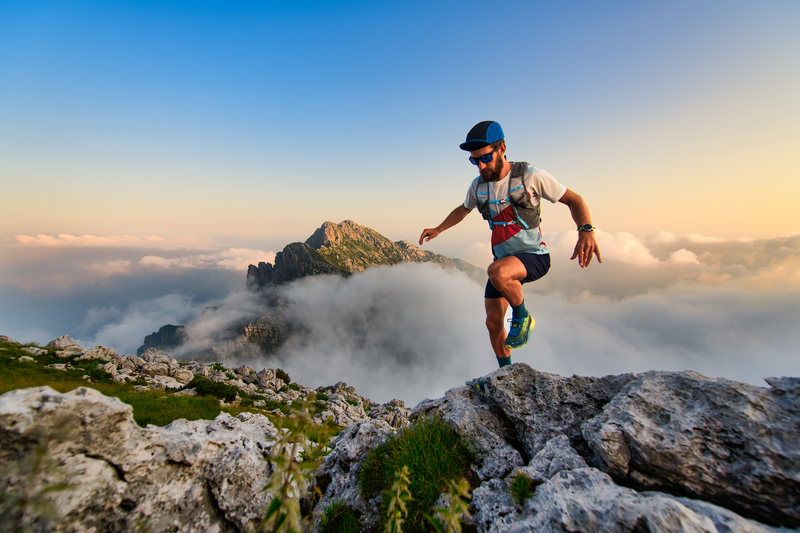
95% of researchers rate our articles as excellent or good
Learn more about the work of our research integrity team to safeguard the quality of each article we publish.
Find out more
ORIGINAL RESEARCH article
Front. Mar. Sci. , 22 January 2025
Sec. Marine Affairs and Policy
Volume 11 - 2024 | https://doi.org/10.3389/fmars.2024.1516586
This article is part of the Research Topic Emerging Computational Intelligence Techniques to Address Challenges in Oceanic Computing View all 4 articles
Intelligent ship monitoring technology, driven by its exceptional data fitting ability, has emerged as a crucial component within the field of intelligent maritime perception. However, existing deep learning-based ship monitoring studies primarily focus on minimizing the discrepancy between predicted and true labels during model training. This approach, unfortunately, restricts the model to learning only from labeled ship samples within the training set, limiting its capacity to recognize new and unseen ship categories. To address this challenge and enhance the model’s generalization ability and adaptability, a novel framework is presented, termed MultiAngle Metric Networks. The proposed framework incorporates ResNet as its foundation. By employing a novel multi-scale loss function and a new similarity measure, the framework effectively learns ship patterns by minimizing sample distances within the same category and maximizing distances between samples of different categories. The experimental results indicate that the proposed framework achieves the highest level of ship monitoring accuracy when evaluated on three distinct ship monitoring datasets. Even in the case of unfamiliar ships, where the detection performance of conventional models significantly deteriorates, the framework maintains stable and efficient detection capabilities. These experimental results highlight the framework’s ability to effectively generalize its understanding beyond the training samples and adapt to real-world scenarios.
Ships are an integral component of the marine transportation system, serving crucial roles in international trade, ocean development, and various other maritime activities. With the diversification of marine mission requirements, ship types and styles have become increasingly diverse. Each mission necessitates ships of varying sizes, structures, functions, and technical equipment to cater to specific marine application scenarios. The increasing variety of ship types and styles poses fresh challenges for the generalization capabilities of existing port management and ship monitoring systems. To address these challenges, there is a pressing need for the development of advanced and adaptable technologies and methodologies. These advancements are essential to ensure the accurate detection and classification of the ever-increasing number of ship types and styles within the maritime domain. Meanwhile, deep-learning-based ship monitoring can be used as a complement to Automatic Identification System (AIS) as an important line of defense for maritime regulatory systems. In addition, a regulatory system with a high degree of generalization not only helps to improve marine monitoring and protection (Almpanidou et al., 2021) but also improves ship management (Zhang et al., 2021) and other works as well as efficient management turnaround efficiency, which can bring considerable benefits for maritime management work.
Automatic ship monitoring models using deep learning techniques are the mainstream nowadays, so the visible-light-based automatic ship monitoring approach has become a major research hotspot in the field of marine monitoring and maritime management in recent years. The ship recognition models used today can be divided into three major categories (Bo et al., 2021), such as target-based recognition models, target detection models, and image slicing models. However, the traditional deep learning model training strategy is still used in the ship monitoring task, which is still plagued by the following four aspects: For one thing, when using the traditional strategy, only ships with predefined categories of characteristics can be recognized, and it is difficult to accurately detect and classify emerging ship types that are not included in the training data. In the context of the diversification of ship types and shapes, there are higher requirements for the model. Second, when relying on only a small number of samples for training, due to the limited number of samples, the model can easily be overfitted on the training set (Tan et al., 2021), resulting in poor generalization of new samples in the prediction stage. Furthermore, the improved generalizability of traditional strategies usually relies on a large amount of labeled data to train the model, and in the maritime domain, obtaining as well as labeling large-scale data is difficult, time-consuming, and expensive (Del Prete et al., 2023), thus limiting the model’s ability to be applied under fewer samples. Lastly, in the current context of ever-diversifying ship types and styles, in order for the model of the traditional strategy to have the ability to discriminate new ships, it needs to adapt its training data by adding a large number of new categories of data while simultaneously labeling and retraining the model. However, this strategy is less efficient. This leads to the problem of lack of generalization of the sample types of ship monitoring datasets at this stage. To a greater extent, it limits the applicability of the model in real maritime management and fails to meet the practical application needs of the maritime management industry.
Thus, in order to solve the problems of existing ship monitoring models in terms of data requirements and performance, such as relying on a large amount of labeled data during training and being prone to overfitting resulting in poor generalization ability on new samples, there is an urgent need to develop a new model to classify and recognize ship types. This model is able to be trained using an existing publicly available ship dataset with a small number of known categories to achieve a model with the ability to discriminate between a larger number of unseen types of ships, and a model comparison of the traditional and less-sample strategy is shown in Figure 1.
Figure 1. Comparison of strategies for all-type ship classification models and traditional deep learning ship classification models.
In response to the problem that the current ship monitoring model training relies on large-scale labeled datasets and does not have the ability to predict and recognize new samples, which makes the model difficult to be applied to the task of the complex marine environment and diverse ship types, this paper proposes a novel model for unfamiliar ship type recognition in ship monitoring in real-world scenarios: a multi-angle metric networks (AMAM-Net), which is an end-to-end recognition model based on multi-scale feature information that can be learned quickly using fewer samples, and can be generalized to all types of ships. In a real ocean environment, when the model faces a new category of samples, it can define a mapping function to enable it to learn to map known samples of different categories into a low-dimensional feature space so that the samples of different categories are distinguishable in the feature space and the prediction of new samples can be performed by calculating the similarity between the categories of sample metrics. During the testing phase, the new unseen samples are categorized instead into the most similar known categories by calculating the distance between a small number of samples from known categories and the new unseen samples. The goal of this strategy is to make the model independent of sample label-based classification methods so that it can accurately identify and classify a variety of emerging ship instances in a real marine environment.
