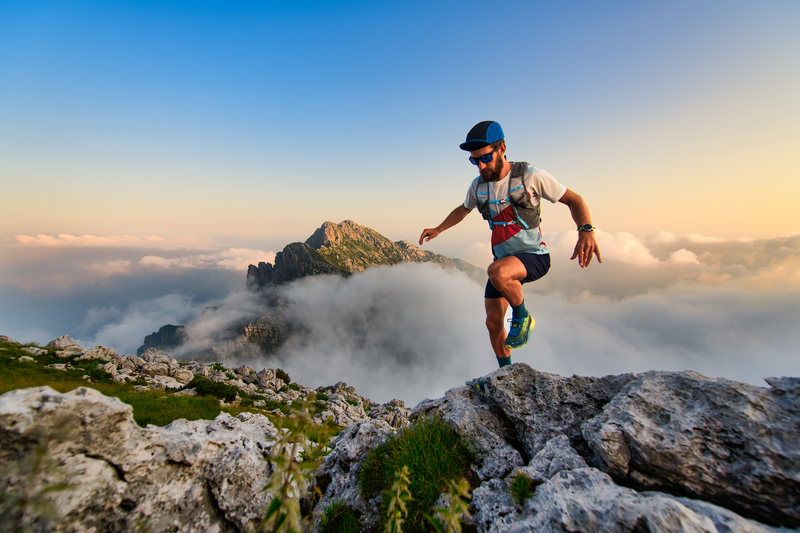
95% of researchers rate our articles as excellent or good
Learn more about the work of our research integrity team to safeguard the quality of each article we publish.
Find out more
ORIGINAL RESEARCH article
Front. Mar. Sci. , 04 April 2025
Sec. Global Change and the Future Ocean
Volume 12 - 2025 | https://doi.org/10.3389/fmars.2025.1504701
This article is part of the Research Topic Impacts of Climate Change on Seaweeds View all 10 articles
Coastal foundation species such as kelps, corals, and seagrasses play vital roles in supporting marine biodiversity and ecosystem services globally, but are increasingly threatened by climate change. In particular, kelp forests are highly dynamic ecosystems experiencing natural fluctuations across seasons and climate cycles, e.g., El Niño Southern Oscillation, Pacific Decadal Oscillation. As climate change increases variability in these cycles and extreme events such as marine heatwaves become more frequent, long term data are essential to understand deviations from the norm and to better estimate trends of change. This study uses a century-long dataset to examine kelp forest responses to regional drivers in Haida Gwaii, British Columbia, by combining remote sensing data from 1973-2021 with a snapshot of kelp distribution derived from historical records from 1867-1945. We reveal complex patterns of change, with kelp losses and resilience varying at different spatial scales. Kelp forests that had likely persisted for over a century exhibited an overall declining trend of 5 ± 2% per decade starting in the 1970s. Throughout the time series kelp area was driven by multi-year impacts of the Pacific Decadal Oscillation, El Nino Southern Oscillation, sea surface temperature anomalies and marine heatwaves, such as the 1998 El Niño and the 2014-2016 marine heatwave known as the ‘Blob’. In the warmest areas, kelp forests completely disappeared during the 1977 Pacific Decadal Oscillation shift. Cooler areas showed greater resilience, buffering the loss at the region wide scale, highlighting the importance of local gradients in understanding areas vulnerable to climate change. Lastly, local in situ surveys showed a lack of urchin barrens, and the presence of turf algae in the study region, further supporting the hypothesis that temperature, not herbivory, drove kelp forest loss in this region.
Coastal ecosystems, such as kelp forests, mangroves, and seagrass meadows, play pivotal roles in supporting global biodiversity by providing habitat, sequestering carbon, and delivering ecosystem goods and services (Wernberg et al., 2019; Cooley et al., 2022). However, the resilience of these ecosystems are threatened by climate change impacts (Krumhansl et al., 2016; Filbee-Dexter and Wernberg, 2018; Wernberg et al., 2019). Among these ecosystems, kelp forests, which cover nearly a third of the world's coastlines (Jayathilake and Costello, 2021), stand out as some of the planet's most productive ecosystems (Pessarrodona et al., 2022) but, despite their global significance, are the second most vulnerable coastal ecosystem to climate change after coral reefs (Cooley et al., 2022).
Climate change poses significant threats to kelp forests through both direct impacts of temperature and indirect effects primarily driven by altered species interactions (Wernberg et al., 2019). Direct impacts include rising sea surface temperatures (SST), storms and marine heatwaves (MHWs)—prolonged periods of anomalously warm water (Hobday et al., 2018). Increases in SST can reduce survival, growth and reproduction through physiological stress, while MHWs can cause sudden mortality by exceeding local temperature thesholds, even in populations far from range edges (Druehl, 1978; Arafeh-Dalmau et al., 2019; Rogers-Bennett and Catton, 2019; McPherson et al., 2021; Filbee-Dexter et al., 2022; Starko et al., 2022, 2024).
These temperature-driven effects manifest differently across geographic regions. At warm range edges, rising temperatures can drive kelp forest tropicalization either directly through temperature stress (Wernberg, 2021) or indirectly through the poleward expansion of tropical grazers (Vergés et al., 2016). In more temperate waters, the impacts of extreme events like MHWs and storms vary depending on local conditions (e.g., Mora-Soto et al., 2024a; Mora-Soto et al., 2024b; Starko et al., 2024). While storms can cause dislodgement through increasing wave height (Dayton and Tegner, 1984), waves may sometimes mitigate MHW impacts by increased mixing or reducing grazing (Hamilton et al., 2020; Starko et al., 2022).With the intensification of the El Niño cycle, MHWs and poor nutrient conditions are predicted to increase with the potential for the entire ocean to enter a permanent MHW state by 2100 (RCP 8.5 scenario; Oliver et al., 2019) likely having severe consequences for kelp forest across the world.
As a result of climate change, kelp forest distributions are already undergoing rapid transformations with significant differences in the scale and direction of change across the globe (Krumhansl et al., 2016). As predicted, these changes have led to significant kelp forest losses in some regions. In Western Australia, for example, approximately 100,000 ha of Ecklonia radiata forests were lost from the warm edge of its distribution during the 2011 marine heatwave (Wernberg, 2021), and in Southern Norway, approximately 780,000 ha of Saccharina latissima forests transitioned to turf reefs from increased warming and eutrophication (Filbee-Dexter et al., 2022). Alternatively, some places have shown stability despite climate change, like Southern Chile and the Falkland Islands, where stable SSTs maintain persistent Macrocystis pyrifera forests (Mora-Soto et al., 2021). In a few locations, kelp forests are even expanding, like South Africa's Ecklonia maxima forests (Bolton et al., 2012) or where sea ice has disappeared in the Canadian Arctic (Filbee-Dexter et al., 2019). These diverse patterns of change highlight the challenge of deciphering relationships between kelp forests and environmental drivers in a time of rapid global change.
Along the west coast of North America, kelps form large floating canopies that experience diverse environmental conditions, leading to complex patterns of change similar to global trends (Krumhansl et al., 2016). The overharvesting and extirpation of sea otters, key predators of herbivorous sea urchins, by the late 1800s, caused grazing-induced losses of kelp forests across North America (Watson and Estes, 2011). However, sea otter reintroductions along the northwest coast of Vancouver Island (Watson and Estes, 2011), and the expansion of remnant populations in central California (Nicholson et al., 2024), have supported kelp forest recovery in a small portion of sea otters’ historical range. More recently, an unprecedented mass of warm water formed in the northeastern Pacific in 2014, compounded by a strong El Niño in 2015-2016, leading to the large-scale MHW event known as ‘The Blob’ (Di Lorenzo and Mantua, 2016). This led to severe kelp forest declines in Baja California (Arafeh-Dalmau et al., 2019) and the transition of kelp forests to urchin barrens in Northern California, exacerbated by the seastar wasting disease epidemic, where the loss of the seastar Pycnopodia helianthoides led to an increase in urchin herbivory (Rogers-Bennett and Catton, 2019). Conversely, during the same 2014-2016 ‘Blob’ MHW, kelp forests remained stable in Southern California and Oregon (Reed et al., 2016; Hamilton et al., 2020). In British Columbia (BC), Canada, research on kelp forests trends remains sparse, with most studies relying on localized in situ surveys or short time series (Sutherland et al., 2008; Watson and Estes, 2011; Schroeder et al., 2019; Starko et al., 2022, 2024). Notably, only a few studies include continuous time series exceeding five years (Mora-Soto et al., 2024a, 2024b; Starko et al., 2024). This limited scope leaves significant knowledge gaps in understanding the complex patterns of change along most of BC's intricate coastline, highlighting the need for longer series to disentangle natural variability from long-term trends.
