- 1Business School, Shandong Management University, Jinan, China
- 2School of Management Science and Engineering, Shandong University of Finance and Economics, Jinan, China
- 3Institute of Marine Economy and Management, Shandong University of Finance and Economics, Jinan, China
- 4School of Urban Planning and Design, Peking University Shenzhen Graduate School, Shenzhen, China
- 5Key Laboratory of Chinese Academy of Sciences Spatial Information Processing and Application System Technology (Department II), Qilu Aerospace Information Research Institute, Jinan, China
Against the backdrop of global warming and rising sea levels, storm surge disasters occur frequently, often forming complex chains of events that lead to severe crises. However, systematic research on storm surge disaster chains is scarce. To characterize these chains, this research proposes a storm surge disaster chain analysis system based on complex networks and Bayesian networks. The system consists of three modules: evaluation, prediction, and measurement. The evaluation module uses a complex network model to quantitatively analyze the vulnerability, key nodes, and critical transmission paths of the disaster chain complex network. The prediction module establishes a Bayesian network-based model to forecast the complex network evolution process, forecasting the occurrence probability and loss scenarios of the disaster events. The measurement module measures and calculates the chain effect based on the dependence relationship and loss degree of the disaster event loss scenario. The results elucidate that most key nodes are primary and secondary disasters such as seawater flooding, flooding, dam damage, rainstorm, and house damage. Meanwhile, edges such as the sea wave–seawater flooding and house damage–human casualties have a critical impact on the storm surge disaster chain complex network. Key evolutionary paths such as strong winds–human casualties and over-warning tide level–social influence need to be focused on. Disaster reduction strategies such as maintaining dams, reinforcing houses, and removing disaster-bearing body can effectively break the chain and mitigate disasters. This research has a reference value for the scientific understanding of storm surge disaster chains and can serve as a scientific basis for comprehensive disaster reduction, disaster preparedness, and disaster relief.
1 Introduction
Storm surge disaster has seriously threatened the public’s personal and property safety, ranking first in the world in marine disasters. According to statistics, in September 2022, the storm surge attributed to “Ian” alone caused economic losses of more than $60 billion to the United States. The increasing number and intensity of hurricanes due to climate change and rising sea temperatures have led to more frequent and powerful storm surges (Takayabu et al., 2015). Storm surge disasters are extremely dangerous, can easily cause other disasters, and form a disaster chain to magnify the impact. This has become a hot topic of concern for countries and researchers. Therefore, identifying key events in the storm surge disaster chain complex network, calculating the chain effect for the disaster, and implementing targeted measures to reduce the disaster are important research topics with significant practical implications for protecting public safety and property and maintaining social stability.
The research on disaster chain involves areas such as rail transit (Amin et al., 2019; Chen et al., 2021; Xu et al., 2022), sudden fire (Sarwar et al., 2018), natural disasters (Hou et al., 2019; Du et al., 2022), and other aspects. The disaster chain research of natural disasters mainly focuses on geology (Li et al., 2017; Moradi et al., 2021) and flood (Liu et al., 2016, 2021; Ma et al., 2022), and there are very few studies on the storm surge disaster chain. Storm surge is a phenomenon of off-normal sea surface increase caused by tropical cyclones (typhoon or hurricane) and extratropical cyclones. It is a sudden marine disaster phenomenon occurring along the ocean coast (Fiore et al., 2009; Li and Li, 2013; Chao et al., 2020). Because of the suddenness, danger, and unpredictability of disasters, they can easily cause personnel and property loss in coastal areas, which poses a serious threat to the economic and social development of coastal cities. Consequently, the study of the storm surge disaster chain is of great significance. However, limited by many factors, such as data collection and method adaptability, few researchers have conducted a quantitative analysis of the disaster chain. Complex networks and Bayesian network (BN) methods have been applied in many fields, and are not widely applied in the natural disasters field, especially in storm surge disaster chains. Therefore, this research adopts a hybrid method of disaster chain and complex network method to analyze the interdependence between disaster events and utilizes BN methods to dynamically evolve the disaster chain and evaluate the storm surge disaster chain network. From previous studies, the chain effect of storm surge disasters has not been studied. Therefore, we reveal the chain effect characteristics of storm surge disasters and calculate the chain effect of storm surge disasters from the perspective of a complex network.
The remaining sections are organized as follows. Section 2 reviews the relevant research. In Section 3, the storm surge disaster chain analysis system has been developed. Section 4 analyzes the results from four aspects using hybrid methods. Section 5 presents the conclusions and proposes some orientations for future work.
2 Literature review
2.1 Study on storm surge disaster chain
Storm surge is one of the most severe marine disasters in coastal areas, and storm surge disaster research has attracted wide attention from researchers (Liu Y. et al., 2020; Androulidakis et al., 2023). The storm surge disaster chain refers to the disaster process in which one disaster event causes another disaster event, and different disasters interact and evolve, showing the chain sequence relationship. However, research on the storm surge disaster chain is relatively weak. The related research mainly covers the disaster mechanism from the storm surge disaster chain (Kiri et al., 2016; Pan et al., 2019), assessment of storm surge disaster chain damage (Oumeraci et al., 2018; Guo et al., 2022), and the mitigation of storm surge disaster chains (Yang et al., 2016; Pan, 2020) and other fields. Suzuki et al. (2014) quantitatively estimated the secondary disaster risk caused by storm surge by improving the storm surge inundation system. Hisamatsu et al. (2020) used storm surge empirical values and stochastic methods to evaluate the probability of strong wind and large waves caused by storm surge. Meanwhile, Wang et al. (2021) used geographic information system technology and open data to simulate the footprint of a disaster chain and quantitatively evaluate the storm surge economic loss.
