- Department of Biology, College of Natural Sciences, Soonchunhyang University, Asan, Republic of Korea
Introduction: As coastal urbanization progresses, anthropogenic activities have significantly impacted mudflats. In South Korea, land reclamation and aquaculture have drastically altered mudflat ecosystems.
Methods: In our study, we utilized five remote sensing indices to assess the impact of anthropogenic activities on Hwangdo Island. We supplemented this with field surveys to evaluate macrobenthic biodiversity and benthic ecological quality, investigating their relationship to anthropogenic activities.
Results and discussion: Spearman’s correlation analysis revealed that terrestrial ecological quality influences macrobenthic biodiversity. The abundance of the predominant species, Pirenella sp., was primarily influenced by normalized differential build-up and bare soil index (NDBSI). Furthermore, biota-environment matching (BIO-ENV) analysis, distance-based multivariate analysis for a linear model (DisLM), and distance-based redundancy analysis (dbRDA) all pinpointed NDBSI as the predominant factor impacting macrobenthic communities in the mudflats of Hwangdo Island. Seasonal changes in NDBSI were mainly attributed to variations in bare soil area resulting from agricultural activities on Hwangdo Island. Overall, macrobenthic communities in the mudflats of Hwangdo Island are mainly indirectly affected by agricultural activities. Moreover, our study offers a fresh perspective on the conservation of Hwangdo Island’s mudflats and provides critical references for the South Korean government in crafting and implementing mudflat protection policies.
1 Introduction
Mudflats are vital transitional zones between terrestrial and marine environments, significantly enhancing marine ecosystems and providing various social services to humans (Zhu et al., 2023). Specifically, mudflats offer ecological services such as wildlife habitats and nutrient cycling, alongside social services including aquaculture and tourism (Lebreton et al., 2019; Newton et al., 2020). Yet, deepening coastal urbanization has led to substantial impacts from anthropogenic activities on mudflats (Zhang et al., 2015). Activities like land reclamation and sewage discharge have decreased mudflat areas and reduced biodiversity (Yang and Chui, 2017; Tan et al., 2016). Although mudflat conservation has garnered extensive attention and many studies have assessed the impact of anthropogenic activities on their biodiversity (Rossi et al., 2007; Du et al., 2019), research on the effects of terrestrial anthropogenic activities on mudflats remains insufficient.
Macrobenthos are essential to mudflat ecosystems (Mohamamad and Jalal, 2018). They serve as a crucial food source for predators and play a vital role in the carbon and nitrogen cycles of mudflats (Pascal et al., 2019; Cook et al., 2004). It is well-established that macrobenthos can reflect the environmental conditions of their habitats (Liang et al., 2024a). This is attributed to their relatively long lifespan, typically around two years, and their sedentary or sessile lifestyle (Baldrighi et al., 2023). Indices based on macrobenthos have been extensively used to assess the ecological quality of coastal environments (Liang et al., 2024b, c). Among these, the AZTI’s Marine Biotic Index (AMBI) and Multivariate AZTI’s Marine Biotic Index (M-AMBI) are particularly prominent in gauging the ecological quality of coastal areas globally (Borja et al., 2019).
In South Korea, mudflats are predominantly located along the western and southern coasts. With rapid economic expansion since the 1970s, the country has experienced escalated land-use conflicts. The South Korean government has extensively undertaken land reclamation projects (Choi, 2014), with the reclaimed area along the western coast totaling 1,700 km² (Koh and Khim, 2014). This has led to irreversible ecological consequences, reducing mudflat areas and causing habitat loss for wildlife (Choi et al., 2010). Although there has been a growing recognition of mudflats’ importance by the South Korean government, their conservation policies currently focus only on the mudflats.
Since the 1960s, the advent of remote sensing technology has evolved over more than five decades. It is now routinely used to evaluate the impacts of human activities on the environment (Liu et al., 2024) and to assess biodiversity in intertidal zones. For instance, the study by Rossiter et al. (2020) utilized remote sensing to determine the distribution of intertidal macroalgae. However, few studies have employed remote sensing to examine the impacts of terrestrial human activities on macrobenthos. Specifically, the research by El-Naggar et al. (2022) investigated the influences of land use and land cover changes on macrobenthos biodiversity. However, further evaluation of additional terrestrial anthropogenic impacts on macrobenthos biodiversity is warranted.
In our study, we utilized four remote sensing indices (i.e., Wetness, NDVI, LST, and NDBSI) to investigate anthropogenic activities on the land surrounding mudflats and a remote sensing ecological index (RSEI) to assess the ecological quality of the terrestrial environment. Together with field survey data, we comprehensively evaluated the impact of human activities on the macrobenthos biodiversity of mudflats. Moreover, our study provides crucial references for the South Korean government in formulating mudflat conservation policies and sheds light on avenues for future research.
2 Materials and methods
2.1 Study area
The study area, Hwangdo Island, is located at coordinates 36°35’47” to 36°36’13” N and 126°22’27” to 126°22’50” E, just north of Cheonsu Bay (Figure 1). Hwangdo Island has an east-west extension of approximately 1.31 km and a north-south width of about 1.65 km, covering a total area of approximately 0.71 km². The buildings on Hwangdo Island are concentrated on the right side, while the rest of the island is primarily composed of farmland and green spaces (Figure 2). The elevation of Hwangdo Island ranges from 0 to 41 m (Figure 2).
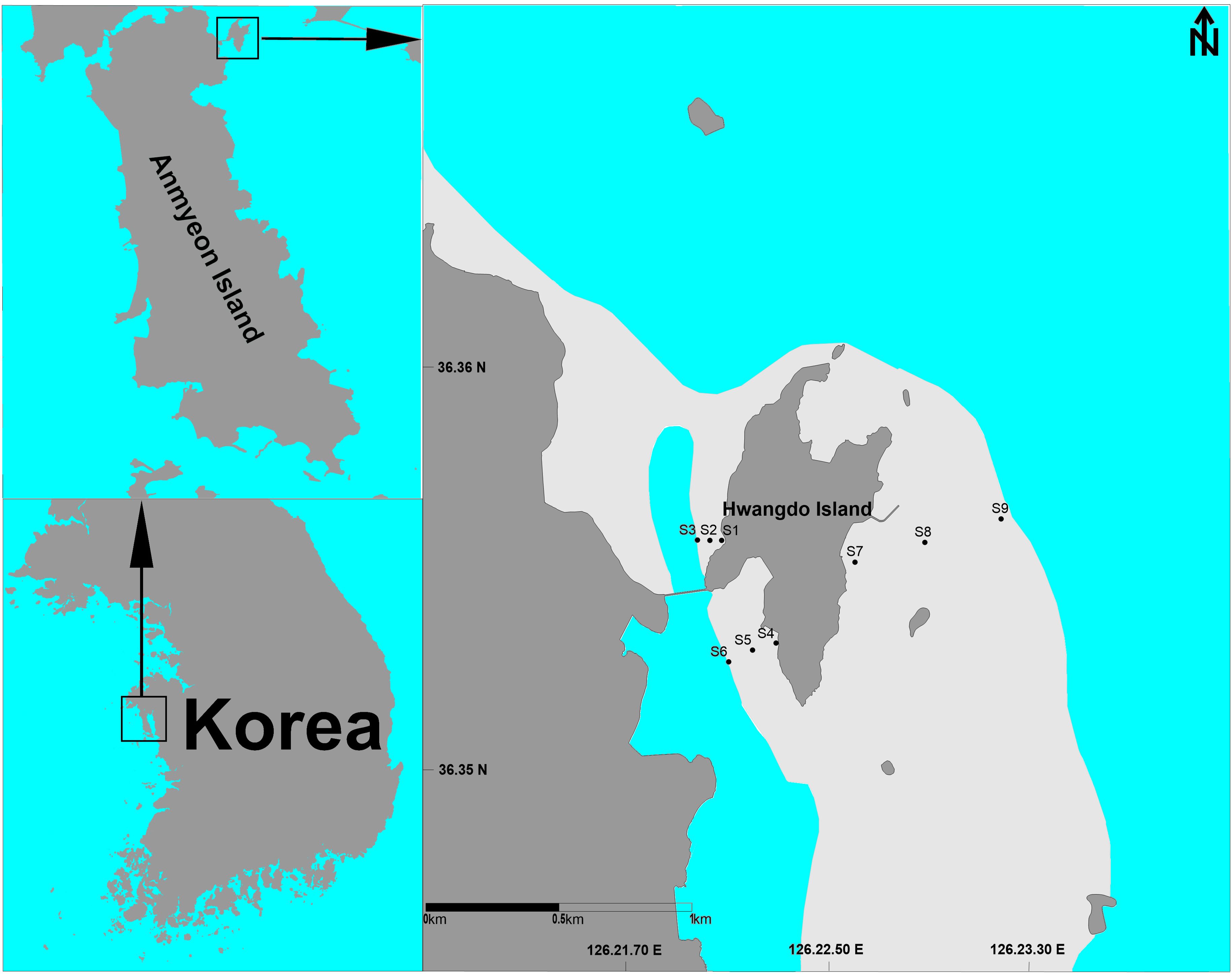
Figure 1. Study area and sampling stations on the mudflats of Hwangdo Island, South Korea. Blue zone, ocean; Grey zone, Land; Light-grey zone, Mudflat.