Experimental results on one realistic maritime ship inspection dataset and two full type classification ship inspection datasets show that AMAM-Net achieves the most efficient ship identification accuracy compared to the traditional model, allowing our implementation to maintain stable monitoring performance in the face of samples of never-before-seen ship categories. In addition, we conducted a series of complementary experiments as a way of evaluating the effectiveness of the strategies used by AMAM-Net. In summary, the main contributions of this study are as follows:
● This paper proposes a triple metric neural network based on multi-scale feature information for all kinds of ship image classification. The method is experimented on different ship datasets and its accuracy is improved by 15.14% compared with other classical deep learning modeling methods.
● In addition, the experimental results improved to 83.23% and 61.41% accuracy relative to the traditional deep learning model approach in the coarse classification task (nine categories) and the detailed classification task (73 categories) in the multi-species ship inspection dataset, achieving 94.61% and 61.54% classification accuracy, respectively, thus demonstrating the generalized performance benefits of AMAM-Net in real maritime management applications.
● The proposed method is a new multi-scale ternary loss function and multi-scale similarity measure comparison method, which effectively improves the ability of the model to capture the local and global features of the image, reduces the error and bias of a single measure, avoids the defects of the existing methods of the insensitivity of the scale and the insufficient consideration of the relative relationship of the samples, and thus improves the robustness of the system.
● Provided a new ship detection dataset that contains multiple categories of ships with both coarse and fine categorization of ship types. Compared with the existing ship inspection dataset, this dataset is more in line with the actual marine environment. The number of ship types increased from five to 73 categories, a 14.6-fold increase. This dataset can better validate the robustness and generalization performance of the model in solving the ship detection task.
The rest is presented in the following sections. Firstly, in Section 2, the state-of-the-art research on traditional ship monitoring methods as well as automatic ship monitoring models based on image recognition is presented. In Section 3, AMAM-Net is described in detail, including the problem statement, network architecture, and ternary generator loss function metric function. Section 4 discusses the experiments and analyzes the results. Finally, the main contributions of this work to maritime management are summarized in Section 5, and future research work to be carried out in depth is presented.
Finally, the main contributions of this work to maritime management are summarized in Section 5, and future research work to be carried out in depth is presented. Our codes are publicly available at https://github.com/jiahuasun03/Unfamiliar-Ship-Type-Recognition.
In this chapter, the research work in the related field in recent years is reviewed, which can be categorized into two main types of research directions: traditional ship monitoring methods and current deep-learning-based ship monitoring methods.
The most notable characteristic of traditional ship monitoring systems is that they rely heavily on onboard radio communication equipment and are usually only able to monitor ships that are normally equipped with such equipment. Much of the previous work has examined how maritime surveillance can be carried out by means of shore-based or airborne radar and Automatic Identification System (AIS) equipment, often with the aim of optimizing the data obtained by these means to improve the accuracy of maritime surveillance management.
Radar has also played an important role in maritime surveillance during the earlier years. Chuang et al. (2015) proposed attempts to identify ship types using adaptive detection techniques by extracting ship echoes in a cross-spectral series of sea surface echoes to HF radar signals. Park et al. (2016) proposed a method for a compact high-frequency (HF) radar system optimized primarily for the observation of surface radial flow velocities and ship orientation for improved performance in detecting ships. da Silva et al. (2022) presented and discussed four methods to estimate the extended target DOA angle to obtain ship detection target results in real maritime scenarios. Shi et al. (2022) proposed an effective method of acquired radar echoes to acquire and analyze m-D signals and extract ship motion parameters on time-varying sea surface for ship target detection and identification in marine environment. In addition, in a follow-up study, it was found that the performance of the monitoring method using AIS was superior to that of the monitoring method using radar (Vesecky et al., 2009). In comparing the performance of the AIS equipment monitoring method and high-frequency radar monitoring method, it was found that high-frequency radar may have situations such as misdetection, but the AIS equipment can only identify a part of the vessels, so it is proposed to combine Bayesian network with AIS and high-frequency radar, respectively, to realize the priority identification of whether there are vessels in a specific monitoring area so as to increase the regional target detection efficiency. Eriksen et al. (2006) used a space-based AIS receiver to monitor vessels at sea, and the effective distance of a space-based AIS receiver communicating in near-Earth orbit is more than 1,000 nautical miles, which is an advantageous condition to monitor a wide range of sea areas. Chaturvedi et al. (2012) conducted a study on maritime surveillance by combining AIS data and recognized and analyzed the vessel targets in the collected TerraSAR-X (R) images and AIS data in order to further identify the vessel targets that distinguish between “enemy” and “friendly” identities in a wide range of sea areas, which helps to determine whether the vessel poses a threat to navigation safety in the sea area or not. Meanwhile, Duan et al. (2022) proposed a semi-supervised deep learning approach for ship trajectory classification via ships based on ship AIS data. In addition, compared with AIS, FMCW radar can detect some small vessels without AIS. This approach expands the scope of maritime monitoring and helps to monitor the vast ocean in real time to improve the safety of marine shipping. Therefore, Hong and Yang (2013) utilized the FMCW radar’s all-weather and low-power operating characteristics to monitor real-time vessel activity information.
In general, although the method of using shipboard electronic communication equipment to monitor ships mentioned above has achieved certain effective results in general, this method shows a high degree of dependence on communication equipment. When the shipboard radio communication equipment fails to work properly, the relevant maritime management department is difficult to obtain timely information about the activities of the ship at sea, which makes the initiative of maritime monitoring and management subject to greater restrictions, and fails to effectively solve the problems of maritime work in the real environment.
In recent years, with the development of deep learning technology and computer vision technology, neural network models are now dominating the application of sea area perception (Thombre et al., 2022) due to their superior ability to fit the data. Compared with the traditional ship monitoring methods, the methods of deep-learning-based ship monitoring have made greater progress in the accuracy of ship recognition. The current ship recognition models mentioned above can be categorized into three types of modeling strategies.
Firstly, based on the target recognition technique, in order to solve the problem that the huge imbalance in the number of samples of different scenes leads to a serious degradation of the ship detection accuracy of synthetic aperture radar (SAR), Zhang et al. (2020) proposed a balanced scene learning mechanism (BSLM) for offshore and nearshore ship detection in SAR images. In view of the limitations such as under-utilization of available information for maritime information, Li et al. (2023b) and Jiang et al. (2024) proposed a two-way integrated ship monitoring system based on knowledge migration that integrates remote satellite equipment and nearshore detection equipment. Wang et al. (2023) proposed a SAR ship recognition method that enhances the features at each scale through multi-scale feature focus and adaptive weighted classifier and adaptively selects effective feature scales for accurate recognition. Yasir et al. (2023a), by incorporating the C3 and FPN + PAN structures, as well as attention mechanisms, successfully addressed the performance degradation caused by complex background interference, variations in ship sizes, and blurred features of small ships in synthetic aperture radar (SAR) ship detection. Nie et al. (2017) used single shot multi box detector as the basic architecture in order to improve the prediction accuracy of distinguishing different types of ships by different levels of feature maps. Chen et al. (2022) discusses proposing a new method for fast small ships using degradation-based reconstruction enhancement for medium-resolution (MR) remote sensing (RS) image. Zeng et al. (2021) investigated ship classification by dual-polarized SAR, and their model was able to efficiently classify ships into eight accurate categories such as cargo, tanker, carrier, container, fishing, dredger, tug, passenger, etc. Połap et al. (2022) proposed an image classifier based on artificial intelligence techniques for automatic ship classification in monitoring systems. The method employs a combination of cascading classifiers and reward-penalty mechanisms that can effectively classify ships. By cascading multiple classifiers, the accuracy and generalization ability of classification can be improved.