2.2 Research on complex network methods
The topology of complex networks is composed of nodes and edges. The mathematical formula related to nodes and edges can even better explain the construction and characteristics of complex networks, such as the centrality of the edge (Oluwajana et al., 2013), the node degree (Li et al., 2009; Jiao et al., 2024), and the network aggregation coefficient (Artameeyanant et al., 2017). The nodes stand for some different study subjects, and the edges stand for some relationship between the nodes (Xiao and Chen, 2003; Morone and Makse, 2015). Because the complex network method can capture the network characteristics for network modeling and evaluation, it is currently applied in various studies as an effective tool (Nazempour et al., 2018; Sun et al., 2018). The disaster chain is neither entirely deterministic nor purely random, making it unsuitable for analysis using traditional networks (Arianos et al., 2009; Zheng et al., 2017). Thus, the network evaluation and analysis of the disaster chain can be completed based on the complex network construction model. Liu and Chao (2015) constructed a risk assessment method of disaster chain utilizing a complex network to simulate the evolutionary progression of a typhoon disaster chain and assess its risk. Chen et al. (2018) proposed a multi-level description framework of disaster chain (STMFDC) utilizing a complex network to analyze the evolutionary progression of a disaster chain from collapse to surface collapse. Cui et al. (2021) constructed the natural disaster chain of typhoons, rainstorms, landslides, and earthquakes, revealing the disaster characteristics and formation process of landslides, earthquakes, dam breaks, and flood discharge. Researchers have tried to use complex networks to conduct natural disaster chain network research, but there are still few studies based on complex networks. Therefore, this study combines the disaster chain theory with the complex network method to model the storm surge disaster chain network and evaluate it.
2.3 Chain effect study
Studies on the evolution of disaster chains and risk level are usually implemented using BNs (Wang et al., 2013; Jager et al., 2017). Hosseini and Ivanov (2019) considered the chain reaction for the first time, using the BN to examine and test the supply chain elasticity index, and calculated the resilience of restoring the broken chain. Huang et al. (2023) applied BNs to deduce disaster chain scenarios and proposed appropriate response strategies. Chain effects can also reflect the nature of the network and the risk level, and measure the evolution results in the network (Dalton et al., 2020; Liu C. et al., 2020). Chain effect studies have mainly focused on disasters (Wei and Chen, 2010; Choi et al., 2020; Hffken et al., 2020), emergencies (Xiao et al., 2017; Wen et al., 2020), the supply chain (Ge et al., 2021; Sawik and Lev, 2022), and other fields. Wu and Li (2013) adopted the root theory to clarify the chain effect of tourism after suffering from natural disasters. Yan et al. (2022) took into account geographical conditions of mining subsidence areas, the cascading impact of geological hazards, and other factors to establish assessment framework for mining geological hazards. Chen et al. (2023) proposed a multifactorial urban agglomeration risk assessment model by integrating socioeconomic foundations, oligopolistic effects, and cascading effects of disasters.
In conclusion, previous storm surge disaster studies show that there are still some challenging issues for improvement:
(1) First of all, compared with other natural disasters such as earthquakes, floods, and other disaster chains, there are fewer studies on the storm surge disaster chain, but storm surge is the most severe marine disaster, which needs more attention.
(2) Second, the disaster chain effect is a crucial component in evaluating the storm surge disaster chain network, but there are few studies on it, and breakthroughs need to be made. At present, studies on the storm surge disaster chain and chain effect mainly focus on qualitative research, and in-depth quantitative research is needed.
(3) Finally, previous studies are mostly based on the qualitative evaluation of static networks, which do not involve the prediction of disaster chain evolution, triggering the dynamic process of probability and node scenario judgment, which needs to be explored.
The main purpose of this study is to propose a network analysis approach to model and evaluate complex networks of storm surge disaster chains. The main contributions of this study can be summarized as follows:
(1) Develop a storm surge disaster chain analysis system and build, evaluate, and predict the storm surge disaster chain complex network, so as to provide suggestions for reducing the impact of storm surge disasters.
(2) Based on the complex network model, the storm surge disaster chain complex network is evaluated from the perspective of individuals, interactions, and networks, and the effectiveness of the assessment results is verified.
(3) The model based on BNs for predicting the evolutionary process of the storm surge disaster chain in a complex network dynamically illustrates the dynamic evolution process of a disaster chain, achieving the prediction of disaster event triggering rate and node scenarios.
(4) Measure and calculate the chain effect of storm surge disasters, evaluate the effectiveness of disaster reduction strategies, and provide a basis for scientific chain breaking and disaster reduction and reducing the impact of the chain effect.
3 The storm surge disaster chain analysis system
The established storm surge disaster chain complex network offers an effective vehicle to identify key disaster events and their interactions. Through the analysis of the disaster chain effect, it can assist reasonable decision-making, reduce the harm caused by disasters, and optimize resource allocation. According to the transmission and internal structure of disaster events in the disaster chain, the analysis system of the storm surge disaster chain is developed. The system includes an evaluation module, a prediction module, and a measurement module.
3.1 Evaluation module
The evaluation module using disaster events and disaster loss results as nodes, with the evolution of disaster events as edge, builds a storm surge disaster chain complex network. From the perspective of individual analysis, interaction analysis, and network analysis, this module assesses the status of nodes, edges, and the overall network, while analyzing the vulnerability, key nodes, and critical transmission pathways of the disaster chain. Currently, there is no ordinary operational framework to explain the internal architecture of disaster events within the storm surge disaster chain.
According to the type, sequence, and impact time limit of the disaster events, the source events that lead to the disaster chain are called the original disaster events and the subsequent evolved disaster events are secondary and derivative disaster events. The output variable of the disaster chain, representing the disaster damage result, is referred to as disaster loss. During the formation of a disaster chain, an event or the damage result of its disaster-bearing body may be the disaster-causing factor of another disaster; that is, the output result of one disaster may be the input factor of another disaster. Therefore, the storm surge disaster chain is regarded as a collection of event factors composed of primary disaster events, secondary disaster events, derivative disaster events, and disaster losses. There is an example of a storm surge disaster chain based on a summary of historical disasters. Rainstorm is a typical storm surge disaster, which will cause flood and debris flow secondary disasters in a specific environment. The three disasters have huge disaster energy, which can damage roads, houses, land disaster bearing bodies and cause serious economic losses. Of course, secondary disaster events and derivative disaster events can be caused by different original disaster events; thus, the same disaster events in storm surge disasters will appear in multiple storm surge disaster chains, but the disaster chain is relatively independent. In storm surge disasters, different disaster chains interlace, showing each other the network characteristics, both systematic and complex, and forming a disaster chain complex network.
3.1.1 Individual analysis
This section realizes the comprehensive importance evaluation of network disaster events. In the storm surge disaster chain complex network, the importance of disaster events can be calculated, and obviously some disaster events are crucial. Disaster events with high comprehensive importance may result in more severe consequences, and more concentration should be focused on disaster prevention and control. The four characteristic parameters, namely, degree centrality of disaster event, betweenness centrality of disaster event, proximity centrality of disaster event, and PageRank value, are selected as indicators to measure the comprehensive importance of disaster events, which are shown in Table 1.