The average tidal range here is 4.59 m, with rainfall predominantly occurring in the summer. The mudflats of Hwangdo Island are significantly impacted by the influx of eutrophic freshwater from the artificial lake to the north and by shellfish farming (Liang et al., 2024d).
2.2 Sample collection and processing
Nine sampling stations were assessed in the mudflats of Hwangdo Island (Figure 1), with stations 1, 4, and 7 in the upper tidal region, stations 2, 5, and 8 in the middle tidal region, and stations 3, 6, and 9 in the lower tidal region. Sampling occurred during the spring tides of February, May, August, and October 2021. At each station, macrobenthic samples were collected twice using a 0.5-meter quadrat sampler, yielding a total sampling area of 0.5m2. In the field, these samples were sieved using a 0.5 mm mesh, and preserved in 5% neutral formalin solution.
During the collection, 500 g of surface sediment samples were also gathered and preserved at -30°C prior to analysis. Salinity and dissolved oxygen (DO) levels of tidal water were measured using a handheld multiparameter instrument (YSI-556, YSI Inc, USA), while the laser rangefinder (LASER 600, Nikon Ltd, Japan) and the personal navigation assistant (GPSMAP 64S, Garmin Ltd, USA) aided in distinguishing tidal regions.
In the laboratory, macrobenthic samples were identified to species level with a stereomicroscope (SZX-10, Olympus Ltd, Japan) and weighed using analytical balances (CP-64, Sartorius AG, Deutschland). chemical oxygen demand (COD) and acid-volatile sulfide (AVS) concentrations in the sediment samples were quantified using titration and detection tube methods, respectively. Sediment samples were heated at 600°C for 2 hours to measure ignition loss, and the mean grain size was determined through dry sieving and pipetting methods. Analyses adhered to the Marine Environmental Process Test Method (National Institute of Fisheries Science, 2010).
2.3 Biotic indices
To determine the dominant species within the macrobenthic community, the relative importance index (IRI) was utilized (Zhao et al., 2024). The formula is as follows:
In this formula, ‘A’ signifies the proportion of a specific taxon’s abundance relative to the total abundance.
‘B’ represents the proportion of a specific taxon’s biomass relative to the total biomass, while ‘F’ indicates the frequency of occurrence. A species is deemed dominant when its IRI value exceeds 1000.
To evaluate the diversity of the macrobenthic community, four traditional diversity indices (i.e., species richness index, Pielou’s evenness index, Simpson index, and Shannon-Wiener diversity index) and two taxonomic distinctness (TD) indices (i.e., average taxonomic distinctness and variation in taxonomic distinctness) were employed (Hill, 1973; Warwick and Clarke, 1995). The formulas for these six diversity indices are displayed in Supplementary Table S1.
Understanding benthic ecological quality is vital for environmental protection. AMBI, Benthic Pollution Index (BPI), and M-AMBI, which are extensively used in South Korea (Liang et al., 2024e), were utilized to assess the benthic ecological quality in Hwangdo Island’s mudflat. AMBI categorizes macrobenthos into five ecological groups according to their sensitivity to organic matter (Borja et al., 2000). Conversely, BPI classifies them into four functional groups based on their feeding types and life history (Liang et al., 2024f). M-AMBI employs factor analysis to integrate AMBI, diversity indices, and species richness for calculation (Muxika et al., 2007). The formulas for these three benthic indices are presented in Supplementary Table S2.
2.4 Remote-sensing indices
With advancements in remote sensing technology, remote sensing-based indices have become widely used to evaluate the impact of human activities on the environment (Li et al., 2020a). To assess human activities on Hwangdo Island, four remote sensing indices were selected: Wetness (Land surface moisture index), NDVI (Normalized Difference Vegetation Index), LST (Land surface temperature index), and NDBSI (Normalized Differential Build-up and Bare Soil Index), along with the remote sensing ecological index (RSEI), which assesses the island’s environmental ecological quality. Due to varying spectral reflectance characteristics of different surface features across wavelength bands, Wetness, NDVI, LST, and NDBSI calculations depend on specific bands (Shan et al., 2019). Wetness indicates surface moisture, NDVI measures greenness, LST indicates surface temperature, and NDBSI indicates dryness (Zhang et al., 2022a). RSEI is calculated using principal component analysis (PCA) and normalization of these four indices (Zhang et al., 2021). The formulas for the five remote-sensing indices are listed in Supplementary Table S3.
The remote sensing images were synchronized with field sampling times. The range of the remote sensing images encompassed the entire terrestrial area of the island. Images for February and May were obtained from Landsat 8, while those for August and October were from Landsat 7. The images were downloaded from the United States Geological Survey website (www.usgs.gov). Since the RSEI is not applicable to water bodies, a masking process was applied to exclude water bodies in this study to avoid influencing the RSEI’s evaluation results (Chen et al., 2023). These remote-sensing images underwent radiometric, atmospheric, and geometric accuracy corrections and had a resolution of 30 meters (Geng et al., 2022). The calculation of the five remote sensing indices was performed using the software Envi 5.3.0.
2.5 Data analyses
To assess environmental characteristics in the mudflats of Hwangdo Island, environmental factors underwent log(x+1) transformation and normalization before principal component analysis (PCA). Spearman’s rank correlation analysis explored the relationships among macrobenthic biodiversity, benthic indices, environmental factors, and remote sensing indices. The redundancy analysis (RDA) examined the associations between dominant species, environmental factors, and remote sensing indices. Prior to the RDA, environmental factors and remote sensing indices were transformed using log(x+1). The Biota-Environment Matching (BIO-ENV) analysis, distance-based multivariate analysis for a linear model (DisLM), and distance-based redundancy analysis (dbRDA) investigated the impact of environmental factors and remote sensing indices on macrobenthic community structure (Clarke et al., 2014). Before conducting these three analyses, environmental factors and remote sensing indices were transformed by log(x+20) and normalized.
Spearman correlation analysis was conducted using OriginPro 2021 (OriginLab Ltd, USA). PCA, BIO-ENV, DisLM, and dbRDA were performed using PRIMER version 7 (PRIMER-E Ltd, NZL), and RDA was implemented using Canoco 5 (www.canoco5.com).
3 Results
3.1 Environment characteristics and remote sensing indices
Table 1 displays the ranges and means of environmental factors. Among the six environmental factors, the coefficient of variation for AVS was the highest. In principal component analysis, the PC1 and PC2 axes accounted for 48.7% of the variance in environmental factors. Stations in February, compared to other months, were primarily located on the left side of the PCA figure (Figure 3), demonstrating that IL, AVS, and DO levels were higher in February than in other months (Supplementary Table S4).
