- 1Key Laboratory of Marine Genetics and Breeding, College of Marine Life Sciences/Key Laboratory of Tropical Aquatic Germplasm of Hainan Province, Sanya Oceanographic Institution, Ocean University of China, Qingdao/Sanya, China
- 2School of Computer and Artifical Intelligence, WuHan University of Technology, WuHan, Hubei, China
In Plectropomus leopardus, Vibrio disease and Hirudo parasitic disease are relatively common. Timely recognition of these diseases can improve the survival rate of Plectropomus leopardus and prevent their spread. However, early-stage diseases are difficult to distinguish due to their small size and subtle characteristics. Traditional manual recognition methods rely on personal experience and subjective judgment, leading to time-consuming and error-prone diagnoses. To address the challenges in detecting and classifying Plectropomus leopardus diseases, this paper proposes PLDNet (Plectropomus Leopardus Disease Detection Network), a real-time detection and recognition method that provides faster and more accurate diagnoses for fish farms. PLDNet incorporates two significant advancements: First, it employs FocalModulation, which enhances the model’s ability to identify key disease characteristics in images. Second, it introduces the MPDIoU (Minimum Point Distance-based Intersection over Union) for bounding box similarity comparison, optimizing the loss function and improving recognition accuracy. This paper also presents the PLDD (Plectropomus Leopardus Disease Dataset), a newly developed dataset that includes comprehensive images of healthy and diseased specimens. PLDD addresses the scarcity of data for this species and serves as a valuable resource for advancing research in marine fish health. Empirical validation of PLDNet was conducted using the PLDD dataset and benchmarked against leading models, including YOLOv8-n, YOLOv9-m, and YOLOv9-c. The results show that PLDNet achieves superior detection performance, with an average detection accuracy of 84.5%, a recall rate of 86.6%, an mAP@o.5 of 88.1%, and a real-time inference speed of 45 FPS. These metrics demonstrate that PLDNet significantly outperforms other models in both accuracy and efficiency, providing practical solutions for real-time fish disease management.
1 Introduction
Plectropomus leopardus, a marine fish of high economic value, is prized for its ornamental qualities and high nutritional value, making it a favorite in the market Khasanah et al. (2019). However, high-density facility-based culture makes Plectropomus leopardus susceptible to diseases, significantly affecting culture efficiency and turning it into a high-risk, high-return industry Li et al. (2024). In particular, Vibrio disease and Hirudo parasitic disease have caused substantial economic losses for aquaculture operations Gai et al. (2022). Outbreaks of these diseases can lead to high mortality rates and reduced market value of infected fish, compounding financial losses for farmers. To mitigate these impacts, targeted detection of Plectropomus leopardus diseases is crucial to reduce illness and mortality Duarte (2014).
Traditional methods for disease detection in Plectropomus leopardus include manual visual observation and rapid pathogen detection kits. Manual observation is inefficient and highly subjective, often resulting in missed detections and limited accuracy. This approach requires significant labor and frequently leads to irregular data recording, which delays and impairs the accuracy of diagnoses. Although rapid pathogen detection kits provide a more convenient method for detecting aquatic diseases, they often lack the necessary sensitivity and specificity, resulting in potential false positives or false negatives. Additionally, these kits require tissue sampling from diseased specimens, which adds complexity and increases the time required for testing. Therefore, precise identification and accurate localization of diseases in Plectropomus leopardus remain critical challenges in aquaculture.
In recent years, fish disease detection methods have evolved significantly, from traditional image processing techniques to more advanced deep learning models, particularly convolutional neural networks (CNNs). These advancements have significantly enhanced the accuracy and efficiency of disease diagnosis. Despite these developments, challenges remain in achieving both high detection accuracy and real-time performance, especially in aquaculture settings where rapid response is critical. The traditional methods, although effective, struggle with processing large datasets quickly enough for timely intervention. To overcome these challenges, this study leverages deep learning-based methods, focusing on optimizing both the accuracy and speed of disease recognition in Plectropomus leopardus.
Detecting diseases in Plectropomus leopardus presents notable challenges, primarily due to the absence of comprehensive datasets tailored to its diseases. Current technologies are inefficient, and the application of deep learning methodologies remains unexplored in disease detection for this species. To address these issues, we have developed the PLDD (Plectropomus Leopardus Disease Detection dataset), filling the existing gap in data availability. This dataset is specifically designed to improve model training and evaluation, directly resolving the limitations imposed by the lack of data. Furthermore, we propose a new method called PLDNet (Plectropomus leopardus Disease Detection Network), to enable real-time disease identification and classification. PLDNet leverages this dataset to offer improved diagnostic capabilities, addressing both the data scarcity and the inefficiencies of existing technologies.
The main contributions of this paper are as follows:
● A comprehensive dataset PLDD is provided, which includes images of both healthy and diseased samples collected from various specimens. This dataset can be used for training and evaluating disease detection models in Plectropomus leopardus, addressing the current lack of available data.
● A new model, PLDNet, is proposed for disease detection and recognition in Plectropomus leopardus. This model introduces two significant advancements. First, it employs FocalModulation, significantly enhancing the model’s ability to identify key disease characteristics in images, particularly for small targets like Hirudo parasitic disease. Second, it incorporates the the MPDIoU (Minimum Point Distance-based Intersection over Union) for bounding box similarity comparison, optimizing the loss function and thereby improving the detection model’s accuracy.
● We implemented the YOLOv8-n, YOLOv9-m, and YOLOv9-c models and compared them against our proposed PLDNet. Our method outperforms the other object detection approaches in both accuracy and speed on the same dataset.
The organization of this paper is as follows: Section 2 covers the related work; Section 3 discusses the materials and methods used in this study; Section 4 presents the results and discussion; Section 5 provides the conclusions and suggests directions for future work.
2 Related work
2.1 Vibrio disease
Vibrio disease is a class of bacterial infections caused by various species of the genus Vibrio, which naturally occur in aquatic environments. These diseases are particularly problematic in fish species such as Plectropomus leopardus, where they can manifest as septicemia, leading to rapid decline in health and high mortality rates Austin and Austin (2016).
