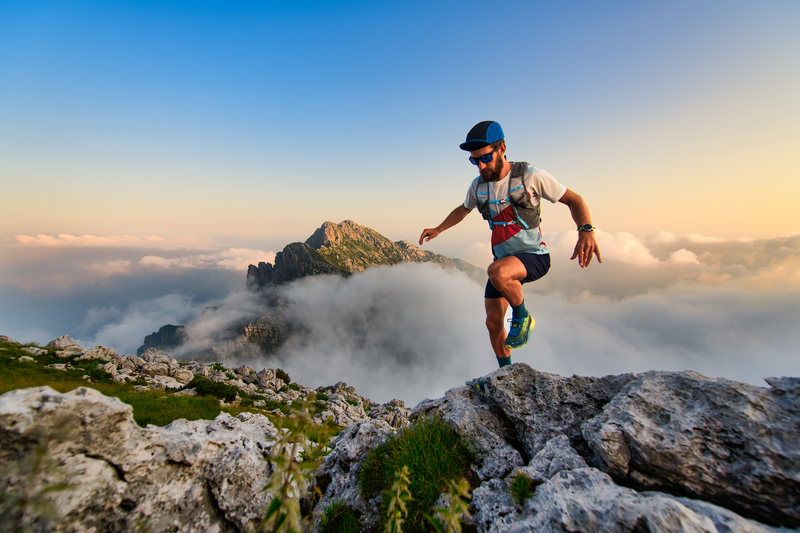
94% of researchers rate our articles as excellent or good
Learn more about the work of our research integrity team to safeguard the quality of each article we publish.
Find out more
ORIGINAL RESEARCH article
Front. Mar. Sci.
Sec. Marine Fisheries, Aquaculture and Living Resources
Volume 12 - 2025 | doi: 10.3389/fmars.2025.1467442
This article is part of the Research Topic Challenges in Fishery Assessment Methodologies View all 11 articles
The final, formatted version of the article will be published soon.
You have multiple emails registered with Frontiers:
Please enter your email address:
If you already have an account, please login
You don't have a Frontiers account ? You can register here
Traditionally, parameters defining life history traits, such as growth, were solely determined through length or age-length databases and then included as fixed in integrated stock assessment models. In current practice, growth parameters are usually estimated within these models (“inside”) and fitted to other datasets. However, for short-lived and small pelagic species, challenges may arise, particularly when there is a high variability in the age-length data or sampling biases are inadequately identified or addressed by these models.To test model effectiveness in capturing the growth dynamics of these species, we propose a comparative analysis following recommended practices for incorporating age-length data into integrated stock assessment models for the specific case of anchovy (Engraulis encrasicolus) stock in the Gulf of Cadiz. The reason is twofold: its significant ecological and economic importance, and the need to improve the accuracy of growth parameter estimates used to inform Total Allowable Catch (TAC) scientific advice.The overarching goal of this analysis is to identify the optimal model configuration that provides accurate growth parameter estimates. Our approach shows that random effects can effectively estimate growth in species with high age-length variability. Furthermore, using the obtained estimates as fixed in the stock assessment model, reduces computational time and enhances the goodness of fit, resulting in a more efficient model. The results address a significant gap in existing integrated models used for scientific advice, which often do not have the “random effects on parameters” feature. Notably, this framework is widely applicable to other short-lived small pelagic species that typically exhibit a high data variability, offering a valuable solution for improving efficiency and robustness in fisheries management decision-making.
Keywords: growth1, anchovy2, age-length3, integrated stock-assessment models4, Gulf of Cádiz5
Received: 19 Jul 2024; Accepted: 10 Feb 2025.
Copyright: © 2025 Rincón Hidalgo, Gamaza Márquez, Zúñiga Basualto, Ramos and Tornero. This is an open-access article distributed under the terms of the Creative Commons Attribution License (CC BY). The use, distribution or reproduction in other forums is permitted, provided the original author(s) or licensor are credited and that the original publication in this journal is cited, in accordance with accepted academic practice. No use, distribution or reproduction is permitted which does not comply with these terms.
* Correspondence:
Margarita María María Rincón Hidalgo, Oceanographic Center of Cádiz,Spanish Institute of Oceanography (IEO), Cádiz, 11006, Spain
Disclaimer: All claims expressed in this article are solely those of the authors and do not necessarily represent those of their affiliated organizations, or those of the publisher, the editors and the reviewers. Any product that may be evaluated in this article or claim that may be made by its manufacturer is not guaranteed or endorsed by the publisher.
Research integrity at Frontiers
Learn more about the work of our research integrity team to safeguard the quality of each article we publish.