Degree centrality can intuitively quantify the significance and impact on disaster events and losses in the disaster chain complex network. The betweenness centrality can reflect the connection ability and control effect of disaster events and disaster losses as the intermediate connection points on the network. Proximity centrality represents the difficulty and dependency for one node reaching other nodes. The PageRank value measures the significance of nodes and their neighborhood disaster events and disaster loss, indicating that the significance of the node in the network can also measure the significance of adjacent nodes.
To determine the importance of network nodes, it is necessary to measure the correlation between the factors influencing the significance of disaster chain nodes, and measure the intrinsic relationship between the four characteristics of degree centrality, betweenness centrality, proximity centrality, and PageRank value and the significance of disaster events and losses. Therefore, this section proposes an improved relative gray correlation analysis to comprehensively evaluate the comprehensive importance of complex network nodes. The steps for the proposed improved relative gray correlation analysis method are as follows.
Construct the association factor sequence with the reference sequence. Degree centrality, betweenness centrality, proximity centrality, and PageRank value were considered as association factor variables .
The maximum value of each corresponding index for n disaster events and disaster losses is counted as the optimal reference sequence, and the minimum value is counted as the worst reference sequence.
Dedimensionalized treatment,
, where . is the dedimensionalized data, and is the average value of the j indicator of the node.
Calculate the gray correlation coefficient. The association coefficients and values were calculated for the best reference sequence and worst reference sequence .
where the resolution coefficient β is between 0 and 1. , , , .
The traditional calculation formula of the gray correlation degree of the optimal reference sequence is , and the average weight ignores the characteristic relationship of the index and is easy to cause the local association trend. Thus, this section introduces information entropy for improvement.
is the relative entropy of the jth index, whose residue degree is , the higher the residual degree, and the higher the importance.
is the weight of the jth index. The optimal reference sequence gray correlation degree for the entropy weight correction is
Similarly, the worst reference sequence of the entropy weight correction is
Then,
is the node synthesis importance, and is the relative correlation degree.
3.1.2 Interaction analysis
This section calculates the vulnerability of the interaction edge between storm surge disaster events and disaster loss, analyzes the evolution characteristics of disasters, and identifies the potential disasters and consequences. The vulnerability of the edge is an indicator to measure the importance of the edge. The vulnerability of the edge in the disaster chain complex network is an inherent property of a certain network, which refers to the degree of influence on the network if the certain edge in the network is deleted. The vulnerability index of the complex network edge in the storm surge disaster chain is shown in Table 2. The greater the vulnerability of network edges, the greater the degree of association with other nodes, and the higher the importance.
The average path length reflects the difficulty of the evolution in a disaster chain network. By removing edge s or more edges, the importance of the connecting edges formed between disaster events and disaster losses in the disaster chain network can be analyzed. The edge betweenness represents the number of shortest paths passing through the edge, and reflects the influence range of connected edges formed between nodes in the disaster chain network. The connectivity refers to the proportion of other nodes connected in the disaster chain complex network to total nodes and reflects the stability in the disaster chain complex network. The vulnerability of the edges in the disaster chain complex network can give expression to the interaction of the nodes and the importance of the connecting edges between the nodes to the network stability and evolution process. The equation is constructed to obtain the degree of edge s contribution to the network vulnerability, where , and are the positive indices and , are the negative indices.
3.1.3 Network analysis
The interweaving of disaster chain forms the disaster chain complex network, which is essential to the overall analysis of the complex network. The purpose is to weaken the internal connection of the disaster chain complex network and cut off the spread and development in the disaster chain. This section evaluates and analyzes network characteristics through the degree correlation, clustering coefficient, and network efficiency of the disaster chain complex network as a measure of the effectiveness of chain breakage in disaster prevention analysis. The degree correlation of complex networks reflects the relationship between moderately large and small nodes in the disaster chain. The range of the degree correlation coefficient is . When , the disaster chain complex network was negatively correlated, indicating that some nodes with higher degrees in the disaster chain complex network are more likely to be connected to nodes with lower degrees. The clustering coefficient reflects the possibility of their neighboring nodes being connected to each other and the degree of aggregation of complex network connections in the disaster chain. The network efficiency reflects the degree of closeness and connectivity in the complex networks. Specific indicators are detailed in Table 3.
3.2 Predictive module
This module is applied with BNs to mine the complex network evolution information of the disaster chain hidden in storm surge disaster data. By learning the cause-and-effect relationship among disaster chain nodes, the initiation probability and disaster loss scenario of secondary and derived disaster events in the network are predicted. Based on the causal semantic inference in the posterior probability problem of the BN, the prediction model of the complex network evolutionary process for the storm surge disaster chain is constructed. According to the evaluation model, the complex network construction and node parameters of the disaster chain are known. Based on the occurrence evidence of a disaster event known in the storm surge disaster database, the reliability of the entire network is updated to obtain the occurrence probability of other disaster events. Among them, the complexity of the inference algorithm is closely related to the topological structure and complexity of the network model. There are multiple algorithms to realize the inference calculation for the network model, but each algorithm is built on the Bayesian formula to achieve the node prediction and inference update, as shown in Equation 19:
In Equation 19, P(C) indicates the probability of C occurrence without evidence support, which is the prior probability; P(C/D) indicates the known query variable, that is, the evidence variable is D, the probability of C occurrence is the posterior probability, and the prediction inference result in the prediction model is the posterior probability. P (D/C) represents the conditional probability distribution for the initial given node, which is the likelihood function. In the disaster chain complex network, it refers to the occurrence probability for the latter disaster event node when the previous disaster event node occurs/does not occur.
3.3 Measurement module
By calculating the chain effect of the disaster chain, this module helps the public and decision-makers understand the evolution pathways and chain effects, and provides scientific basis for the work of chain breaking disaster reduction. The definition of effects varies slightly in different disciplinary fields. Here, storm surge disasters are likened to toxins. In toxicology experiments, effects refer to the biological changes in an individual, organ, or tissue caused by a certain dose of exogenous chemicals in contact with the body. Therefore, in this research, the chain effect for storm surge disasters refers to the degree of change in disaster risk after the primary disaster event of storm surge reaches a certain intensity and triggers secondary or derivative disaster events, thereby generating secondary or derivative disaster factors and forming a hierarchical chain effect.
