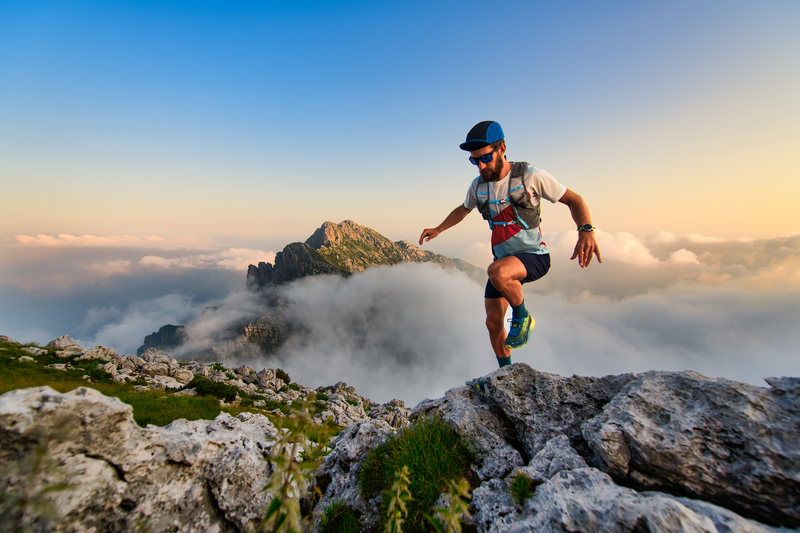
95% of researchers rate our articles as excellent or good
Learn more about the work of our research integrity team to safeguard the quality of each article we publish.
Find out more
ORIGINAL RESEARCH article
Front. Mar. Sci. , 13 February 2025
Sec. Coastal Ocean Processes
Volume 12 - 2025 | https://doi.org/10.3389/fmars.2025.1539210
This article is part of the Research Topic Climate Change and Human Impact: Assessing Vulnerability and Intensification of Hazards in Estuarine and Coastal Zones View all 11 articles
Forward-looking sonar object detection plays a vital role in marine applications such as underwater navigation, surveillance, and exploration, serving as an essential underwater acoustic detection method. However, the challenges posed by seabed reverberation noise, complex marine environments, and varying object scales significantly hinder accurate detection of diverse object categories. To overcome these challenges, we propose a novel semantic-spatial feature enhanced detection model, namely YOLO-SONAR, tailored for marine object detection in forwardlooking sonar imagery. Specifically, we introduce the competitive coordinate attention mechanism (CCAM) and the spatial group enhance attention mechanism (SGEAM), both integrated into the backbone network to effectively capture semantic and spatial features within sonar images, while feature fusion is employed to suppress complex marine background noise. To address the detection of small-scale marine objects, we develop a context feature extraction module (CFEM), which enhances feature representation for tiny object regions by integrating multi-scale contextual information. Furthermore, we adopt the Wise-IoUv3 loss function to mitigate the issue of class imbalance within marine sonar datasets and stabilize the model training process. Experimental evaluations conducted on real-world forward-looking sonar datasets, MDFLS and WHFLS, demonstrate that the proposed detection model outperforms other state-of-the-art methods, achieving an average precision (mAP) of 81.96% on MDFLS and 82.30% on WHFLS, which are improvements of 7.65% and 12.89%, respectively, over the best-performing existing methods. These findings highlight the potential of our approach to significantly advance marine object detection technologies, facilitating more efficient underwater exploration and monitoring.
With the rapid advancement of underwater intelligent detection technology, sonar-based object detection has become a pivotal tool in a wide range of marine applications, including underwater salvage (Neettiyath et al., 2024), shipwreck identification (Character et al., 2021), mine detection (Hożyń, 2021), and marine mapping (Fakiris et al., 2019). As a crucial marine detection technology, forward-looking sonar utilizes multiple beams to scan target areas, forming images through the reception of echo signals (Liu and Ye, 2023). Its ability to synthesize beams of different frequencies enables a wide detection range and fast imaging, which makes it ideal for real-time underwater exploration tasks (Kasetkasem et al., 2020). However, due to challenges like environmental noise, seabed reverberation, and equipment-related noise, the imaging resolution of forward-looking sonar is often compromised, and cluttered background information introduces significant complexity for object detection (Hurtos et al., 2015). As illustrated in Figure 1, the presence of complex noise and clutter makes it extremely difficult to accurately distinguish object categories using human vision alone.
Figure 1. Forward-looking sonar image with the interference of complex noise and clutter information.
In recent years, sonar image object detection has received increasing attention, leading to the development of various methods designed to handle challenging underwater acoustic environments. These methods can broadly be classified into traditional feature-based methods and deep learning (DL)-based methods. Traditional feature-based approaches rely on extracting contour, edge, and texture features from sonar images, and then applying a classifier to achieve object detection. For instance, Abu et al. (Abu and Diamant, 2019) used a weighted likelihood ratio to extract statistical features from sonar images, followed by support vector machine (SVM) classification for object recognition. Zhou et al. (Zhou et al., 2022) employed fuzzy C-means and K-means clustering to extract significant regional features, followed by nonlinear transformation and Fisher discrimination for object classification. Alaie et al. (Komari Alaie and Farsi, 2018) used maximum likelihood estimation to determine pixel distributions, followed by Bayesian classification to achieve recognition results. Other researchers, such as He et al. (He et al., 2023) and Zheng et al. (Zhang et al., 2023a), applied advanced filtering techniques like sparse matrix decomposition and non-local mean filtering to reduce noise and enhance features for object detection. Although these traditional methods offer certain advantages, their reliance on manually crafted features often limits their ability to generalize to diverse and complex underwater scenes.
With the emergence of convolutional neural networks (CNNs) in computer vision (Li et al., 2021), DL-based sonar object detection methods have been widely adopted, leveraging CNNs to learn deep, meaningful features directly from sonar images. DSA-Net (Li et al., 2024) uses feature pyramids and dual spatial attention mechanisms to enhance multi-scale feature extraction, effectively improving detection accuracy under complex conditions. To handle side-scan sonar images, MLFFNet (Wang et al., 2022b) uses feature extraction modules to identify critical features while mitigating seabed clutter through feature similarity. YOLOv3-DPFIN (Kong et al., 2019) employs a dual-path structure for feature extraction, enabling accurate multi-scale feature fusion and classification of sonar objects. AGFENet (Wang et al., 2021) leverages multi-scale convolution and channel attention to address the detection of tiny objects, while MBSNN (Wang et al., 2022a) uses dual attention mechanisms to enhance feature extraction and local-global modeling to improve accuracy. RMFENet (Zhao et al., 2023) integrates semantic and spatial features by a composite backbone model and utilizes a rotating IoU mechanism to optimize object localization.