The pathogenicity of Vibrio species is linked to their ability to produce toxins and invade the host’s immune system, making early detection crucial for disease management Colquhoun and Sørum (2001). Historically, detecting vibriosis in fish has relied on traditional methods such as direct microscopic examination, cultural isolation on selective media, and biochemical tests. These methods, while valuable for confirming the presence of Vibrio, are labor-intensive, time-consuming, and often require a high degree of expertise Bowker et al. (2011).
One of the primary challenges in detecting Vibrio disease is their asymptomatic nature during the early stages. The subtle clinical signs and the small size of the affected areas make it difficult for traditional methods to accurately diagnose the disease in its initial phases Defoirdt et al. (2011). In response to these challenges, there has been a push towards developing more sophisticated diagnostic tools. Recent advancements include the use of molecular techniques such as PCR, which offers increased sensitivity and specificity in detecting Vibrio species Panicker et al. (2004). Additionally, the integration of immunological methods like enzyme-linked immunosorbent assays (ELISA) has provided another layer of detection capability Li et al. (2010).
Despite these advancements, current detection methods face significant limitations, especially regarding their suitability for real-time, in-field diagnostics. The reliance on specialized equipment and reagents often makes these methods impractical for immediate, on-site use, leading to delays in treatment and management Defoirdt et al. (2011). These challenges underscore the need for innovative diagnostic solutions that provide rapid, accurate, and user-friendly detection of Vibrio diseases in aquaculture. PLDNet addresses these issues by leveraging cutting-edge computer vision techniques to enable real-time detection at the point of care.
2.2 Hirudo parasitic disease
Hirudo parasitic disease is a significant issue in aquaculture, causing substantial economic losses and health challenges for fish populations. This parasitic condition is primarily characterized by the presence of leeches that attach to the host, leading to physical damage, stress, and secondary infections. The complexity of managing this disease is compounded by the life cycle of the parasites and their resilience to conventional treatments.
Cruz-Lacierda et al. Pérez (2009) explored various parasitic diseases affecting fish and shrimp culture, noting the substantial impact of parasites like Hirudo on aquaculture productivity. The study highlighted the difficulty of controlling these parasites due to their complex life cycles and the limited effectiveness of single-measure treatments. Integrated management approaches, combining knowledge of parasite biology and effective treatment methods, were emphasized as crucial for disease control in aquaculture settings.
The life cycle of the parasites and their resilience to conventional treatments add to the complexity of managing this disease. Research indicates that the attachment of leeches can cause severe pathological changes in fish, including tissue damage and immunological responses. For example, Woo and Bruno Buchmann (2015) reported that Hirudo parasitic behavior leads to extensive tissue damage and immunosuppression in the host, increasing susceptibility to secondary infections. These pathological changes can significantly impair fish health and growth, leading to reduced aquaculture productivity.
Effective management strategies often require a combination of improved aquaculture practices, the development of resistant fish strains, and innovative treatment methods. According to Schlotfeldt and Alderman Lieke et al. (2020), incorporating improved water quality management, regular monitoring, and the use of biological control agents can enhance disease control. Additionally, the development of fish strains with genetic resistance to parasitic infections has shown promise in reducing the incidence of Hirudo parasitic disease.
Recent advancements in diagnostic technologies have also contributed to better management of Hirudo parasitic disease. For instance, molecular diagnostic tools, such as PCR-based assays, have been developed to detect parasitic DNA in fish tissues, allowing for early and accurate diagnosis Keeling et al. (2013). Early detection is critical for implementing timely and effective treatment strategies, minimizing the impact of the disease on aquaculture operations.
In summary, the effective management of Hirudo parasitic disease in aquaculture requires a multifaceted approach that includes improved aquaculture practices, genetic resistance, innovative treatment methods, and advanced diagnostic technologies. These strategies collectively address the complex challenges posed by the life cycle and resilience of Hirudo parasites, ultimately enhancing the health and productivity of aquaculture systems. The PLDNet approach is proposed to enhance the detection and classification of this challenging Hirudo parasitic disease, ultimately improving the health and productivity of aquaculture systems.
2.3 The detection of fish diseases
Traditional expert system detection methods rely on the experience and knowledge of experts who diagnose diseases by dissecting and analyzing fish samples. This approach is not only time-consuming and labor-intensive but also requires highly specialized skills Wagner (2017). To address these issues, researchers have developed various fish disease detection methods based on image processing and computer vision technologies.
Fish disease detection methods can be broadly categorized as follows. Camera image detection uses standard cameras to capture images of the fish’s surface and detect abnormalities through image processing techniques. This method is non-invasive and relatively easy to operate, making it suitable for large-scale field applications. Microscope image detection, on the other hand, uses high-resolution microscope images to detect minute lesions, making it more appropriate for laboratory environments due to its complexity. Spectral image detection and fluorescence image detection employ spectral characteristics and fluorescent markers, respectively, to provide detailed detection information, though these methods require more advanced and expensive instrumentation. Ultrasound image detection and sensor-based methods enable rapid, real-time fish disease detection through non-destructive testing. However, these methods require sophisticated equipment and techniques.
In addition to these traditional techniques, recent advancements in deep learning have revolutionized fish disease detection. Convolutional neural networks (CNNs) have been applied to classify and detect fish diseases with higher accuracy and efficiency. For example, Shaveta Malik et al. Malik et al. (2017) applied image processing techniques and machine learning algorithms to identify diseased fish, achieving high-accuracy classification through PCA-based dimensionality reduction. Md. Jueal Mia et al. Mia et al. (2022) proposed an automated fish disease recognition method combining computer vision with expert systems, achieving an accuracy of 88.87% with a Random Forest classifier. Noraini Hasan et al. Hasan et al. (2022) used a multi-layer CNN architecture for fish disease classification, achieving an impressive accuracy of 94.44%, demonstrating the power of deep learning in fish disease recognition.