Within the technical field of target detection, etc., in order to solve the problems of low accuracy and poor generalization ability in multi-scale ship detection, Hu et al. (2022) proposed a framework of anchorless network using balanced attention (BANet) for multi-scale ship detection in SAR images. Aiming at the performance problem of maritime ship monitoring when in low visibility environments, a learning parameter sharing (LPS) approach was adopted, and Qu et al. (2023) proposed an Image Enhancement Monitoring Network (LPSNet). Meanwhile, Li et al. (2023a) proposed a ship detection model called the Leap-Forward-Learning-Decay and Curriculum Learning-Based Network that combines an innovative strategy of leap-frog learning rate decay and a curriculum-based learning strategy to enhance its detection performance, making it more suitable for maritime monitoring in real-world environments. Zha et al. (2022) proposed a novel ship detection model based on multi-feature transformation and fusion (MFTF-Net) to solve the monitoring performance problems such as missed detection and false detection. Meanwhile, this technique can also be applied to the management of ship’s deadweight tonnage. Hou et al. (2023) proposed a method to manage the ship’s deadweight tonnage by using the rotationally invariant task-aware spatial de-entanglement (RITSD) algorithm. This method implements a method of obtaining regression formulas for the ship’s deadweight tonnage (DWT) as well as its shape by analyzing high-resolution satellite images. This new method provides a new way for maritime transportation monitoring and we can better manage and monitor maritime transportation activities. Zhang et al. (2023) employed the ghost module and transformer to enable the model to achieve fast and accurate object detection capabilities even in complex maritime environments.
Finally, the semantic slicing domain aspect technology, Cui et al. (2019) utilized a novel multi-scale ship detection method based on SAR images by densely refining the Convolutional Block Attention Module (CBAM) tandem feature maps in Pyramid Networks (DAPNs), which can extract a wide range of resolving and semantic information to achieve high accuracy ship monitoring. Finally, in the technical field of image semantic segmentation, for example, in order to solve problems such as the lack of consideration of the characteristics of the target ship task leading to low overall accuracy, Shao et al. (2023) proposed a scale-in-scale (SIS) idea for SAR hull instance segmentation, and Huang and Li (2021) proposed a hybrid task for improved ship instance segmentation cascade enhanced model (Zhang and Zhang, 2022). Huang and Li (2021) proposed a new directional silhouette matching network architecture using multi-scale features and instance-level masks for realizing ship instance segmentation under a single sample and without anchor frames. Roy et al. (2023) proposed a deep learning-based model that is sufficient to classify ships and no ships and to localize the ships in the original image using the bounding box technique. Yasir et al. (2023b), by optimizing the network architecture design, network feature fusion structure, and improving the feature optimization module, achieved a more precise and efficient ship instance segmentation in high-resolution ship remote sensing images. In addition, a deep learning-based autoencoder model is also utilized to segment the classified ships again, which helps in the early identification of possible threats at sea.
The abovementioned work provides an effective reference for improving the accuracy of ship monitoring, which is an important achievement of research in the field of maritime monitoring. At the same time, the abovementioned research works are still all discussed from the perspective of traditional deep learning tasks, and the research on the robustness and generalization ability of deep learning-based ship detection models in ship type recognition is still relatively lacking, which greatly limits the feasibility as well as practicality of the models’ application in real nearshore maritime management.
The overall workflow of the AMAM-Net network proposed in this paper is divided into two phases, containing modules such as triad generation module, triple feature extraction module, multi-scale ternary loss function, and multi-scale similarity measure module, as shown in Figure 2 below. The first phase is called the triad pre-training phase, in which we first train the network model on a large-scale classification dataset and utilize the transfer learning technique in order to improve the performance of detecting ships near the shore. Subsequently, a triplet generator is utilized to generate a preprocessed dataset with multiple triplets based on the categories of the ship images, where each triplet consists of an anchor sample, a positive example sample, and a counterexample sample, where the anchor sample and the positive example sample belong to the same ship category, and the counterexample sample belongs to a different ship category. Then, this dataset containing multiple triples is fed into the feature extractor with ResNet34 as the backbone, respectively. The final pooling layer is modified to set the fully connected layer output dimensions as a way to output dimension-specific feature vectors for each of the three samples, mapping these samples into a low-dimensional feature space. The difference between these distances is measured in the low-dimensional feature space by defining a multiscale ternary loss function. Through the backpropagation algorithm, the model can update the network parameters based on the gradient information of the loss function, thus gradually optimizing the learning process of feature representation, while in the second all-type evaluation phase after the training is completed, it consists of the triple feature extraction module that has been trained with the composition of the AMAM-Net network and can be used to predict the class of unknown samples. For an unseen query sample, its feature representation is extracted by the feature extractor and compared with the feature representation of the known categories in the support set. By computing the multi-scale similarity between the two vectors of the comparison, the new sample can be matched to the category that is most similar to its feature representation.
Subsequently, a comprehensive description of this AMAM-Net network model is presented, including the problem statement, ternary generator, ternary feature extractor, multi-scale triplet loss function, and multi-scale similarity measurer.
In this study, we treat the task as a supervised classification problem aiming at recognizing ship types in images. The model aims to classify ship types displayed in ship profile images captured by an optical nearshore surveillance camera. In the provided dataset, , corresponds to the kth ship outboard profile image, the category of the ship image is represented by the label , and N denotes the total number of images in the dataset. The main objective of this work is to predict the category of ships and the output of this task can be expressed as .
The preparation of data for the AMAM-Net network necessitates initial passage through a triad generator, which processes a raw dataset accompanied by corresponding labels. Each category within the dataset encompasses a multitude of sample images, each annotated with a label that signifies its respective category. The triad generator is pivotal in the training regimen of the AMAM-Net network as it fabricates triplets that are indispensable for the acquisition of a discriminative feature representation. A triplet comprises an anchor sample , a positive example sample hailing from the same category, and a negative example sample originating from a disparate category. The theoretical underpinning of triplets is predicated on their capacity to instruct the model in learning an embedding space where samples from the same category are more proximate to one another than to those from divergent categories. Following the ingestion of the entire training dataset into the triad generator, it becomes imperative to forge pairs of positive and negative examples predicated upon the anchor sample categories. For each anchor sample, a sample from a distinct category must be randomly selected to serve as a counter-example sample . An exemplar of triadic preprocessing data is delineated in Figure 3. The triad generator will then construct triplets from these positive and negative example pairs, which will subsequently be utilized to train the model. This training endeavors to align the positive example samples more closely with the anchors, ensuring that the embeddings of the negative example samples are more distant from the anchors than those of the positive examples. The dataset is amalgamated by integrating the generated triplets into a data subset that encompasses anchor point samples, positive examples, and counterexample samples, all of which are instrumental in training the deep learning model. This methodology capitalizes on the triplet loss function, which is engineered to attenuate the distance between the anchor and positive samples while amplifying the distance to the negative samples. This enforces a relative distance constraint that is advantageous for the learning of a salient feature space. Concurrently, data augmentation methodologies are employed to enact a spectrum of transformations and expansions upon the training data, thereby enhancing the performance and generalization capabilities of the model to a notable extent. The operational specifics of the triad generator are articulated in Figure 3, Algorithm 1.