Despite these advancements, the imaging characteristics of forward-looking sonar, such as low resolution, complex background noise, and the presence of small-sized objects, continue to pose challenges for accurate detection. To address these challenges, we propose a novel semantic-spatial feature enhanced detection model tailored for forward-looking sonar images, focusing specifically on marine environments. Our model, named YOLO-SONAR, is based on the high-performing YOLOv7 (Wang et al., 2023) architecture, but incorporates additional enhancements to better suit sonar imagery in complex underwater conditions. Firstly, to suppress the interference of seabed clutter information, the YOLO-SONAR detector uses the constructed competitive coordinate attention (CCAM) to obtain the valuable feature information of the target area and filter the redundant feature interference. Secondly, the spatial group enhance attention mechanism (SGEAM) is used to extract the semantic and spatial feature information contained in sonar image to improve the positioning accuracy for different object categories. Then, to solve the problem of tiny object detection in forward-looking sonar images, the context feature extraction module (CFEM) is used to mine the feature similarity and multi-scale feature information between different object categories to enhance the feature representation of tiny object regions. Finally, the Wise-IoUv3 is used as the optimal loss function of YOLO-SONAR detector to solve the class imbalance problem of forward-looking sonar data and stabilize the model training process. The main contributions of this article are as follows.
● We propose a novel object detection model, YOLO-SONAR, designed specifically for forward-looking sonar imagery in marine environments. This model can fully extract and enhance semantic and spatial features, thereby significantly improving detection accuracy.
● To suppress the interference from seabed reverberation noise and background clutter, we introduce CCAM and SGEAM to establish inter-feature correlations and filter redundant information. Additionally, CFEM is integrated to boost the model’s ability to detect tiny objects.
● The Wise-IoUv3 loss function is employed to address class imbalance and overfitting issues. Moreover, we introduce a new dataset, WHFLS, consisting of real-world forward-looking sonar images, to support future development in marine sonar detection.
The remainder of this article is organized as follows: Section 2 presents the overview of related works. Section 3 details the proposed method and its key components. Experimental and analysis are provided in Section 4, and Section 5 provides the discussion. Lastly, the conclusion is drawn in Section 6.
For deep learning-based sonar object detection, effectively extracting valuable features while filtering out redundant information during feature fusion is crucial for improving detection performance in complex underwater environments. Therefore, in this section, we review related works on feature extraction and feature fusion, focusing particularly on their impact on sonar image analysis.
Visual attention mechanisms (Guo et al., 2022) serve as core components in enhancing the feature extraction capabilities of CNNs models, allowing them to focus on valuable regions, improve edge and detail perception, and alleviate class imbalance issues. Visual attention has been widely applied across various computer vision tasks to improve model performance by capturing critical features. For instance, in order to capture the correlations between channel and spatial features, DANet (Fu et al., 2019) utilizes channel attention to strengthen local feature representation, while spatial attention is employed to establish contextual relationships. Deng et al. (Deng et al., 2021) proposed an attention-gated network, leveraging a dual-gating mechanism to focus on essential regions while suppressing background noise. To mitigate feature redundancy, Tao et al. (Tao et al., 2020) designed a multi-scale self-guided attention mechanism to filter redundant information through correlations between local and global features. Dai et al. (Dai et al., 2022) utilized the Transformer framework to capture fine-grained local and global feature information, significantly enhancing feature extraction. Cao et al. (Cao et al., 2022) introduced Swin-UNet, which learns both local and global feature information, employing a cross-scale feature fusion strategy to reduce semantic loss during feature transfer. MSFENet Shi et al. (2024) employs a dual attention mechanism, namely Squeeze-and-Excitation (SE) and Efficient Channel Attention (ECA), for the detection of underwater sonar objects. By integrating Swin-Transformer with the Convolutional Block Attention Module (CBAM), CBYOLO Wen et al. (2024) effectively captures the detailed information of sonar objects, thereby enhancing detection accuracy. Inspired by these advances in attention mechanisms and Transformer models, we introduce the Competitive Coordinate Attention Mechanism (CCAM) and Spatial Group Enhance Attention Mechanism (SGEAM) to our proposed model. These components help enhance feature representation while effectively suppressing redundant information, thereby improving the robustness of the sonar object detection in complex underwater environments.
To improve the robustness, confidence, and accuracy of object detection, context feature fusion strategies have been widely employed (Li et al., 2020). Context feature fusion integrates multi-scale features to strengthen contextual representation, enhance the accuracy of tiny object detection, and reduce semantic inconsistencies. In response to feature loss issues caused by multiple pooling operations, Chen et al. (Chen et al., 2021) proposed a bidirectional pyramid feature fusion strategy, which fuses high-resolution detail features with low-resolution structural features to achieve better feature complementation. Lv et al. (Lv et al., 2022) developed a multi-scale feature adaptive fusion approach to enhance the detection of small objects, particularly in sonar imagery where such objects are often present. Zhang et al. (Zhang et al., 2023b) introduced a global-local feature guidance module to effectively obtain both local and global information, using multi-scale fusion to hierarchically integrate features of varying sizes. In low-resolution scenarios, Chen et al. (Chen et al., 2023) used high-resolution feature fusion modules to mitigate the influence of background noise, thereby enhancing feature representation for object regions through contextual fusion. LGFENet Wang et al. (2024) enhances the model’s perception of tiny object regions by improving its ability to aggregate global contextual information, thereby increasing detection accuracy. However, existing feature fusion strategies struggle to adequately address the challenges associated with complex underwater environments, such as reverberation noise and cluttered backgrounds. These issues can lead to feature redundancy, reducing the overall effectiveness of the detection model. To overcome these limitations, we designed the Context Feature Extraction Module (CFEM), which fuses multi-scale features across different object categories, thereby improving the accuracy of tiny object detection in forward-looking sonar images.