Recent advancements in biological detection for aquaculture have also contributed significantly to the development of fish disease detection systems. For example, Xinyu Xie et al. Xie et al. (2021) applied Mask Scoring R-CNN to intelligently detect mango disease spores, providing valuable insights into the adaptation of deep learning architectures, such as Mask R-CNN, for disease recognition in various biological contexts, including aquatic species. Similarly, Bing Han et al. Han et al. (2022) introduced Mask_LaC R-CNN to measure the morphological features of fish, a technique that can be directly adapted for detecting diseases by analyzing subtle morphological changes in fish caused by infections. Furthermore, Longqin Gong et al. Gong et al. (2022) explored a semi-supervised, attention-based method for underwater fish tracking, which plays a vital role in real-time disease detection in natural aquatic environments. Their approach emphasizes the potential of combining attention mechanisms with deep learning to enhance the accuracy and robustness of fish disease detection systems, particularly in dynamic and complex aquaculture settings.
Despite these advancements, the performance of existing methods remains limited, particularly when it comes to real-time detection and handling small or subtle disease markers. These methods typically fail to achieve the speed required for timely diagnosis, which is crucial in aquaculture settings. To address these issues, the introduction of PLDNet represents a significant step forward. PLDNet integrates advanced deep learning techniques, including FocalModulation and MPDIoU, which enhance the model’s ability to detect and localize disease features quickly and accurately. PLDNet not only improves detection speed but also enhances the precision of detecting small and subtle disease markers that traditional methods may overlook, making it a valuable tool for real-time aquaculture disease management.
2.4 FocalModulation and MPDIoU in object detection
Recent advancements in object detection have introduced various techniques to optimize both the accuracy and speed of deep learning models Wu et al. (2020). FocalModulation is one such innovation designed to improve feature extraction across multiple spatial scales. It has been widely applied to enhance model performance, particularly in tasks requiring the identification of small or subtle features Yang et al. (2022). FocalModulation allows the model to focus more on the critical regions of an image, which is crucial for detecting small disease markers in Plectropomus leopardus, such as the attachment sites in Hirudo parasitic disease and early lesions in Vibrio disease.
MPDIoU is another advanced technique that improves the precision of bounding box localization. Traditional Intersection over Union (IoU) metrics can struggle to accurately localize small objects or subtle features. MPDIoU addresses this by focusing on the minimum point distance between predicted and ground truth boxes, which enhances detection performance by ensuring more accurate localization of disease markers Siliang and Yong (2022).
These methods, FocalModulation and MPDIoU, have been integrated into the PLDNet architecture to address the challenges of accurate disease detection and localization in Plectropomus leopardus. While traditional object detection methods struggle with small or subtle disease features, PLDNet leverages these techniques to improve both the speed and accuracy of disease detection in real-time, making it a valuable tool for aquaculture.
3 Materials and methods
3.1 PLDD
3.1.1 Data collection
The dataset used in this study was meticulously curated to facilitate comprehensive research on diseases affecting Plectropomus leopardus. Images were collected from various aquaculture facilities specializing in the breeding of Plectropomus leopardus. The collection process focused on capturing both healthy specimens and those exhibiting symptoms of common diseases, such as Vibrio disease and Hirudo parasitic disease, as shown in Figure 1. The dataset includes a total of 1,041 images, classified into three categories: healthy Plectropomus leopardus, Hirudo parasitic disease, and Vibrio disease. Each image was meticulously annotated by experienced veterinarians and marine biologists to ensure accurate documentation of the disease status.
3.1.2 Dataset features
The dataset comprises various high-resolution images that capture the subtle details crucial for disease detection in Plectropomus leopardus. It includes a spectrum of disease severities and stages, providing a comprehensive basis for robust model training and evaluation. Detailed annotations accompany each image, offering precise localization of disease symptoms and facilitating in-depth analysis and model learning.
3.1.3 Data preprocessing
Training a robust model typically requires a substantial amount of annotated data, which is challenging to collect, especially for complex actions that are time-consuming and labor-intensive to annotate Liu et al. (2018); Meng et al. (2018). Before commencing model training, the dataset underwent rigorous preprocessing steps to enhance the input images’ variability and improve the training model’s robustness, thereby optimizing model performance and generalization capabilities. This preprocessing included techniques such as random cropping, rotation, flipping, saturation adjustment, and adding noise to augment the dataset. These augmentations increased the variability and improved the model’s robustness under different viewing angles and environmental conditions. Figure 2 illustrates the processed images. Through these data augmentation methods, the original dataset of 429 images was expanded to 1,041 images. Additionally, pixel values of the images were normalized to a standard range to ensure consistent training conditions across the dataset. The dataset was then divided into distinct training, validation, and test sets to facilitate effective model parameter learning, hyperparameter tuning, and final model evaluation.
3.2 PLDNet
3.2.1 The framework of PLDNet
As illustrated in Figure 3, PLDNet (Plectropomus leopardus Disease Detection Network) is a convolutional neural network specifically designed for the real-time detection and classification of diseases in Plectropomus leopardus. The architecture of PLDNet integrates several advanced components to enhance detection accuracy and efficiency.
The network begins with convolutional layers that perform initial feature extraction from input images. These layers capture essential low-level features such as edges, textures, and simple patterns, which form the foundation for identifying disease characteristics.
Following the initial convolutional stages, the network incorporates the ResNCSPELAN4 blocks, which are designed to deepen the network’s capacity to learn complex and hierarchical features. The ResNCSPELAN4 blocks enable the model to effectively capture intricate patterns and subtle disease markers, which are crucial for accurate detection in medical imaging.
To handle multi-scale features, PLDNet integrates the FocalModulation technique. This technique refines the network’s ability to focus on important regions within the images, enhancing its sensitivity to small and subtle disease features. FocalModulation plays a pivotal role in the feature extraction process of PLDNet by focusing attention on critical regions of the input image. This mechanism enables the network to prioritize the most relevant disease-related features while downplaying irrelevant background information. Within this process, the FocalModulation module dynamically adjusts attention across the feature maps, selectively highlighting regions that exhibit subtle disease characteristics. This targeted enhancement allows PLDNet to improve the detection of small-scale or less obvious symptoms, which might otherwise be overshadowed by the surrounding healthy tissue. By effectively enhancing these important areas, FocalModulation significantly improves the network’s ability to capture and classify complex disease patterns.