In this study, we investigate environmental heterogeneity and geographic scale within a region of BC's complex coastline to understand the variability in kelp forest responses to regional climate drivers, such as the Pacific Decadal Oscillation (PDO), El Niño Southern Oscillation (ENSO), North Pacific Gyre Oscillation (NPGO), SST anomalies, and MHW metrics. To achieve this, we compiled a long-term dataset of floating kelp forest canopy area from the Cumshewa Inlet and Gray Bay region (Figure 1), located within the Haida Gwaii archipelago, Canada, where local indigenous communities have observed recent declines (HMTK Participants et al., 2011; MaPP, 2021). Specifically, we assessed long-term changes in kelp forest distribution by comparing historical data (1867-1945) with satellite imagery (1973 to 2021). Using the satellite imagery time series (1973 to 2021; Table 1), we then quantified kelp forest trends and environmental drivers of change at regional (800 km²), subregional (five distinct areas), and local (1 km segments) scales. Based on the observed increases in climate disturbances in this region, we hypothesized that greater environmental heterogeneity at the regional scale would confer higher resilience. Specifically, we expected less drastic declines and more recovery after climatic events, like El Niño and MHW events, at the regional scale. In contrast, we expected more variable responses at the local and sub-regional scales, where outcomes would likely depend on each area’s position along local environmental gradients, such as SST, fetch, wind and tidal current.
Figure 1. (a) Map of the study region where the maximum kelp area across the complete time series (1973-2021) is shown in gray. (b) Map of the Haida Gwaii archipelago and study region shown in red in relation to the mainland coast of British Columbia and Alaska, showing bathymetry in meters (m) below chart datum and the location of the Bonilla Island Lighthouse, the location of the in situ SST data. (c) Image showing an example of the size and density of giant kelp (Macrocystis) forests found within the study region in relation to a 10 m research vessel.
Table 1. Summary of medium- to high resolution archived satellite imagery used to build the time series of kelp canopy area where the resolution refers to the spatial resolution of the multispectral imagery, inputs refer to band and band indices (NDVI: Normalized Difference Vegetation Index, GNDVI: NDVI with green instead of red, RE: red-edge, NIR: near-infrared) used in the object-based image classification and global accuracy refers to the measure of global accuracy attained in the validation of the classification.
Haida Gwaii is a remote archipelago located off Canada's mainland Pacific coast (Figure 1) and the recognized Aboriginal title lands of the Haida Nation (Haida Nation and Canada, 2024a, 2024b). The archipelago is characterized by cool, nutrient-rich marine environments which support extensive Nereocystis luetkeana and Macrocystis pyrifera (Lindstrom, 2023;syn. Macrocystis tenuifolia) kelp forests (HMTK Participants et al., 2011; Lee et al., 2021). The boundaries of the study region were defined to include the Hlk̲inul ChiiG̲as.sgii SG̲aagiidaay Protection Management Zone designated in the Haida Gwaii Marine Plan (MaPP, 2015) to protect an extensive kelp forest. Our study region of 800 km² on the northeast coast of Moresby Island (Figure 1) spans a sea surface temperature gradient of ~3°C and a strong exposure (fetch, wind and tidal current) gradient, allowing us to represent a wide range of conditions while minimizing the impact of confounding variables when comparing across vast distances. Sea otters were extirpated from Haida Gwaii during the maritime fur trade (1785-1840) and have not yet returned (Lee et al., 2021). This region is characterized by dense, large Macrocystis forests throughout (Figure 1c), with smaller dense patches of Nereocystis forests around Cumshewa Head (Figure 1a). These kelp forests are detectable from medium- to high-resolution satellites allowing us to monitor kelp forest change back to the 1970s (Gendall et al., 2023). Haida people note the importance of the Cumshewa and Flagstaff areas within the study region as crucial ecological and cultural areas for Macrocystis harvest for the herring-roe-on-kelp fishery (in Haida: k’aaw), however, have observed kelp declines in the early 2000s (HMTK Participants et al., 2011; MaPP, 2021) highlighting the need for increased monitoring and management (MaPP, 2015).
The time series comprises two components: (i) a historical snapshot of century-old kelp presence recorded in British Admiralty charts, and (ii) kelp canopy area derived from satellite imagery collected from 1973 to 2021. The British Admiralty charts data were created between 1867 and 1945 (hereafter historical kelp; Costa et al., 2020). Charts with scales ranging from 1:6,080 to 1:500,000 were scanned, georeferenced, and manually digitized to capture hand-drawn kelp features. In cases where overlapping kelp features were present on multiple charts, in the same region, the highest-resolution charts were prioritized. Each kelp feature was assigned a reliability category based on the depth of the seafloor below the feature, with all features within the study region classified as 'Very High' reliability due to their occurrence in depths shallower than 40 m. These charts, originally designed to map potential navigational risks for mariners, provide only a snapshot of kelp presence and should not be used to infer absences, as not all kelp forests may have been mapped. As such, the historical kelp distribution (Costa et al., 2020) was visually compared with the kelp distribution derived from the satellite imagery time series and was not included in any statistical analyses.
To construct a satellite imagery time series (1973-2021) of floating kelp forest canopy area (hereafter kelp area), we adopted the methodological framework outlined in Gendall et al. (2023). This framework includes: 1) imagery compilation and assessment, 2) imagery preprocessing, 3) classification, and 4) validation (see Supplementary Methods for detailed description of each step). To collect and assess the imagery, we compiled a dataset of archived remote sensing imagery from 1973 to 2021, with resolutions ranging from high (0.5 m) to medium (60 m; Table 1). Images were selected based on criteria (Supplementary Table S1, see Supplementary Methods) controlling for cloud cover, tidal conditions (Supplementary Figure S1), haze, waves, glint, and acquisition month (Schroeder et al., 2019; Cavanaugh et al., 2021; Gendall et al., 2023). The final image dataset consisted of 52 suitable images spanning 48 years. Imagery preprocessing included geometric corrections, atmospheric corrections, masking of land, deepwater and soft substrate (Gendall et al., 2023). Steep sloping bathymetry result in a narrow band of depths that are habitable to kelp before becoming too deep, limiting the extent of kelp forests to thin, fringing strips close to shore making detection difficult from medium-resolution satellites (Gendall et al., 2023). To mitigate uncertainties related to mapping small kelp forest canopies at varying resolutions, we excluded these areas of steep sloping bathymetry, exceeding 11.4%, from the analysis (Gendall et al., 2023). However, these steep sloping nearshore areas that were removed only accounted for 0.01% of the overall kelp area in the study region (Gendall et al., 2023).
To improve the detectability of kelp from non-kelp features in the imagery, we generated band indices/ratios specific to kelp (Table 1; Supplementary Table S2), which were linearly enhanced and integrated with the original bands for input into the classification process. Satellite imagery was classified utilizing eCognition Developer Software (Trimble Germany, 2011) with enhanced indices/ratios and bands as inputs. The classification results were validated by cross-referencing with available field and historical data (Gendall et al., 2023; Supplementary Table S3). When considering all sensors included in the validation, the overall global accuracy ranged between 88% and 94% (Table 1; Supplementary Table S3), where most errors were associated with sparse, fringing, or partially submerged kelp canopy (Gendall et al., 2023).
At the regional scale of analysis, we investigated the total kelp area of the entire study region through time and examined the relationship with regional drivers, including the PDO, ENSO, NPGO, SST anomalies, and MHW metrics. Since the dominant kelp in the region is Macrocystis, a perennial species, the annual averages, or sums in the case of MHW metrics, for the year preceding the month when most images were acquired were used. As most of images were acquired in July, annual measures were therefore calculated as the conditions experienced between August of the previous year and July of the sampling year (hereafter referred to as year and denoted by the year the image was acquired).
Annual averages of PDO (Huang et al., 2017), NPGO (Di Lorenzo et al., 2008), and ENSO (NOAA, 2015) were calculated from monthly data spanning 1969 to 2021. Annual SST anomalies (°C) and MHW metrics were calculated using daily in situ SST measures from the Bonilla Island lighthouse station (53°29'34.5" N; 130°38'17.0" W, Figure 1, Chandler, 2010). Bonilla Island lighthouse, located approximately 80 km from the study region, is the nearest site with continuous SST data dating back to the 1960s. Both MHW metrics and SST anomalies were calculated using a 55-year climatology starting in 1966. SST anomalies were calculated as the annual average temperature (°C) deviations above or below the time series climatological average. MHW metrics were calculated using the heatwaveR package in R (Schlegel and Smit, 2018), where a MHW was defined as a period of at least five consecutive days during which SST exceed the 90th percentile of the climatological average (Hobday et al., 2016). MHW days were calculated as the sum of the total number of days classified as a MHW in a given year. MHW cumulative intensity was calculated as the sum of daily temperature anomalies (°C) exceeding the climatological threshold, accumulated over the duration of all MHW events within a given year. While satellite-derived SST data, such as the Optimum Interpolation Sea Surface Temperature (OISST; Huang et al., 2021), are available for the study region, they do not cover the full temporal duration of the time series. A comparison of MHW metrics from Bonilla lighthouse and OISST data from the study region, revealed strong correlations for both MHW days (r = 0.90, df = 39, p<0.001) and MHW cumulative intensity (r = 0.85, df = 39, p<0.001; Supplementary Figure S2). Therefore, Bonilla lighthouse data were deemed suitable to represent MHW conditions within the study region.