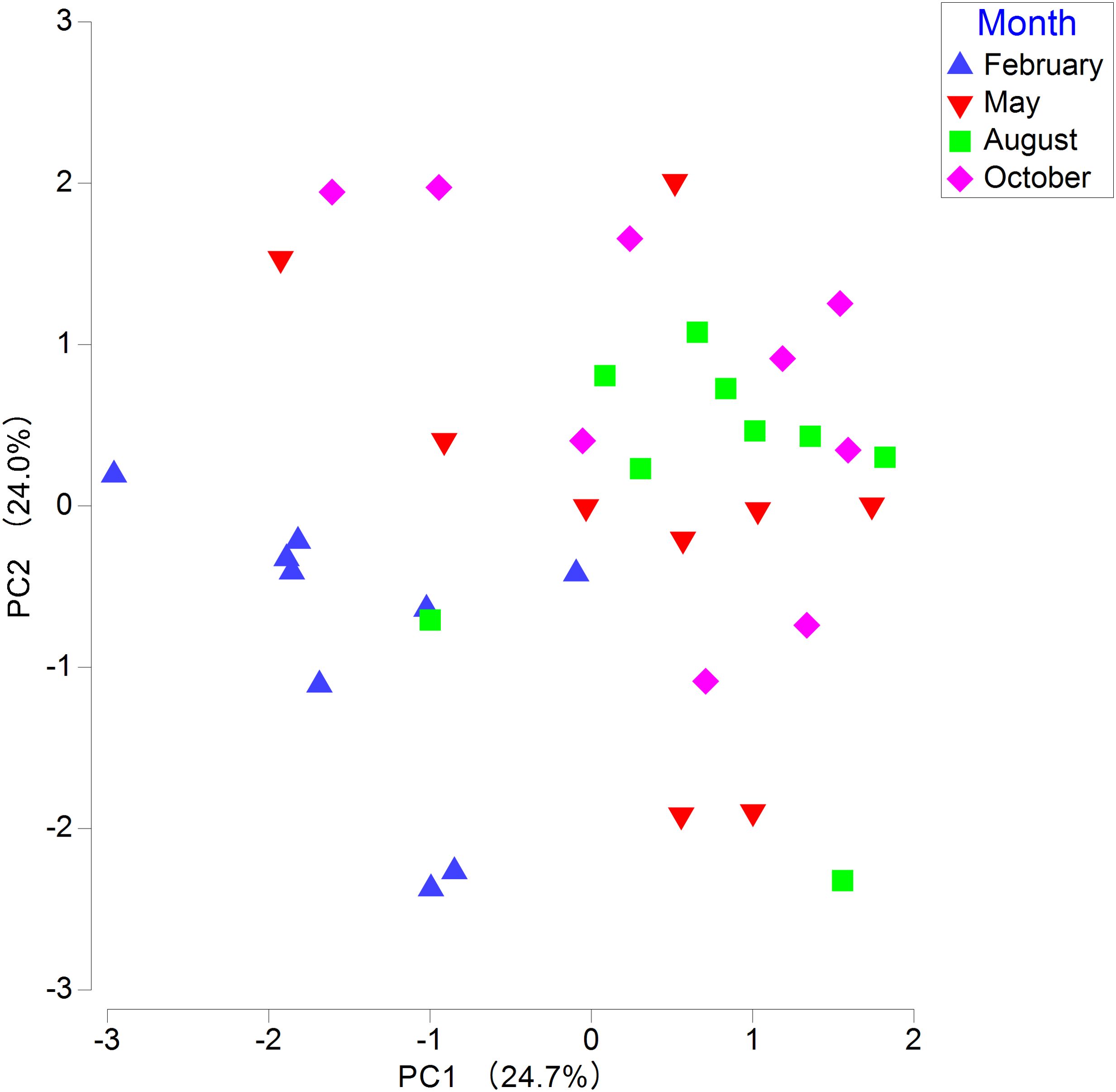
Figure 3. Principal component analysis (PCA) of environmental factors in the mudflat of Hwangdo Island.
The average values for four remote sensing indices (i.e., RSEI, NDVI, Wetness, and LST) were lowest in February, while the average value of NDBSI was lowest in August. Three remote sensing indices (NDVI, Wetness, and LST) reached their peak average values in August; the highest average value of RSEI occurred in May, and LST also had its highest average in August. The averages of remote sensing indices for Hwang Island across four months are summarized in Supplementary Table S5. The environmental ecological quality on Hwangdo Island was lowest in February and highest in May (Figure 4).
3.2 Macrobenthos characteristics and dominant species
In our study, we identified a total of 129 taxa of macrobenthos. Annelids were the most numerous, comprising 50 taxa, followed by mollusks with 36 taxa, arthropods with 32 taxa, echinoderms with 3 taxa, and other animals with 8 taxa. In February, the number of species reached its minimum with 59 taxa, whereas in October, it peaked at 76 taxa (Figure 5). The average abundance of species was 263.6 individuals per m2, and the average biomass was 104.6 g per m2 in the mudflat of Hwangdo Island. Supplementary Figures S1 and S2 depict the abundance and biomass of species at each station. Three dominant taxa were identified, among which Pirenella sp. displayed the highest IRI value of 2587 (Table 2).
3.3 Diversity indices
The species richness index (d) averaged the lowest at 3.01 in February, while the highest average was 4.10 in May. The Pielou’s evenness index (J’) averaged the lowest at 0.70 in October, while the highest averages of 0.79 occurred in May and August. The Shannon-Wiener diversity index (H’) had its lowest average values of 2.72 in February and October, peaking at 3.40 in May. The Simpson index (1-Lambda’) saw its lowest average at 0.73 in October, with the highest at 0.86 in May. The average taxonomic distinctness (Delta+) was lowest at 88.82 in May, and highest at 90.17 in August. In contrast, the variation in taxonomic distinctness (Lambda+) varied from a low of 265.82 in February to a high of 362.02 in August (Figure 6). Diversity indices for each station are shown in Supplementary Figures S3-S8.
Delta+ values at 11 stations fell below the theoretical average, while Lambda+ values at 24 stations were also beneath the theoretical average. Notably, the Delta+ value at station 9 in October deviated from the 95% confidence interval. Similarly, the Lambda+ values at station 4 in October and August, and station 9 in August, deviated from the 95% confidence interval (Figure 7).
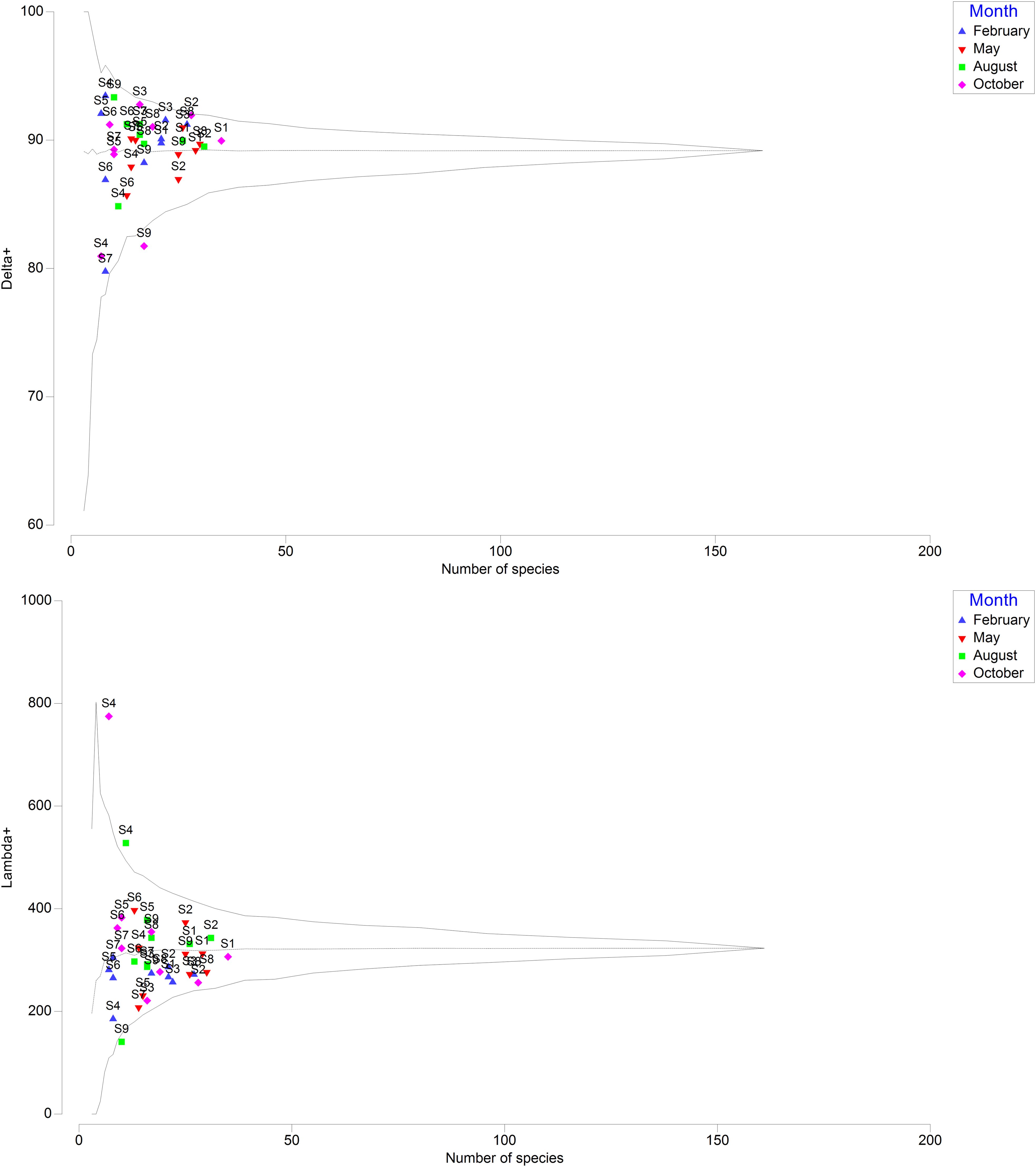
Figure 7. The 95% confidence interval funnel charts demonstrate the distribution of classification indices (Delta+ and Lambda+) at the sampling stations.