An upsampling operation is applied after the FocalModulation layer, followed by a concatenation with the corresponding feature maps from earlier layers. This fusion enhances the model’s capacity to aggregate contextual information across different resolutions, further improving its accuracy in detecting disease features. Multiple ResNCSPELAN4 blocks are again used in this stage to refine the features before the final classification.
PLDNet introduces the the MPDIoU as a similarity measure for bounding box predictions, significantly improving the precision and accuracy of disease localization.
The PLDNet framework also includes an auxiliary branch that aids in training by providing additional gradient signals, helping the model to converge faster and more effectively. The output from this auxiliary branch is integrated with the main output to produce the final predictions.
Overall, the advanced components within PLDNet, including the ResNCSPELAN4 blocks, FocalModulation, and the MPDIoU loss function, work together to create a robust and efficient network capable of detecting even the most challenging disease features in Plectropomus leopardus. This design ensures that PLDNet is a valuable tool for aquaculture, enabling precise and real-time disease monitoring.
3.2.2 FocalModulation
One of the key innovations in PLDNet is the integration of FocalModulation, which significantly enhances the network’s ability to detect disease features across multiple scales. By dynamically adjusting its focus, the FocalModulation module enables PLDNet to capture and pool features from both fine-grained local contexts and broader spatial regions Yang et al. (2022). This ensures that even the smallest and most subtle disease markers are effectively identified, thereby increasing the model’s adaptability to diverse object sizes and shapes, and enhancing its robustness.
FocalModulation is a key component that enhances the network’s focus on crucial regions within an image. This enhancement involves three main processes: focal contextualization, gated aggregation, and an element-wise affine transformation.
3.2.2.1 Focal contextualization
This process encodes visual contexts from different spatial ranges—short, medium, and long. Given an input feature map X, it is first projected into a new feature space as . Subsequently, L depth-wise convolutional layers extract contextual information hierarchically:
where is the contextualization function at the ℓ-th level, this hierarchical contextualization allows the network to capture context at different granularity levels, enhancing its ability to perceive fine details.
3.2.2.2 Gated aggregation
After encoding the visual contexts, the network selects gated aggregation to combine these contexts into a modulator selectively. This is achieved by first obtaining spatial and level-aware weights , where is a lightweight linear function responsible for calculating the gating weights. The final output is then computed by performing a weighted sum through element-wise multiplication to obtain a single feature map of the same size as the input X:
Here, represents the channel for level ℓ.
3.2.2.3 Element-wise affine transformation
Finally, the modulator obtained through gated aggregation, , is applied to the query token via an element-wise affine transformation:
In this equation, The query token is learned during training to emphasize disease-related regions, enhancing detection accuracy for subtle markers and suppressing irrelevant background areas, denotes element-wise multiplication, is a linear layer function modeling the relationships between different channels, where and are the gating value and visual feature at location ℓ of and respectively, The final output, , is the enhanced feature map that reflects the combination of the query token and the aggregated features, ensuring more accurate disease detection
The FocalModulation formula can be expressed as (3). FocalModulation, as shown in Figure 3, significantly boosts the model’s ability to discern fine details, which is crucial for early-stage disease detection. This comprehensive approach ensures that PLDNet can effectively manage the varying scales and complexities of disease features in Plectropomus leopardus, providing a more accurate and reliable diagnosis.
3.2.3 MPDIoU
Another key innovation in PLDNet is the use of the MPDIoU (Minimum Point Distance-based Intersection over Union) for bounding box similarity comparison. Traditional IoU metrics often fail to capture the precise alignment required for small objects and subtle features. MPDIoU addresses this by focusing on the minimum point distance between predicted and ground truth boxes, providing a more accurate measure of bounding box overlap Siliang and Yong (2022). This novel approach optimizes the loss function during training, leading to improved localization and classification performance. The use of MPDIoU ensures that the network can more precisely identify and delineate disease-affected regions in the images.
MPDIoU is calculated by minimizing the distance between the corresponding points of the predicted bounding box and the ground truth bounding box. The coordinates of the top-left and bottom-right points define a unique rectangle, and the distances between these points are minimized. The calculation is as follows:
● Define the coordinates of the top-left and bottom-right points of the ground truth box and the predicted box .
● Compute the squared distances between the corresponding points:
● Normalize these distances by the width and height of the bounding boxes:
where and denote the areas of the ground truth and predicted bounding boxes, respectively.
The loss function based on MPDIoU can be defined as:
This loss function is minimized during training to ensure that the predicted bounding boxes closely match the ground truth boxes, taking into account both the overlap area and the distances between corresponding points. By incorporating these factors, MPDIoU provides a more comprehensive measure of bounding box similarity, improving the model’s performance in localizing and classifying objects, especially in cases involving small and subtle features.
4 Results and discussion
4.1 Experimental setup
To evaluate the performance of the proposed PLDNet in detecting and classifying diseases in Plectropomus leopardus, a series of experiments were conducted. The experimental setup is divided into four main components: Hardware Configuration, Software Environment, Dataset, Training Procedure:
Hardware Configuration: All experiments were conducted on a system equipped with an NVIDIA RTX 4060Ti GPU, 32GB RAM, and an Intel i5-13400F CPU.
Software Environment: The model was implemented using Python 3.11 and torch 3.1. The training and testing processes were managed using the PyTorch framework, and data preprocessing was performed with OpenCV.
Dataset: The dataset comprises 1041 annotated images of Plectropomus leopardus, including both healthy specimens and those affected by various diseases such as Vibrio disease and Hirudo parasitic disease. The dataset was split into 70% training, 20% validation, and 10% testing sets. Data augmentation techniques such as rotation, scaling, and flipping were applied to increase the variability of the training set.
