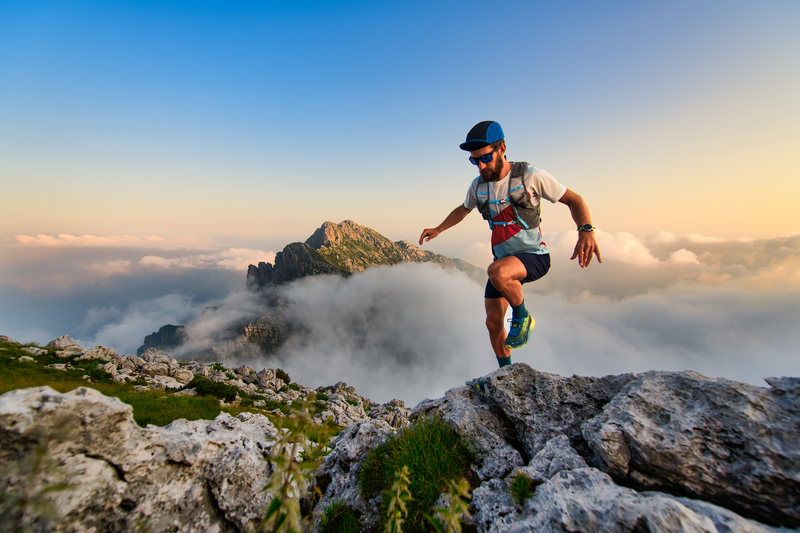
95% of researchers rate our articles as excellent or good
Learn more about the work of our research integrity team to safeguard the quality of each article we publish.
Find out more
ORIGINAL RESEARCH article
Front. Mar. Sci.
Sec. Ocean Observation
Volume 12 - 2025 | doi: 10.3389/fmars.2025.1539371
This article is part of the Research Topic Remote Sensing Applications in Oceanography with Deep Learning View all 10 articles
The final, formatted version of the article will be published soon.
You have multiple emails registered with Frontiers:
Please enter your email address:
If you already have an account, please login
You don't have a Frontiers account ? You can register here
Sonar image object detection plays a crucial role in obstacle detection, target recognition, and environmental perception for autonomous underwater vehicles (AUVs). However, the complex underwater acoustic environment introduces various interferences, such as noise, scattering, and echo, which hinder the effectiveness of existing object detection methods in achieving satisfactory accuracy and robustness. To address these challenges in forward-looking sonar (FLS) images, we propose a novel multi-level feature aggregation network (MLFANet). Specifically, to mitigate the impact of seabed reverberation noise, we design the low-level feature aggregation module (LFAM), which enhances key low-level image features, such as texture, edges, and contours in the object regions. Given the common presence of shadow interference in sonar images, we introduce the discriminative feature extraction module (DFEM) to suppress redundant features in the shadow regions and emphasize the object region features. To tackle the issue of object scale variation, we design the multi-scale feature refinement module (MFRM) to improve both classification accuracy and positional precision by refining the feature representations of objects at different scales. Additionally, the CIoU-DFL loss optimization function is constructed to address class imbalance in sonar data and reduce model computational complexity. Extensive experimental results demonstrate that our method outperforms state-of-the-art detectors on the Underwater Acoustic Target Detection (UATD) dataset. Specifically, our approach achieves the mean average precision (mAP) of 81.86%, improvement of 7.85% compared to the best-performing existing model. These results highlight the superior performance of our method in marine environments.
Keywords: Autonomous Underwater Vehicles, Forward-looking sonar, Marine object detection, feature extraction, Feature fusion
Received: 04 Dec 2024; Accepted: 18 Mar 2025.
Copyright: © 2025 Wang, Guo, Zhang and Zhang. This is an open-access article distributed under the terms of the Creative Commons Attribution License (CC BY). The use, distribution or reproduction in other forums is permitted, provided the original author(s) or licensor are credited and that the original publication in this journal is cited, in accordance with accepted academic practice. No use, distribution or reproduction is permitted which does not comply with these terms.
* Correspondence:
Shanwen Zhang, Department of Electronic Information, Xijing University, Xi'an, China
Disclaimer: All claims expressed in this article are solely those of the authors and do not necessarily represent those of their affiliated organizations, or those of the publisher, the editors and the reviewers. Any product that may be evaluated in this article or claim that may be made by its manufacturer is not guaranteed or endorsed by the publisher.
Research integrity at Frontiers
Learn more about the work of our research integrity team to safeguard the quality of each article we publish.