In order to realize the effective monitoring of ships near the shore, we input the output from the triad generator: the i-th triad containing into the triple feature extraction module for feature extraction, and we utilize the basic module of the residual network as the backbone network. In the triple feature extraction module, the samples of each ternary are first fed into the corresponding feature extractor separately, and the ship image information is fed to the convolutional layer for higher-level representation. The convolutional layer is the core layer of the convolutional neural network (CNN), which extracts the information by convolutional kernel scanning. The formula for the convolutional layer is shown below:
where s is the feature obtained by extracting the image, x is the input of the image to the convolutional layer, w is the weight of the convolutional kernel, i, j are the dimensions of the extracted information, and m, n are the dimensions of the convolutional kernel. Finally, the last pooling layer of the original ResNet34 is removed and the fully-connected layer is modified to output dimension-specific feature vectors to obtain the most representative features for each image sample. In addition, the triple feature extraction module performs sample feature extraction as shown in Algorithm 2.
In this paper, multi-scale triplet loss is used as a loss function for model training by minimizing the distance between samples of the same category and maximizing the distance between samples of different categories, even in the absence of label information. Compared to contrastive loss (Hadsell et al., 2006), it can more fully utilize the information between samples and provide richer similarity information. Also, to more fully utilize the relative relationship between the three samples, the loss function can be defined by a variety of different scales of distance (Euclidean, cosine distance, etc.). The Triplet Loss formula is as follows:
where is the total loss function, which weights and sums the loss values of multiple scales, represent different weight parameters to balance the influence of each distance measure, Margin is the minimum interval between the distance of and to prevent the loss value from being too small, and + means that when the value in [] is greater than zero, the value is taken as the loss.
where is the Euclidean distance metric between the anchor sample and the positive sample, is the Euclidean distance metric between the anchor sample and the negative sample; is the cosine distance metric between the anchor sample and the positive sample, and is the cosine distance metric between the anchor sample and the negative sample. The samples are the inputs for each ternary, k is the number of input image ternaries, and is the mapping function of the network, and the size of the whole training set is N.
In the prediction stage, the optimal model is tested by calling the trained optimal model, and the support set and query set of all kinds of ship detection: , are inputted into the feature extractor in order to obtain the feature vectors of the support and query samples, and the mean operation is performed on the support samples of the same type, which is later imported into the multi-scale similarity metric module. For the scale features, using measures such as Euclidean distance, cosine similarity, etc., the similarity of each scale similarity value is summed by adding factors with different weights (λ, µ, etc.) to obtain similarity, and the multidimensionality metric module is used to match the feature vectors obtained from the support set and the query set, and the group with the maximum similarity is selected as the predicted category label, and the comparison process is shown in Figure 4 as follows, and finally complete the image classification as follows:
Figure 4. Multi-scale similarity metrics module for the few-sample case. The support sample points used for comparison are computed based on the average of the multiple support sample vectors (dashed lines) under each class, and the similarity is computed by the distance relationship between them.
where λ, µ… are different weight parameters to balance the influence of each distance measure; is the similarity value between and samples, which is used as the confidence level of each category, and yi is the category with the largest cosine similarity value in each group as the predicted category label of the samples, and the specific operation is shown in Algorithm 3.
This section describes the experimental results and details of the AMAM-Net network, including the construction of the experimental dataset, the configuration of experimental parameters, the evaluation metrics, and the sensitivity analysis of the network parameters. Experiments are then conducted and compared between AMAM-Net and other methods. To demonstrate the effectiveness of AMAM-Net, we finally apply the enhanced results to real-time images captured by realistic nearshore surveillance equipment for different types of ship detection tasks.
In this study, a total of four ship detection datasets, the adapted deep learning ship dataset, the realistic environment ship detection dataset, and two multi-category ship detection datasets based on coarse versus fine classification, respectively, are used to train or evaluate the performance of AMAM-Net. The original deep learning ship dataset is a publicly available deep learning image task dataset, consisting of 6,252 images of five types of ships, with data examples of the set as well as the statistics shown in Table 1, respectively. The dataset contains a large number of high-quality images with clear contrast and sharp focus and a sufficient number of images of each type to make it a suitable choice for training deep learning models. In addition, because the original dataset has a wide range of classification criteria, resulting in a category containing a variety of ships belonging to the same broad category, such as the cargo ship category that contains multiple samples such as general cargo ships, bulk carriers, container ships, etc., which causes some trouble for the similarity learning of the model, so we have been careful in processing of the same type of ships to be extracted, as shown in Table 1, and ultimately obtained a training dataset with seven categories of ships with a large number of image samples in the training datasets. Our dataset is publicly available at https://doi.org/10.6084/m9.figshare.27874146.
On the other hand, the Realistic Maritime Ship Inspection Dataset and the Full Type Classification Ship Inspection Dataset, which has both detailed and rough classification criteria, are both datasets with small data volumes, which we specifically collected to test as well as to evaluate the robustness and generalization ability of each model. The image quality of the Realistic Maritime Ship Inspection Dataset better reflects the realism of the conditions during the nearshore inspection task and includes a variety of weather-related factors, but with categories that are known to the model at the time of training, thus providing a more accurate and representative assessment of the robustness of the models. The Full Type Classification Ship Inspection Dataset, on the other hand, focuses on the diversity of ship types and contains a nine-category classification task dataset for the rough classification of ships and a 73-category classification task dataset for detailed classification, respectively. The main purpose is to verify whether the model has better generalization ability in the face of more new unseen categories. The data samples in the above datasets were captured using optical surveillance cameras. Compared with the deep learning blood vessel dataset, the images in this dataset are more realistic and can effectively reflect the robustness and generalization ability of the model. The statistics of these four datasets are listed in Tables 1, 2, respectively, and the visual representation of the samples in the dataset is provided in Figure 5 accordingly.
Figure 5. Sample images of a randomly selected portion of the full type classification ship inspection dataset.
We used a pre-trained ResNet 34 model on the ImageNet dataset as the backbone of the AMAM-Net monitoring module. The entire model was optimized using a multi-scale ternary loss function to combine multiple ternary distance measures in a weighted manner. We empirically set the batch size to 32 and the learning rate to 0.001. Based on the experimental results, average stochastic gradient Descent (ASGD) was used as the optimization algorithm, the spacing parameter value (margin) was set to 0.875, and the feature dimension vectors with a nominal dimension value of 2,048 dimensions were selected. The experiments were performed on a computer including a 64-bit Windows 11 operating system, a 12th generation Intel Core i7-12700 processor, 32 GB of RAM, and NVIDIA GeForce RTX 3060. The PyTorch deep learning framework, version 11.7, was used, with PyCharm as the main software compilation tool and Python 3.9 as the programming language.
The AMAM-Net ship identification performance is evaluated quantitatively in terms of accuracy, precision, recall, and F1, which are as follows:
where we use a number of terms to describe the accuracy of the prediction results. When a positive sample is correctly predicted as positive, it is called true positive (TP). Similarly, when we correctly predict a negative sample as negative, it is called true negative (TN). However, when incorrectly predicting a negative sample as positive, we call it false positive (FP). Conversely, when we incorrectly predict a positive sample as negative, we call it false negative (FN). The abovementioned metrics can help us evaluate the accuracy and performance of the model.
