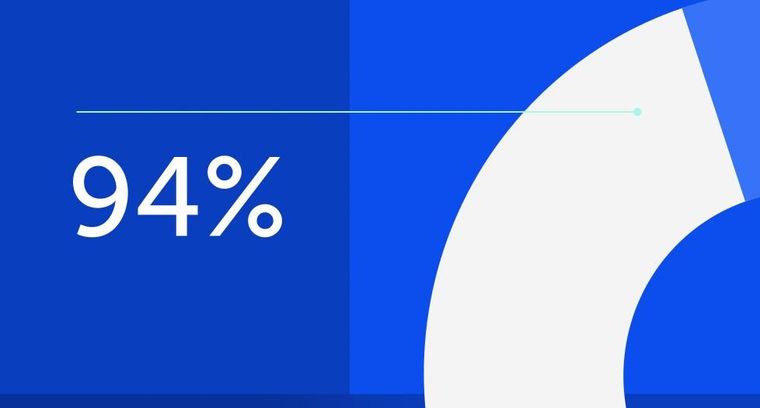
94% of researchers rate our articles as excellent or good
Learn more about the work of our research integrity team to safeguard the quality of each article we publish.
Find out more
ORIGINAL RESEARCH article
Front. Mar. Sci.
Sec. Ocean Observation
Volume 12 - 2025 | doi: 10.3389/fmars.2025.1555262
The final, formatted version of the article will be published soon.
Select one of your emails
You have multiple emails registered with Frontiers:
Notify me on publication
Please enter your email address:
If you already have an account, please login
You don't have a Frontiers account ? You can register here
Compared to structured ocean environments, unstructured ocean environments are inherently more complex. In such unstructured environments, the presence of narrow waterways poses unique navigational hurdles for autonomous surface vehicles (ASVs) due to their restricted connectivity. Current path planning algorithms designed for unstructured environments, particularly those characterized by narrow spaces, often face difficulties in efficiently exploring the target area while producing high-quality paths. In this study, we tackle the aforementioned complexities by incorporating progressive sampling and point cloud clustering, which jointly expedite the detection of constrained waterways in unstructured marine environments. More specifically, we generate multiple random trees from these sampling points, thereby bolstering both navigational accuracy and overall computational efficiency. Building upon these core techniques, we introduce a novel extension of the traditional rapidly-exploring random trees (RRT) connect algorithm-referred to as multiple RRT-connect (multi-RRT-connect)-aimed at swiftly determining a viable path between prescribed start and goal coordinates. As the number of samples expands, the random trees gradually enlarge and interlink, mirroring the functionality of classic RRT-connect and ultimately forming a continuous corridor. Subsequently, the derived path undergoes iterative refinement and optimization, culminating in a significantly reduced trajectory length.We subjected the proposed algorithm to rigorous testing through comprehensive simulations alongside meticulous comparisons with established state-of-the-art solutions. The results highlight the algorithm's distinct advantages across multiple dimensions such as path construction success, computational efficiency, and trajectory refinement quality, thereby underscoring its potential to advance autonomous navigation in challenging maritime settings.
Keywords: Autonomous surface vehicles, unstructured marine environments, constricted waterways, Rapidly exploring random tree, point cloud clustering
Received: 04 Jan 2025; Accepted: 09 Apr 2025.
Copyright: © 2025 Meng, Ku, Pu, Shi and Gong. This is an open-access article distributed under the terms of the Creative Commons Attribution License (CC BY). The use, distribution or reproduction in other forums is permitted, provided the original author(s) or licensor are credited and that the original publication in this journal is cited, in accordance with accepted academic practice. No use, distribution or reproduction is permitted which does not comply with these terms.
* Correspondence: Wenlong Meng, Harbin Institute of Technology, Weihai, Weihai, 264200, China
Disclaimer: All claims expressed in this article are solely those of the authors and do not necessarily represent those of their affiliated organizations, or those of the publisher, the editors and the reviewers. Any product that may be evaluated in this article or claim that may be made by its manufacturer is not guaranteed or endorsed by the publisher.
Supplementary Material
Research integrity at Frontiers
Learn more about the work of our research integrity team to safeguard the quality of each article we publish.