This section first reviews the structure of YOLOv7, and then describes the proposed detector structure named YOLO-SONAR. As the object detection model with high detection accuracy, YOLOv7 (Wang et al., 2023) is composed of input layer, backbone network, Neck unit and Head structure. Specifically, the input layer scales the original image size to 640 × 640, the backbone network performs convolution operations to obtain feature information with different sizes, the Neck unit fuses multi-scale feature information, and the Head structure obtains the detection results by performing non-maximum suppression (NMS) operation on the predicted anchor box coordinates, categories scores, and confidence. The specific structure of YOLOv7 is shown in Figure 2, including CBS block composed of convolution combined with batch normalization (BN) and ReLU activation function, extended efficient layer aggregation network (E-ELAN), multipath convolution (MPConv) and SPPCSPC module constructed by spatial pyramid pooling (SPP) combined with contextual spatial pyramid convolution (CSPC). For CBS block, it uses convolution, normalization and activation functions to extract multi-scale feature maps, and transmits features with different sampling rates to the Neck unit by channel information fusion operations. The E-ELAN module consists of two different branches, one of which uses convolution operation to transform the number of channels, and the other uses convolution kernels with different sizes to obtain multi-scale feature information. The E-ELAM module enables the model to obtain rich feature information by controlling the gradient of different paths. The MPConv downsamples the feature map by max-pooling and convolution with the stride of 2, which can fuse the feature information of different paths and branches to obtain sematic information. The SPPCSP structure consists of SPP and CSPS, which firstly splits the feature into different branches, one of which uses the CBS block for deep feature extraction, and the other uses CBS combined with max-pooling to obtain multi-scale features, and then fuses the features of different branches by the concat function.
To improve the object detection accuracy of sonar image in complex underwater environment, based on YOLOv7 detector, we propose YOLO-SONAR detection model for forward-looking sonar image object detection. As shown in Figure 3, we first embed the constructed competitive coordinate attention mechanism (CCAM) and spatial group enhance attention mechanism (SGEAM) into the backbone network of the original YOLOv7 to enhance the feature extraction performance of the model, reduce the feature information loss and suppress the seabed reverberation noise interference in complex underwater environments. Then, the context feature extraction module (CFEM) is used to replace the E-ELAN structure of the YOLOv7 detector to improve the detection and poisoning accuracy of the object detector for tiny objects in forward-looking sonar image. Finally, Wise-IoUv3 is used as the loss function of YOLO-SONAR detector to solve the problem of unbalanced number of object categories in sonar images and stabilize the model training process.
Since the collection process of sonar image is affected by the seabed environment, there is reverberation noise interference in sonar image. To solve this problem, we propose a competitive coordinate attention mechanism (CCAM), which obtains valuable feature information and suppresses reverberation noise interference by performing the competition between semantic and spatial information. As shown in Figure 4, the proposed CCAM first decomposes the global pooling into max-pooling and average-pooling, which can reduce the feature map dimension and smooth the image to minimize noise interference. In the process of feature extraction, CCAM preprocesses the input features to generate global descriptors and . The specific calculation is as follows.
where represents the feature information of the feature mapping on position . To obtain the location information in the feature mapping, the average-pooling and max-pooling operations are used to aggregate the channel dimension features. Specifically, the semantic features with hight and width are calculated as follows.
The above tensors are input into the adaptive mechanism module to obtain tensors and with rich feature information.
where and are the adjustment coefficient in the range of . The tensors and are spliced, and then input into the conversion function composed of convolution for multi-scale feature fusion.
where represents the feature splicing operation, denotes the nonlinear activation function, and is the intermediate feature mapping. The and are decomposed into different tensors, which are applied to the excitation operations in the horizontal and vertical directions of the channel. Then, the combination of and is used as the joint input of the excitation operation, and the specific calculation is as follows.
where represents the activation function, and denotes the Sigmoid activation function. The feature and are scaled to obtain the weight information of feature and .
where denotes the element-wise multiplication, and . The overall calculation formula of the proposed CCAM is as follows.
where and represent the modeling of semantic features and spatial features on the channel dimension.
In the process of sonar image feature extraction, the problem of spatial feature information loss arises with the increase of convolution layer. However, for the sonar image object detection task, spatial features can provide valuable location information for the model to position the sonar image object region. To further enhance the representation of spatial feature information, we construct a spatial group enhance attention mechanism (SGEAM), and the specific structure is shown in Figure 5. The proposed SGEAM first groups the input features in the channel dimension to form multiple sub-feature maps. Then, the attention mechanism Guo et al. (2022) is guided by the similarity between the global features and local features contained in each group, and the spatial average function is used to approximate the global statistical features to the spatial vector obtained by group learning.
where , the correlation coefficient between the global feature and other features is calculated by dot product operation. The correlation coefficient measures the similarity between global feature and local feature . The feature information corresponding to each position in the feature map is calculated as follows.
To reduce the difference of correlation coefficient between different samples, the correlation coefficient of spatial dimension is normalized. The specific calculation is as follows.
where denotes the constant introduced to stabilize the numerical transformation. To ensure that the normalization operation used in the model can achieve the identity transformation, parameters and are introduced for each coefficient , and the specific calculation is as follows.
To obtain the enhance feature vector , the Sigmoid function is used to scale the original feature by the generated important coefficient on the spatial dimension.
Since SGE can enhance spatial and semantic dimension information in parallel, the calculation process is as follows.
The above enhanced features are calculated by convolution operation to obtain , where , and the feature fusion is carried out by Equation 24.
The tiny object feature information in sonar image mainly focuses on the shallow features with rich location and detail information, and the semantic information in the deep features plays an important role in improving the detection accuracy for tiny object categories. To solve the problem of poor detection effect caused by low-level feature loss of tiny objects in the feature extraction process, we construct a context feature extraction module (CFEM) to integrate the context information of the shallow feature into the deep feature information to improve the model detection accuracy for tiny object regions. As shown in Figure 6 the proposed CFEM first uses atrous convolution with different atrous coefficients to expand the receptive filed of the input feature map X, learns the local context information of the tiny object, and obtains features X1, X2 and X3, respectively. The specific calculation is as follows.
where dconv (·) denotes atrous convolution, conv (·) represents the standard convolution used to reduce the number of channels, and the feature map is spliced to obtain the fusion feature map F. The channel attention mechanism (Wang et al., 2021) is used to adjust the channel weight, and the pooling operation is used to compress the dimension of the feature map. The channel attention weight is obtained by multi-layer perceptron (MLP) and normalization function of pooling feature, and it is splited into weights α, β and γ corresponding to features X1, X2 and X3, which are calculated as follows.
where denotes the chunk function used to split the attention weight, and S (·) represents the Sigmoid function to obtain the normalized weight; denotes the multi-layer perceptron function composed of convolution, pooling and activation functions; Pa (·) and Pm(·) denote average-pooling and max-pooling operations respectively, which are used to compress the H × W × C; feature map to obtain the feature vector with 1 × 1 × C; size. By multiplying the obtained weights with the input features, the importance of different input features is adjusted to enhance the representation of valuable features and suppress noise interference. The feature Y of fusing context information is calculated as follows.