Therefore, through the definition of chain effect, the chain effect of a storm surge disaster is affected by the possibility of other events triggered by disaster events, the severity of consequences caused by disaster events or disaster losses, the difficulty of disaster chain damage, and the degree of interaction and influence between disaster events. The more important the disaster event is, the greater the risk, loss, and harm it may cause. The greater the contribution of the complex network edge to the vulnerability of the disaster chain, the easier it is for the disaster chain to evolve, and the smaller the trigger probability of the disaster event, the shorter the length of the disaster chain. Therefore, the calculation formula for the chain effect of a storm surge disaster is defined as:
represents the chain effect of disaster event i or disaster loss j caused by disaster events; indicates the comprehensive importance of disaster event or disaster loss j on the disaster chain; is the triggering rate between disaster event i and j; is the loss degree of disaster event or disaster loss j; is the vulnerability of interaction edge between disaster event i and j. In a storm surge disaster chain complex network, a certain disaster chain occurs as disaster event a, which eventually leads to disaster loss n. The chain effect of this disaster chain is defined as:
where H is the chain effect for the whole chain; Hmn represents that chain effect between disaster event m and n in the disaster chain where disaster event a occurs and disaster loss n ends. When disaster event a occurs , .
According to Equation 21, the storm surge disaster chain effect can be calculated, and the comprehensive significance of disaster events and the vulnerability of the edge in the disaster chain are obtained by the evaluation module. To what extent a disaster event will trigger secondary and derivative disasters, the triggering rate between disaster events needs to be measured, and this probability is obtained by the prediction module.
4 Results analysis
The main purpose of the analysis system is to present the evolution process in the disaster chain by analyzing the topological characteristics of complex networks. It is possible to identify key nodes, clarify the interaction between disaster events, and calculate the chain effect, in order to achieve precise breakpoints and disaster reduction, and minimize the risks and hazards generated by storm surge disaster chains.
4.1 Data collection
To establish a storm surge disaster chain complex network with high authenticity, conducting a lot of research on the disaster-causing events and disaster chain caused by the storm surge on the basis of real disasters and reliable data is necessary. In general, there are historical disaster data, engineering reports, and research literature as the three main sources of data (Papathoma-Koehle et al., 2015; Chen and Cui, 2017), which are applied to seize the interaction relationships between diverse disaster-causing events in the storm surge disaster chain complex networks. In this study, data on 220 storm surge disasters in coastal China from 1989 to 2022 were collected. These data will be applied to support the identification of disaster events, disaster-causing factors, and disaster losses, and to establish the relationship among disaster events and disaster losses. Through the historical data and the experience of building disaster chains in previous literature, the learning database for the storm surge disaster chain complex network structure is obtained. The experimental environment is macOS 10.14.6, Intel(R) Core CPU (Core-i5 2.6GHz), 8G RAM. For the methods of this paper, different tools are used. A complex network was implemented in Gephi software, BNs were implemented in GeNie2.2 software, and all the other models were run using the MATLAB R2016b software.
4.2 Network establishment
According to the above storm surge disaster data and the transmission process for the disaster chain, 33 high-frequency and representative disaster events and disaster loss results were identified. They serve as the node elements in the storm surge disaster chain complex network structure, as shown in Table 4.
Considering the identified disaster chain nodes and the interactive influence matrix with disaster events, a storm surge disaster chain complex network can be preliminarily established, in which the nodes are connected by edges. The relationship between the evolution and interaction of the two nodes is recorded as 1, and the 0–1 matrix is used to abstract the storm surge disaster chain complex network. The preliminary determination of the complex network structure of the disaster chain is based on objective data, and then tested by subjective data by interviewing experts in the field. Redundant relationships are eliminated and missing links are supplemented. The relationship matrix between primary disaster events and secondary, derived disaster events is shown in Table 5.
The connecting edges between nodes represent the impact relationship between disaster events, and a complex network evolution model for the disaster chain is constructed. G and K represent the set of nodes and edges, where , corresponds to 5 primary disaster events, corresponds to 16 secondary disaster events, corresponds to 7 derivative, disaster events, and corresponds to 5 disaster loss results. The influence relationship between complex network nodes can be constructed into an adjacency matrix, where represents whether the primary disaster event affects the primary disaster event , and represents whether the ith primary disaster event affects the jth secondary disaster event. The influence value is 1; otherwise, it is 0, from which we can obtain the 0–1 matrix of the influence relationship between complex network nodes. According to the adjacency matrix, a complex network evolution model of the storm surge disaster chain is drawn as shown in Figure 1, which has 33 nodes and 214 directed edges.
By conducting structural analysis on the network, the key nodes and evolution patterns of the disaster chain can be clarified. For instance, in Figure 1, the primary disaster event “strong wind (Node A1)” will lead to secondary and derivative disaster events such as “crop damage (Node B9)” and “traffic tie-up (Node C2)”.
4.3 Results analysis
This study presents the results of individual analysis, interaction analysis, network analysis, and chain effect analysis in the constructed storm surge disaster chain complex network. Some findings can be obtained based on the study results.
4.3.1 Individual analysis
4.3.1.1 The node feature analysis
Gephi and Matlab software were used to construct a complex network model and calculate the metrics. The power function fitting of the node degree distribution in the complex network is shown in Figure 2. The fitting equation R2 is 0.8271, and the power law exponent of this equation is −0.706. It can be construed that the node degree for the disaster chain complex network follows the power law distribution, which conforms to the characteristics of the scale-free network. The scale-free network nodes have a high aggregation degree and “small clusters” characteristics. Removing such nodes has a small effect on the scale-free network structure. In case the hub nodes are damaged, the network will be seriously damaged. Therefore, individual analysis should be conducted to identify key disaster events.
4.3.1.2 The node importance analysis
The degree centrality, betweenness centrality, proximity centrality, and PageRank value in the storm surge disaster chain complex network are obtained from Equations 1, 2, 3, 4, respectively. The specific indicators are shown in Table 6. The comprehensive importance is obtained from Equation 11, and the comprehensive importance ranking is shown in Figure 3.