3.4 Benthic ecological quality
The lowest average value of AMBI in October was 1.25, whereas the highest occurred in February at 1.70. The lowest average value of BPI in February was 69.45, and the highest was 77.43 in October. The lowest and highest average values of M-AMBI in February and May were 0.58 and 0.68, respectively (Figure 8). AMBI and BPI rated the benthic ecological quality at all stations as either high or good (Supplementary Figures S9, S10). Although M-AMBI rated most stations as high or good, it classified stations 4-7 in February, station 9 in August, and station 4 in October as moderate (Supplementray Figure S11).
3.5 Statistical analysis
In Spearman’s correlation analysis, the mean grain size showed a positive correlation with the number of species, species abundance, d, H’, AMBI, and M-AMBI. IL correlated positively with species abundance yet negatively with J’ and 1-Lambda’. DO negatively correlated with species biomass and Lambda+. Furthermore, AVS exhibited a negative correlation with Lambda+ (Figure 9).
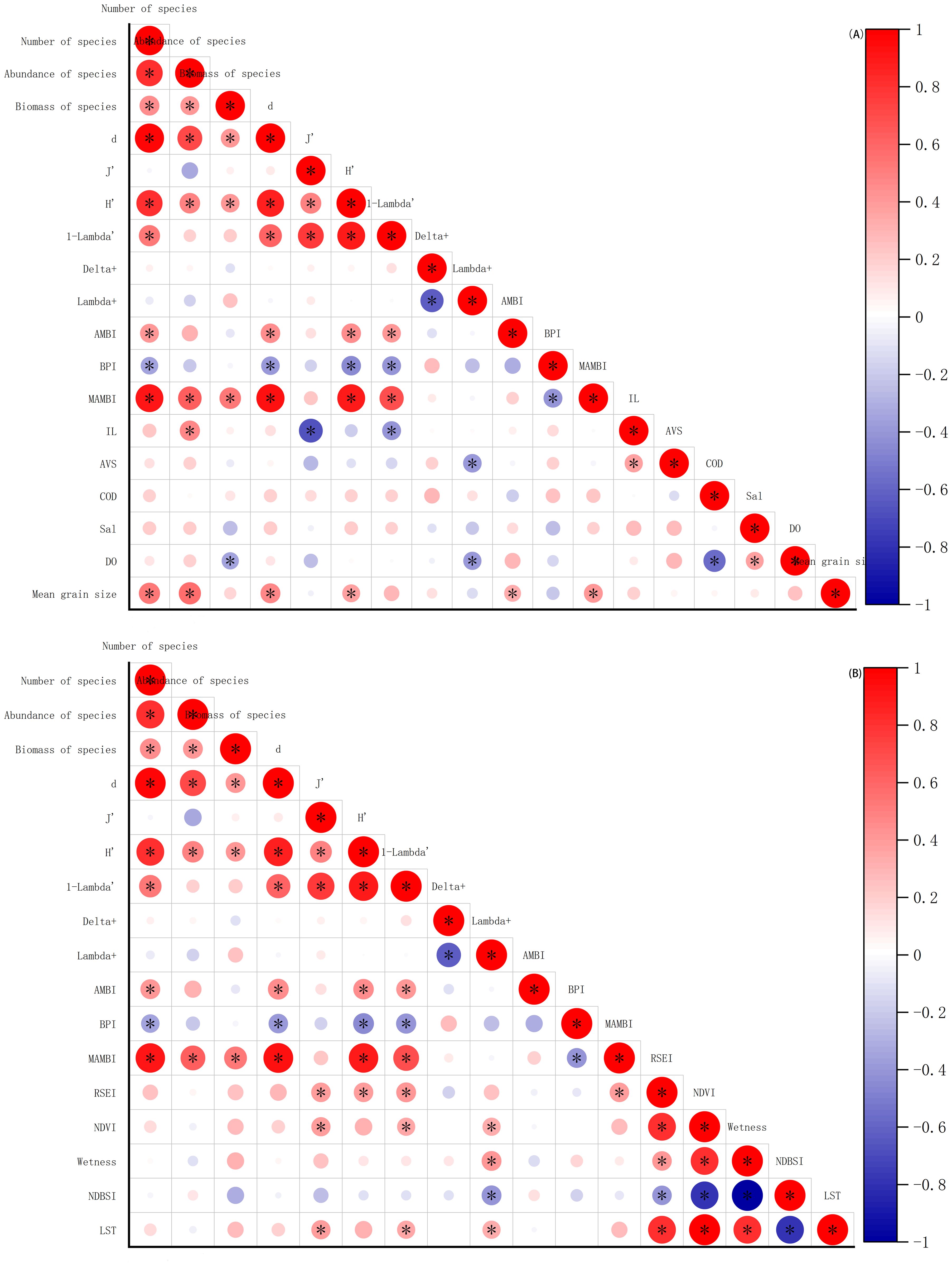
Figure 9. Results of Spearman’s correlation analysis among environmental factors, remote sensing indices, diversity indices, and benthic indices. *, p<0.05.
RSEI was positively correlated with J’, H,’ 1-Lambda’, and M-AMBI. NDVI correlated positively with J’, H,’ and 1-Lambda’. Wetness showed a positive correlation with Lambda+, while NDBSI was negatively correlated with Lambda+. LST correlated positively with J’, 1-Lambda’, and Lambda+ (Figure 9B).
The results of the RDA analysis indicated that Axis 1 and Axis 2 collectively explained 44.4% of the variation in dominant species. Pirenella sp was positively correlated with salinity (Sal), NDBSI, DO, mean grain size, and AVS. Macrophthalmus japonicus also showed a positive correlation with Sal, NDBSI, DO, and mean grain size. Conversely, Nassarius festivus was negatively correlated with COD, NDVI, and RSEI, while Ruditapes philippinarum showed negative correlations with Sal, NDBSI, and DO (Figure 10).
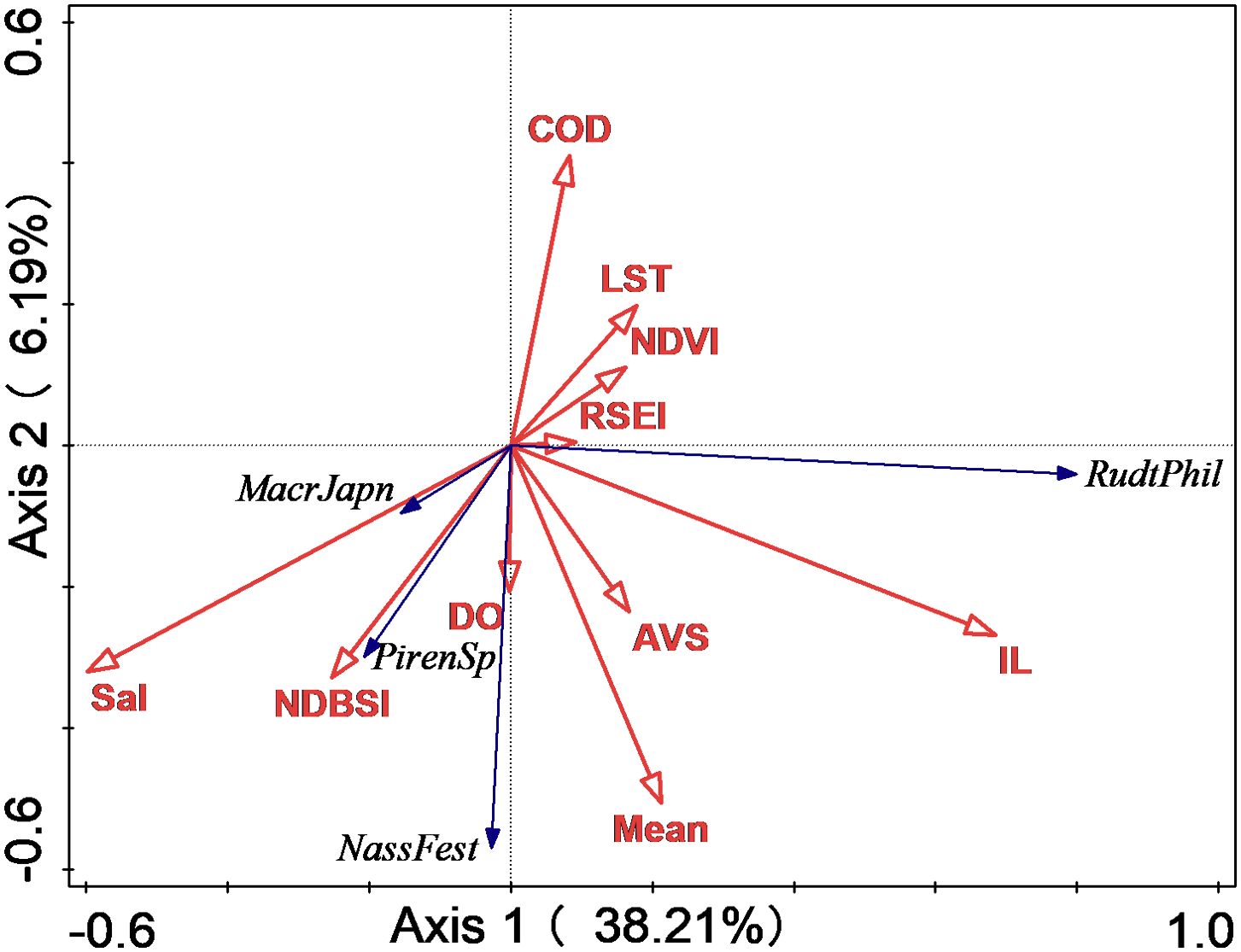
Figure 10. Redundancy analysis (RDA) ranking diagram of environmental factors, remote sensing indices, and dominant species in the mudflats of Hwangdo Island. AVS, acid-volatile sulfide; COD, chemical oxygen demand; DO, dissolved oxygen; IL, ignition loss; Sal, salinity; Mean, mean grain size; RSEI, remote sensing ecological index; NDBSI, normalized difference built-up and soil index; NDVI, normalized difference vegetation index; PirenSp, Pirenella sp; RudtPhil, Ruditapes philippinarum; MacrJapn, Macrophthalmus japonicus; NassFest, Nassarius festivus.