The input features and Y with inconsistent semantic information are used to obtain the dynamic fusion weights δ and (1 − δ) of the input feature map by the attention feature fusion, and then the weight is multiplied with the input feature to obtain the fusion feature Z. The noise interference in sonar image can be suppressed by the feature fusion operation. The specific calculation of the fusion feature Z is as follows.
where represents the attention weight matrix obtained using the convolution block attention mechanism (CBAM). For the specific calculation of CBAM, it first obtains the weight ωc by the channel attention mechanism, and then obtains the attention weight ωs by the spatial attention mechanism (Fu et al., 2019). To further enrich the semantic information of the fusion feature, the obtained weight ωs is multiplied by the fusion feature Z, and then the attention weight Zo = S (Z × ωs) is obtained by using the normalization function.
For the object detection task, the setting of loss function directly affects the accuracy and confidence of object detection results. The function of the loss function is to optimize the position error between the detected object and true object, and generate a prediction result that fits the ground truth bounding box. However, severe interference from reverberant noise on the seafloor results in poor resolution and severe category imbalance in the sonar images. To solve this problem, we use Wise-IoUv3 Tong et al. (2023) as the loss function of YOLO-SONAR to balance the influence of different resolution images on the model training results. The Wise-IoU introduces category weights into the initial IoU, that is, assigns weight information to each object category, and then weights in the calculation process of different categories of IoU to obtain more accurate detection results. The calculation details of Wise-IoU are shown in Figure 7, which has developed different improved versions (Wise-IoUv1, Wise-IoUv2, Wise-IoUv3). Specifically, The Wise-IoUv1 is a two-stage attention mechanism based on distance metric, which solves the negative impact of low-quality data on the model training process. The specific calculation process is as follows.
where the use of ∗ can separate Wg and Hg from the calculation graph, which can effectively improve the convergence efficiency, and reduce the attention to the center point distance under the condition of fitting the predicted bounding box with the ground truth bounding box to enhance the model generalization performance. The Wise-IoUv2 refers to the design principle of focal loss, and constructs a monotonic focusing coefficient r (r > 0) based on Wise-IoUv1, which effectively solves the problem of data imbalance, so that the model can focus on difficult samples and improve the detection performance. The specific calculation is as follows.
On the basis of Wise-IoUv1, the Wise-IoUv3 constructs a non-monotone focusing coefficient r by outlier degree. The specific calculation is as follows.
where β denotes the outlier degree used to characterize the quality of the regression box; represents the sliding average with momentum m, and its dynamic update enables β to obtain the optimal value, which can effectively solve the problem of slow training convergence; α and δ are hyperparameters, when the outlier degree of the regression box satisfies β = C, the regression box can obtain the optimal gradient gain. The Wise-IoUv3 can focus on the anchor box with poor quality and improve the positioning accuracy of the model to the object region.
In this section, we first introduce the forward-looking sonar image object detection datasets, and specific experimental details. Then, the proposed method is compared with the existing state-of-the-art detection method to verify the effectiveness and feasibility of the YOLO-SONAR detector.
The MDFLS dataset is a publicly available ocean sonar object detection dataset (Singh and Valdenegro-Toro, 2021). This dataset uses ARIS Explorer 3000 as the forward-looking sonar image collect device, which contains 1,868 original sonar images with 320 × 648 resolution and eleven object categories (bottle, can, chain, drink-carton, hook, propeller, shampoo-bottle, standing-bottle, tire, valve and wall). Some samples are shown in Figures 8A, B, it can be seen that it is difficult for the human-eye to directly recognize the specific object category in sonar image due to the interference of resolution and seabed reverberation noise. Since the difference in object scale, some categories only occupy fewer pixels. The statistical information in Figure 8C explains the object categories unbalanced distribution in the dataset. In the experiment, we divide the dataset into training set, test set and verification set in a ratio of 7:2:1.
To further verify the robustness of the YOLO-SONAR detector, we construct a forward-looking sonar image dataset WHFLS. This dataset uses BlueView M900 as the acquisition equipment to obtain sonar images in real ocean scenes. The WHFLS dataset contains 3,752 original sonar images with resolution of 1024 × 646 and three object categories (victim, boat and plane). Some samples are shown in Figure 9 it can be observed that although the object size in the dataset is large, the resolution of the object region is extremely poor due to the influence of the ocean environment. In addition, there is serious ocean clutter interference in the image, which brings great challenges to the object detection task. We randomly selected 2,625 images from the dataset as the training set, 750 images as the test set, and 377 images as the verification set.
The proposed YOLO-SONAR detector is implemented on the Nvidia RTX 3090 GPU with 24 GB memory using Pytorch 2.1.0 and MMdetection 3.1.0. All experiments are performed on workstations equipped with Intel i9-12900T CPU, 64GB RAM, and Ubuntu 18.04 operating system. The training epoch of the model is set as 24, and the batch size is set as 8. In the training optimization process, we set the initial learning rate as 0.001, and use the stochastic gradient descent (SGD) (Amari, 1993) with momentum of 0.9 as the optimizer. To quantitatively evaluate the performance of YOLO-SONAR detector, precision, recall, mAP, F1_score, FPS and parameters, which are widely used in object detection tasks, are used as metrics.
To illustrate the effectiveness and advantages of the proposed method, we compare it with other object detection methods such as Faster R-CNN (Ren et al., 2016), CenterNet (Duan et al., 2019), RetinaNet (Ross and Dollár, 2017), Cascade R-CNN (Cai and Vasconcelos, 2018), Sparse-RCNN (Sun et al., 2021), VarifocalNet (Zhang et al., 2021), EfficientDet (Tan et al., 2020), YOLOv8 Ultralytics (2023), YOLOv10 Ultralytics (2024), ViTDet Li et al. (2022) and CO-DETR Zong et al. (2023) on the MDLFS dataset. The quantitative evaluation results are shown in Table 1, from which it can be observed that the YOLO-SONAR detector obtains the optimal sonar object detection result compared to other methods, and its mAP reaches 81.96%. The reason is that YOLO-SONAR fuses semantic features and spatial features, fully exploits the valuable feature information contained in the forward-looking sonar image, and filters the interference of background noise and clutter information. For Faster R-CNN with poor detection results, its mAP is only 62.30% because it cannot effectively obtain the detailed features and context information of the object region. Benefiting from the multi-scale feature extraction structure and powerful global context modeling ability, EfficientDet obtains the better detection results for tiny object category. While YOLOv8 and YOLOv10 show competitive performance with mAPs of 75.30% and 76.55%, respectively, they fall short of YOLO-SONAR, particularly in detecting irregular objects. Similarly, ViTDet achieves a mAP of 74.59%, performing well in some categories but struggling with complex scene objects. CO-DETR, with a mAP of 75.69%, is competitive with YOLOv8 and YOLOv10 but still lags behind YOLO-SONAR, especially in detecting tiny objects (e.g., Shampoo-Bottle: 57.89% vs. 68.26% for YOLO-SONAR). Figure 10 shows the visual detection results of sonar images by different object detection methods. The proposed YOLO-SONAR can accurately detect and locate different object categories and obtain the highest confidence. For the different object detection models compared, it cannot obtain satisfactory detection results and suffers from the problems of missing detection and false alarms. Moreover, the PR curve of Figure 11A shows that YOLO-SONAR has a significant improvement in precision and recall compared with other methods, which further demonstrates the effectiveness and advantages of the proposed method.