In order to deeply investigate the performance of AMAM-Net network in terms of robustness, stability, and migration on different data, AMAM-Net is compared with a variety of widely used image classification models based on deep learning, including the well-known LeNet, AlexNet, VGGNet, ResNet, GoogleNet, DenseNet, and MobileNet models. MobileNet and other models. All baseline models are tested for robust performance on the Realistic Maritime Ship Inspection Dataset, while the generalization performance is evaluated on the Full Type Classification Ship Inspection Dataset. The abovementioned models are chosen because they have shown excellent results in many image classification tasks and have the potential to achieve excellent results in detection tasks as well.
The comparative experimental results given in the left side of Table 3 show that AMAM-Net has excellent monitoring accuracy in the known sample set. Using the AMAM-Net that has been trained on the Preprocessed Deep Learning Vessel Dataset, in the Realistic Maritime Ship Inspection Task, there is no need to re-train it, relying only on the Realistic Maritime Ship Inspection Dataset to extract five samples as the support set. With Ship Inspection Dataset as the support set, the detection accuracy of AMAM-Net is improved by up to 37.855% to 87.006% compared with baseline models in the classification task, which proves that it can more accurately predict the category of the samples and effectively reduce the misclassification and improve the classification accuracy.
In addition, we also analyzed the model accuracy and recall, and the experimental results are shown in Figure 6. It can be seen that the average-AUC and average-PR values of AMAM-Net are significantly higher than those of other models, and its average-AUC and average-PR values are 0.96 and 0.90, respectively, which indicate that our model has a high level between accuracy and recall. Because of the high cost of misclassification situations in maritime surveillance management tasks, automatic detection models are required to maintain high accuracy while maximizing recall. In contrast, the average-AUC and average-PR of LeNet are only 0.66 and 0.37, respectively, which make it difficult for it to maintain a good prediction ability in the maritime monitoring task in the real environment.
In order to further verify the superiority of the generalization performance of the AMAM-Net network, we purposely collected a ship image inspection dataset with more categories, aiming at comparing the deeper generalization performance of the models, which is demonstrated in the middle as well as the right side of Table 3. In the Full Type Classification Ship Inspection Dataset, the AMAM-Net network still maintains a high detection and classification performance on the large-scale category classification problem. The detection accuracies of 94.614% and 75.013% are achieved in the rough classification with nine categories and the detailed classification with 73 categories, respectively, and the detection accuracies are improved by 83.917% and 63.897% on average compared with baseline models, which shows that the AMAM-Net model can be used for large-scale ship classification in the face of the more challenging tasks. The test results show that the AMAM-Net network model, when faced with the more challenging task of large-scale ship classification, can obtain the ability to discriminate many new, unseen, and unfamiliar ship types with only a small number of support samples, and the generalization performance of AMAM-Net has been greatly improved compared with many baseline models. In addition, some baseline models, such as LeNet-5, which was originally designed for handwritten digit recognition tasks, cannot be directly scaled up to adapt to more categories of classification tasks due to the fact that the network structure of these models, as well as the size of the output layer, is fixed. Because its network structure cannot accommodate these larger numbers of categories, it was not possible to experiment with these baseline models and obtain their performance metrics in this experiment.
We used the confusion matrix to check the prediction results in the deep learning model using the traditional strategy and in AMAM-Net. The experimental results in the error analysis in The Realistic Maritime Ship Inspection Task are shown in Figure 7. In the Realistic Maritime Ship Inspection Task, accurately distinguishing between cargo and tankers is a problem with some difficulty for both the baseline model and AMAM-Net. Typically, the baseline model tends to make errors in the prediction of these two types of vessels and is more inclined to incorrectly label tankers as cargo. In contrast, our proposed model performs better in the identification of tankers, with only 5.9% of cargo samples being incorrectly categorized as tankers. At the same time, there are some results from our model that show the 12.7% misclassification rate in predicting cargo as tankers. In addition, our model also achieved a high accuracy rate in recognizing cruise. Whereas the baseline model is more likely to misclassify cruises as military, carrier, or tankers, our model has only a low probability of misclassifying them as tankers.
Figure 7. Confusion matrices of each model. (A) LeNet, (B) AlexNet, (C) VGG11, (D) ResNet34, (E) ResNet101, (F) DenseNet121, (G) MobileNetV2, (H) GoogleNet, and (I) AMAM-Net.
We also recorded the extraction effect of the triple feature extraction module, in real time. At each stage of training, the feature vectors are extracted and output by inputting the query samples into the feature extractor, and then the output vectors are downscaled using the t-SNE method and visualized by mapping them into the three-dimensional space. As shown in Figure 8, the sample points of each category of AMAM-Net after a period of time of learning are changed gradually from highly confusing to similar clusters, and at the same time the boundary between clusters is clear. This experimental result proves the effectiveness of the triple feature extraction module. The triple feature extraction module can effectively map samples with similar features to similar locations in the feature space, and can better capture the common and different features of ship data between different types.
Figure 8. After reducing the high-dimensional feature vector to three dimensions using the t-SNE dimensionality reduction method, each sample point is labeled in the corresponding embedding space. (A) Early stage of training (accuracy: 24.71%). (B) Middle stage of training (accuracy: 58.36%). (C) End stage of training (accuracy: 93.56%).
Meanwhile, we conducted more explorations by defining a problem of N ways k shots to evaluate the model’s ability to generalize in a few-sample learning task, and the results are shown in Table 4, where the model needs to learn k samples from a support set containing N categories and make classification predictions on the query set. To investigate how different numbers of reference samples in different support sets affect ship monitoring performance for three tasks, we tested the effect of support sets containing a single, five, and 10 support sample counts on the model’s classification results. The results in Full Type Rough Classification Ship Inspection Task show that when the number of reference samples is increased from single to five, the classification accuracy of the model is improved by 19.601% to 94.614%. However, in the Full Type Meticulous Classification Ship Inspection Task, the number of categories in the query set, N, changes from nine to 73, which unsurprisingly decreases the model’s performance by nearly 30%, but as the number of reference samples continues to increase to 10, the model’s classification accuracy improves by 5.823% over 61.543%, and the model’s classification accuracy improves by 5.823% over 61.543%. Thus, in general, classification performance decreases as the number of N values increases, and the most direct way to compensate for this performance degradation is by adding k reference samples.
However, in the Realistic Maritime Ship Inspection Task, when the k-value of the support set was increased from five to 10, there was a problem that the accuracy decreased by about 1%. Due to the increase in the number of reference samples in the support set, more similar or redundant samples will appear and thus may cause the model to focus excessively on some specific samples while ignoring the feature information of other samples. At the same time, the distribution of samples between categories may overlap, leading to increased interference between categories. This makes it more difficult for the model to distinguish differences between categories, thus reducing the accuracy on the query set.
We delve into optimization algorithms for ship monitoring that are applicable to real-world environments and conduct extensive experiments on them. We tested six common optimizers such as Average Stochastic Gradient Descent (ASGD), Adaptive Moment Estimation (Adam), Adam with Weight Decay (AdamW), Adaptive Delta (Adadelta), Stochastic Gradient Descent (SGD), and Root Mean Square Propagation (RMSprop) with recorded metrics of their best performance during training. This result can provide some reference for other studies on ship monitoring tasks.
