. No use, distribution or reproduction is permitted which does not comply with these terms.
* Correspondence: Xiaolong Yang, University of Science and Technology Beijing, Beijing, China
Disclaimer: All claims expressed in this article are solely those of the authors and do not necessarily represent those of their affiliated organizations, or those of the publisher, the editors and the reviewers. Any product that may be evaluated in this article or claim that may be made by its manufacturer is not guaranteed or endorsed by the publisher.
Supplementary Material
Research integrity at Frontiers
Learn more about the work of our research integrity team to safeguard the quality of each article we publish.