To statistically compare kelp with regional drivers, annual kelp area was normalized as the percentage of the maximum kelp area observed through time and linear regressions were employed to assess temporal trends and relationships with regional drivers at multi-year scales. We examined the influence of previous years' conditions by considering one-, two-, and three-year metrics of regional drivers. The regional drivers exhibited some level of correlation (Supplementary Table S4), in which ENSO, PDO, SST anomalies and MHW metrics displayed positive correlations, and the NPGO showed an inverse correlation. Across all climate variables, SST anomalies showed a warming trend of 0.62°C between 1973 and 2021 (Supplementary Table S5). We assessed model residuals for normality (Faraway, 2004), and selected the best models based on the Akaike Information Criteria adjusted for a small sample size (AICc, Hurvich and Tsai, 1993). Univariate relationships between each regional driver and kelp area were tested, followed by the creation of multivariate models incorporating significant variables. Since most regional drivers were correlated (Supplementary Table S4) and largely represent temperature, we did not include non-significant predictors or test for interactions unlikely to exist. This approach simplifies analyses, reduces overfitting, and focuses on the most important drivers of kelp forest area (Anderson and Burnham, 2002; Coelho et al., 2019). All statistical analyses were conducted using R Studio (R Core Team, 2021) with the lme package (Bates et al., 2015).
Subregions were defined by clustering areas together with similar local environmental conditions of SST, fetch, wind and tidal currents. Landsat Analysis Ready Data (ARD) Surface Temperature (ST) from Landsat 5, 7, and 8 thermal sensors were used to quantify local SST conditions, as they offer the highest resolution SST data currently available (30 m; Dwyer et al., 2018; Wachmann et al., 2024). Local SST climatology was derived from all available cloud-free Landsat ARD ST images from July or August between 1984 to 2021 (1988, 1989, 2007, 2009 to 2011, 2014, 2015, and 2017) as clouds greatly increase the inaccuracy of SST measurements (Wachmann et al., 2024). As a proxy for exposure, fetch was estimated at 50 m intervals along the coastline and refers to the sum of fetch (max = 200 km), which is calculated by summing the distance to the nearest land mass at every 5° bearing around points on the shoreline (Gregr et al., 2019). Local wind conditions were represented using a raster of mean wind power density (W/m², 250 m resolution) at 10 m above sea level sourced from the Global Wind Atlas (Davis et al., 2023) and tidal currents were represented by root mean square average tidal speed (m/s) obtained from the BC Marine Conservation Analysis Marine Atlas (BCMCA, 2011).
We divided the entire study region into segments, each approximately 1 km in shoreline length, following the methodology outlined by Berry et al. (2003; Supplementary Figure S3, See Supplementary Methods) from the shoreline to the 20 m bathymetry limit using a bathymetry dataset from the Canadian Hydrographic Service (Davies et al., 2019). All local environmental conditions of SST, fetch, wind, tidal currents and depth were averaged per 1 km segment. We then employed a spatially constrained cluster analysis (k nearest neighbors = 4) in ArcMap (ESRI, 2018) to group 1 km segments into subregions based on local conditions excluding depth. To prevent statistical redundancies and the overweighting of correlated variables in the spatially constrained cluster analysis (Ketchen and Shook, 1996), we removed correlated drivers using Spearman rank-order correlations.
Similarly to the regional scale assessment, we normalized the timeseries of kelp area as a percentage of the maximum kelp area for each subregion and used linear regressions to show trends through time and relationships with regional drivers of PDO, ENSO, NPGO, SST anomalies, MHW days and MHW cumulative intensity. We defined 'resilience' at both the regional and subregional scale as areas that were variable but experienced no declining trends. In other words, we describe resilience as areas that exhibited resistance, ability to persist through disturbance, and/or recovery, the ability to bounce back following a disturbance (as defined by Holling, 1973) but did not specifically separate and quantify resistance and recovery.
At the local scale, a kelp persistence analysis (Schroeder et al., 2019) was performed on the 1 km segments (Supplementary Figure S3). Persistence was quantified as the percentage of years kelp was present in a segment across the entire time series, with 100% indicating continuous presence, lower values indicating reduced persistence, and 0% indicating a complete absence of kelp from a given segment. To better understand how local environmental conditions influence persistence, we examined the relationships between persistence and local conditions of SST, fetch, wind, tidal current and depth using linear regressions.
In August 2021, we conducted field surveys to investigate factors influencing kelp forest dynamics that could not be captured through remote sensing data, and to lend insight into the patterns of change documented in the time series analyses. In particular, we used photo-quadrats and a remotely operated vehicle (ROV) to quantify substrate type, urchin presence, and understory seaweed composition in areas where kelp had disappeared, persisted or was never present. Photoquadrats consisted of a goPro Hero 7 affixed to a tripod mounted on a 1m² quadrat. Both the photo-quadrats and the QYSEA Fifish V6 ROV were deployed from a boat at points across the study region. To ensure we spanned a gradient of no kelp to persistent kelp forests, we first used a stratified random sampling approach to create points in the northern part (Gray Bay and Flagstaff subregions) of the study region and opportunistically haphazardly added points in the field to increase sample size. To provide a comparison between persistent kelp forests in the northern and southern subregions we were able to sample eight points within the Cumshewa West subregion. However due to logistical constrainst were unable to sample within the Cumshewa East and Mathers Creek subregions.
A single representative frame was used from the ROV footage to avoid issues of spatial auto-correlation, issues of video quality in high current areas, and to make the ROV footage comparable with the photoquadrat data. The combined photoquadrat (n = 44) and ROV data (n = 4), resulted in a total sample size of 48. We identified urchin abundance, dominant substrate types, and dominant understory seaweeds present in ImageJ software (Schneider et al., 2012). Substrate types were classified into five categories: sand (0.06-2 mm grain size diameter), granule (2-4 mm), pebble (4-64 mm), cobble (64-256 mm), and boulder (0.25-3 m; Greene et al., 1999). Understory algae were categorized into three dominant functional groups: turf (short benthic algae), branched (Desmerestia sp.), and kelp (large brown algae in the order Laminariales).
The historical distribution of kelp from approximately a century ago (1867-1945; Figure 2a) closely mirrored the distribution of the maximum kelp area observed in satellite imagery from 1973 (8.18 km2; Figure 2b). However, since the historical kelp distribution was represented as artistic, hand-drawn features on maps, direct comparisons of kelp area were not possible.
Figure 2. (a) Map of the historic distribution of kelp canopy derived from British Nautical charts. (b-h) Maps of kelp canopies derived from satellite imagery from notable years.
Our satellite imagery analysis revealed a regional declining trend of 5 ± 2% (± SE) per decade between 1973 and 2021 (p<0.05, R² = 0.11, df = 1,33), with significant variability driven by regional drivers like the ENSO, PDO, SST anomalies and MHW metrics (Figure 3; Table 2). Warmer conditions coincided with lower kelp area, with environmental factors explaining up to 29% of the variance in kelp area (Table 2; Supplementary Table S6). Our best models identified the ENSO (one-year averages), SST anomalies (one-year to two year averages) and MHW metrics (MHW days and MHW cumulative intensity, one- to two-year sums) as equally good predictors (ΔAICc < 2) of regional kelp area (Table 2; Supplementary Table S6).
Figure 3. (a) Time series of normalized kelp area from 1973 to 2021 with major events displayed in red (b) Annual z-scores of climate indices, Pacific Decadal Oscillation (PDO), El Niño Southern Oscillation (ENSO), and North Pacific Gyre Oscillation (NPGO; inverted scale), such that positive values represent warm conditions (red) and negative values (blue) represent cool conditions. (c) Annual SST anomalies calculated using a 55-year historical average starting in 1966, where bars represent either positive (red) or negative (blue) anomalies in degrees Celsius above or below the time series average. (d) Annual sum of marine heatwave days calculated as number of days above the 90th percentile of the climatological average.
Table 2. Lowest AICc value of linear regressions of normalized kelp area by regional driver for the regional and subregional scale of analysis (p value < 0.5 *, <0.01**, <0.001***).
The time series exhibited periodic losses and recoveries with kelp area initially declining by 75% between 1973 and 1977 (Figures 2b, c, 3a) coinciding with the PDO phase shift (Figure 3b). This PDO phase shift was marked by an annual average SST anomaly of 0.26°C and a 10 day MHW in may of 1977 (Figures 3b–d). Subsequent fluctuations were tied to major El Niño events (e.g., 1983, 1998) and the 2014-2016 ‘Blob’ MHW. In 1998, one of the strongest El Niño events on record occurred, with SST of 1.23°C above average, a total of 193 MHW days and a MHW cumulative intensity of 348.67°C (Figures 3b–d). This event aligned with the time series minima, where only 5% of the kelp area (0.43 km²) remained (Figure 2e), however recovered to 33% by 1999 (Figure 2f).