In the experimental session, we set the Margin parameter to 0.5 and the output embedding vector dimension to 128. The experimental results are shown in Figure 9, where ASGD performs best on the validation set with a large number of categories, while the optimization algorithms such as Adam, AdamW, and RMSprop are less effective. An accuracy level of 86.95% was achieved when using ASGD. The next best is SGD with 85.34% accuracy. The reason for the poor performance of algorithms such as Adam is that such optimizers usually have the feature of adaptive learning rate, but with a small sample size, the adaptive learning rate may lead to excessive fluctuations in the learning rate, which, in turn, affects the performance of the model. In contrast, the ASGD algorithm uses a fixed learning rate, which may make it easier to find a suitable learning rate for ship datasets with many categories and few samples. Meanwhile, ASGD can update the parameters by means of average stochastic gradient descent, which is conducive to more stable optimization of the model by reducing the variance of parameter updates. Therefore, ASGD optimization algorithm has better performance in the ship monitoring tasks with more types of ships mentioned in this paper.
Figure 9. Accuracy curves of the query set for different optimizers for the rough classification nearshore detection task.
In order to further investigate the effectiveness of AMAM-Net and optimize the model performance, the study of how the variation of the spacing parameter (margin) affects the multi-scale triplet loss is carried out. The experimental results show that the spacing parameter is proved to have a significant effect on how the model learns and organizes the feature space, and the optimal point of the model’s performance is when margin is equal to 0.875, and the specific experimental results are shown in Figure 10.
Figure 10. Accuracy curves of the query set for different margin values for the rough classification nearshore detection task.
However, the interval parameter setting needs to be adjusted for different task conditions, and too large an interval parameter may cause the model to have difficulty in finding an appropriate classification boundary, especially if there is noise in the dataset or there is overlap between the categories, which may lead to the model being very sensitive and prone to overfitting. At the same time, if the interval parameter is set too small, it may lead to overly loose classification criteria and the model will be insensitive to differences between samples. This may result in a model that performs well in terms of accuracy on the training set, but shows poor generalization ability in the face of new, unseen samples.
The selection of the embedding dimension is a critical decision, and we chose a range of embedding dimensions for our experiments, so in this paper, we chose values of embedding dimension sizes with a larger coverage to get a more comprehensive understanding of the effect of dimension size on the performance of the model. The experimental results show that increasing the dimensionality improves the performance of the model and that the dimensionality of the output of the fully connected layer is optimal at 1,024 dimensions. The experimental results are shown in Figure 11.
Figure 11. Accuracy curves of the query set for different margin values for the rough classification nearshore detection task.
Under the current ship detection task, setting higher dimensional feature vectors does not necessarily lead to better results. When the dimensionality is the initial dimension of 64 dimensions, the model may not be able to capture the complex relationships in the data and lose important information, leading to an insufficient expression of the semantics of the features, resulting in performance degradation. When the dimensionality is greater than 1,024 dimensions, the data becomes sparse in the high dimensional space, which may lead to a decrease in the degree of difference in the distance between the sample points, and the dimensionality catastrophe leads to an increase in the sparsity of the data, and the distance between the samples becomes even sparser, which increases the complexity of the model training and inference. In addition, when the embedding dimension is too large, the model may overfit the training data because the model can over-remember subtle features of each sample. This may result in the model performing poorly on unseen data and lacking generalization ability. This can negatively affect the training and inference of the model, and it becomes difficult to distinguish the boundaries between different categories.
The methodology and results presented in this paper can provide insights for researchers and administrators in the nearshore maritime and other related fields. For researchers in the field of nearshore maritime, our work demonstrates the following research advantages: the monitoring model training strategy approach used in this study is different from the traditional training method, which makes it possible to utilize the existing dataset supplemented with a small number of samples for all types of ships in the directions of type identification and monitoring, collision avoidance (Li et al., 2020), and target discovery and localization and provides some reference support for research. In addition, the research in this paper is the first attempt to train and predict ship monitoring models with the strategy of less sample learning. In our study, the classification accuracies using the few-sample approach strategy outperform those using the traditional deep learning strategy in a variety of prediction tasks with different quantities. This demonstrates the significance of the few-sample learning strategy for model generalization performance improvement and gives insights to researchers in this field to carry out analytical studies applied to real maritime management monitoring systems.
At the same time, for managers working in the maritime shipping industry, this study provides the following two managerial implications: (1) This study contributes to the safety management of the activities of shipping. Illegal intrusion into controlled waters and waterway smuggling have become one of the biggest hazards to shipping safety. By using our network framework to build a ship intelligent patrol system, predictive analysis of real-time collected ship data provides real-time references for managers’ decision-making; (2) With the globalization of navigation and the development of dehumanization of ships, the increasing demand for the regulation of ship behaviors and the limited resources of transportation service contradiction is obvious, and the frequent occurrence of maritime accidents has become a problem. The research in this paper can be used as a supplement to the monitoring system for abnormal ship behaviors (Liu and Shi, 2020), such as illegal fishing (Arias and Pressey, 2016), and specific sailing patterns or stopover behaviors of illegally extracting vessels. Real-time monitoring enables timely detection and reporting of illegal activities. In summary, depth-based ship monitoring technology has a wide range of applications. It can obtain more comprehensive information on marine activities and contribute to comprehensive and effective marine decision-making. Our work can play an important role in monitoring maritime traffic, safeguarding maritime rights and interests, and improving maritime warning. We can use deep learning image ship monitoring technology to analyze water traffic in specific seas, bays, and ports and to address issues such as shipwreck rescue, illegal fishing, illegal smuggling, and illegal oil dumping by ships. Through this analysis, we can provide predictive references to support maritime management. This technology is an important part of the next-generation maritime management system.
In this paper, a novel automatic ship monitoring model based on image multi-scale feature information recognition is proposed to enhance maritime management in the context of ship category and shape diversity. Specifically, the proposed method consists of a triad generation module, a triple feature extraction module, a multiscale ternary loss function, and a multiscale similarity measure module. The triad generation module aims to extract the original dataset to construct a triad for training the deep learning model, and the triple feature extraction module extracts the feature vectors of specified dimensions for each sample, which, through the multi-scale triplet loss and the back propagation of the network, enables the model to learn an embedding function capable of mapping the samples of the same category to similar locations in the embedding space. embedding function. Then, in the testing phase, the embedding vector distance between the query samples and the support samples can be calculated. Finally, the classification is accomplished by comparing the similarity probabilities between the vectors using a multi-scale similarity metric module. Experiments on several different ship detection tasks show that AMAM-Net can be generalized to different multi-class classification and recognition tasks and achieve efficient generalization performance on different tasks because AMAM-Net uses a different training strategy from that based on traditional deep learning. In addition, experiments on the Realistic Maritime Ship Inspection Dataset demonstrate the robustness of the AMAM-Net method with significantly improved detection accuracy compared to other traditional methods. It brings significant improvement to the efficiency of nearshore maritime management in the context of the diversification of ship types and shapes.