In the complex network, the top 10 in terms of comprehensive importance are seawater flooding, flood, eagre, rainstorm, overtopping dam, sea wave, dam damage, strong wind, house damage, and floodplain. Among these disaster events, seven nodes are secondary disasters, and three nodes are primary disasters, indicating the significant importance of secondary disasters in the storm surge disaster chain complex network. The impact of natural disasters is beyond doubt, but primary disasters are almost inevitable, which are the impact of storm surges themselves. Secondary disaster events such as house damage, dam damage, and seawater flooding are also the hub of the disaster chain complex network. If they are attacked, the impact on the network structure is enormous. Derivative disasters are directly affected not only by primary disasters such as strong winds and sea waves, but also by secondary disasters caused by primary disasters. The comprehensive importance on top include life disorder, traffic tie-up, freshwater pollution, and enterprise shutdown. The difference in importance between various derivative disaster events is not significant. However, due to their own characteristics, the derivative disaster events are in the backward position in the comprehensive importance ranking. Because they are at the end of the disaster chain and serve as the output of disaster events, disaster loss nodes have obvious duality and relatively poor centrality, with the lowest comprehensive importance degree. The comprehensive importance gap of primary, secondary, and derivative disaster events is obvious, but the internal gap is not significant, among which the comprehensive importance of environmental damage nodes is the lowest.
4.3.2 Interaction analysis
The typical scale-free networks in complex networks allow the networks to follow a power law distribution, where the nodes can have different degrees to illustrate their importance level. The interaction between nodes reflects the network edge characteristics and analyzes the interaction effects between disaster events and disaster loss to intuitively study the evolution of the storm surge disaster chain. Meanwhile, the vulnerability and robustness of network connection edges have been measured, providing a scientific basis for blockchain disaster reduction. Using the Matlab software program, the average path length, connectivity, and edge betweenness were calculated in combination with Equations 12, 13, 14, 15 of the disaster chain complex network. Thus, the vulnerability of each edge in the disaster chain complex network is calculated. The results that are sorted by vulnerability are shown in Table 7.
From the table, either the edges with high vulnerability are the source of disaster losses in the disaster chain, or the nodes with high comprehensive importance in the disaster chain complex network are connected. If these edges are artificially destroyed, the entire disaster chain complex network can be greatly disrupted, reducing the occurrence conditions of other nodes in the network. Among them, the vulnerability of sea wave–seawater flooding, house damage–human casualties, dam damage–seawater flooding, overtopping dam–seawater flooding, strong winds–sea waves, freshwater pollution–diseases, over-warning tide level–seawater flooding, seawater flooding–freshwater pollution ranks high, with fragility levels of 77.73, 77.19, 75.63, 73.96, 73.83, 59.09, 57.98, and 57.07, respectively. These are key edges in the network and are prone to forming a long chain of disasters, causing greater disaster losses. Simultaneously removing these edges will have a major impact for the network, leading to a decrease in network connectivity. It can be seen that seawater flooding is a node with high comprehensive importance and a key node affecting network vulnerability. However, seawater flooding–eagre, eagre–flood, flood–debris flow, flood–landslides, flood–aquaculture damage, debris flow–power and communication facilities damage, life disorder–people affected, disasters–human casualties, and enterprise shutdowns–economic losses are relatively weak edges. These edges have obvious two-end characteristics, which are either the edges of the derivative disaster aggregation in the early stage of the disaster, or the edges connecting the comparative terminal nodes, with weak correlation to other nodes and slightly lower importance. Thus, identifying key edges and nodes can achieve damage to the critical chain, reduce the impact of disasters, and provide greater protection for people’s lives and property safety.
4.3.3 Network analysis
The above content is presented from individual analysis and interaction analysis to analyze the micro situation of disaster chain. This section analyzes the overall situation of disaster chain complex network from the perspective of aggregation sub-network, network clustering, and network connectivity.
4.3.3.1 Network feature analysis
The Pearson correlation coefficient λ of the node degree of the two sides in the disaster chain complex network can describe the degree correlation of the disaster chain complex network. By analyzing the degree correlation of the storm surge disaster chain complex network, the coefficient of network degree correlation is λ = 0.0736 and the network has a positive correlation. This positive correlation proves that the nodes with a large node degree in the disaster chain network are more inclined to connect to other factors with a large node degree. That is, when the disaster event with a strong interaction ability occurs, the disaster event caused by it also has a strong interaction ability. Similar to the characteristics of scale-free networks and power distributions, there are hub nodes in complex disaster chain networks that are prone to forming small groups. Meanwhile, the complex network of storm surge disasters causes disasters quickly, with significant amplification effects and disaster results. While blocking disaster events with strong interactive capabilities, attention should also be paid to disaster events in other disaster chains caused by them. However, the decentralization of disaster chain paths poses difficulties for disaster prevention and mitigation.
4.3.3.2 Cluster-based sub-network analysis
The Louvain algorithm is used to divide the disaster events and disaster loss aggregation sub-network, and the disaster chain node cluster group, and its main principle is to evaluate the module degree of aggregation sub-network closeness. The sub-network has closer internal relationships compared to external relationships, resulting in more pronounced interactions between disaster events and disaster losses. Storm surge disaster chain disaster events and disaster losses are divided into three groups, namely, cluster sub-network 1: A1, A2, A3, A4, B12, B14, B15, D2, D4; cluster sub-network 2: A5, B1, B2, B3, B10, B13, B16, C2, C3, C4, C5, C6, C7, D3, D5; and cluster sub-network 3: B4, B5, B6, B7, B8, B9, B11, C1, D1. Disaster events were more interactive within the group. Cluster sub-network 1 indicates that primary disasters such as strong wind, surge, and sea wave are important factors causing casualties and economic losses. Cluster sub-network 2 shows that rainstorm, flood, debris flow, and other disasters are the main impact factors of land facilities such as production equipment, and road and bridge infrastructure damage, and are also the leading factors of derivative disaster events, which can easily cause environmental damage and have a social impact. In cluster sub-network 3, nodes such as seawater flooding, dam damage, crop damage, and house damage are the dominant factors causing disasters to people. There is an obvious phenomenon in the cluster sub-network, and the agglomeration of disaster events with a large node degree can prove the positive correlation of the disaster chain complex network. Meanwhile, the core factors in the disaster event cluster sub-network can become the key points of the blocking and control of the disaster chain. Therefore, controlling core factors can reduce the aggregation degree and chain effect.
4.3.3.3 Network clustering analysis
The clustering coefficient of the complex network in the disaster chain expresses the degree of aggregation of disaster events. The high clustering coefficient of a disaster event proves that it is closely related to other adjacent disaster events, and its adjacent nodes in the network are easily connected and clustered into groups. The average clustering coefficient represents the degree of interconnectedness within the disaster chain complex network. After calculation, the average clustering coefficient of the complex network is 0.3386 > 0.1000, demonstrating that the disaster events in the storm surge disaster chain complex network have high aggregation degree and strong interaction, and have the characteristics of “small groups”. The clustering coefficient of disaster events is shown in Figure 4. Among them, the clustering coefficient of derivative disasters is higher, indicating that the agglomeration of derivative disasters “within the group” is better. The clustering coefficient of disaster loss nodes is the smallest, indicating low interaction between them.