In the study region, the 2014-2016 ‘Blob’ MHW had minimal impacts on regional SST in 2014, but led to SST anomaly of 0.92°C with a total of 179 MHW days in 2015, and in 2016 a SST anomaly of 0.91°C with a total of 114 MHW days (Figures 3b–d). This event corresponded with the second and third lowest kelp areas at 13% and 17% in 2015 and 2016, respectively (Figures 2g, 3a). Following the ‘Blob’, SST remained above average for the duration of the study period (2016-2021), however, not as extreme, allowing kelp forests to recover to 48% by 2021 (Figures 2h, 3a).
We employed a spatially constrained cluster analysis to group local 1 km segments into subregions based on fetch, local SST, wind, and tidal current. Fetch, wind, and tidal current exhibited high correlations (fetch and wind r = 0.78, fetch and tidal current r = 0.86, wind and tidal current r = 0.80, df = 237, p<0.001). Consequently, only fetch and SST were included in the cluster analysis. The pseudo-F-statistic analysis (Milligan and Cooper, 1985) defined five as the number of optimal subregions (pseudo-f-statistic: 4 groups = 272.92, 5 groups = 303.61, 6 groups = 279.9233; Supplementary Table S7). Gray Bay (subregion 1) and Flagstaff (subregion 2) were characterized by warm SSTs and moderate fetch, wind and current speeds (Figure 4a; Supplementary Table S7). Cumshewa East and West (subregions 3 and 4, respectively) were characterized by cooler SST, however, Cumshewa West had lower fetch, wind and tidal current (i.e. was more sheltered) than Cumshewa East. Mathers Creek (subregion 5) was characterized by warmer SSTs and low fetch, wind and current speeds.
Figure 4. (a) Map of subregions derived from the environmental cluster analysis showing the local SST climatology across the region and average temperature experienced in each subregion derived from the Landsat satellite thermal band. (b-f) Time series of normalized kelp area from 1973 to 2021 for each subregion with major events displayed in red.
Five subregions exhibited varying responses to climatic events, largely driven by differences in SST. Warmer more exposed subregions like Gray Bay and Flagstaff experienced drastic declines, with complete kelp loss in Gray Bay after 1977 (Figures 4a, b). Flagstaff showed a long-term decline of 9 ± 2% per decade (p<0.001, R² = 0.34, df = 1,33), with minimal recovery after the loss in the late 1970s (Figures 2c, d, 4c). Three-year SST averages best explained the variation (42 %) in the Flagstaff subregion (Table 2; Supplementary Table S8). By the early 21st century, approximately 90% of the original kelp area in the Flagstaff subregion had disappeared (Figure 4c).
In contrast, the cooler Cumshewa East and Cumshewa West subregions displayed resilience, with no significant long-term declining trends (Figures 4a, d, e). Kelp area in both subregions still fluctuated with environmental conditions, with ENSO (one-year and two-year averages) and MHW metrics (MHW days and MHW cumulative intensity, one- to three-year sums) explaining up to 25% (Cumshewa East) and 34% (Cumshewa West) of variation in kelp area (Table 2; Supplementary Tables S9, S10). However, unlike the northern subregions, these cooler subregions demonstrated a greater capacity for recovery following events, such as the 1977 PDO shift, the 1983 El Niño, and the 1998 El Niño (Figures 4d, e). Kelp area declined drastically during the 2014-2016 MHW in both subregions, however, Cumshewa West rebounded to approximately 77% (2017-2021), while Cumshewa East only reached 28% (Figures 4d, e).
The Mathers Creek subregion, despite being geographically warmer like Flagstaff and Gray Bay subregions, exhibited resilience similar to Cumshewa East and Cumshewa West (Figures 4a, f). In the Mathers Creek subregion, the PDO (one-year averages) and ENSO (one- and two-year averages) explained up to 24% of the variation in kelp area (Table 2; Supplementary Table S11). This subregion supported the lowest proportion of kelp area (maximum of 0.21 km2 in 1990; Figure 4f), which was largely located around the mouth of Mathers Creek in the cooler portion of the subregion.
At the local scale, variability of kelp persistence was significantly explained by local conditions of SST, wind, tidal current and depth where less wind, shallower depths and lower tidal currents coincided with higher persistence (Supplementary Table S12). Specifically, our best model included SST, wind and depth, which explained 41% of variation in kelp persistence (Supplementary Table S12). In Gray Bay, shallow water segments (2-5 m depth) experienced the warmest SST and exhibited no kelp persistence (0%; Figure 5a). Segments further offshore in Gray Bay showed low persistence (3-5%) and coincided with areas where kelp forests had entirely disappeared during the late 1970s PDO shift. In the Flagstaff subregion, kelp canopy persistence closely mirrored the SST gradient, where the warmer northern segments displayed low persistence (3-31%) with no recovery, and cooler southern segments demonstrated high persistence (67-95%), regularly recovering after climate events when cross referenced with the time series. In the more resilient subregions, the more sheltered nearshore segments in Cumshewa East (3), Cumshewa West (4), and Mathers Creek (5) maintained persistent kelp forests. Further offshore within the shallower mid-channel areas of Cumshewa East (3) and Cumshewa West (4), a core kelp area known as Fairbairn Shoals remained persistent throughout the time series.
Figure 5. (a) Locations of in situ survey sites overlaid over a map of the local persistence metric. (b, c) Stacked bar plots showing (b) the percent of substrate classes and (c) dominant understory algae classes documented in areas of no kelp, kelp loss and kelp persistence.
Where kelp was lost, field surveys suggested the habitat transitioned to turf-dominated reefs rather than urchin barrens. In particular, we observed only five individual urchins within the study region (n = 48), and in subregions Gray Bay and Flagstaff, where kelp was lost, the understory community was predominantly composed of short turf seaweeds and branched brown algae such as Desmarestia spp. on subtrate primarily composed of a mix of granule and cobble (Figures 5b, c). In the Flagstaff subregion, only one site where kelp was lost supported an understory kelp community; this site was closest to the remaining kelp forests. In contrast, where kelp canopy persisted, an abundant understory of kelp species were found on a variety of substrate types from pebble to boulders and bedrock (Figures 5b, c).
Climate change poses multifaceted threats, driving rapid shifts in kelp forest distributions, with some regions experiencing severe reductions, while others have remained stable or even expanded, complicating efforts to identify and disentangle drivers operating across spatial and temporal scales (e.g., Cavanaugh et al., 2011; Krumhansl et al., 2016; Pfister et al., 2018; Hamilton et al., 2020; Starko et al., 2022; Mora-Soto et al., 2024a). Using remote sensing (1973-2021) and historical data (1867-1945) from Haida Gwaii, we found that the kelp forest distribution in the early 1970s closely matched historical distribution, suggesting century-long stability prior to the significant declines observed in the late 1970s in the study region. In the satellite time series, kelp area fluctuated with regional drivers at one-, two- and three- year averages showing multi-year impacts where warmer conditions corresponding with reduced kelp area. While we observed a long-term declining trend at the regional scale, this decline was less severe than the declines observed in the warmest areas of the local and subregional scales, supporting the initial hypothesis that greater environmental heterogeneity confers higher resilience at broader spatial scales. Local and subregional kelp dynamics exhibited more variable trends, supporting the second hypothesis that the response to regional drivers is primarily determined by positioning along local gradients such as SST. Moreover, in situ observations supported that temperature, not urchin grazing, was the primary driver of loss and resilience in this study region.
The inclusion of historical data and medium resolution satellites revealed substantial kelp forest losses that would have gone undetected using only high-resolution satellite imagery. Specifically, we discovered a major episodic decline in the 1970s of kelp forests that had likely persisted for over a century – a finding that emerged only through our analysis of pre-1984 data sources, including nautical charts (1867-1945) and early Landsat imagery. The creation of long-term time series are crucial, as shorter time series (< 20 years) can mask important patterns in kelp forest resilience due to high interannual variability (Bell et al., 2020; Wernberg et al., 2019). While most remote sensing of kelp canopy on the Pacific Coast of North America focuses on 30 m Landsat imagery from 1984 onwards (e.g., Cavanaugh et al., 2011; Bell et al., 2020; Hamilton et al., 2020), researchers have successfully integrated diverse historical records to establish long-term baselines. Examples include the use of early 20th century kelp census data in Washington (Pfister et al., 2018), historical surveys from unpublished theses and aerial photography dating to the 1930s in South Australia (Carnell and Keough, 2019), Darwin's observations on the Voyage of the Beagle in Southern Chile (Mora-Soto et al., 2021), and nineteenth-century nautical charts in the Salish Sea (Mora-Soto et al., 2024b) – the latter revealing similar patterns of kelp loss in warmer regions. This underscores how incorporating historical data sources, despite their varying quality, resolution and methodologies, is essential for establishing accurate pre-industrial ecological baselines and understanding the true magnitude of changes that may otherwise be obscured by recent data alone.
