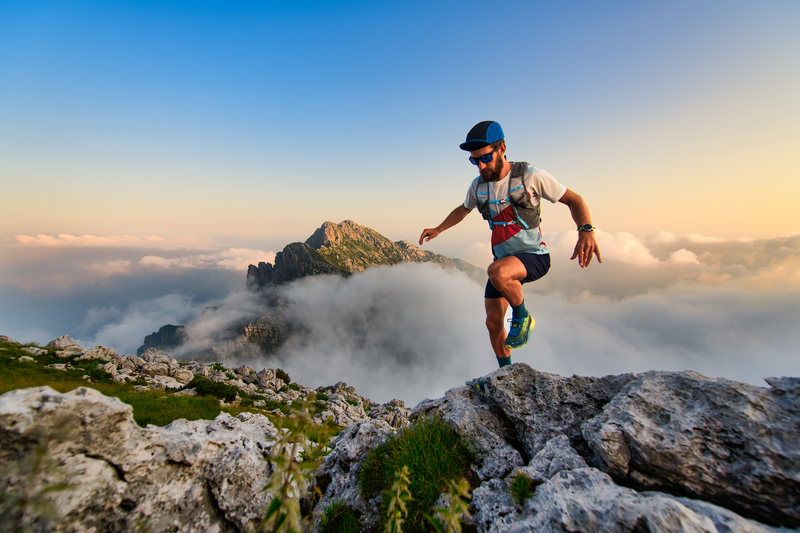
94% of researchers rate our articles as excellent or good
Learn more about the work of our research integrity team to safeguard the quality of each article we publish.
Find out more
METHODS article
Front. Mar. Sci.
Sec. Ocean Observation
Volume 12 - 2025 | doi: 10.3389/fmars.2025.1528921
This article is part of the Research Topic Remote Sensing Applications in Oceanography with Deep Learning View all 7 articles
The final, formatted version of the article will be published soon.
You have multiple emails registered with Frontiers:
Please enter your email address:
If you already have an account, please login
You don't have a Frontiers account ? You can register here
Chlorophyll-a (Chl-a) plays a vital role in assessing environmental health and understanding the response of marine ecosystems to physical factors and climate change. In situ sampling, remote sensing, and moored buoys or floats are commonly employed methods for obtaining Chl-a in marine science research. Although in situ sampling, buoys, and floats could provide accurate data, they are limited by the spatial and temporal resolution. Remote sensing offers continuous and broad spatial coverage, while it is often hindered by cloud cover in the South China Sea (SCS). This study discussed the feasibility of a predictive model by linking the physical factors (e.g., wind field, surface currents, sea surface height (SSH), and sea surface temperature (SST)) with surface Chl-a in the SCS based on the ResUnet. The ResUnet architecture performs well in capturing non-linear relationships between variables, with the model achieving a prediction accuracy exceeding 90%. The results indicate that (1) the combination of oceanic dynamical and meteorological data could effectively estimate the Chl-a based on deep learning methods; (2) the combination of meteorological and SST effectively reproduces Chl-a in the northern SCS, while adding surface currents and SSH improves model performance in the southern SCS; (3) With the addition of surface currents and SSH, the model effectively captures the high Chl-a patches induced by eddies. This research presents a viable method for estimating surface Chl-a concentrations in regions where they are highly correlated with dynamic factors, using deep learning and comprehensive oceanic and atmospheric data.
Keywords: ResUnet, chlorophyll-a, deep learning, South China Sea, Physical factors
Received: 15 Nov 2024; Accepted: 12 Feb 2025.
Copyright: © 2025 Fang, Li, Jiang, Shu and Xiu. This is an open-access article distributed under the terms of the Creative Commons Attribution License (CC BY). The use, distribution or reproduction in other forums is permitted, provided the original author(s) or licensor are credited and that the original publication in this journal is cited, in accordance with accepted academic practice. No use, distribution or reproduction is permitted which does not comply with these terms.
* Correspondence:
Ao Li, China University of Geosciences Wuhan, Wuhan, 430074, Hubei Province, China
Haoyu Jiang, College of Life Sciences and Oceanography, Shenzhen University, Shenzhen, 518060, Guangdong Province, China
Chan Shu, Huanggang Normal University, Huanggang, 438000, Hubei Province, China
Peng Xiu, Xiamen University, Xiamen, China
Disclaimer: All claims expressed in this article are solely those of the authors and do not necessarily represent those of their affiliated organizations, or those of the publisher, the editors and the reviewers. Any product that may be evaluated in this article or claim that may be made by its manufacturer is not guaranteed or endorsed by the publisher.
Research integrity at Frontiers
Learn more about the work of our research integrity team to safeguard the quality of each article we publish.