Training Procedure: The network was trained for 400 epochs using the Adam optimizer with an initial learning rate of 0.001. The learning rate was reduced by a factor of 0.1 every 30 epochs to facilitate fine-tuning. A batch size of 16 was used, and early stopping was implemented to prevent overfitting, with validation loss monitored and a patience of 10 epochs before halting training.
4.2 Metrics for evaluating performance
The performance of PLDNet was evaluated using several standard metrics, including Accuracy, Precision, Recall, F1-Score, mAP and FPS to ensure a comprehensive assessment:
Accuracy: Measures the proportion of correctly identified instances among the total instances.
where is the number of true positives, is the number of true negatives, is the number of false positives, and is the number of false negatives.
Precision and Recall: Precision measures the proportion of true positive detections among all positive detections, while Recall measures the proportion of true positive detections among all actual positives.
These metrics help in understanding the trade-off between the model’s ability to identify true disease cases and its tendency to generate false positives.
F1-Score: The harmonic mean of precision and recall, providing a single metric to balance the trade-off between precision and recall.
mAP (mean Average Precision): Calculated as the average of the precision values at different recall levels, mAP is used to summarize the precision-recall curve in a single value.
where N is the number of classes, and is the average precision for class .
FPS (Frames Per Second): Measures the number of frames the model can process per second, reflecting its real-time processing speed. A higher FPS indicates better model performance for real-time applications.
where “Total Time Taken (ms)” is the total time taken to process the frames in milliseconds.
4.3 Model training
PLDNet underwent a pretraining phase to accelerate its ability to recognize and classify diseases in Plectropomus leopardus. This phase leveraged the PLDD, enabling the model to learn essential feature representations, such as texture changes and patterns linked to different disease states. By identifying key features early, PLDNet enhanced its generalization capabilities, ensuring robust performance across various disease types and environmental variations.
For the final training phase, the complete PLDD, which includes conditions such as Vibrio disease and Hirudo parasitic disease, was used. This thorough training process contributed to PLDNet’s superior performance. In contrast, the comparison models did not undergo pretraining, focusing solely on the full training phase with the same dataset.
During training, the model’s performance was compared against three baseline models: YOLOv8-n, YOLOv9-m, and YOLOv9-c. These models were selected due to their proven efficacy in object detection tasks, serving as benchmarks to evaluate the effectiveness of PLDNet.
As shown in Figure 4, PLDNet consistently maintains lower loss values throughout the entire training process compared to the baseline models, such as YOLOv8-n, YOLOv9-m, and YOLOv9-c. This indicates that PLDNet benefits from a more stable and efficient learning process over time. The smooth and continuous reduction in loss demonstrates the network’s ability to refine its predictions effectively, even as training progresses. While all models display a general downward trend in loss, PLDNet’s significantly lower loss values across epochs emphasize its superior performance in detecting small-scale and complex disease features, such as Hirudo parasitic disease. This reflects its robustness and enhanced capability in handling subtle and intricate disease characteristics compared to the baseline models.
The chart clearly shows that PLDNet outperforms the YOLOv8-n, YOLOv9-m, and YOLOv9-c models. The initial sharp decrease in loss for PLDNet, followed by a smooth and gradual decline, reflects its superior ability to learn complex patterns within the dataset. Notably, while the loss for YOLOv9-c stabilizes at a higher value, PLDNet continues to reduce loss, demonstrating its robustness in identifying small-scale features such as Hirudo parasitic disease.
In our experiments, we observed that using the MPDIoU loss function resulted in faster convergence and improved accuracy compared to traditional IoU-based loss functions. This enhancement was especially prominent in challenging scenarios requiring precise localization, where small and subtle disease features need to be detected accurately.
4.4 Ablation study
To further evaluate the effectiveness of the key innovations introduced in PLDNet, we conducted an ablation study to assess the individual contributions of the FocalModulation and the MPDIoU. The goal of this study was to isolate the impact of each of these components on the overall performance of the disease detection model.
In this experiment, we created three variations of the PLDNet:
● PLDNet (without FocalModulation): This model was trained without the FocalModulation mechanism, keeping the rest of the architecture unchanged. The goal was to assess how the absence of FocalModulation impacts the model’s ability to focus on subtle disease features.
● PLDNet (without MPDIoU loss): In this version, we removed the MPDIoU loss function and replaced it with a traditional Intersection over Union (IoU) loss. This allowed us to evaluate the contribution of MPDIoU in improving localization accuracy, particularly for closely spaced or partially occluded disease features.
● PLDNet (full version): This model includes both the FocalModulation mechanism and MPDIoU loss, representing the complete PLDNet architecture.
The results of the ablation study are summarized in Table 1. The evaluation metrics used include Precision, Recall, mAP@0.5, and mAP@0.5-0.95.
The results of the ablation study indicate that both FocalModulation and MPDIoU loss are crucial for enhancing the performance of PLDNet. Removing the FocalModulation mechanism resulted in a slight decrease in both recall and mAP values, highlighting the importance of this component in improving the model’s ability to detect subtle disease features. Similarly, the exclusion of MPDIoU loss led to a small decrease in the mAP@0.5-0.95 score, suggesting that MPDIoU is particularly beneficial for improving localization accuracy in challenging cases with closely spaced or partially occluded disease features.
These findings demonstrate that both the FocalModulation mechanism and the MPDIoU loss function significantly contribute to the performance of PLDNet in detecting and localizing disease features.
4.5 Detection performance
The detection performance of the proposed PLDNet model was thoroughly evaluated and compared against three established models: YOLOv8-n, YOLOv9-c, and YOLOv9-m. The evaluation metrics considered include Precision, Recall, mAP@0.5, mAP@0.5-0.95 and FPS which are summarized in Table 2.