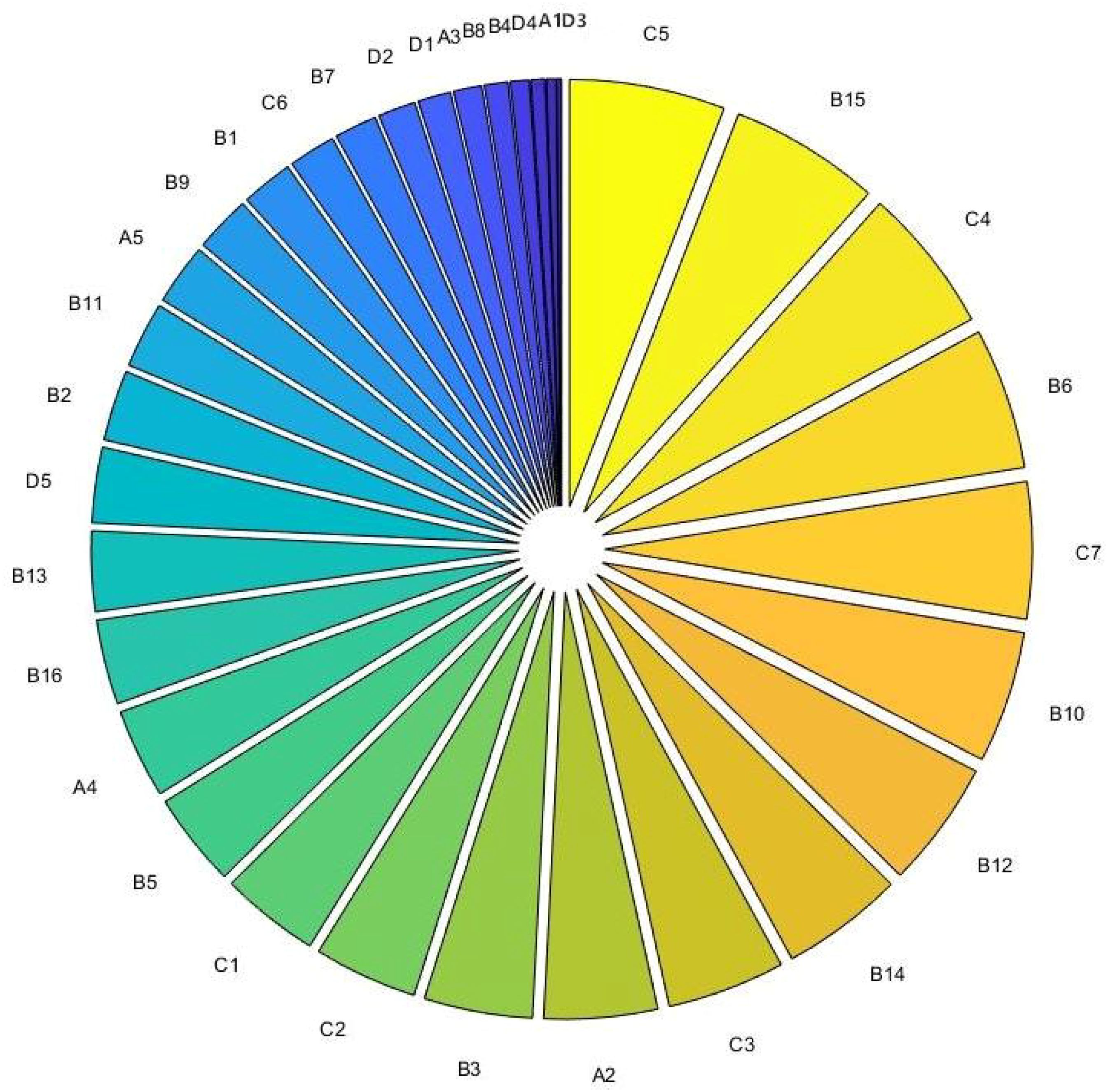
Figure 4. Clustering coefficient of disaster events in the storm surge disaster chain complex network.
From Figure 4, it can be seen that the clustering coefficients for ship damage, freshwater pollution, eagre, life disorder, landslide, etc. are all higher than the average clustering coefficient. This indicates that the closer the connection between these disaster events and adjacent events, the easier it is for them to form groups. It is worth noting that the top-ranked disaster events are mainly secondary disasters and derivative disasters. Diseases, fresh water pollution, traffic tie-up, enterprise shutdown, and life disorder are easy-to-generate derivative disaster-causing factors. In the disaster chain, it has the characteristics of many nodes and far greater than the degree, which is the main direct derivative disaster factors with obvious results in the disaster chain. The eagre and surge are the events that directly cause disasters in the sea and land, and are closely related to other secondary disasters and derivative disasters. Ship damage, the maritime platform damage, and aquaculture damage are directly caused by primary disasters. The adjacent nodes are prone to produce secondary disaster factors. The disaster location generally has obvious regional characteristics at sea and are closely interconnected to the original disaster events. There is no disaster event with a clustering coefficient of 0 in the complex network of the entire disaster chain, indicating that the disaster event in the network has a strong interaction and a close connection, and confirming the characteristics of “small groups”. However, the clustering coefficient of these primary disasters with high comprehensive importance, such as over-warning tide level and strong wind, is small. Because it is a primary disaster event that can easily cause losses, it cannot be easily affected by other disaster events, and the interaction between the adjacent nodes is relatively small. Secondary disaster events such as overtopping dam, seawater flooding, and crop damage mainly exist in the long disaster chains, resulting in a lowaggregation coeffificient. However, there is a clear dispersion of disaster loss nodes such as environmental damage and economic losses, which are dispersed in different clusters. There is less interaction between adjacent nodes in the chain, which corresponds to the grouping of cluster sub-networks.
4.3.3.4 Network connectivity analysis
The connectivity of a complex network in a disaster chain refers to the ability of all connected nodes or edges to form a new network after removing one or more disaster chain nodes and interactive edges, which can maintain its original circulation efficiency. The stronger the network connectivity, the more robust and less susceptible the network is to damage, making it difficult to achieve effective disaster prevention and mitigation. According to the calculation, the network efficiency of the complex network of the original storm surge disaster chain is 0.4611, with good connectivity and resistance to damage (Zhou and Wang, 2018). If the complex network nodes and edges of the disaster chain are disrupted, the connectivity of the network will deteriorate, which is the main method to evaluate the effect of the disaster reduction strategy.