Furthermore, in order to bring more substantial improvements to the efficiency of maritime management, the following two directions will be considered in future research: firstly, by integrating other information generation and scheduling techniques, the robustness and generalization ability of the model will be further improved by obtaining more samples to construct a more comprehensive training dataset. The second is that in real maritime management, the acquisition of ship visible image samples is not only limited by the amount of data but also by the quality of the data, such as those affected by weather conditions or due to lens distortion. Therefore, we can comprehensively analyze the methods to improve the robustness of the samples when they are perturbed to provide certain reference values for safe navigation management.
Thanks to the fact that only a small number of samples are required to train the AMAM-Net network, our AMAM-Net can be effectively generalized to a large number of categories of detection tasks and maintains high accuracy as well as robustness, which practically improves the efficiency of nearshore maritime management in the face of large-scale information on vessel traffic activities.
The original contributions presented in the study are included in the article/supplementary material. Further inquiries can be directed to the corresponding authors. Our codes are publicly available at https://github.com/jiahuasun03/Unfamiliar-Ship-Type-Recognition.
JS: Formal Analysis, Funding acquisition, Investigation, Visualization, Writing – original draft. JL: Formal Analysis, Funding acquisition, Investigation, Visualization, Writing – original draft. RL: Data curation, Funding acquisition, Resources, Writing – review & editing. LW: Writing – review & editing. LC: Conceptualization, Methodology, Software, Writing – review & editing. MS: Conceptualization, Data curation, Funding acquisition, Methodology, Resources, Software, Writing – review & editing.
The author(s) declare financial support was received for the research, authorship, and/or publication of this article. This research was funded by the National Natural Science Foundation of China (Grant No. 52171346), the Ocean Young Talent Innovation Programme of Zhanjiang City (Grant No. 2022E05002), the Young Innovative Talents Grants Programme of Guangdong Province (Grant No.2022KONCX024), the special projects of key fields of Universities in Guangdong Province (Grant No. 2023ZDZX3003), The China Institute of Navigation Young Elite Scientist Sponsorship Program by CIN (Grant No. YESSCIN2023008), the Natural Science Foundation of Guangdong Province (Grant No. 2021A1515012618), the program for scientific research start-up funds of Guangdong Ocean University, the China Transportation Education Research Association (Grant No. JTYB20-28), the Guangdong Provincial Education Teaching Reform Research Project (Grant No. 010202132201), Zhanjiang Social Science Association (Grant No. ZJ20YB0), the College Student Innovation Team of Guangdong Ocean University (Grant No. CXTD2024018), the College Student Innovation Team of Guangdong Ocean University (Grant No. CXTD2023020).
The authors declare that the research was conducted in the absence of any commercial or financial relationships that could be construed as a potential conflict of interest.
The author(s) declare that no Generative AI was used in the creation of this manuscript.
All claims expressed in this article are solely those of the authors and do not necessarily represent those of their affiliated organizations, or those of the publisher, the editors and the reviewers. Any product that may be evaluated in this article, or claim that may be made by its manufacturer, is not guaranteed or endorsed by the publisher.
Almpanidou V., Doxa A., Mazaris A. D. (2021). Combining a cumulative risk index and species distribution data to identify priority areas for marine biodiversity conservation in the black sea. Ocean. Coast. Manage. 213, 105877. doi: 10.1016/j.ocecoaman.2021.105877
Arias A., Pressey R. L. (2016). Combatting illegal, unreported, and unregulated fishing with information: A case of probable illegal fishing in the tropical eastern pacific. Front. Mar. Sci. 3. doi: 10.3389/fmars.2016.00013
Bo L., Xiaoyang X., Xingxing W., Wenting T. (2021). Ship detection and classification from optical remote sensing images: A survey. Chin. J. Aeronautics. 34, 145–163. doi: 10.1016/j.cja.2020.09.022
Chaturvedi S. K., Yang C.-S., Ouchi K., Shanmugam P. (2012). Ship recognition by integration of sar and ais. J. Navigation. 65, 323–337. doi: 10.1017/S0373463311000749
Chen J., Chen K., Chen H., Zou Z., Shi Z. (2022). A degraded reconstruction enhancementbased method for tiny ship detection in remote sensing images with a new large-scale dataset. IEEE Trans. Geosci. Remote Sens. 60, 1–14. doi: 10.1109/TGRS.2022.3180894
Chuang L. Z., Chung Y.-J., Tang S. (2015). A simple ship echo identification procedure with seasonde hf radar. IEEE Geosci. Remote Sens. Lett. 12, 2491–2495. doi: 10.1109/LGRS.8859
Cui Z., Li Q., Cao Z., Liu N. (2019). Dense attention pyramid networks for multi-scale ship detection in sar images. IEEE Trans. Geosci. Remote Sens. 57, 8983–8997. doi: 10.1109/TGRS.36
da Silva A. B. C., Joshi S. K., Baumgartner S. V., de Almeida F. Q., Krieger G. (2022). Phase correction for accurate doa angle and position estimation of ground-moving targets using multi-channel airborne radar. IEEE Geosci. Remote Sens. Lett. 19, 1–5. doi: 10.1109/LGRS.2022.3144735
Del Prete R., Graziano M. D., Renga A. (2023). Unified framework for ship detection in multifrequency sar images: A demonstration with cosmo-skymed, sentinel-1, and saocom data. Remote Sens. 15, 1582. doi: 10.3390/rs15061582
Duan H., Ma F., Miao L., Zhang C. (2022). A semi-supervised deep learning approach for vessel trajectory classification based on ais data. Ocean. Coast. Manage. 218, 106015. doi: 10.1016/j.ocecoaman.2021.106015
Eriksen T., Høye G., Narheim B., Meland B. J. (2006). Maritime traffic monitoring using a space-based ais receiver. Acta Astronautica. 58, 537–549. doi: 10.1016/j.actaastro.2005.12.016
Hadsell R., Chopra S., LeCun Y. (2006). ‘‘Dimensionality Reduction by Learning an Invariant Mapping,’’ 2006 IEEE Computer Society Conference on Computer Vision and Pattern Recognition (CVPR'06) (New York, NY, USA), 1735–1742. doi: 10.1109/CVPR.2006.100
Hong D.-B., Yang C.-S. (2013). Algorithm implementation for detection and tracking of ships using fmcw radar. J. Korean. Soc. Mar. Environ. Energy 16, 1–8. doi: 10.7846/JKOSMEE.2013.16.1.1
Hou M., Li Y., Xie M., Wang S., Wang T. (2023). Monitoring vessel deadweight tonnage for maritime transportation surveillance using high resolution satellite image. Ocean. Coast. Manage. 239, 106607. doi: 10.1016/j.ocecoaman.2023.106607
Hu Q., Hu S., Liu S. (2022). Banet: A balance attention network for anchor-free ship detection in sar images. IEEE Trans. Geosci. Remote Sens. 60, 1–12. doi: 10.1109/TGRS.2022.3146027
Huang Z., Li R. (2021). Orientated silhouette matching for single-shot ship instance segmentation. IEEE J. Selected. Topics. Appl. Earth Observations. Remote Sens. 15, 463–477. doi: 10.1109/JSTARS.2021.3132005
Jiang X., Li J., Huang Z., Huang J., Li R. (2024). Exploring the performance impact of soft constraint integration on reinforcement learning-based autonomous vessel navigation: Experimental insights. Int. J. Naval. Architecture. Ocean. Eng. 16, 100609. doi: 10.1016/j.ijnaoe.2024.100609
Li J., Sun J., Li X., Yang Y., Jiang X., Li R. (2023a). Lfld-clbased net: A curriculum-learning-based deep learning network with leap-forward-learning-decay for ship detection. J. Mar. Sci. Eng. 11, 1388. doi: 10.3390/jmse11071388
Li J., Wang H., Guan Z., Pan C. (2020). Distributed multi-objective algorithm for preventing multi-ship collisions at sea. J. Navigation. 73, 971–990. doi: 10.1017/S0373463320000053
Li J., Yang Y., Li X., Sun J., Li R. (2023b). Knowledge-transfer-based bidirectional vessel monitoring system for remote and nearshore images. J. Mar. Sci. Eng. 11, 1068. doi: 10.3390/jmse11051068
Liu D., Shi G. (2020). Ship collision risk assessment based on collision detection algorithm. IEEE Access 8, 161969–161980. doi: 10.1109/ACCESS.2020.3013957
Nie G.-H., Zhang P., Niu X., Dou Y., Xia F. (2017). “Ship detection using transfer learned single shot multi box detector,” in ITM web of conferences (EDP Sciences), vol. 12, 01006.