The dbRDA analysis indicated that environmental factors and remote sensing indices impact the community structure of benthic organisms. In the fitted model, dbRDA axes 1 and 2 explained 46.4% of the variability. Specifically, 17.6% of the total variation was accounted for by dbRDA axes 1 and 2 (Figure 11). In Figure 10, stations sampled in February and May were primarily located in the upper left quadrant, demonstrating that NDBSI and NDVI predominantly influenced the benthic community structure. Conversely, stations sampled in August and October were mainly located in the lower right quadrant, indicating that NDBSI and AVS primarily affected the community structure. Eigenvectors of environmental factors and remote sensing indices for the dbRDA axes 1 and 2 are detailed in Supplementary Table S6.
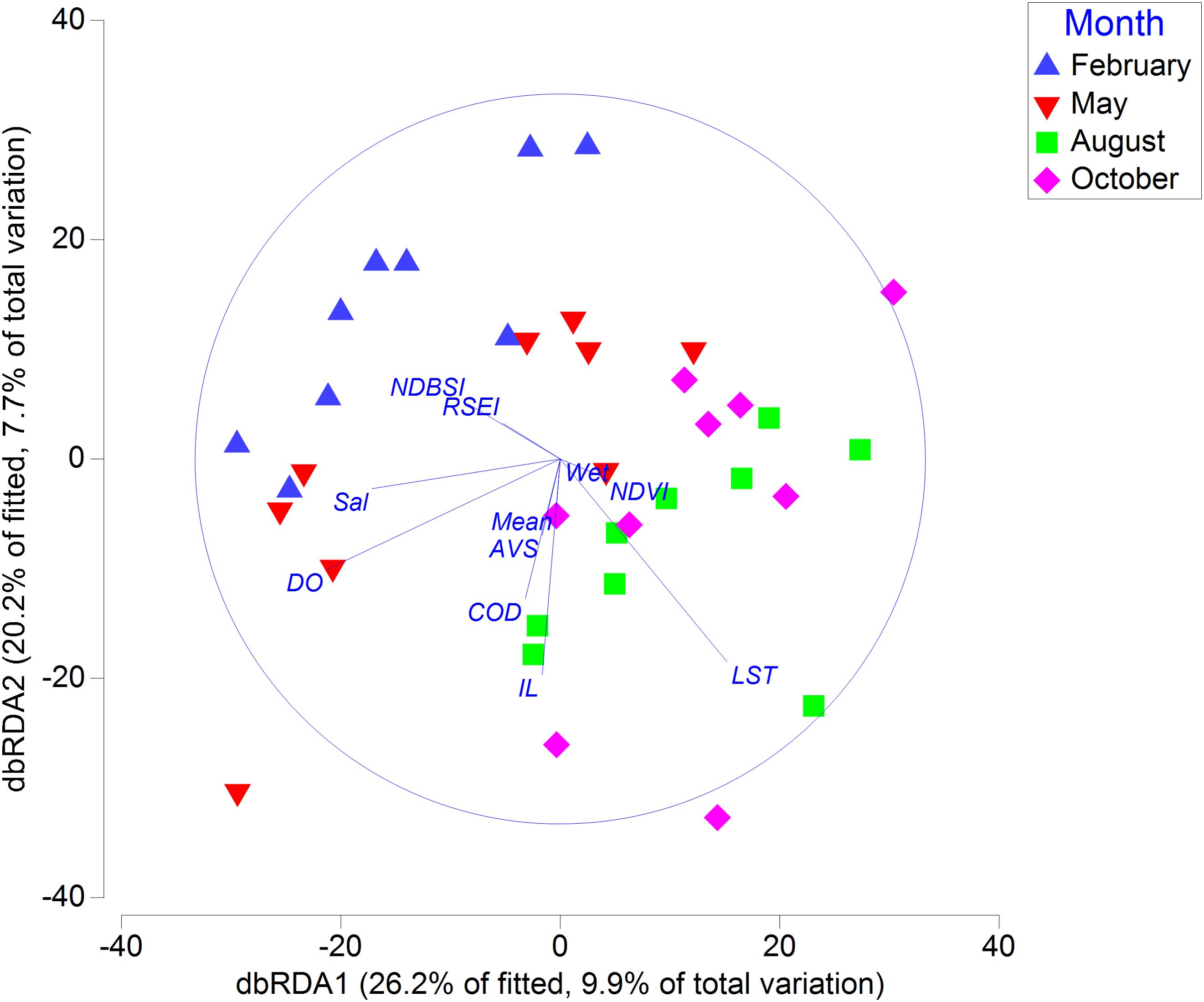
Figure 11. Distance-based redundancy analysis (dbRDA) ordination visually represents the relationships between stations constrained by macrobenthos communities, environmental variables, and remote sensing indices. AVS, acid-volatile sulfide; COD, chemical oxygen demand; DO, dissolved oxygen; IL, ignition loss; Sal, Salinity; Mean, mean grain size; RSEI, remote sensing ecological index; NDBSI, normalized difference built-up and soil index; NDVI, normalized difference vegetation index.
Results from the best linear models based on distance (DistLM) indicated that IL (F=1.53, p<0.05), COD (F=1.84, p<0.05), Sal (F=2.02, p<0.01), DO (F=2.31, p<0.01), mean grain size (F=2.01, p<0.01), wetness (F=2.14, p<0.01), NDBSI (F=2.86, p<0.01), and LST (F=2.31, p<0.01) significantly influenced the community structure of macrobenthic organisms. In BIO-ENV analysis, NDBSI had the highest impact on the macrobenthic community structure (Supplementary Table S7).
4 Discussion
4.1 Environmental characteristics and remote sensing indices in Hwangdo, Island
In our study, the mean grain size of the mudflat on Hwangdo Island was found to be similar to the results reported by Lee et al. (2023). The concentrations of AVS, COD, and IL in the mudflat of Hwangdo Island were lower than those found in the subtidal sediments of the northern part of Cheonsu Bay (Yoon et al., 2021).
The average RSEI value on Hwangdo Island was lower than that of Haitan Island in China, which is undergoing rapid urbanization (Lin et al., 2022), and it was comparable to the average RSEI value of the highly urbanized Taihu Lake Basin in China (Zhou and Liu, 2022). The RSEI of Hwangdo Island was primarily influenced by NDBSI and NDVI, as detailed in Supplementary Table S8. Gao et al. (2021) also reported that NDVI is a primary remote sensing index influencing RSEI in the Yellow River Basin. In February, the average RSEI value was the lowest, due to the higher NDVI and LST during this month negatively influencing it compared to other months (Supplementary Tables S5, S7). This trend aligns with findings from other studies (Ji et al., 2022). In Figure 3, lower RSEI values were concentrated on the right side of the island, which coincides with the location of most buildings on Hwangdo Island. Zhang et al. (2022) reported that buildings typically have a negative impact on RSEI. However, in August, the RSEI value was notably highest on the right side of the island. Principal component analysis revealed that NDBSI was the primary factor influencing the RSEI during this period. On the left side of the island, agricultural activities significantly increased the extent of bare soil, raising NDBSI values and subsequently lowering the RSEI. In contrast, the right side, predominantly consisting of built-up areas without farmland, was unaffected by these changes, resulting in comparatively higher RSEI values.
