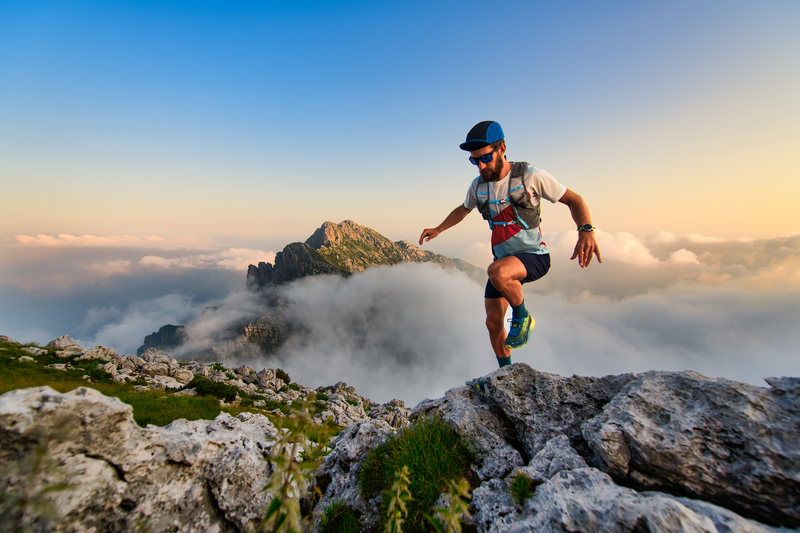
95% of researchers rate our articles as excellent or good
Learn more about the work of our research integrity team to safeguard the quality of each article we publish.
Find out more
ORIGINAL RESEARCH article
Front. Mar. Sci.
Sec. Coastal Ocean Processes
Volume 12 - 2025 | doi: 10.3389/fmars.2025.1537066
This article is part of the Research Topic Geoinformatics and Machine Learning for the Study of Coastal Systems View all articles
The final, formatted version of the article will be published soon.
You have multiple emails registered with Frontiers:
Please enter your email address:
If you already have an account, please login
You don't have a Frontiers account ? You can register here
Understanding beach dynamics and the long-term evolution of beach nourishment projects is critical for sustainable coastal management, particularly in the face of rising sea levels and increasingly variable storm climates. This study examines the development of a large-scale sand nourishment (600,000 m³) in the southwestern Baltic Sea over 25 months (October 2021-November 2023) using UAV-derived digital surface models (DSMs) and machine learning (ML). High-frequency, multitemporal UAV surveys enabled detailed analyses of the development of the nourished beach and dune. Results revealed that the volumetric impact of the 100-year flood in October 2023 was comparable to the cumulative effects of the October 2022-January 2023 storm season. This demonstrates that both episodic extreme events and the cumulative impacts shape the morphological evolution of the nourishment. The study also highlights sediment transport reversals under easterly winds, promoting longer-term stability by retaining sediment within the system. By standardizing volumetric analyses using tools equipped with ML, this research provides actionable insights for adaptive management and establishes a framework for comparable, accurate assessments of nourishment lifetime. In particular, these methods efficiently capture subtle variations in coastline orientation, wave incidence angles, and resulting alongshore beach dynamics, offering valuable insights for optimizing nourishment strategies. These findings underscore the importance of continuous, high-resolution monitoring in developing sustainable strategies for storm-driven erosion and sea level rise.
Keywords: sand nourishments1, Machine Learning2, RTK-UAV3, co-alignment4, 100-year flood5
Received: 29 Nov 2024; Accepted: 01 Apr 2025.
Copyright: © 2025 Tiede, Leon Lovell, Jordan, Moghimi and Schlurmann. This is an open-access article distributed under the terms of the Creative Commons Attribution License (CC BY). The use, distribution or reproduction in other forums is permitted, provided the original author(s) or licensor are credited and that the original publication in this journal is cited, in accordance with accepted academic practice. No use, distribution or reproduction is permitted which does not comply with these terms.
* Correspondence:
Jan Tiede, Leibniz University Hannover, Hanover, Germany
Disclaimer: All claims expressed in this article are solely those of the authors and do not necessarily represent those of their affiliated organizations, or those of the publisher, the editors and the reviewers. Any product that may be evaluated in this article or claim that may be made by its manufacturer is not guaranteed or endorsed by the publisher.
Research integrity at Frontiers
Learn more about the work of our research integrity team to safeguard the quality of each article we publish.