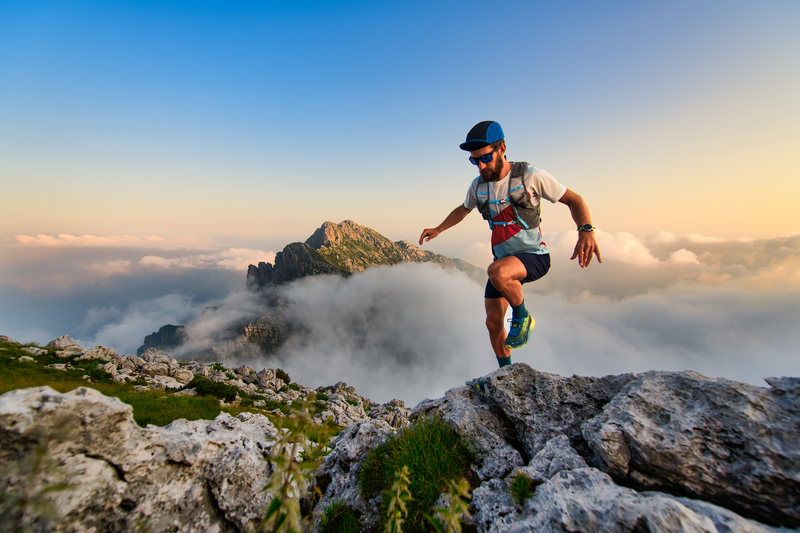
94% of researchers rate our articles as excellent or good
Learn more about the work of our research integrity team to safeguard the quality of each article we publish.
Find out more
ORIGINAL RESEARCH article
Front. Mar. Sci.
Sec. Marine Affairs and Policy
Volume 12 - 2025 | doi: 10.3389/fmars.2025.1547658
This article is part of the Research Topic Emerging Computational Intelligence Techniques to Address Challenges in Oceanic Computing View all 4 articles
The final, formatted version of the article will be published soon.
You have multiple emails registered with Frontiers:
Please enter your email address:
If you already have an account, please login
You don't have a Frontiers account ? You can register here
The Automatic Identification System (AIS) is one of the most important navigation assistance systems and plays a pivotal role in vessel monitoring. However, some fishing vessels disguise themselves as other vessel types during fishing bans to engage in illegal fishing activities, causing significant damage to marine ecosystem. To address this challenge and accurately identify vessel types, a BP-AdaBoost classification algorithm is developed by integrating backpropagation (BP) neural networks with ensemble learning techniques. The proposed algorithm leverages the AdaBoost method to combine multiple BP neural network weak classifiers into a strong classifier, effectively mitigating the slow convergence rate and susceptibility to local optima inherent in BP neural networks. By configuring the output nodes of the BP neural network to match the number of target classes, the AdaBoost algorithm achieves robust multi-class classification functionality. Historical AIS data are analyzed to extract static features, vessel behavior features, and temporal features for vessel classification. To minimize model overfitting, the Maximal Information Coefficient algorithm is employed to assess feature importance, and optimal feature combinations are determined through systematic feature selection experiments. Experiments are conducted using AIS data from the Pearl River Estuary in China, targeting the classification of cargo ships, fishing vessel, tanker, and passenger ships. The performance of the proposed method is compared with other machine learning algorithms. The results demonstrated classification accuracies of 90.8% for cargo ships, 95.6% for fishing vessels, 97.5% for tankers, and 98% for passenger ships, with an overall classification accuracy of 95%. Additionally, the BP-AdaBoost algorithm exhibited superior performance across other classification evaluation metrics. Specifically, the proposed algorithm outperformed the BP neural network by 4.5% and the support vector machine by 12.6% in overall classification accuracy. These findings indicate that the BP-AdaBoost algorithm is capable of effectively identifying vessel types based on historical trajectory data, providing a solid foundation for combating illegal fishing, detecting abnormal vessels, and identifying irregular vessel behaviors.
Keywords: AIS data, fishing vessel recognition, vessel classification, Trajectory feature, BP-Adaboost algorithm
Received: 18 Dec 2024; Accepted: 10 Feb 2025.
Copyright: © 2025 Han, Zhou, Weng, Chen and Liu. This is an open-access article distributed under the terms of the Creative Commons Attribution License (CC BY). The use, distribution or reproduction in other forums is permitted, provided the original author(s) or licensor are credited and that the original publication in this journal is cited, in accordance with accepted academic practice. No use, distribution or reproduction is permitted which does not comply with these terms.
* Correspondence:
Yang Zhou, Wuhan University of Technology, Wuhan, China
Disclaimer: All claims expressed in this article are solely those of the authors and do not necessarily represent those of their affiliated organizations, or those of the publisher, the editors and the reviewers. Any product that may be evaluated in this article or claim that may be made by its manufacturer is not guaranteed or endorsed by the publisher.
Research integrity at Frontiers
Learn more about the work of our research integrity team to safeguard the quality of each article we publish.