4.2 Macrobenthic biodiversity and benthic ecological quality on Hwangdo Island
Annelida was the phylum with the highest number of species in the mudflats of Hwangdo Island. In the mudflats of Garolim Bay and Cheonsu Bay, Annelida also represented the phylum with the highest number of species (Liang et al., 2024b, d). The dominant species, Pirenella sp., had the highest IRI value and was predominantly found in the middle tidal region. Pirenella sp. is a sediment-feeding gastropod with a wide distribution not only in the mudflat of the western coast of South Korea but also in the Red Sea, the Mediterranean, and the Indian Ocean (Zuschin and Ebner, 2015). In the RDA, Pirenella sp. was positively correlated with AVS. Orabi et al. (2018) indicated that the genus Pirenella has a higher environmental pollution tolerance than other gastropods.
TD indices are typically more sensitive to anthropogenic activities than traditional diversity indices because they consider the taxonomic differences among macrobenthos (Hu and Zhang, 2016). Two TD indices suggested that Station 9 could have been impacted by anthropogenic activities (Figure 6). Station 9 is situated near a shellfish aquaculture facility. Intertidal shellfish farming can lead to macrobenthic community simplification and organic matter deposition (Bendell-Young, 2006). Furthermore, the disturbance of sediment during shellfish collection can adversely impact the macrobenthic community. However, it is important to note that TD indices are not flawless. Some studies have shown that TD indices are more responsive to natural stressors than anthropogenic pressures. Additionally, habitat selection and the choice of taxa for calculation can affect their effectiveness (Li et al., 2020b).
Although AMBI and BPI assessed the benthic ecological quality of all stations as high or good, M-AMBI rated some stations as moderate. In contrast to AMBI and BPI, M-AMBI incorporates the diversity and abundance of macrobenthos (Muxika et al., 2007). Some studies suggest that M-AMBI outperforms univariate benthic indices. For instance, M-AMBI proved more suitable for evaluating the benthic ecological quality of the Yangtze River Estuary than both AMBI and BOPA (Yan et al., 2020).
In the correlation analysis, the three benthic indices displayed poor performance in relation to environmental factors and remote sensing indices. The ecological groups of AMBI and M-AMBI are designed around European coastal ecosystems. The same species may exhibit varied behaviors across different regions (Wu et al., 2022). In the study by Liang et al. (2024f), adapting the ecological groups to South Korean coastal ecosystems enhanced the effectiveness of both AMBI and M-AMBI. We recommend calibrating the ecological groups before employing AMBI and M-AMBI to ensure an accurate evaluation of benthic ecological quality in the study area. Concerning the BPI index, while developed based on South Korean bay ecosystems (Seo et al., 2014), its application in intertidal zones should be exercised with caution. In Liang et al. (2024d, g), BPI showed poor performance in sandy beaches and mudflats, aligning with the findings of this study.
4.3 Anthropogenic activities’ impact on macrobenthic community
In the correlation analysis, RSEI was positively correlated with J’, H’, 1-Lambda’, and M-AMBI, suggesting that terrestrial environmental ecological quality can influence the biodiversity of macrobenthos in mudflats. Anthropogenic activities significantly affected RSEI (Jiang et al., 2023). On Hwangdo Island, these activities impact not only terrestrial ecological quality but also the ecological environment of the mudflats. Only NDBSI showed a negative correlation with Lambda+, which focuses on species abundance and taxonomic distinctness (Stamou et al., 2017). In the RDA, three dominant species (excluding Ruditapes philippinarum) were positively associated with NDBSI, indicating that an increase in NDBSI leads to a rise in the abundance of these species, reducing Lambda+.
In dbRDA, the monthly variations in macrobenthic communities were primarily influenced by NDBSI. Both DistLM and BIO-ENV analyses indicated a significant impact of NDBSI on macrobenthic communities. These variations in NDBSI were mainly driven by urbanization and land reclamation (Liu et al., 2021). The farmland area on Hwangdo Island is approximately 0.3 km², accounting for about 42.2% of the total island area. The NDBSI value peaked in February and reached its lowest in August, subsequently increasing from October. This pattern aligns with the crop planting and harvesting schedule, suggesting that NDBSI is predominantly affected by changes in the bare soil area due to agriculture on Hwangdo Island.
Agricultural activities typically release large amounts of wastewater containing pesticides and fertilizers into the surrounding environment (Nagendran, 2011). When this untreated agricultural wastewater is discharged into mudflats, it detrimentally affects macrobenthic communities (Llanos et al., 2019). On Hwangdo Island, NDBSI appears to be a key factor influencing macrobenthic communities, with its seasonal variations closely aligned with periods of agricultural activity. Similarly, our previous research on the intertidal zones of Anmyeon Island highlighted those agricultural activities contributed to significant shifts in macrobenthic community structure (Liang et al., 2025). Although direct evidence linking agricultural activities to these impacts was still limited, the observed correlation between NDBSI and macrobenthic communities, together with consistent findings from these two neighboring studies sites, suggested that agricultural activities play an important role in shaping macrobenthic communities in intertidal zone ecosystems.
4.4 Recommendations for mudflat protection
While the South Korean government increasingly focuses on the protection of mudflats and has enacted policies such as banning shellfish farming and establishing mudflat protection zones (Kim, 2010), these measures predominantly address only the mudflats and do not commonly extend to adjacent environments. We recommend that the South Korean government thoroughly considers the indirect impacts of terrestrial human activities on mudflats in their policy formulation. Only by fully assessing the impacts of human activities can effective protection policies be developed.
The South Korean government has enacted four laws aimed at protecting the coastal environment: the Coastal Management Act, the Marine Environment Management Act, the Conservation and Management of Marine Ecosystems Act, and the Wetlands Conservation Act (Koh and Khim, 2014). However, in light of the growing urgency in mudflat protection, we advise that the South Korean government rapidly progresses in establishing a dedicated mudflat protection law.
5 Conclusions
In this study, we utilized five remote sensing indices (i.e.,Wetness, NDVI, LST, NDBSI, and RSEI) to evaluate terrestrial anthropogenic impacts on Hwangdo Island and conducted field surveys to examine the macrobenthos in the mudflats. Our findings suggest that macrobenthic biodiversity correlates with terrestrial ecological quality, with NDBSI being the primary factor influencing macrobenthic communities in Hwangdo Island’s mudflats. Seasonal variations in NDBSI on Hwangdo Island were influenced by changes in bare soil areas due to agricultural activities. We posit that these agricultural activities, primarily agricultural wastewater, may indirectly impact the macrobenthic communities in the island’s mudflats.
Although the South Korean government has proposed various policies to protect the mudflat environment, the conservation policies have not fully considered the impact of terrestrial human activities, and no specific laws have been enacted to protect the mudflat environment. We recommend that the South Korean government comprehensively assess the impact of anthropogenic activities on mudflats when formulating conservation policies and expedite the enactment of specific laws to protect this environment. Only in this way can South Korea’s mudflats be effectively protected and their biodiversity enhanced.
Data availability statement
The raw data supporting the conclusions of this article will be made available by the authors, without undue reservation.
Ethics statement
The manuscript presents research on animals that do not require ethical approval for their study.
Author contributions
JL: Conceptualization, Data curation, Formal Analysis, Funding acquisition, Investigation, Methodology, Project administration, Resources, Software, Supervision, Validation, Visualization, Writing – original draft, Writing – review & editing. CM: Funding acquisition, Visualization, Writing – original draft, Writing – review & editing.
Funding
The author(s) declare financial support was received for the research, authorship, and/or publication of this article. This work was supported by the Soonchunhyang University Research Fund.
Acknowledgments
We used Grammarly software during the writing process to check the grammar and improve the manuscript’s readability.
Conflict of interest
The authors declare that the research was conducted in the absence of any commercial or financial relationships that could be construed as a potential conflict of interest.
Generative AI statement
The author(s) declare that no Generative AI was used in the creation of this manuscript.
Publisher’s note
All claims expressed in this article are solely those of the authors and do not necessarily represent those of their affiliated organizations, or those of the publisher, the editors and the reviewers. Any product that may be evaluated in this article, or claim that may be made by its manufacturer, is not guaranteed or endorsed by the publisher.