As indicated in Table 2, PLDNet outperforms the baseline models in several key metrics. Notably, it achieved the highest mAP@0.5and mAP@0.5-0.95 scores of 0.881 and 0.653, respectively, demonstrating superior accuracy in detecting and localizing disease features in Plectropomus leopardus images. While YOLOv9-m showed slightly higher Precision, PLDNet achieved a balanced performance across all metrics, including a FPS of 37.04, making it a more reliable choice for real-time disease detection in aquaculture.
The PR (Precision-Recall) curves, depicted in Figure 5, further illustrate the comparative performance of these models. The PR curve of PLDNet consistently shows higher values across various recall thresholds, particularly in the lower recall range. This indicates that PLDNet maintains high precision even when a broader set of potential disease instances is identified, a key advantage in minimizing false positives while ensuring comprehensive detection.
Additionally, the F1 curve analysis, shown in Figure 6, reveals that PLDNet maintains superior F1 scores across varying confidence thresholds compared to the other models. The F1 score, which balances precision and recall, is a critical metric for evaluating the overall effectiveness of the detection model. The consistency of PLDNet’s F1 score, particularly in the mid to high-confidence range, underscores its robustness in making accurate predictions without sacrificing recall. This balance is vital for real-time applications, where both accuracy and speed are necessary to ensure timely disease detection and intervention in aquaculture environments.
The detection results of the four models—YOLOv8-n, YOLOv9-m, YOLOv9-c, and PLDNet are visually compared in Figure 7. This figure illustrates the models’ abilities to identify and label disease features in Plectropomus leopardus. Notably, YOLOv8-n tends to over-label certain areas, resulting in multiple detections of the same disease instance, as seen with the repeated detection of Vibrio disease. In contrast, both YOLOv9-m and YOLOv9-c demonstrate more restrained detection outputs but occasionally miss subtle disease features, potentially compromising comprehensive disease monitoring.
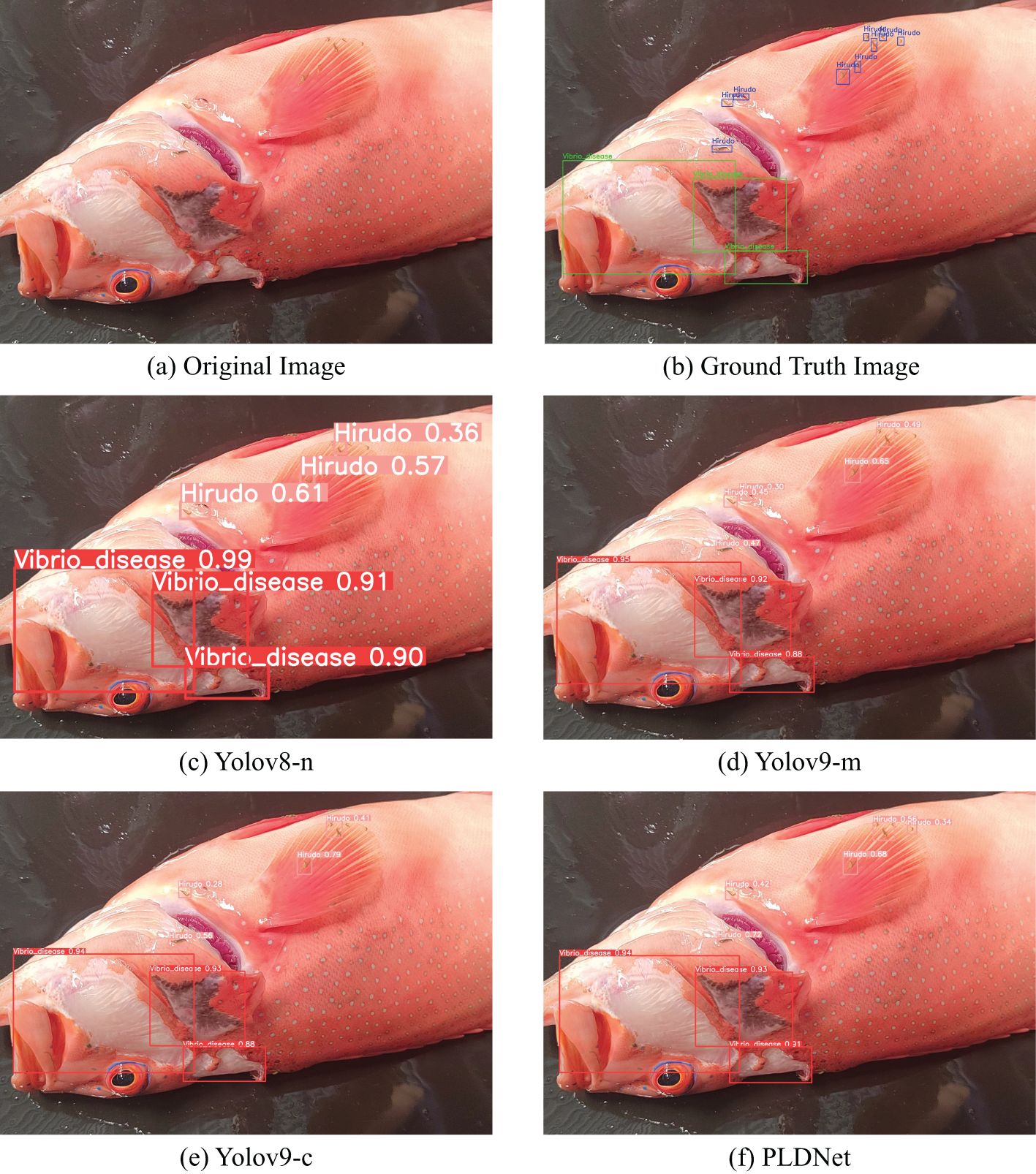
Figure 7. Model Detection Results (A) Original Image; (B) Ground Truth Image; (C–F) Detection results of different models.
PLDNet, however, strikes a balanced approach. It offers a precise and consistent identification of disease features, successfully capturing both Hirudo and Vibrio disease with fewer false positives compared to YOLOv8-n. The improved detection accuracy is evident in its ability to distinguish between closely related disease instances, reflecting its superior feature learning capabilities. This visual analysis aligns with the quantitative results shown in Table 2, where PLDNet achieves higher mAP scores and recall, underscoring its reliability in real-time disease detection in aquaculture environments.