4.3.4 Chain effect analysis
4.3.4.1 Evolutionary process prediction
(According to the described method and combined with the specific data of storm surge, the chain effect of storm surge disaster is further analyzed. The comprehensive importance and vulnerability of the edge of the disaster chain node have been known. This section uses the system to measure the triggering rate of disaster events. The probability is between 0 and 1, and the closer the value is to 1, the more likely the trigger between storm surge disasters is. Conversely, the smaller is the probability. The relationship among the nodes in the disaster chain network can be described by the triggering rate between the disaster events, which enables the quantitative analysis of the network. First, the value domain and the scenario of the disaster chain nodes are determined. The scenario division of complex network nodes in disaster chains often adopts binary discretization processing, that is, two scenarios of disaster event occurrence and non-occurrence. Combined with the data of disaster news reports, relevant literature, statistical yearbook, emergency disaster database, and disaster field standards, the value domain of each node in the disaster chain is shown in Table 8.
The model sample database was established, and the occurrence evidence of each node variable in the database was analyzed. The value domain was divided based on the scenario of the complex network nodes, and then the evolution probability parameters of each node in the disaster chain complex network were received. Based on this, the node relationships are constructed according to the disaster chain complex network, and parameter learning is carried out based on the storm surge database. The prediction model for the evolutionary process of the storm surge disaster chain complex network was constructed by using the GeNie2.2 software as shown in Figure 5.
4.3.4.2 Triggering rate prediction
The BN is used to show the occurrence probability of different scenario nodes. In order to determine the disaster event evolution process of the disaster chain, the triggering rate between the disaster chain nodes is analyzed, and the scenario probability parameter of a certain parent node is changed to obtain the scenario probability of the child node, so as to determine the triggering rate of a certain parent node. The probability of rainstorm scenario 2 is changed to 1, that is, , is changed to , . When the rainstorm exceeds 250 mm/d, the scenario probability of each node in the disaster chain complex network is calculated and the triggering rate of its sub-nodes in the same disaster chain can be determined, so as to realize the measurement of chain effect. When the rainstorm is set to more than 250 mm/d, the probability of eagre, flood, and crop damage caused by the rainstorm increases, by 93%, 85%, and 87%, respectively. The evolution of the disaster chain has resulted in a growth for the degree of disaster losses such as the people affected, environmental damage, and economic losses. In the process of disaster chain evolution, the occurrence of disaster events such as house damage, flood, debris flow, landslides, and maritime platform damage disasters will result in disaster losses such as people affected, human casualties, and environmental damage losses. Among them, the probability of causing extremely serious affected to people reaches 74%, and the probability of causing more serious social influence is 55%. The presentation is shown in Figure 6. Similarly, by improving the scenario probability of nodes, the triggering rate between disaster links can be obtained.
4.3.4.3 Chain effect analysis
According to the described method formula and the specific data of storm surge, the chain effect of storm surge disaster is further analyzed. According to the chain effect formula, the comprehensive importance of disasters in the storm surge disaster chain complex network, the triggering rate of disaster events, and the vulnerability of disaster events have been known. The degree of loss L is assigned according to the scenario of loss. When the primary disaster event scenario is 1, the loss degree is 1, and when the scenario is 2, the loss degree is 2. When the secondary and derivative disaster event scenario is 1, the loss degree is 0, and when the scenario is 2, the loss degree is 2. When the disaster loss result scenario is 1, the loss degree is 1. When the disaster loss result scenario is 2, the loss degree is 2. When the disaster loss result scenario is 3, the loss degree is 3. The chain effect of storm surge disasters calculated by Matlab is shown in Table 9.
By analyzing the probability parameters and interaction of node scenarios in BNs, and measuring the chain effect of the chain, five key disaster chain paths related to the disaster loss results of storm surge disaster chain are obtained, which are the strong winds–human casualties evolution path, the strong winds–people affected evolution path, the over-warning tide level–social influence evolution path, the strong winds–environmental damage evolution path, and the rainstorm–economic losses evolution path.
Path 1, the strong winds–human casualties evolution path: strong winds → sea waves → dam damage → seawater flooding → house damage → human casualties. The disaster chain has the highest chain effect, which includes four key edges, among which the comprehensive importance of primary disaster and secondary disaster nodes ranks in the top eight, and the comprehensive importance of seawater flooding nodes is the highest. This disaster chain gathers three spatial elements and has a very high disaster energy. Marine defense engineering should be strengthened to reduce the incidence rate of seawater flooding. The stability of buildings should be strengthened to minimize casualties.
Path 2, the strong winds–people affected evolution path: strong winds → sea waves → seawater flooding → crop damage → people affected. This disaster chain is not the longest chain, but it has a high incidence of inter-disaster disasters and high node importance, including three key edges. The disaster chain contains an important disaster-bearing body, namely, crops. Crops cannot be moved, and most of the affected areas and the affected people belong to the rural areas. Once affected, the economic impact will be significant. For this path, crops that meet the harvest standards can be harvested in advance and effectively covered. After the disaster, timely drainage and planting should be carried out to prevent pests and diseases.
Path 3, over-warning tide level–social influence evolution path: over-warning tide level → dam damage → seawater flooding → house damage → social panic → social influence. The chain effect of this disaster chain is relatively high. It has three key edges and three secondary disaster event nodes with high comprehensive importance, which is a typical long chain with fewer primary disasters and derivative disasters and multiple disasters. Pay attention to this path in addition to the measures mentioned earlier to prevent seawater flooding. Regularly inspect the towering buildings to eliminate hidden dangers. Before the disaster, focus on strengthening the building structure to increase the stability of the building. Seal the house in time to prevent rainwater leakage.
Path 4, strong winds–environmental damage evolution path: strong winds → sea waves → dam damage → floodplain → land salinization → environmental damage. This disaster chain is a typical long chain, where strong winds and waves are coupled to enhance the triggering rate of disaster events, the chain effect in the disaster chain, and the degree of disaster losses. This disaster chain includes the main disaster-bearing bodies along the coast, dams, mudflat, coastal vegetation, and land. Paying attention to this path can guide people to reinforce and raise dams through disaster prevention and mitigation measures, reduce their vulnerability, and prevent dam damage and flooding. Timely carry out land drainage and irrigation to accelerate soil desalination. Timely carry out chemical improvements to reduce losses.