Supplementary material
The Supplementary Material for this article can be found online at: https://www.frontiersin.org/articles/10.3389/fmars.2025.1533891/full#supplementary-material
References
Baldrighi E., Pizzini S., Punzo E., Santelli A., Strafella P., Scirocco T., et al. (2023). Multi-benthic size approach to unveil different environmental conditions in a Mediterranean harbor area (Ancona, Adriatic Sea, Italy). PeerJ 11, e15541. doi: 10.7717/peerj.15541
Bendell-Young L. I. (2006). Contrasting the community structure and select geochemical characteristics of three intertidal regions in relation to shellfish farming. Envir. Conserv. 33, 21–27. doi: 10.1017/S0376892906002864
Borja A., Chust G., Muxika I. (2019). Forever young: The successful story of a marine biotic index, in: Advances in Marine Biology. Elsevier pp, 93–127. doi: 10.1016/bs.amb.2019.05.001
Borja A., Franco J., Pérez V. (2000). A marine biotic index to establish the ecological quality of soft-bottom benthos within european estuarine and coastal environments. Mar. pollut. Bull. 40, 1100–1114. doi: 10.1016/S0025-326X(00)00061-8
Chen N., Cheng G., Yang J., Ding H., He S. (2023). Evaluation of urban ecological environment quality based on improved RSEI and driving factors analysis. Sustainability 15, 8464. doi: 10.3390/su15118464
Choi K.-H., Lee S.-M., Lim S.-M., Walton M., Park G.-S. (2010). Benthic habitat quality change as measured by macroinfauna community in a tidal flat on the west coast of Korea. J. Oceanogr 66, 307–317. doi: 10.1007/s10872-010-0027-7
Choi Y. R. (2014). Modernization, Development and Underdevelopment: Reclamation of Korean tidal flats 1950s–2000s. Ocean Coast. Manage. 102, 426–436. doi: 10.1016/j.ocecoaman.2014.09.023
Clarke K. R., Gorley R. N., Somerfield P. J., Warwick R. M. (2014). Changes In Marine Communities: An Approach to Statistical Analysis and Interpretation. 3rd ed. (Auckland, New Zealand: Plymouth Marine Laboratory: PRIMER-E Ltd).
Cook P., Butler E., Eyre B. (2004). Carbon and nitrogen cycling on intertidal mudflats of a temperate Australian estuary. I. Benthic Metab. Mar. Ecol. Prog. Ser. 280, 25–38. doi: 10.3354/meps280025
Du J., Shi B., Li J., Wang Y. P. (2019). Muddy coast off Jiangsu, China: physical, ecological, and anthropogenic processes, in: sediment dynamics of chinese muddy coasts and estuaries. Elsevier 3, 25–49. doi: 10.1016/B978-0-12-811977-8.00003-0
El-Naggar H. A., Salem E. S., El-Kafrawy S. B., Bashar M. A., Shaban W. M., El-Gayar E. E., et al. (2022). An integrated field data and remote sensing approach for impact assessment of human activities on epifauna macrobenthos biodiversity along the western coast of Aqaba Gulf. Ecohydrology 15, e2400. doi: 10.1002/eco.2400
Gao W., Zhang S., Rao X., Lin X., Li R. (2021). Landsat TM/OLI-based ecological and environmental quality survey of yellow river basin, inner Mongolia section. Remote Sens. 13, 4477. doi: 10.3390/rs13214477
Geng J., Yu K., Xie Z., Zhao G., Ai J., Yang L., et al. (2022). Analysis of spatiotemporal variation and drivers of ecological quality in fuzhou based on RSEI. Remote Sens. 14, 4900. doi: 10.3390/rs14194900
Hill M. O. (1973). Diversity and evenness: A unifying notation and its consequences. Ecology 54, 427–432. doi: 10.2307/1934352
Hu G., Zhang Q. (2016). Seasonal variations in macrobenthic taxonomic diversity and the application of taxonomic distinctness indices in Bohai Bay, northern China. Ecol. Indic. 71, 181–190. doi: 10.1016/j.ecolind.2016.07.001
Ji J., Tang Z., Zhang W., Liu W., Jin B., Xi X., et al. (2022). Spatiotemporal and multiscale analysis of the coupling coordination degree between economic development equality and eco-environmental quality in China from 2001 to 2020. Remote Sens. 14, 737. doi: 10.3390/rs14030737
Jiang Q., Shi Z., Liang Q., He G., Zhao L., He L. (2023). Coupling RESI with multi-scenario LULC simulation and spatiotemporal variability analysis: an ecological spatial constraint approach. Sustainability 15, 15757. doi: 10.3390/su152215757
Kim S. G. (2010). The evolution of coastal wetland policy in developed countries and Korea. Ocean Coast. Manage. 53, 562–569. doi: 10.1016/j.ocecoaman.2010.06.017
Koh C.-H., Khim J. S. (2014). The Korean tidal flat of the Yellow Sea: Physical setting, ecosystem and management. Ocean Coast. Manage. 102, 398–414. doi: 10.1016/j.ocecoaman.2014.07.008
Lebreton B., Rivaud A., Picot L., Prévost B., Barillé L., Sauzeau T., et al. (2019). From ecological relevance of the ecosystem services concept to its socio-political use. The case study of intertidal bare mudflats in the Marennes-Oléron Bay, France. Ocean Coast. Manage. 172, 41–54. doi: 10.1016/j.ocecoaman.2019.01.024
Lee J.-H., Woo H. J., Jung H.-S., Jeong J. B., Jang Y., Ryu J.-H., et al. (2023). Mean particle size, organic carbon, and porewater salinity distribution of surface sediments using a dataset from the hwangdo tidal flat, taean, western korea. J. Coast. Res. 116, 245–249. doi: 10.2112/JCR-SI116-050.1
Li Z., Liu Z., Heino J., Jiang X., Wang J., Tang T., et al. (2020). Discriminating the effects of local stressors from climatic factors and dispersal processes on multiple biodiversity dimensions of macroinvertebrate communities across subtropical drainage basins. Sci. Total Environ. 711, 134750. doi: 10.1016/j.scitotenv.2019.134750
Li J., Pei Y., Zhao S., Xiao R., Sang X., Zhang C. (2020a). A review of remote sensing for environmental monitoring in China. Remote Sens. 12, 1130. doi: 10.3390/rs12071130
Liang J., Huang H.-R., Ma C.-W., Son D.-S., Kim S.-K. (2024b). Using the heavy metal indices and benthic indices to assess the ecological quality in the tidal flats of garolim bay, South Korea. Water 16, 736. doi: 10.3390/w16050736
Liang J., Huang H.-R., Shu M.-Y., Ma C.-W. (2025). Assessing the impact of land-based anthropogenic activities on the macrobenthic community in the intertidal zones of anmyeon island, South Korea. Land 14, 62. doi: 10.3390/land14010062
Liang J., Ma C.-W., Kim K.-B. (2024a). Ecological risk assessment of heavy metals in surface sediments and their impact on macrobenthos in Asan Bay. South Korea. Front. Mar. Sci. 11. doi: 10.3389/fmars.2024.1450396
Liang J., Ma C.-W., Kim K.-B. (2024c). Comparing the environmental impacts of pollution from two types of industrial zones on the coast. Front. Mar. Sci. 11. doi: 10.3389/fmars.2024.1433536
Liang J., Ma C.-W., Kim S.-K., Park S.-H. (2024d). Assessing the benthic ecological quality in the intertidal zone of cheonsu bay, korea, using multiple biotic indices. Water 16, 272. doi: 10.3390/w16020272
Liang J., Ma C.-W., Kim K.-B., Son D.-S. (2024f). Can the ecological quality of several bays in South Korea be accurately assessed using multiple benthic biotic indices? JMSE 12, 1179. doi: 10.3390/jmse12071179
Liang J., Ma C.-W., Son D.-S. (2024e). Using the heavy metal and biotic indices to assess ecological quality in the central area of the east sea, South Korea. Water 16, 1230. doi: 10.3390/w16091230
Liang J., Shu M.-Y., Huang H.-R., Ma C.-W., Kim S.-K. (2024g). Using benthic indices to assess the ecological quality of sandy beaches and the impact of urbanisation on sandy beach ecosystems. JMSE 12, 487. doi: 10.3390/jmse12030487
Lin L., Hao Z., Post C. J., Mikhailova E. A. (2022). Monitoring ecological changes on a rapidly urbanizing island using a remote sensing-based ecological index produced time series. Remote Sens. 14, 5773. doi: 10.3390/rs14225773
Liu C., Yang M., Hou Y., Zhao Y., Xue X. (2021). Spatiotemporal evolution of island ecological quality under different urban densities: A comparative analysis of Xiamen and Kinmen Islands, southeast China. Ecol. Indic. 124, 107438. doi: 10.1016/j.ecolind.2021.107438
Liu Y., Zhou T., Yu W. (2024). Analysis of changes in ecological environment quality and influencing factors in chongqing based on a remote-sensing ecological index mode. Land 13, 227. doi: 10.3390/land13020227
Llanos E. N., Jaubet M. L., Elías R. (2019). The intertidal benthic community of SW Atlantic as an environmental indicator of 50 years of human-mediated changes. Mar. Biol. Res. 15, 458–475. doi: 10.1080/17451000.2019.1674875
Mohamamad A., Jalal K. C. A. (2018). Macrobenthic diversity and community composition in the pahang estuary, Malaysia. J. Coast. Res. 82, 206–211. doi: 10.2112/SI82-030.1
Muxika I., Borja Á., Bald J. (2007). Using historical data, expert judgement and multivariate analysis in assessing reference conditions and benthic ecological status, according to the European Water Framework Directive. Mar. pollut. Bull. 55, 16–29. doi: 10.1016/j.marpolbul.2006.05.025
Nagendran R. (2011). “Agricultural waste and pollution,” in Waste (Amsterdam, Netherlands: Elsevier), 341–355. doi: 10.1016/B978-0-12-381475-3.10024-5
National Institute of Fisheries Science (2011). Notification of marine environmental process test standards. Available online at: https://www.nifs.go.kr/board/actionBoard0052List.do?BBS_CL_CD=ALL (Accessed March 01, 2024).