Our findings emphasize that regional drivers, such as the PDO, ENSO, SST anomalies, and MHW metrics, are critical in explaining kelp forest dynamics, with impacts extending beyond immediate SST stress, with multi-year metrics explaining more variation in kelp area than single-year averages. Many studies corroborate that these regional drivers at multiple scales, from MHW metrics to large scale climate cycles like the PDO and ENSO, impact kelp forest conditions, where warm conditions are detrimental to kelp forests (e.g., Cavanaugh et al., 2011; Bell et al., 2020; Arafeh-Dalmau et al., 2019; Wernberg et al., 2019; McPherson et al., 2021; Wernberg, 2021). The extended multi-year impacts likely result from complete mortality of whole kelp plants rather than surface die back, as mortality reduces the reproductive capacity of the remaining kelp forest even after favorable conditions return (Cavanaugh et al., 2011; Pfister et al., 2018; Schroeder et al., 2019; Starko et al., 2022).
Regional drivers not only influence SST but also interact with other oceanic conditions exacerbating not only changes in kelp forests but the ecosystem as a whole. For instance, the significant kelp declines in the 1970s coincided with the 1977 PDO shift, which brought warmer SSTs, a deepening of the thermocline, low nutrient availability and increased ocean stratification across the North Pacific (McGowan et al., 2003; Parnell et al., 2010). This shift triggered a cascade of declines in other coastal systems, particularly in California such as reduced plankton biomass, larval fish abundance, fisheries landings, and similarly, Macrocystis biomass (McGowan et al., 2003; Parnell et al., 2010). Notably, the documented loss of kelp forests in this study predates the widespread coral reef declines attributed to the 1983 El Niño (Oliver et al., 2009) challenging the notion that kelp forests are not sensitive indicators of broader climate change impacts (Reed et al., 2016).
Local heterogeneity in factors like SST can either ameliorate or exacerbate the responses of foundation species like kelp forests to regional drivers, leading to complex patterns of decline and resilience (Russell and Connell, 2012; Starko et al., 2022). In our study region, cooler areas, like Cumshewa East and West, contribute to this resilience, as kelp forests there exhibited a stronger ability to endure and recover after adverse regional conditions. This spatial pattern of temperature-dependant responses has been documented elsewhere on the Pacific Coast, with losses concentrated in warmer areas during the 2014-2016 ‘Blob’ MHW in Barkley Sound (Starko et al., 2022) and the southern Salish Sea (Mora-Soto et al., 2024a). The complex coastline of British Columbia and Alaska creates high environmental heterogeneity (Starko et al., 2019; Cavanaugh et al., 2021), making it crucial to consider local factors such as temperature, wave exposure, and trophic dynamics (e.g., Watson and Estes, 2011; Rogers-Bennett and Catton, 2019; McPherson et al., 2021; Starko et al., 2022, 2024) to understand kelp forest variability and accurately forecast species responses to climate change moving forward (Russell and Connell, 2012).
In addition to temperature, local patterns of exposure, wind and current can either ameliorate or exacerbate the response of kelp forest to regional drivers. In more exposed locations, storms can tear up large swaths of kelp forests like the intense storms during the 1983 El Niño in Point Loma, California, that led to a loss of 560 ha of kelp forests (Dayton and Tegner, 1984). Recent work shows that storm tracks have intensified on the coast of BC from the 1960s onwards (Abeysirigunawardena, 2010) and with the significant negative relationship between kelp persistence and wind in the study region, storms could have compounded or caused some of the loss or variation not explained by regional or local drivers in this study.
Multiple lines of evidence, the lack of urchins, and presence of turf reefs, in our 2021 field survey suggest that temperature, rather than herbivory, likely drove kelp forest declines in the study region. Despite the presence of urchin barrens (HMTK Participants et al., 2011; Lee et al., 2021), and the absence of sea otters since the late 1800s (Lee et al., 2021) in Haida Gwaii, our field survey found no urchin barrens and very few individual urchins in areas of kelp loss and persistence. The absence of urchin barrens is particularly notable as they are known to persist for years to decades due to urchins’ ability to slow metabolic activity when food resources are scarce (Spindel et al., 2021). Additionally, the patchy mix of unconsolidated substrate – consisting of sand, pebbles, cobbles and boulders, is known to dissuade urchins from foraging (Laur et al., 1986) and may limit the distribution of urchins across the study region. Notably, in Gray Bay, where kelp was never present, both turf and eelgrass dominated shallow areas on sand and pebbles. This distribution pattern aligns with known habitat requirements, as canopy forming kelp require larger hard substratum such as cobbles, boulders or bedrock to provide secure attachment points (Druehl, 1978; Gregr et al., 2019; Starko et al., 2022), suggesting that substrate type likely plays a key role in determining kelp forest distribution across the region. Additionally, in areas where kelp disappeared, we found predominantly turf and branched algae (Desmarestia spp.) – a transition pattern commonly observed from temperature driven kelp losses in other regions of the globe, such as Western Australia (Wernberg, 2021), Atlantic Canada, France and Denmark (Filbee-Dexter and Wernberg, 2018). While our conclusion about herbivore impacts in this system is supported by two lines of evidence, our single time-point highlights the need for further research to fully understand trophic dynamics in this ecosystem.
The regional and local drivers included in this study did not account for all variation observed in kelp area and persistence. Variability in other potential drivers, such as nutrients (e.g., Zimmerman and Kremer, 1986), light availability (e.g., Deysher and Dean, 1986), salinity (e.g., Druehl, 1978) and local stressors from human activities like pollution or overharvesting, are known to impact kelp forests (e.g., Foster and Schiel, 2010; Pfister et al., 2018), but data were unavailable for the region. Warmer SST often coincides with lower nutrients and salinity (Druehl, 1978), which generally slows kelp growth, weakens tissue and diminishes reproduction (Zimmerman and Kremer, 1986). As such, nutrients likely play an important role alongside SST in regulating kelp dynamics but were unavailable in this region. Although light data were unavailable, the significant relationship we found between depth and kelp persistence likely reflects the influence of light availability, as light attenuation increases with water column depth (Timmer et al., 2022).
While coastal development, point-source pollution and overharvesting can drive kelp declines (Foster and Schiel, 2010; Pfister et al., 2018), anthropogenic stressors are likely minimal in Haida Gwaii given its small population (< 5000 people) and minimal development (Statistics Canada, 2017) when compared to densely populated coastal areas with observed kelp declines like in Washington or South Australia (Krumhansl et al., 2016; Pfister et al., 2018). Although Macrocystis kelp was historically harvested for the herring-roe-on-kelp commercial fisheries in the Flagstaff subregion, BC’s strict harvest limits leave the majority of the kelp plants intact minimizing harvest impacts. While turbidity related to logging runoff on Haida Gwaii (Klein et al., 2012) could impact kelp, the persistence of healthy kelp forests at the mouth of Mathers Creek—the region's only river—suggests minimal impacts from both turbidity and reduced salinity. Instead, the river's outflow may actually buffer SST impacts as evidenced by the retention of kelp forests in the Mathers Creek subregion despite losses in the similarly warm Flagstaff and Gray Bay subregions.
Previous work suggests that interannual changes in kelp area up to 7% could potentially arise from errors associated with measuring kelp area at different spatial resolutions (Gendall et al., 2023). While measurement uncertainty is an inherent challenge in remote sensing of kelp forests, the magnitude of kelp decline we observed far exceeds the potential error threshold identified by Gendall et al. (2023). Additionally, our focus on long-term trends and persistence over multiple decades helps mitigate the influence of such errors on our overall findings (Magurran et al., 2010). This approach to analyzing temporal patterns is sound as it emphasizes directional changes over long time scales rather than year-to-year fluctuations that might be influenced by artifacts or uncertainties in remote sensing measurements. Additionally, our use of multiple temporal metrics—including both area changes at the regional and subregional scale and persistence patterns at the local scale—provide complementary lines of evidence that strengthen our confidence in the patterns of kelp forest change herein.
This century-long analysis of kelp forests in Hada Gwaii elucidates the complex interplay between climate change and kelp forest dynamics operating across multiple spatial scales. Our analysis showed that kelp forests that likely remained stable for over a century began showing significant declines in the 1970s, with a regional loss of 5 ± 2% per decade. These responses varied across spatial scales. With local environmental conditions – particulary SST – playing an important role in determining outcomes. Warmer areas experienced complete kelp loss following the 1977 PDO shift, while, cooler areas exhibited greater resilience and the capacity to recover from large-scale climatic events, such as el Niño and MHW events. The absence of urchin barrens and presence of turf-dominated reefs in areas of kelp loss suggest that temperature, rather than herbivory, was the primary driver of decline in this study region. These findings offer valuable insights for the integration of historical data and the consideration of scale-dependent responses when assessing climate change impacts on coastal ecosystems. Continued monitoring and conservation efforts remain essential to ensure the persistence and resilience of these vital coastal ecosystems.