In conclusion, the experimental results clearly indicate that PLDNet provides a substantial improvement in disease detection performance for Plectropomus leopardus. The model’s superior precision, recall, and mAP scores, combined with its efficient learning capabilities, make it a valuable tool for real-time disease monitoring in aquaculture.
4.6 Discussion
The experimental results presented in this study demonstrate that the proposed PLNet model significantly outperforms existing models in detecting diseases in Plectropomus leopardus. The superior performance of PLDNet can be attributed to several key innovations introduced in this research. Notably, the integration of FocalModulation effectively enhances the model’s ability to capture multi-scale features, which is crucial for identifying both small and subtle disease manifestations. Additionally, introducing the MPDIoU metric further improves the model’s accuracy, particularly in challenging scenarios where disease features are closely spaced or partially obscured.
The improved detection accuracy and efficiency of PLDNet hold significant implications for the aquaculture industry. Early and precise detection of diseases is paramount in reducing mortality rates and minimizing economic losses, making this model a valuable tool for real-time disease monitoring Pires et al. (2021). By enabling timely intervention, PLDNet can contribute to healthier fish populations and more sustainable aquaculture practices.
When compared to existing methodologies, such as those proposed by Shaveta Malik et al. and Md. Jueal Mia et al., PLDNet demonstrates clear advantages in both speed and accuracy. Previous approaches have often struggled with the trade-off between these two aspects, particularly in real-time applications. PLDNet addresses these limitations through its advanced architectural design and innovative metrics, providing a more robust and accurate solution for the detection of diseases in Plectropomus leopardus. This study not only validates the effectiveness of the proposed model but also sets a new benchmark for future research in the domain of aquaculture disease detection.
5 Conclusion
In this study, we present PLDNet, a novel convolutional neural network for real-time detection and classification of diseases in Plectropomus leopardus. Utilizing techniques like FocalModulation and MPDIoU, PLDNet outperforms existing models (YOLOv8-n, YOLOv9-m, YOLOv9-c) in key metrics, achieving the highest mean Average Precision (mAP) scores and demonstrating superior precision and recall. This model enhances early disease detection, crucial for reducing mortality and economic losses in aquaculture. Future work could involve expanding the dataset and refining the model for even greater accuracy. Overall, PLDNet marks a significant advancement in fish disease monitoring, offering a valuable tool for sustainable aquaculture practices.
Data availability statement
The raw data supporting the conclusions of this article will be made available by the authors, without undue reservation. The source code for this project is available on GitHub via the following link: https://github.com/MRxue-0418/PLDNet-REAL-TIME. For cloning the repository, use the following command in your terminal: git clone https://github.com/MRxue-0418/PLDNet-REAL-TIME.git.
Ethics statement
The animal study was approved by Institutional Animal Care and Use Committee. The study was conducted in accordance with the local legislation and institutional requirements.
Author contributions
ML: Data curation, Resources, Writing – review & editing, Writing – original draft, Investigation, Methodology. RX: Writing – review & editing, Conceptualization, Investigation, Methodology, Writing – original draft, Formal analysis. CW: Writing – review & editing, Formal analysis, Resources. JH: Writing – review & editing, Supervision, Validation. ZB: Writing – review & editing, Funding acquisition, Supervision. GX: Writing – review & editing, Conceptualization. JZ: Writing – review & editing, Investigation, Methodology, Project administration.
Funding
The author(s) declare financial support was received for the research, authorship, and/or publication of this article. This study was supported by the Key Research and Development Project of Hainan Province (ZDYF2023XDNY176), Project of Hainan Seed Industry Laboratory Foundation(B23H10004), Project of Sanya Yazhouwan Science and Technology City Management Foundation(SKJC-2023-01-004).
Conflict of interest
The authors declare that the research was conducted in the absence of any commercial or financial relationships that could be construed as a potential conflict of interest.
Generative AI statement
The author(s) declare that no Generative AI was used in the creation of this manuscript.
Publisher’s note
All claims expressed in this article are solely those of the authors and do not necessarily represent those of their affiliated organizations, or those of the publisher, the editors and the reviewers. Any product that may be evaluated in this article, or claim that may be made by its manufacturer, is not guaranteed or endorsed by the publisher.
References
Austin B., Austin D. A. (2016). Bacterial fish pathogens: disease of farmed and wild fish (Switzerland: Springer).
Bowker J., Trushenski J., Tuttle-Lau M., Straus D., Gaikowski M., Goodwin A., et al. (2011). Guide to using drugs, biologics, and other chemicals in aquaculture. Am. Fisheries Soc. Fish Culture Section.
Buchmann K. (2015). Impact and control of protozoan parasites in maricultured fishes. Parasitology 142, 168–177. doi: 10.1017/S003118201300005X
Colquhoun D., Sørum H. (2001). Temperature dependent siderophore production in vibrio salmonicida. Microbial pathogenesis 31, 213–219. doi: 10.1006/mpat.2001.0464
Defoirdt T., Sorgeloos P., Bossier P. (2011). Alternatives to antibiotics for the control of bacterial disease in aquaculture. Curr. Opin. Microbiol. 14, 251–258. doi: 10.1016/j.mib.2011.03.004
Duarte C. M. (2014). Global change and the future ocean: a grand challenge for marine sciences. Front. Mar. Sci. 1, 63. doi: 10.3389/fmars.2014.00063
Gai C., Liu J., Zheng X., Xu L., Ye H. (2022). Identification of vibrio ponticus as a bacterial pathogen of coral trout plectropomus leopardus. Front. Cell. Infection Microbiol. 12, 1089247. doi: 10.3389/fcimb.2022.1089247
Gong L., Hu Z., Zhou X. (2022). “A few samples underwater fish tracking method based on semi-supervised and attention mechanism,” in 2022 6th international conference on robotics, control and automation (ICRCA). Xiamen, China: IEEE. 18–22.