Table 1. Comparisons with other methods on MDFLS dataset in the precision (%) of different sonar objects and mAP (%), where the bold font is the highest score.
To further verify the robustness and feasibility of the YOLO-SONAR detector, we compared it with different object detection models on the WHFLS dataset. The quantitative evaluation results of different methods are shown in Table 2. Since the dataset contains three different types of objects, we use mAP(S), mAP(M) and mAP(L) to measure the detection accuracy of object detection model for small, medium and large size objects. In addition, FPS and Parameters are used to compare the algorithm complexity of different object detection models. It can be seen from Table 2 that the YOLO-SONAR detector till obtains the optimal detection results, and its mAP value reaches 82.30%. Since the WHFLS dataset is interfered by more serious seabed clutter information, the compared object detection models cannot obtain better object detection accuracy. For example, RetinaNet only obtains 32.16% detection precision for the victim category with small object size. The reason is that the model fails to extract the valuable feature information of small-size object region and is seriously disturbed by the background information. For Varifocalnet, which obtains the second-best result, it can better solve the problem of object scale transformation due to the use of multi-scale deformation convolution structure. While YOLOv8 and YOLOv10 achieve competitive mAPs of 71.27% and 72.22%, respectively, they fall short of YOLO-SONAR, particularly in detecting small objects. Similarly, ViTDet achieves a mAP of 70.45%, performing well in some categories but struggling with small objects. CO-DETR, with a mAP of 71.35%, is competitive with YOLOv8 and YOLOv10 but still lags behind YOLO-SONAR, especially in detecting small objects (e.g., Victim: 60.34% vs. 72.96% for YOLO-SONAR). In addition, we analyze the computational complexity of different object detection models. Table 2 shows that the proposed method has obvious advantages, and its FPS and parameters are the best of all the compared methods. YOLO-SONAR achieves a competitive FPS of 33.6 with 45.68M parameters, making it suitable for real-time applications. The visualization of Figure 12 show that YOLO-SONAR can correctly detect and locate the objects in sonar images, and the compared models have the problems of missing detection and false alarms. The PR curves of different object detection methods on the WHFLS are shown in Figure 11B, the proposed method achieves a win–win situation between precision and recall, which demonstrates the robustness and feasibility of the YOLO-SONAR detector.
Table 2. Comparisons with other methods on WHFLS dataset in the precision (%), mAP(S)(%), mAP(M)(%), mAP(L)(%), mAP (%), F1_score (%), FPS and Parameters (M), where the bold font is the highest score.
Due to the complex nature of the underwater environment and acoustic channels, as well as the attenuation, reverberation, scattering, multipath effects, and side-lobe interference experienced by sound waves during propagation, sonar images often contain substantial noise. To assess the robustness of YOLO-SONAR against underwater noise interference, Gaussian noise, Poisson noise, and Multiplicative noise are added to the original sonar images, each with a signal-to-noise ratio (SNR) of 45 dB. The results in Table 3 demonstrate the noise robustness of the YOLO-SONAR model under Gaussian, Poisson, and Multiplicative noise conditions at an SNR of 45 dB, compared to a no-noise baseline. The model maintains relatively high performance across all noise types, with Precision, Recall, F1_score, Average IoU, AP, and mAP metrics showing only moderate degradation compared to the baseline. The most significant performance drop occurs under Multiplicative noise, indicating it poses a greater challenge. Despite this, YOLO-SONAR’s ability to sustain high detection accuracy and reliability in noisy environments underscores its robustness and suitability for real-world applications where noise is prevalent. Future work could focus on further enhancing its resilience, particularly to Multiplicative noise, to narrow the performance gap with the no-noise scenario. The experimental results presented in Figure 13 provide a qualitative assessment of YOLO-SONAR’s detection performance under various types of noise interference. Each subfigure visually demonstrates the model’s ability to detect objects under different noise conditions, offering insights into its robustness and reliability in challenging environments. Overall, these visualizations underscore YOLOSONAR’s robustness in maintaining detection accuracy across different noise conditions. The model’s consistent performance in the presence of Gaussian, Poisson, and multiplicative noise highlights its potential for practical applications in environments where noise interference is inevitable. These results complement the quantitative metrics provided in Table 3, offering a comprehensive evaluation of YOLO-SONAR’s effectiveness in real-world scenarios.
Figure 13. Visualization of YOLO-SONAR’s detection results under different categories of noise interference. (A) Original Images. (B) Gaussian Noise. (C) Poisson Noise. (D) Multiplicative Noise.
The proposed YOLO-SOLO detector includes core components CCAM, SGEAM and CFEM. To demonstrate the effectiveness of these components, we perform ablation experiments on the sonar object detection dataset MDFLS. In the experiments, we use the original YOLOv7 detector as baseline model and use the mAP, mAP(S), mAP(M), mAP(L) and F1_score as evaluation metrics. Figure 14 shows the visualization detection results of the baseline model YOLOv7 combined with different components, and Table 4 shows the quantitative evaluation results of the ablation experiment. Moreover, Figure 15 shows the visualization comparison of the feature extraction process for different components.
Figure 14. The visualization detection results in ablation experiments of the proposed method on MDFLS dataset. (A) YOLOv7. (B) YOLOv7+CCAM. (C) YOLOv7+CCAM+SGEAM. (D) YOLOv7+CCAM+SGEAM+CFEM.
Table 4. Quantitative evaluation results of ablation experiments on the MDFLS dataset in the mAP(S)(%), mAP(M)(%), mAP(L)(%), mAP (%) and F1_score (%), where the bold font is the highest score.
Figure 15. The visualization comparison of feature maps for different modules. (A) YOLOv7. (B) YOLOv7+CCAM. (C) YOLOv7+CCAM+SGEAM. (D) YOLOv7+CCAM+SGEAM+CFEM.