The datasets presented in this study can be found in online repositories. The names of the repository/repositories and accession number(s) can be found below: https://github.com/liannagendall/HaidaGwaii_Kelp and https://doi.org/10.5281/zenodo.14984911.
LG: Conceptualization, Data curation, Formal analysis, Funding acquisition, Investigation, Methodology, Project administration, Resources, Software, Supervision, Validation, Visualization, Writing – original draft, Writing – review & editing. MH-L: Conceptualization, Funding acquisition, Methodology, Resources, Supervision, Writing – review & editing. AW: Data curation, Formal analysis, Methodology, Writing – review & editing. SS: Conceptualization, Methodology, Writing – review & editing. LR: Conceptualization, Methodology, Writing – review & editing. SC: Conceptualization, Methodology, Resources, Writing – review & editing. LL: Conceptualization, Methodology, Resources, Writing – review & editing. NG: Conceptualization, Resources, Writing – review & editing. MC: Conceptualization, Data curation, Formal analysis, Funding acquisition, Methodology, Project administration, Resources, Software, Supervision, Validation, Writing – review & editing.
The author(s) declare that financial support was received for the research and/or publication of this article. This research was supported through a Natural Sciences and Engineering Research Council of Canada (NSERC) Alliance grant (Ref. number: ALLRP 566735 - 21) and NSERC Discovery grant awarded to MC. LG was also supported during this research through a MITACS Accelerate internship with the Hakai Institute.
We thank the Hakai Institute for partially funding this work, as well as the Canadian Hydrographic Service, Transport Canada, the Department of Fisheries and Oceans Canada (in particular Joanne Lessard), Environment and Climate Change Canada, and ShoreZone, for providing satellite data and ground-truth data. A special thanks to Parks Canada and the Council of the Haida Nation for assistance with field equipment on Haida Gwaii.
The authors declare that the research was conducted in the absence of any commercial or financial relationships that could be construed as a potential conflict of interest.
The author(s) declare that no Generative AI was used in the creation of this manuscript.
All claims expressed in this article are solely those of the authors and do not necessarily represent those of their affiliated organizations, or those of the publisher, the editors and the reviewers. Any product that may be evaluated in this article, or claim that may be made by its manufacturer, is not guaranteed or endorsed by the publisher.
The Supplementary Material for this article can be found online at: https://www.frontiersin.org/articles/10.3389/fmars.2025.1504701/full#supplementary-material
Abeysirigunawardena D. S. (2010).Climate variability and change impacts on coastal environmental variables in British Columbia, Canada. Available online at: https://dspace.library.uvic.ca/handle/1828/2664 (Accessed November 06, 2023).
Anderson D. R., Burnham K. P. (2002). Avoiding pitfalls when using information-theoretic methods. J. Wildl. Manage. 66, 912. doi: 10.2307/3803155
Arafeh-Dalmau N., Montaño-Moctezuma G., Martínez J. A., Beas-Luna R., Schoeman D. S., Torres-Moye G. (2019). Extreme marine heatwaves alter kelp forest community near its equatorward distribution limit. Front. Mar. Sci. 6. doi: 10.3389/fmars.2019.00499
Bates D., Mächler M., Bolker B., Walker S. (2015). Lme4: Linear mixed-effects models using “Eigen” and S4 (R package version 1.1-21). Available online at: https://CRAN.R-project.org/package=lme4 (Accessed March 27, 2022).
BCMCA (2011). Marine Atlas of Pacific Canada: a product of the British Columbia Marine Conservation Analysis (BCMCA) (Vancouver, BC, Canada: British Columbia Marine Conservation Analysis).
Bell T. W., Allen J. G., Cavanaugh K. C., Siegel D. A. (2020). Three decades of variability in California’s giant kelp forests from the Landsat satellites. Remote Sens. Environ. 238, 110811. doi: 10.1016/j.rse.2018.06.039
Berry H. D., Sewell A. T., Wyllie-Echeverria S., Reeves B. R., Mumford T. F., Skalski J. R., et al. (2003). Puget Sound Submerged Vegetation Monitoring Project: 2000 - 2002 Monitoring Report (Olympia, WA: Washington State Department of Resources), Vol. 170.
Bolton J., Anderson R., Smit A., Rothman M. (2012). South african kelp moving eastwards: the discovery of ecklonia maxima (Osbeck) papenfuss at de hoop nature reserve on the south coast of south africa. Afr. J. Mar. Sci. 34, 1. doi: 10.2989/1814232X.2012.675125
Carnell P. E., Keough M. J. (2019). Reconstructing historical marine populations reveals major decline of a kelp forest ecosystem in Australia. Estuaries Coasts 42, 765–778. doi: 10.1007/s12237-019-00525-1
Cavanaugh K. C., Bell T., Costa M., Eddy N. E., Gendall L., Gleason M. G., et al. (2021). A review of the opportunities and challenges for using remote sensing for management of surface-canopy forming kelps. Front. Mar. Sci. 8. doi: 10.3389/fmars.2021.753531
Cavanaugh K., Siegel D., Reed D., Dennison P. (2011). Environmental controls of giant-kelp biomass in the Santa Barbara Channel, California. Mar. Ecol. Prog. Ser. 429, 1–17. doi: 10.3354/meps09141
Chandler P. C. (2014). Sea surface temperature and salinity trends observed at lighthouses and weather buoys in British Columbia, 2013. In: Perry R. I. (Ed). State of the physical, biological and selected fishery resources of Pacific Canadian marine ecosystems in 2013. Can. Tech. Rep. Fish. Aquat. Sci., 3102. Available online at: https://www.researchgate.net/profile/Tyson-Carswell/publication/304380333_Costa_M_Carswell_T_Sweeting_R_Young_E_2014_Spatial-temporal_phytoplankton_bloom_initiation_in_the_Strait_of_Georgia_derived_from_MODIS_imagery_2002-2013_In_RI_Perry_Ed_State_of_the_physical_biological/links/576d75ec08ae0b3a3b75528f/Costa-M-Carswell-T-Sweeting-R-Young-E-2014-Spatial-temporal-phytoplankton-bloom-initiation-in-the-Strait-of-Georgia-derived-from-MODIS-imagery-2002-2013-In-RI-Perry-Ed-State-of-the-physical-b.pdf (Accessed May 20, 2024).
Coelho M. T. P., Diniz-Filho J. A., Rangel T. F. (2019). A parsimonious view of the parsimony principle in ecology and evolution. Ecography 42, 968–976. doi: 10.1111/ecog.04228
Cooley S., Schoeman D., Bopp L., Byod P., Donner S., Ito S., et al. (2022). Ocean and coastal ecosystems and their services. (Cambridge, United Kingdom: Cambridge University Press). doi: 10.1017/9781009325844
Costa M., Le Baron N., Tenhunen K., Nephin J., Willis P., Mortimor J. P., et al. (2020). Historical distribution of kelp forests on the coast of British Columbia: 1858–1956. Appl. Geogr. 120, 102230. doi: 10.1016/j.apgeog.2020.102230
Davies S. C., Gregr E. J., Lessard J., Bartier P., Wills P. (2019). Coastal digital elevation models integrating ocean bathymetry and land topography for marine ecological analyses in Pacific Canadian waters (Ottawa, ON, Canada: Fisheries and Oceans Canada). Available online at: http://epe.lac-bac.gc.ca/100/201/301/weekly_acquisitions_list-ef/2019/19-43/publications.gc.ca/collections/collection_2019/mpo-dfo/Fs97-6-3321-eng.pdf (Accessed August 27, 2020).