Newton A., Icely J., Cristina S., Perillo G. M. E., Turner R. E., Ashan D., et al. (2020). Anthropogenic, direct pressures on coastal wetlands. Front. Ecol. Evol. 8. doi: 10.3389/fevo.2020.00144
Orabi O. H., El-Badry A.E.-M.A., Alhejoj I. (2018). Response of mollusk assemblages to environmental conditions: A case study from the burullus lagoon, Egypt. J. Geol Soc. India 91, 583–588. doi: 10.1007/s12594-018-0908-8
Pascal P.-Y., Bocher P., Lefrançois C., Nguyen H. T., Chevalier J., Dupuy C. (2019). Meiofauna versus macrofauna as a food resource in a tropical intertidal mudflat. Mar. Biol. 166, 144. doi: 10.1007/s00227-019-3588-z
Rossi F., Forster R. M., Montserrat F., Ponti M., Terlizzi A., Ysebaert T., et al. (2007). Human trampling as short-term disturbance on intertidal mudflats: effects on macrofauna biodiversity and population dynamics of bivalves. Mar. Biol. 151, 2077–2090. doi: 10.1007/s00227-007-0641-0
Rossiter T., Furey T., McCarthy T., Stengel D. B. (2020). Application of multiplatform, multispectral remote sensors for mapping intertidal macroalgae: A comparative approach. Aquat. Conserv. 30, 1595–1612. doi: 10.1002/aqc.3357
Seo J.-Y., Lim H.-S., Choi J.-W. (2014). Threshold value of Benthic Pollution Index (BPI) for a muddy healthy benthic faunal community and its application to Jinhae Bay in the southern coast of Korea. Ocean Sci. J. 49, 313–328. doi: 10.1007/s12601-014-0030-9
Shan W., Jin X., Ren J., Wang Y., Xu Z., Fan Y., et al. (2019). Ecological environment quality assessment based on remote sensing data for land consolidation. J. Cleaner Production 239, 118126. doi: 10.1016/j.jclepro.2019.118126
Stamou G., Polyzou C., Karagianni A., Michaloudi E. (2017). Taxonomic distinctness indices for discriminating patterns in freshwater rotifer assemblages. Hydrobiologia 796, 319–331. doi: 10.1007/s10750-016-2894-4
Tan K. S., Acerbi E., Lauro F. M. (2016). Marine habitats and biodiversity of Singapore’s coastal waters: A review. Regional Stud. Mar. Sci. 8, 340–352. doi: 10.1016/j.rsma.2016.01.008
Warwick R., Clarke K. (1995). New “biodiversity” measures reveal a decrease in taxonomic distinctness with increasing stress. Mar. Ecol. Prog. Ser. 129, 301–305. doi: 10.3354/meps129301
Wu H.-Y., Fu S.-F., Hu W.-J., Chen F.-G., Cai X.-Q., Chen Q.-H., et al. (2022). Response of different benthic biotic indices to eutrophication and sediment heavy metal pollution, in fujian coastal water, East China sea. Chemosphere 307, 135653. doi: 10.1016/j.chemosphere.2022.135653
Yan J., Sui J., Xu Y., Li X., Wang H., Zhang B., et al, et al. (2020). RAssessment of the benthic ecological status in adjacent areas of the Yangtze River Estuary, China, using AMBI, M-AMBI and BOPA biotic indices. Mar. Pollut. Bull 153, 111020. doi: 10.1016/j.marpolbul.2020.111020
Yang Y., Chui T. F. M. (2017). Aquatic environmental changes and ecological implications from the combined effects of sea-level rise and land reclamation in Deep Bay, Pearl River Estuary, China. Ecol. Eng. 108, 30–39. doi: 10.1016/j.ecoleng.2017.08.003
Yoon Y.-K., Lee S.-W., Yoon J.-J. (2021). Assessment of the degree of contamination in marine sediments at cheonsu bay, west coast of korea. J. Coast. Res. 114, 31–35. doi: 10.2112/JCR-SI114-007.1
Zhang J., Jiao G., Ye Q., Gu X. (2022). The impact of urban expansion on the urban thermal environment: A case study in nanchang, jiangxi, China. Sustainability 14, 16531. doi: 10.3390/su142416531
Zhang Y., She J., Long X., Zhang M. (2022a). Spatio-temporal evolution and driving factors of eco-environmental quality based on RSEI in Chang-Zhu-Tan metropolitan circle, central China. Ecol. Indic. 144, 109436. doi: 10.1016/j.ecolind.2022.109436
Zhang L., Wu B., Yin K., Li X., Kia K., Zhu L. (2015). Impacts of human activities on the evolution of estuarine wetland in the Yangtze Delta from 2000 to 2010. Environ. Earth Sci. 73, 435–447. doi: 10.1007/s12665-014-3565-2
Zhang T., Yang R., Yang Y., Li L., Chen L. (2021). Assessing the urban eco-environmental quality by the remote-sensing ecological index: application to tianjin, north China. IJGI 10, 475. doi: 10.3390/ijgi10070475
Zhao X., Cai L., Rao Y., Yang D., Zhou X., Wang D., et al. (2024). Comparison of macrozoobenthic communities and environmental variables in the subtidal zones between a nearly closed bay and an open bay. Regional Stud. Mar. Sci. 73, 103464. doi: 10.1016/j.rsma.2024.103464
Zhou J., Liu W. (2022). Monitoring and evaluation of eco-environment quality based on remote sensing-based ecological index (RSEI) in taihu lake basin, China. Sustainability 14, 5642. doi: 10.3390/su14095642
Zhu M., Li Y., Wang L., Zhang W., Niu L., Hu T. (2023). Unraveling antibiotic resistomes associated with bacterial and viral communities in intertidal mudflat aquaculture area. J. Hazardous Materials 459, 132087. doi: 10.1016/j.jhazmat.2023.132087
Keywords: macrobenthos, mudflat, anthropogenic activities, benthic biotic indices, remote sensing ecological index, South Korea
Citation: Liang J and Ma C-W (2025) Impact of anthropogenic activities on the biodiversity of macrobenthos and benthic ecological quality in the mudflats of Hwangdo Island, South Korea: field surveys and remote sensing assessments. Front. Mar. Sci. 12:1533891. doi: 10.3389/fmars.2025.1533891
Received: 25 November 2024; Accepted: 20 January 2025;
Published: 05 February 2025.
Edited by:
Liangliang Li, Beijing Institute of Technology, ChinaReviewed by:
Baoquan Li, Chinese Academy of Sciences (CAS), ChinaXinglin Du, East China Normal University, China
Copyright © 2025 Liang and Ma. This is an open-access article distributed under the terms of the Creative Commons Attribution License (CC BY). The use, distribution or reproduction in other forums is permitted, provided the original author(s) and the copyright owner(s) are credited and that the original publication in this journal is cited, in accordance with accepted academic practice. No use, distribution or reproduction is permitted which does not comply with these terms.
*Correspondence: Chae-Woo Ma, Y3dvb21hQHNjaC5hYy5rcg==