Han B., Hu Z., Su Z., Bai X., Yin S., Luo J., et al. (2022). Mask_lac r-cnn for measuring morphological features of fish. Measurement 203, 111859. doi: 10.1016/j.measurement.2022.111859
Hasan N., Ibrahim S., Aqilah Azlan A. (2022). Fish diseases detection using convolutional neural network (cnn). Int. J. Nonlinear Anal. Appl. 13, 1977–1984. doi: 10.22075/ijnaa.2022.5839
Keeling S., Brosnahan C., Johnston C., Wallis R., Gudkovs N., McDonald W. (2013). Development and validation of a real-time pcr assay for the detection of a eromonas salmonicida. J. Fish Dis. 36, 495–503. doi: 10.1111/jfd.2013.36.issue-5
Khasanah M., Nurdin Kadir N., Jompa J. (2019). Reproductive biology of three important threatened/near-threatened groupers (plectropomus leopardus, epinephelus polyphekadion and plectropomus areolatus) in eastern Indonesia and implications for management. Animals 9, 643. doi: 10.3390/ani9090643
Li H., Ye M.-Z., Peng B., Wu H.-K., Xu C.-X., Xiong X.-P., et al. (2010). Immunoproteomic identification of polyvalent vaccine candidates from vibrio parahaemolyticus outer membrane proteins. J. Proteome Res. 9, 2573–2583. doi: 10.1021/pr1000219
Li X., Zhao S., Chen C., Cui H., Li D., Zhao R. (2024). Yolo-fd: An accurate fish disease detection method based on multi-task learning. Expert Syst. Appl. 258, 125085. doi: 10.1016/j.eswa.2024.125085
Lieke T., Meinelt T., Hoseinifar S. H., Pan B., Straus D. L., Steinberg C. E. (2020). Sustainable aquaculture requires environmental-friendly treatment strategies for fish diseases. Rev. Aquaculture 12, 943–965. doi: 10.1111/raq.12365
Liu F., Xu X., Qing C., Jin J. (2018). “Probability matrix svm+ learning for complex action recognition,” in Internet Multimedia Computing and Service: 9th International Conference, ICIMCS 2017, Qingdao, China, August 23-25, 2017. 403–410 (Singapore: Springer).
Malik S., Kumar T., Sahoo A. (2017). “Image processing techniques for identification of fish disease,” in 2017 IEEE 2nd International Conference on Signal and Image Processing (ICSIP). 55–59 (Singapore: IEEE).
Meng L., Hirayama T., Oyanagi S. (2018). Underwater-drone with panoramic camera for automatic fish recognition based on deep learning. IEEE Access 6, 17880–17886. doi: 10.1109/ACCESS.2018.2820326
Mia M. J., Mahmud R. B., Sadad M. S., Al Asad H., Hossain R. (2022). An in-depth automated approach for fish disease recognition. J. King Saud University-Computer Inf. Sci. 34, 7174–7183. doi: 10.1016/j.jksuci.2022.02.023
Panicker G., Myers M. L., Bej A. K. (2004). Rapid detection of vibrio vulnificus in shellfish and gulf of Mexico water by real-time pcr. Appl. Environ. Microbiol. 70, 498–507. doi: 10.1128/AEM.70.1.498-507.2004
Pérez J. M. (2009). Parasites, pests, and pets in a global world: new perspectives and challenges. J. Exotic Pet Med. 18, 248–253. doi: 10.1053/j.jepm.2009.09.003
Pires N. M., Dong T., Yang Z., da Silva L. F. (2021). Recent methods and biosensors for foodborne pathogen detection in fish: Progress and future prospects to sustainable aquaculture systems. Crit. Rev. Food Sci. Nutr. 61, 1852–1876. doi: 10.1080/10408398.2020.1767032
Siliang M., Yong X. (2022). Mpdiou: A loss for efficient and accurate bounding box regression. arXiv abs/2307.07662.
Wagner W. P. (2017). Trends in expert system development: A longitudinal content analysis of over thirty years of expert system case studies. Expert Syst. Appl. 76, 85–96. doi: 10.48550/arXiv.2307.07662
Wu X., Sahoo D., Hoi S. C. (2020). Recent advances in deep learning for object detection. Neurocomputing 396, 39–64. doi: 10.1016/j.neucom.2020.01.085
Xie X., Wang J., Hu Z., Zhao Y. (2021). “Intelligent detection of mango disease spores based on mask scoring r-cnn,” in 2021 5th Asian Conference on Artificial Intelligence Technology (ACAIT). Haikou, China: IEEE. 768–774.
Keywords: deep learning, disease detection, Plectropomus leopardus, Vibrio disease, Hirudo parasitic disease
Citation: Liu M, Xue R, Wei C, Hu J, Bao Z, Xu G and Zhou J (2025) PLDNet: real-time Plectropomus leopardus disease recognition. Front. Mar. Sci. 12:1507104. doi: 10.3389/fmars.2025.1507104
Received: 07 October 2024; Accepted: 24 January 2025;
Published: 17 February 2025.
Edited by:
Chao Zhou, Beijing Research Center for Information Technology in Agriculture, ChinaReviewed by:
Zhuhua Hu, Hainan University, ChinaShijing Liu, Chinese Academy of Fishery Sciences (CAFS), China
Sijia Zhang, Dalian Ocean University, China
Copyright © 2025 Liu, Xue, Wei, Hu, Bao, Xu and Zhou. This is an open-access article distributed under the terms of the Creative Commons Attribution License (CC BY). The use, distribution or reproduction in other forums is permitted, provided the original author(s) and the copyright owner(s) are credited and that the original publication in this journal is cited, in accordance with accepted academic practice. No use, distribution or reproduction is permitted which does not comply with these terms.
*Correspondence: Junwei Zhou, anVud2VpemhvdUBtc24uY29t; Cun Wei, c29pd2VpY0BvdWMuZWR1LmNu
†These authors have contributed equally to this work