Davis N. N., Badger J., Hahmann A. N., Hansen B. O., Mortensen N. G., Kelly M., et al. (2023). The global wind atlas: A high-resolution dataset of climatologies and associated web-based application. Bull. Am. Meteorol. Soc. 104, E1507–E1525. doi: 10.1175/BAMS-D-21-0075.1
Dayton P. K., Tegner M. J. (1984). Catastrophic storms, El Nino, and patch stability in a southern California kelp community. Science 224, 283–285. doi: 10.1126/science.224.4646.283
Deysher L. E., Dean T. A. (1986). Interactive effects of light and temperature on sporophyte production in the giant kelp Macrocystis pyrifera. Mar. Biol. 93, 17–20. doi: 10.1007/BF00428650
Di Lorenzo E., Mantua N. (2016). Multi-year persistence of the 2014/15 North Pacific marine heatwave. Nat. Clim. Change 6, 1042–1047. doi: 10.1038/nclimate3082
Di Lorenzo E., Schneider N., Cobb K. M., Franks P. J. S., Chhak K., Miller A. J., et al. (2008). North Pacific Gyre Oscillation links ocean climate and ecosystem change. Geophys. Res. Lett. 35, L08607. doi: 10.1029/2007GL032838
Druehl L. D. (1978). The distribution of Macrocystis integrifolia in British Columbia as related to environmental parameters. Can. J. Bot. 56, 69–79. doi: 10.1139/b78-007
Dwyer J. L., Roy D. P., Sauer B., Jenkerson C. B., Zhang H. K., Lymburner L. (2018). Analysis ready data: enabling analysis of the landsat archive. Remote Sens. 10, 1363. doi: 10.3390/rs10091363
Filbee-Dexter K., Wernberg T., Fredriksen S., Norderhaug K. M., Pedersen M. F. (2019). Arctic kelp forests: Diversity, resilience and future. Glob. Planet. Change. 172, 1–14. doi: 10.1016/j.gloplacha.2018.09.005
Filbee-Dexter K., Wernberg T. (2018). Rise of turfs: A new battlefront for globally declining kelp forests. BioScience 68, 64–76. doi: 10.1093/biosci/bix147
Filbee-Dexter K., Wernberg T., Barreiro R., Coleman M. A., de Bettignies T., Feehan C. J., et al. (2022). Leveraging the blue economy to transform marine forest restoration. J. Phycol. 58, 198–207. doi: 10.1111/jpy.13239
Foster M. S., Schiel D. R. (2010). Loss of predators and the collapse of southern California kelp forests (?): Alternatives, explanations and generalizations. J. Exp. Mar. Biol. Ecol. 393, 59–70. doi: 10.1016/j.jembe.2010.07.002
Gendall L., Schroeder S. B., Wills P., Hessing-Lewis M., Costa M. (2023). A multi-satellite mapping framework for floating kelp forests. Remote Sens. 15, 1276. doi: 10.3390/rs15051276
Greene H. G., Yoklavich M. M., Starr R. M., O’Connell V. M., Wakefield W. W., Sullivan D. E., et al. (1999). A classification scheme for deep seafloor habitats. Oceanol. Acta 22, 663–678. doi: 10.1016/S0399-1784(00)88957-4
Gregr E. J., Palacios D. M., Thompson A., Chan K. M. A. (2019). Why less complexity produces better forecasts: an independent data evaluation of kelp habitat models. Ecography 42, 428–443. doi: 10.1111/ecog.03470
Haida Marine Traditional Knowledge (HMTK), Council of the Haida Nation, Haida Oceans Technical Team, Winbourne J. (2011). Haida Marine Traditional Knowledge Volume III (Skidegate, BC, Canada: Council of the Haida Nation).
Haida Nation, Canada (2024a). Chiixuujin / Chaaw Kaawgaa Big Tide (Low Water) Haida Title Lands Agreement. Available online at: https://www.haidanation.ca/wp-content/uploads/2024/12/CHIIXUUJIN-CHAAW-KAAWGAA-BIG-TIDE-LOW-WATER-SIGNED-IN-COUNTERPART-2024-12-04.pdf (Accessed December 3, 2024).
Haida Nation, Canada (2024b). Gaayhllxid/ Gíihlagalgang “Rising Tide” Haida Title Lands Agreement. Available online at: https://www.haidanation.ca/wp-content/uploads/2024/04/GG-Haida-Title-Lands-Agreement-April-6-2024.pdf (Accessed December 3, 2024).
Hamilton S. L., Bell T. W., Watson J. R., Grorud-Colvert K. A., Menge B. A. (2020). Remote sensing: generation of long-term kelp bed data sets for evaluation of impacts of climatic variation. Ecology 101, e03031. doi: 10.1002/ecy.3031
Hobday A. J., Alexander L. V., Perkins S. E., Smale D. A., Straub S. C., Oliver E. C. J., et al. (2016). A hierarchical approach to defining marine heatwaves. Prog. Oceanogr. 141, 227–238. doi: 10.1016/j.pocean.2015.12.014
Hobday A., Oliver E., Sen Gupta A., Benthuysen J., Burrows M., Donat M., et al. (2018). Categorizing and naming marine heatwaves. Oceanog 31, 162–173. doi: 10.5670/oceanog.2018.205
Holling C. S. (1973). Resilience and stability of ecological systems. Annu. Rev. Ecol. System. 4, 1–23. doi: 10.1146/annurev.es.04.110173.000245
Huang B., Liu C., Banzon V., Freeman E., Graham G., Hankins B., et al. (2021). Improvements of the Daily Optimum Interpolation Sea Surface Temperature (DOISST) Version 2.1. doi: 10.1175/JCLI-D-20-0166.1
Huang B., Thorne P. W., Banzon V. F., Boyer T., Chepurin G., Lawimore J. H., et al. (2017). PDO from NOAA Extended Reconstructed Sea Surface Temperature (ERSST), Version 5. doi: 10.7289/V5T72FNM
Hurvich C. M., Tsai C.-L. (1993). A corrected akaike information criterion for vector autoregressive model selection. J. Time Ser. Anal. 14, 271–279. doi: 10.1111/j.1467-9892.1993.tb00144.x
Jayathilake D. R. M., Costello M. J. (2021). Version 2 of the world map of laminarian kelp benefits from more Arctic data and makes it the largest marine biome. Biol. Conserv. 257, 109099. doi: 10.1016/j.biocon.2021.109099
Ketchen D. J., Shook C. L. (1996). The application of cluster analysis in strategic management research: an analysis and critique. Strat. Manage. J. 17, 441–458. doi: 10.1002/(SICI)1097-0266(199606)17:6<441::AID-SMJ819>3.0.CO;2-G
Klein R. D., Lewis J., Buffleben M. S. (2012). Logging and turbidity in the coastal watersheds of northern California. Geomorphology 139–140, 136–144. doi: 10.1016/j.geomorph.2011.10.011
Krumhansl K. A., Okamoto D. K., Rassweiler A., Novak M., Bolton J. J., Cavanaugh K. C., et al. (2016). Global patterns of kelp forest change over the past half-century. Proc. Natl. Acad. Sci. U.S.A. 113, 13785–13790. doi: 10.1073/pnas.1606102113
Laur D. R., Ebeling A. W., Reed D. C. (1986). Experimental evaluations of substrate types as barriers to sea urchin (Strongylocentrotus spp.) movement. Mar. Biol. 93, 209–215. doi: 10.1007/BF00508258
Lee L. C., Daniel McNeill G., Ridings P., Featherstone M., Okamoto D. K., Spindel N. B., et al. (2021). Chiixuu tll iinasdll: indigenous ethics and values lead to ecological restoration for people and place in gwaii haanas. Ecol. Rest. 39, 45–51. doi: 10.3368/er.39.1-2.45
Lindstrom S. C. (2023). A reinstated species name for north-eastern Pacific Macrocystis (Laminariaceae, Phaeophyceae). Notulae Algarum 290. Available at: https://www.notulaealgarum.com/2023/documents/Notulae%20Algarum%20No.%20290.pdf (Accessed May 20, 2024).
Magurran A. E., Baillie S. R., Buckland S. T., Dick J. M., Elston D. A., Scott E. M., et al. (2010). Long-term datasets in biodiversity research and monitoring: assessing change in ecological communities through time. Trends Ecol. Evol. 25, 574–582. doi: 10.1016/j.tree.2010.06.016
Marine Plan Partnership for the North Pacific Coast (MaPP) (2021). Regional Kelp Monitoring on the North Pacific Coast: A Community -Based Monitoring Initiative to Inform Ecosystem-Based Management. Available online at: https://storymaps.arcgis.com/stories/78945b1d95ec4b9fab5142670088174f (Accessed April 25, 2024).
McGowan J. A., Bograd S. J., Lynn R. J., Miller A. J. (2003). The biological response to the 1977 regime shift in the California Current. Deep Sea Res. Part II: Topical Stud. Oceanogr. 50, 2567–2582. doi: 10.1016/S0967-0645(03)00135-8
McPherson M. L., Finger D. J. I., Houskeeper H. F., Bell T. W., Carr M. H., Rogers-Bennett L., et al. (2021). Large-scale shift in the structure of a kelp forest ecosystem co-occurs with an epizootic and marine heatwave. Commun. Biol. 4, 298. doi: 10.1038/s42003-021-01827-6
Milligan G. W., Cooper M. C. (1985). An examination of procedures for determining the number of clusters in a data set. Psychometrika. 50, 2. doi: 10.1007/BF02294245
Mora-Soto A., Capsey A., Friedlander A. M., Palacios M., Brewin P. E., Golding N., et al. (2021). One of the least disturbed marine coastal ecosystems on Earth: Spatial and temporal persistence of Darwin’s sub-Antarctic giant kelp forests. J. Biogeogr. 48, 2562–2577. doi: 10.1111/jbi.14221
Mora-Soto A., Schroeder S., Gendall L., Wachmann A., Narayan G. R., Read S., et al. (2024a). Kelp dynamics and environmental drivers in the southern Salish Sea, British Columbia, Canada. Front. Mar. Sci. 11. doi: 10.3389/fmars.2024.1323448
Mora-Soto A., Schroeder S., Gendall L., Wachmann A., Narayan G., Read S., et al. (2024b). Back to the past: long-term persistence of bull kelp forests in the Strait of Georgia, Salish Sea, Canada. Front. Mar. Sci. 11. doi: 10.3389/fmars.2024.1446380
Nicholson T. E., McClenachan L., Tanaka K. R., Houtan K. S. V. (2024). Sea otter recovery buffers century-scale declines in California kelp forests. PloS Climate 3, e0000290. doi: 10.1371/journal.pclm.0000290
NOAA Center for Weather and Climate Prediction, Climate Prediction Center (2015). El Nino Suthern Oscillation (ENSO). Available online at: https://origin.cpc.ncep.noaa.gov/products/analysis_monitoring/ensostuff/ONI_v5.php (Accessed March 05, 2022).