To illustrate the effectiveness of proposed CCAM, it is combined with the baseline model to demonstrate the improvement of sonar object detection accuracy. It can be seen from Table 3 that compared with the baseline model, YOLOv7 combined with CCAM is superior to the baseline on different evaluation metrics. Specifically, the mAP is increased from 43.12% to 51.65%, and the F1_score is increased from 34.57% to 43.26%, which further explains the improvement of CCAM for sonar object detection performance. The visualization detection results in Figure 14 shows that the use of CCAM can enhance the positioning precision of the baseline model to the object region and improve the confidence score of the object category recognition. The visualization results in Figure 15 show that CCAM enables the model to focus on feature extraction in the object region to suppress noise interference.
Compared with the existing attention mechanism, SGEAM uses the form of group feature reconstruction to enhance the spatial feature information to alleviate the loss of spatial information in feature extraction process. To verify the performance improvement of the detector using SGEAM, we combine the baseline with CCAM and SGEAM to detect sonar objects. It can be seen from Table 4 that the baseline combined with CCAM and SGEAM significantly improves the detection accuracy of the original YOLOv7 for sonar objects. For example, mAP increases from 51.65% to 63.43%, and F1_score increases from 43.26% to 56.85%. In addition, the combination of SGEAM improves the detection accuracy for tiny objects, with mAP(s) increasing from 25.36% to 42.58%. It can be seen from Figure 14 that the introduction of SGEAM improves the positioning and recognition accuracy for different object categories. The results in Figure 15 show that the use of SGEAM can effectively suppress the influence of clutter information on the feature extraction process.
The function of CFEM is to obtain the multi-scale feature information contained in sonar image and perform feature fusion to improve the detection accuracy for tiny objects. To verify the effectiveness of CFEM in improving the performance of object detection, we combine the baseline detector with CCAM, SGEAM and CFEM to achieve sonar object detection. It can be seen from Table 4 that the combination of different components significantly improves the detection accuracy of the baseline model. For example, for sonar object categories of different sizes, mAP(s) increases from 25.36 to 72.86%, mAP(M) increases from 39.85% to 82.36%, and mAP(L) increases from 64.17% to 91.23%. The sonar object detection results in Figure 14 and the feature extraction visualization information in Figure 15 show that the introduction of CFEM effectively improves the positioning and recognition accuracy of the detector for tiny object categories, and significantly enhances the feature representation of different object regions.
The MDFLS and WHFLS datasets exhibit inherent biases that may impact the generalizability and performance of YOLO-SONAR. First, the resolution bias between the datasets (320times648 for MDFLS vs. 1024times646 for WHFLS) could affect the model’s ability to generalize across different image qualities, particularly in detecting small objects where lower resolution may result in less detailed representations. Second, the object type bias, with MDFLS containing 11 diverse categories (e.g., bottle, can, chain) and WHFLS focusing on only 3 (victim, boat, plane), may limit the model’s adaptability to datasets with a broader range of object categories. Third, the environmental bias, arising from the datasets being collected in specific marine conditions, may not fully capture the diversity of underwater environments (e.g., varying water clarity, seabed types, and lighting conditions), potentially affecting performance in real-world scenarios. Finally, the scale bias, characterized by an uneven distribution of small, medium, and large objects across the datasets, could skew the model’s performance toward detecting larger objects, as seen in WHFLS, where boats and planes dominate. These highlight the need for more diverse and representative datasets to improve the model’s robustness and generalizability in complex marine environments.
While YOLO-SONAR demonstrates superior performance in marine object detection, it is not without limitations. First, the model’s computational complexity, although balanced for real-time applications, may pose challenges for deployment on resource-constrained platforms such as underwater drones. Second, the model’s scalability to larger and more diverse datasets remains to be evaluated, as its performance has primarily been tested on the MDFLS and WHFLS datasets. Third, YOLO-SONAR is specifically designed for forward-looking sonar images, and its applicability to other imaging modalities, such as side-scan sonar or optical underwater imaging, requires further investigation. Finally, the model’s reliance on annotated data for training highlights the need for more efficient data annotation methods or alternative learning paradigms, such as semi-supervised or unsupervised learning.
In this paper, we propose YOLO-SONAR, a novel object detection model specifically designed for forward-looking sonar images in complex marine environments. YOLO-SONAR incorporates three key components: competitive coordinate attention mechanism (CCAM), spatial group enhance attention mechanism (SGEAM), and context feature extraction module (CFEM). These components improve feature extraction, enhance object region representation, and boost the accuracy of detecting tiny objects. Experimental results on real-world sonar datasets, MDFLS and WHFLS, show that YOLO-SONAR outperforms existing methods, achieving the average precision (mAP) of 81.96% on MDFLS and 82.30% on WHFLS, with improvements of 7.65% and 12.89%, respectively. Ablation studies further confirm the effectiveness of each component. This demonstrates that YOLO-SONAR is a robust and efficient solution for marine object detection. Future work will explore the integration of unsupervised or semi-supervised learning techniques to further enhance the model’s generalization capability and adaptability in diverse marine environments.
The raw data supporting the conclusions of this article will be made available by the authors, without undue reservation.
ZW: Writing – original draft, Writing – review & editing. JG: Writing – review & editing. SZ: Writing – review & editing. NX: Writing – review & editing.
The author(s) declare that financial support was received for theresearch, authorship, and/or publication of this article. This work is supported by the National Natural Science Foundation of China(61671465),the National Natural Science Foundation of China(61624931),the Neural Science Foundation of Shaanxi Province(2021JM-537),the Youth Talent Support Program of Shaanxi Science and Technology Association (23]K0701), the Xi'an Science and Technology Planning Projects (20240103), the China Postdoctoral Science Foundation under Grant (2024M754225), the Natural Science Foundation of Jiangsu Province (BK20230441), and the Key Laboratory of Land Satellite Remote Sensing Application, Ministry of Natural Resources of the Peopled's Republic of China (KLSMNR-K202309).
The authors declare that the research was conducted in the absence of any commercial or financial relationships that could be construed as a potential conflict of interest.
The author(s) declare that no Generative AI was used in the creation of this manuscript.
All claims expressed in this article are solely those of the authors and do not necessarily represent those of their affiliated organizations, or those of the publisher, the editors and the reviewers. Any product that may be evaluated in this article, or claim that may be made by its manufacturer, is not guaranteed or endorsed by the publisher.