Park S., Cho C. J., Ku B., Lee S., Ko H. (2016). Simulation and ship detection using surface radial current observing compact hf radar. IEEE J. Oceanic. Eng. 42, 544–555. doi: 10.1109/JOE.2016.2603792
Połap D., Włodarczyk-Sielicka M., Wawrzyniak N. (2022). Automatic ship classification for a riverside monitoring system using a cascade of artificial intelligence techniques including penalties and rewards. ISA. Trans. 121, 232–239. doi: 10.1016/j.isatra.2021.04.003
Qu J., Gao Y., Lu Y., Xu W., Liu R. W. (2023). Deep learning-driven surveillance quality enhancement for maritime management promotion under low-visibility weathers. Ocean. Coast. Manage. 235, 106478. doi: 10.1016/j.ocecoaman.2023.106478
Roy K., Chaudhuri S. S., Pramanik S., Banerjee S. (2023). Deep neural network based detection and segmentation of ships for maritime surveillance. Comput. Syst. Sci. Eng. 44 647–662. doi: 10.32604/csse.2023.024997
Shao Z., Zhang X., Wei S., Shi J., Ke X., Xu X., et al. (2023). Scale in scale for sar ship instance segmentation. Remote Sens. 15, 629. doi: 10.3390/rs15030629
Shi F., Li Z., Zhang M., Li J. (2022). Analysis and simulation of the micro-doppler signature of a ship with a rotating shipborne radar at different observation angles. IEEE Geosci. Remote Sens. Lett. 19, 1–5. doi: 10.1109/LGRS.2022.3166209
Tan Z., Zhang Z., Xing T., Huang X., Gong J., Ma J. (2021). Exploit direction information for remote ship detection. Remote Sens. 13, 2155. doi: 10.3390/rs13112155
Thombre S., Zhao Z., Ramm-Schmidt H., Vallet García J. M., Malkamäki T., Nikolskiy S., et al. (2022). Sensors and ai techniques for situational awareness in autonomous ships: A review. IEEE Trans. Intelligent. Transportation. Syst. 23, 64–83. doi: 10.1109/TITS.2020.3023957
Vesecky J. F., Laws K. E., Paduan J. D. (2009). ‘‘Using HF surface wave radar and the ship Automatic Identification System (AIS) to monitor coastal vessels,’’ 2009 IEEE International Geoscience and Remote Sensing Symposium (Cape Town, South Africa), III-761–III-764. doi: 10.1109/IGARSS.2009.5417876
Wang C., Pei J., Luo S., Huo W., Huang Y., Zhang Y., et al. (2023). Sar ship target recognition via multiscale feature attention and adaptive-weighed classifier. IEEE Geosci. Remote Sens. Lett. 20, 1–5. doi: 10.1109/LGRS.2023.3259971
Yasir M., Shanwei L., Mingming X., Hui S., Hossain M. S., Colak A. T. I., et al. (2023a). Multi-scale ship target detection using sar images based on improved yolov5. Front. Mar. Sci. 9. doi: 10.3389/fmars.2022.1086140
Yasir M., Zhan L., Liu S., Wan J., Hossain M. S., Isiacik Colak A. T., et al. (2023b). Instance segmentation ship detection based on improved yolov7 using complex background sar images. Front. Mar. Sci. 10. doi: 10.3389/fmars.2023.1113669
Zeng L., Zhu Q., Lu D., Zhang T., Wang H., Yin J., et al. (2021). Dual-polarized sar ship grained classification based on cnn with hybrid channel feature loss. IEEE Geosci. Remote Sens. Lett. 19, 1–5. doi: 10.1109/LGRS.2021.3067678
Zha M., Qian W., Yang W., Xu Y. (2022). Multifeature transformation and fusion-based ship detection with small targets and complex backgrounds. IEEE Geosci. Remote Sens. Lett. 19, 1–5. doi: 10.1109/LGRS.2022.3192559
Zhang J., Jin J., Ma Y., Ren P. (2023). Lightweight object detection algorithm based on yolov5 for unmanned surface vehicles. Front. Mar. Sci. 9. doi: 10.3389/fmars.2022.1058401
Zhang S., Chen J., Wan Z., Yu M., Shu Y., Tan Z., et al. (2021). Challenges and countermeasures for international ship waste management: Imo, China, United States, and eu. Ocean. Coast. Manage. 213, 105836. doi: 10.1016/j.ocecoaman.2021.105836
Zhang T., Zhang X. (2022). Htc+ for sar ship instance segmentation. Remote Sens. 14, 2395. doi: 10.3390/rs14102395
Keywords: ship classification, deep learning, few-shot learning, maritime management, vessel monitoring
Citation: Sun J, Li J, Li R, Wu L, Cao L and Sun M (2025) Addressing unfamiliar ship type recognition in real-scenario vessel monitoring: a multi-angle metric networks framework. Front. Mar. Sci. 11:1516586. doi: 10.3389/fmars.2024.1516586
Received: 24 October 2024; Accepted: 13 December 2024;
Published: 22 January 2025.
Edited by:
Maohan Liang, National University of Singapore, SingaporeReviewed by:
Chunlei Liu, Shanghai Maritime University, ChinaCopyright © 2025 Sun, Li, Li, Wu, Cao and Sun. This is an open-access article distributed under the terms of the Creative Commons Attribution License (CC BY). The use, distribution or reproduction in other forums is permitted, provided the original author(s) and the copyright owner(s) are credited and that the original publication in this journal is cited, in accordance with accepted academic practice. No use, distribution or reproduction is permitted which does not comply with these terms.
*Correspondence: Liang Cao, Y2FvbGlhbmdAZ2RvdS5lZHUuY24=; Molin Sun, c3VubW9saW5AZ2RvdS5lZHUuY24=
†These authors have contributed equally to this work
Disclaimer: All claims expressed in this article are solely those of the authors and do not necessarily represent those of their affiliated organizations, or those of the publisher, the editors and the reviewers. Any product that may be evaluated in this article or claim that may be made by its manufacturer is not guaranteed or endorsed by the publisher.
Research integrity at Frontiers
Learn more about the work of our research integrity team to safeguard the quality of each article we publish.