Oliver J. K., Berkelmans R., Eakin C. M. (2009). “Coral Bleaching in Space and Time,” in Coral Bleaching: Patterns, Processes, Causes and Consequences. Eds. van Oppen M. J. H., Lough J. M. (Springer, Berlin, Heidelberg), 21–39. doi: 10.1007/978-3-540-69775-6_3
Oliver E. C. J., Burrows M. T., Donat M. G., Sen Gupta A., Alexander L. V., Perkins-Kirkpatrick S. E., et al. (2019). Projected marine heatwaves in the 21st century and the potential for ecological impact. Front. Mar. Sci. 6. doi: 10.3389/fmars.2019.00734
Parnell P. E., Miller E. F., Cody C. E. L., Dayton P. K., Carter M. L., Stebbinsd T. D. (2010). The response of giant kelp (Macrocystis pyrifera) in southern California to low-frequency climate forcing. Limnol. Oceanogr. 55, 2686–2702. doi: 10.4319/lo.2010.55.6.2686
Pessarrodona A., Assis J., Filbee-Dexter K., Burrows M. T., Gattuso J.-P., Duarte C. M., et al. (2022). Global seaweed productivity. Sci. Adv. 8, eabn2465. doi: 10.1126/sciadv.abn2465
Pfister C. A., Berry H. D., Mumford T. (2018). The dynamics of Kelp Forests in the Northeast Pacific Ocean and the relationship with environmental drivers. J. Ecol. 106, 1520–1533. doi: 10.1111/1365-2745.12908
R Core Team (2021). R: A language and environment for statistical computing (Version 4.1.2). Available online at: https://www.R-project.org/ (Accessed November 06, 2023).
Reed D., Washburn L., Rassweiler A., Miller R., Bell T., Harrer S. (2016). Extreme warming challenges sentinel status of kelp forests as indicators of climate change. Nat. Commun. 7, 13757. doi: 10.1038/ncomms13757
Rogers-Bennett L., Catton C. A. (2019). Marine heat wave and multiple stressors tip bull kelp forest to sea urchin barrens. Sci. Rep. 9, 15050. doi: 10.1038/s41598-019-51114-y
Russell B. D., Connell S. D. (2012). Origins and consequences of global and local stressors: incorporating climatic and non-climatic phenomena that buffer or accelerate ecological change. Mar. Biol. 159, 2633–2639. doi: 10.1007/s00227-011-1863-8
Schlegel R. W., Smit A. J. (2018). heatwaveR: A central algorithm for the detection of heatwaves and cold-spells. J. Open Source Softw. 3, 821. doi: 10.21105/joss.00821
Schneider C. A., Rasband W. S., Eliceiri K. W. (2012). NIH Image to ImageJ: 25 years of image analysis. Nat. Methods 9, 671–675. doi: 10.1038/nmeth.2089
Schroeder S. B., Boyer L., Juanes F., Costa M. (2019). Spatial and temporal persistence of nearshore kelp beds on the west coast of British Columbia, Canada using satellite remote sensing. Remote Sens. Ecol. Conserv. 6, 327–343. doi: 10.1002/rse2.142
Spindel N. B., Lee L. C., Okamoto D. K. (2021). Metabolic depression in sea urchin barrens associated with food deprivation. Ecology 102, 1–5. doi: 10.1002/ecy.v102.11
Starko S., Bailey L. A., Creviston E., James K. A., Warren A., Brophyid M. K., et al. (2019). Environmental heterogeneity mediates scale- dependent declines in kelp diversity on intertidal rocky shores. PloS One 14, e0213191. doi: 10.1371/journal.pone.0213191
Starko S., Neufeld C. J., Gendall L., Timmer B., Campbell L., Yakimishyn J., et al. (2022). Microclimate predicts kelp forest extinction in the face of direct and indirect marine heatwave effects. Ecol. Appl., e2673. doi: 10.1002/eap.2673
Starko S., Timmer B., Reshitnyk L., Csordas M., McHenry J., Schroeder S., et al. (2024). Local and regional variation in kelp loss and stability across coastal British Columbia. Mar. Ecol. Prog. Ser. 733, 1–26. doi: 10.3354/meps14548
Statistics Canada (2017). Focus on Geography Series 2016 Census (Ottawa, Ontario: Statistics Canada).
Sutherland I. R., Karpouzi V., Mamoser M., Carswell B. (2008). Kelp inventory 2007 : Areas of the British Columbia Central Coast from Hakai Passage to the Bardswell Group (Victoria, BC, Canada: Oceans and Marine Fisheries Branch, B.C., Ministry of Environment, Fisheries and Oceans Canada B.C., Ministry of Agriculture and Lands and Heiltsuk Tribal Council).
Timmer B., Reshitnyk L. Y., Hessing-Lewis M., Juanes F., Costa M. (2022). Comparing the use of red-edge and near-infrared wavelength ranges for detecting submerged kelp canopy. Remote Sens. 14, 2241. doi: 10.3390/rs14092241
Vergés A., Doropoulos C., Malcolm H. A., Skye M., Garcia-Pizá M., Marzinelli E. M., et al. (2016). Long-term empirical evidence of ocean warming leading to tropicalization of fish communities, increased herbivory, and loss of kelp. Proc. Natl. Acad. Sci. U.S.A. 113, 13791–13796. doi: 10.1073/pnas.1610725113
Wachmann A., Starko S., Neufeld C. J., Costa M. (2024). Validating landsat analysis ready data for nearshore sea surface temperature monitoring in the northeast pacific. Remote Sens. 16, 920. doi: 10.3390/rs16050920
Watson J., Estes J. A. (2011). Stability, resilience, and phase shifts in rocky subtidal communities along the west coast of Vancouver Island, Canada. Ecol. Monogr. 81, 215–239. doi: 10.1890/10-0262.1
Wernberg T. (2021). “Marine Heatwave Drives Collapse of Kelp Forests in Western Australia,” in Ecosystem Collapse and Climate Change. Eds. Canadell J. G., Jackson R. B. (Springer International Publishing, Cham), 325–343. doi: 10.1007/978-3-030-71330-0_12
Wernberg T., Krumhansl K., Filbee-Dexter K., Pedersen M. F. (2019). “Chapter 3 - Status and Trends for the World’s Kelp Forests,” in World Seas: an Environmental Evaluation, 2nd ed., vol. 57–78 . Ed. Sheppard C. (Academic Press, Cambridge, MA, USA). doi: 10.1016/B978-0-12-805052-1.00003-6
Keywords: kelp forest canopy, El Niño, marine heatwave, resilience, persistence, scale-dependent responses, sea surface temperature, Pacific Decadal Oscillation (PDO)
Citation: Gendall L, Hessing-Lewis M, Wachmann A, Schroeder S, Reshitnyk L, Crawford S, Lee LC, Guujaaw N and Costa M (2025) From archives to satellites: uncovering loss and resilience in the kelp forests of Haida Gwaii. Front. Mar. Sci. 12:1504701. doi: 10.3389/fmars.2025.1504701
Received: 01 October 2024; Accepted: 18 February 2025;
Published: 04 April 2025.
Edited by:
Christopher Edward Cornwall, Victoria University of Wellington, New ZealandReviewed by:
François Thoral, University of Waikato, New ZealandCopyright © 2025 Gendall, Hessing-Lewis, Wachmann, Schroeder, Reshitnyk, Crawford, Lee, Guujaaw and Costa. This is an open-access article distributed under the terms of the Creative Commons Attribution License (CC BY). The use, distribution or reproduction in other forums is permitted, provided the original author(s) and the copyright owner(s) are credited and that the original publication in this journal is cited, in accordance with accepted academic practice. No use, distribution or reproduction is permitted which does not comply with these terms.
*Correspondence: Lianna Gendall, bGlhbm5hLmdlbmRhbGxAdXdhLmVkdS5hdQ==
Disclaimer: All claims expressed in this article are solely those of the authors and do not necessarily represent those of their affiliated organizations, or those of the publisher, the editors and the reviewers. Any product that may be evaluated in this article or claim that may be made by its manufacturer is not guaranteed or endorsed by the publisher.
Research integrity at Frontiers
Learn more about the work of our research integrity team to safeguard the quality of each article we publish.