Abu A., Diamant R. (2019). A statistically-based method for the detection of underwater objects in sonar imagery. IEEE Sensors J. 19, 6858–6871. doi: 10.1109/JSEN.7361
Amari S.-I. (1993). Backpropagation and stochastic gradient descent method. Neurocomputing 5, 185–196. doi: 10.1016/0925-2312(93)90006-O
Cai Z., Vasconcelos N. (2018). “Cascade r-cnn: Delving into high quality object detection,” in Proceedings of the IEEE conference on computer vision and pattern recognition, Salt Lake City, UT, USA. 6154–6162. doi: 10.1109/CVPR.2018.00644
Cao H., Wang Y., Chen J., Jiang D., Zhang X., Tian Q., et al. (2022). “Swin-unet: Unet-like pure transformer for medical image segmentation,” in European conference on computer vision (Springer), Tel Aviv, Israel, 205–218. doi: 10.48550/arXiv.2105.05537
Character L., Ortiz A. Jr., Beach T., Luzzadder-Beach S. (2021). Archaeologic machine learning for shipwreck detection using lidar and sonar. Remote Sens. 13, 1759. doi: 10.3390/rs13091759
Chen Z., Ji H., Zhang Y., Zhu Z., Li Y. (2023). High-resolution feature pyramid network for small object detection on drone view. IEEE Trans. Circuits Syst. Video Technol. 34, 475–489. doi: 10.1109/TCSVT.2023.3286896
Chen J., Mai H., Luo L., Chen X., Wu K. (2021). “Effective feature fusion network in bifpn for small object detection,” in 2021 IEEE international conference on image processing (ICIP), Anchorage, AK, USA (IEEE), 699–703. doi: 10.1109/ICIP42928.2021.9506347
Dai L., Liu H., Tang H., Wu Z., Song P. (2022). Ao2-detr: Arbitrary-oriented object detection transformer. IEEE Trans. Circuits Syst. Video Technol. 33, 2342–2356. doi: 10.1109/TCSVT.2022.3222906
Deng W., Shi Q., Li J. (2021). Attention-gate-based encoder–decoder network for automatical building extraction. IEEE J. Selected Topics Appl. Earth Observations Remote Sens. 14, 2611–2620. doi: 10.1109/JSTARS.4609443
Duan K., Bai S., Xie L., Qi H., Huang Q., Tian Q. (2019). “Centernet: Keypoint triplets for object detection,” in Proceedings of the IEEE/CVF international conference on computer vision, Seoul, Korea (South). 6569–6578. doi: 10.1109/ICCV.2019.00667
Fakiris E., Blondel P., Papatheodorou G., Christodoulou D., Dimas X., Georgiou N., et al. (2019). Multi-frequency, multi-sonar mapping of shallow habitats—efficacy and management implications in the national marine park of zakynthos, Greece. Remote Sens. 11, 461. doi: 10.3390/rs11040461
Fu J., Liu J., Tian H., Li Y., Bao Y., Fang Z., et al. (2019). “Dual attention network for scene segmentation,” in Proceedings of the IEEE/CVF conference on computer vision and pattern recognition, Long Beach, CA, USA. 3146–3154. doi: 10.1109/CVPR.2019.00326
Guo M.-H., Xu T.-X., Liu J.-J., Liu Z.-N., Jiang P.-T., Mu T.-J., et al. (2022). Attention mechanisms in computer vision: A survey. Comput. Visual media 8, 331–368. doi: 10.1007/s41095-022-0271-y
He J., Chen J., Xu H., Ayub M. S. (2023). Small target detection method based on low-rank sparse matrix factorization for side-scan sonar images. Remote Sens. 15, 2054. doi: 10.3390/rs15082054
Hożyń S. (2021). A review of underwater mine detection and classification in sonar imagery. Electronics 10, 2943. doi: 10.3390/electronics10232943
Hurtos N., Ribas D., Cufí X., Petillot Y., Salvi J. (2015). Fourier-based registration for robust forward-looking sonar mosaicing in low-visibility underwater environments. J. Field Robotics 32, 123–151. doi: 10.1002/rob.21516
Kasetkasem T., Tipsuwan Y., Tulsook S., Muangkasem A., Leangaramkul A., Hoonsuwan P. (2020). A pipeline extraction algorithm for forward-looking sonar images using the self-organizing map. IEEE J. Oceanic Eng. 46, 206–220. doi: 10.1109/JOE.48
Komari Alaie H., Farsi H. (2018). Passive sonar target detection using statistical classifier and adaptive threshold. Appl. Sci. 8, 61. doi: 10.3390/app8010061
Kong W., Hong J., Jia M., Yao J., Cong W., Hu H., et al. (2019). Yolov3-dpfin: A dual-path feature fusion neural network for robust real-time sonar target detection. IEEE Sensors J. 20, 3745–3756. doi: 10.1109/JSEN.7361
Li Z., Liu F., Yang W., Peng S., Zhou J. (2021). A survey of convolutional neural networks: analysis, applications, and prospects. IEEE Trans. Neural Networks Learn. Syst. 33, 6999–7019. doi: 10.1109/TNNLS.2021.3084827
Li Y., Mao H., Girshick R., He K. (2022). Exploring plain vision transformer backbones for object detection. In: Avidan S., Brostow G., Cissé M., Farinella G. M., Hassner T. (eds) Computer Vision - ECCV 2022. ECCV 2022. Lecture Notes in Computer Science, 13669. (Springer, Cham). doi: 10.1007/978-3-031-20077-9_17
Li X., Song D., Dong Y. (2020). Hierarchical feature fusion network for salient object detection. IEEE Trans. Image Process. 29, 9165–9175. doi: 10.1109/TIP.83
Li Z., Xie Z., Duan P., Kang X., Li S. (2024). Dual spatial attention network for underwater object detection with sonar imagery. IEEE Sensors J. 24, 6998–7008. doi: 10.1109/JSEN.2023.3336899
Liu H., Ye X. (2023). Forward-looking sonar image stitching based on midline template matching in polar image. IEEE Trans. Geosci. Remote Sens. 62, 1–10. doi: 10.1109/TGRS.2023.3348153
Lv H., Qian W., Chen T., Yang H., Zhou X. (2022). Multiscale feature adaptive fusion for object detection in optical remote sensing images. IEEE Geosci. Remote Sens. Lett. 19, 1–5. doi: 10.1109/LGRS.2022.3178787
Neettiyath U., Sugimatsu H., Koike T., Nagano K., Ura T., Thornton B. (2024). Multirobot multimodal deep sea surveys: Use in detailed estimation of manganese crust distribution. IEEE Robotics Automation Magazine 31, 84–95. doi: 10.1109/MRA.2023.3348304
Ren S., He K., Girshick R., Sun J. (2016). Faster r-cnn: Towards real-time object detection with region proposal networks. IEEE Trans. Pattern Anal. Mach. Intell. 39, 1137–1149. doi: 10.1109/TPAMI.2016.2577031
Ross T.-Y., Dollár G. (2017). “Focal loss for dense object detection,” in proceedings of the IEEE conference on computer vision and pattern recognition. 2980–2988.
Shi P., He Q., Zhu S., Li X., Fan X., Xin Y. (2024). Multi-scale fusion and efficient feature extraction for enhanced sonar image object detection. Expert Syst. Appl. 256, 124958–124969. doi: 10.1016/j.eswa.2024.124958
Singh D., Valdenegro-Toro M. (2021). “The marine debris dataset for forward-looking sonar semantic segmentation,” in Proceedings of the ieee/cvf international conference on computer vision, Montreal, BC, Canada. 3741–3749. doi: 10.1109/ICCVW54120.2021.00417
Sun P., Zhang R., Jiang Y., Kong T., Xu C., Zhan W., et al. (2021). “Sparse r-cnn: End-to-end object detection with learnable proposals,” in Proceedings of the IEEE/CVF conference on computer vision and pattern recognition, Nashville, TN, USA. 14454–14463. doi: 10.1109/CVPR46437.2021.01422
Tan M., Pang R., Le Q. V. (2020). “Efficientdet: Scalable and efficient object detection,” in Proceedings of the IEEE/CVF conference on computer vision and pattern recognition, Seattle, WA, USA. 10781–10790. doi: 10.1109/CVPR42600.2020.01079
Tao A., Sapra K., Catanzaro B. (2020). Hierarchical multi-scale attention for semantic segmentation. Computer Vision and Pattern Recognition, arXiv preprint arXiv:2005.10821. doi: 10.48550/arXiv.2005.10821
Tong Z., Chen Y., Xu Z., Yu R. (2023). Wise-iou: bounding box regression loss with dynamic focusing mechanism. Computer Vision and Pattern Recognition, arXiv preprint arXiv:2301.10051. doi: 10.48550/arXiv.2301.10051
Ultralytics (2023). Yolov8: A state-of-the-art object detection model. Available online at: https://github.com/ultralytics/ultralytics (Accessed 2023-10-15).
Ultralytics (2024). Yolov10: The next generation of real-time object detection. Available online at: https://github.com/ultralytics/ultralytics (Accessed 2024-01-01).
Wang C.-Y., Bochkovskiy A., Liao H.-Y. M. (2023). “Yolov7: Trainable bag-of-freebies sets new state-of-the-art for real-time object detectors,” in Proceedings of the IEEE/CVF conference on computer vision and pattern recognition, Vancouver, BC, Canada. 7464–7475.
Wang J., Feng C., Wang L., Li G., He B. (2022a). Detection of weak and small targets in forward-looking sonar image using multi-branch shuttle neural network. IEEE Sensors J. 22, 6772–6783. doi: 10.1109/JSEN.2022.3147234
Wang Z., Guo J., Zeng L., Zhang C., Wang B. (2022b). Mlffnet: Multilevel feature fusion network for object detection in sonar images. IEEE Trans. Geosci. Remote Sens. 60, 1–19. doi: 10.1109/TGRS.2022.3214748
Wang Z., Zhang S., Huang W., Guo J., Zeng L. (2021). Sonar image target detection based on adaptive global feature enhancement network. IEEE Sensors J. 22, 1509–1530. doi: 10.1109/JSEN.2021.3131645
Wang W., Zhang Q., Qi Z., Huang M. (2024). Centernet-saccade: Enhancing sonar object detection with lightweight global feature extraction. Sensors 24, 2357–2368. doi: 10.3390/s24020665
Wen X., Wang J., Cheng C., Zhang F., Pan G. (2024). Underwater side-scan sonar target detection: Yolov7 model combined with attention mechanism and scaling factor. Remote Sens. 16, 2492–2503. doi: 10.3390/rs16132492
Zhang H., Wang Y., Dayoub F., Sunderhauf N. (2021). “Varifocalnet: An iou-aware dense object detector,” in Proceedings of the IEEE/CVF conference on computer vision and pattern recognition, Nashville, TN, USA. 8514–8523. doi: 10.1109/CVPR46437.2021.00841
Zhang M., Cai W., Wang Y., Zhu J. (2023a). A level set method with heterogeneity filter for side-scan sonar image segmentation. IEEE Sensors J. 24, 584–595. doi: 10.1109/JSEN.2023.3334765
Zhang Y., Wu C., Zhang T., Liu Y., Zheng Y. (2023b). Self-attention guidance and multiscale feature fusion-based uav image object detection. IEEE Geosci. Remote Sens. Lett. 20, 1–5. doi: 10.1109/LGRS.2023.3265995
Zhou T., Si J., Wang L., Xu C., Yu X. (2022). Automatic detection of underwater small targets using forward-looking sonar images. IEEE Trans. Geosci. Remote Sens. 60, 1–12. doi: 10.1109/TGRS.2022.3181417
Zhao Z., Wang Z., Wang B., Guo J. (2023). Rmfenet: Refined multi-scale feature enhancement network for arbitrary oriented sonar object detection. IEEE Sensors J. 23, 29211–29226. doi: 10.1109/JSEN.2023.3324476
Keywords: marine object detection, forward-looking sonar, semantic-spatial feature enhancement, attention mechanism, feature fusion
Citation: Wang Z, Guo J, Zhang S and Xu N (2025) Marine object detection in forward-looking sonar images via semantic-spatial feature enhancement. Front. Mar. Sci. 12:1539210. doi: 10.3389/fmars.2025.1539210
Received: 04 December 2024; Accepted: 21 January 2025;
Published: 13 February 2025.
Edited by:
Yue Ma, Wuhan University, ChinaReviewed by:
Huang Yu-An, Northwestern Polytechnical University, ChinaCopyright © 2025 Wang, Guo, Zhang and Xu. This is an open-access article distributed under the terms of the Creative Commons Attribution License (CC BY). The use, distribution or reproduction in other forums is permitted, provided the original author(s) and the copyright owner(s) are credited and that the original publication in this journal is cited, in accordance with accepted academic practice. No use, distribution or reproduction is permitted which does not comply with these terms.
*Correspondence: Nan Xu, aGh1eHVuYW5AZ21haWwuY29t
Disclaimer: All claims expressed in this article are solely those of the authors and do not necessarily represent those of their affiliated organizations, or those of the publisher, the editors and the reviewers. Any product that may be evaluated in this article or claim that may be made by its manufacturer is not guaranteed or endorsed by the publisher.
Research integrity at Frontiers
Learn more about the work of our research integrity team to safeguard the quality of each article we publish.