Path 5, rainstorm–economic losses evolution path: rainstorm → flood → debris flow → house damage → economic loss. In the disaster chain about economic losses, the losses caused are more easily reflected, the chain is relatively short, and the measures are more targeted, but there are fewer nodes that can be implemented. The chain effect is more prominent in the evolution path of rainstorm economic losses. Divide flood and debris flow disaster-sensitive areas. Pay attention to highly sensitive areas, remove the main disaster-bearing materials in the area, strengthen ecological restoration and regional vegetation coverage, and improve water retention capacity and environmental stability. Improve the disaster prediction accuracy to reduce risks. Increase investment in water conservancy facilities and enhance drainage capacity.
4.3.4.4 Assessment of disaster reduction strategies
The nodes and their related edges that affect the chain effect and critical path are obtained by the measurement module, and they are intentionally deleted in complex networks to block the occurrence of key disaster events and critical chains, and then evaluate the effectiveness of eliminating them in reducing disasters. Thus, targeted disaster reduction strategies can be formulated. This section evaluates the effectiveness of targeted chain breaking measures by examining the changes in clustering coefficients and network efficiency before and after intentional network destruction.
Given the assessment of the disaster chain by the disaster chain analysis system, three risk reduction strategies have been developed to mitigate the spread and impact of the disaster chain. Strategy 1 focuses on reducing the impact of seawater flooding caused by dam damage and overtopping dam due to primary disasters, that is, removing nodes B5 and B7. After removing relevant nodes, the disaster chain of sea waves → dam damage → seawater flooding, overtopping dam → dam damage → seawater flooding will disappear, and the network density can be reduced by 13.18% to 0.4003. The suggestion is to enhance the prediction ability of storm surge path, strengthen the marine defense engineering in highly sensitive areas, and reduce the incidence rate of seawater flooding. Strategy 2 focuses on mitigating the impact of emergency relief due to damage to road and bridge infrastructure and power communication facilities, that is, node B13 and B16 are removed, After removing the relevant nodes, the disaster chain of rainstorm → road and bridge infrastructure damage→ traffic tie-up, strong wind → power and communication facilities damage → enterprise shutdown will disappear, reducing the network density to 0.4021, a decrease of 12.80%. The suggestion is to plan road construction reasonably, strengthen the construction of emergency traffic channel, and strengthen the emergency power supply and communication equipment and management of key units. The third is to reduce the impact of human casualties and economic losses by protecting against personnel-related disasters, that is, removing nodes B11, B14, and B15. After the removal of relevant nodes, the disaster chain of rainstorm → house damage → economic loss, strong wind → the maritime platform damage → human casualties, sea waves → ship damage will disappear, which can reduce the network density to 0.3853, with a decrease of 16.44%. It is suggested to strengthen the disaster structure before the disaster; increase the stability of houses, factories, and marine platforms; and prepare for the ships and personnel in advance. Comparing the three strategies, it is found that strategy 3 is the primary solution to improve the impact of the storm surge disaster chain complex network. Disaster-bearing bodies play an important role in the disaster chain complex network, and strengthening the protection of disaster bearing has a huge impact on reducing disasters. If there are sufficient manpower and material resources, strengthened ocean defense engineering, sufficient emergency power communication equipment, timely protection of disaster prone areas in areas where storm surge disasters are about to occur, and the above three strategies are implemented, the network density will be reduced to 0.2705, a significant decrease of 41.33% compared to the original network. Therefore, the adoption of targeted disaster prevention and mitigation strategies in disaster-sensitive areas can greatly reduce the complexity of the storm surge disaster complex network, thereby reducing the impact of disasters.
Important nodes, critical edges, and chain effect indicators can guide disaster prevention and reduction from different aspects. Before the occurrence of storm surge disasters, it can be investigated and prevented according to the key factors and events calculated by the system. After the occurrence of disasters, the path of further evolution of the disaster can be cut off based on the key edges with larger indicators. Cutting off key disaster events and their corresponding disaster chains, disrupting the conditions for the formation of complex networks in the disaster chain, and providing a basis for refined disaster prevention and reduction thereby reduce the risks and losses caused by storm surges.
5 Conclusions and future work
In order to realize the scientific identification, accurate simulation, and effective calculation and evaluation of the storm surge disaster chain, and provide reference for the formulation of disaster prevention and mitigation policies in the future, this study proposes the analysis system for the storm surge disaster chain. The system consists of three modules: evaluation, prediction, and measurement. The evaluation module abstracts the disaster chain complex network and evaluates the vulnerability of key disaster events and key transmission chain and disaster chain network. The prediction module constructs a complex network evolution process prediction model for the disaster chain, and deduces the probability of storm surge disaster scenario evolution. The main function of the measurement module is to measure the chain effect of storm surge disasters, identify the key evolution path, put forward targeted measures of storm surge chain reduction, quantitatively discuss the chain breaking strategy and disaster reduction effect, and ameliorate the early warning, resistance, and disaster reduction ability of storm surge disasters. The results for the research are summarized as follows: (1) the storm surge disaster chain complex network has the characteristics of the scale-free network. Through the comprehensive analysis of different indicators, it is found that the hub disasters are concentrated in secondary disasters such as seawater flooding, flooding, dam damage, and house damage, as well as primary disasters such as rainstorm and sea waves. Meanwhile, the key chains such as waves–seawater flooding, house damage–human casualties, and seawater flooding–freshwater pollution play a crucial role in the disaster chain complex network. (2) The storm surge disaster chain complex network forms three key sub-networks. The average clustering coefficient for the complex network in the original disaster chain is 0.3386, and the network efficiency is 0.4611, which analyzes the vulnerability and connectivity of the storm surge disaster chain complex network effectively. (3) The prediction module is used to deduce the probability of complex network evolution scenarios and the triggering rate of disaster events, and predict the disaster loss scenarios. (4) Calculate the chain effect of storm surge disasters, identify the five key paths which are highly related to the disaster loss results and formulate and evaluate the adaptive strategies of storm surge disasters. In conclusion, this research should offer scientific guidance for disaster management decision-makers and coastal residents, improve the level of regional disaster prevention and mitigation, and guarantee the social economy of coastal areas. In the future, this system can be applied to other disaster chains and emergency event chains. Meanwhile, the advantages of disaster big data can be utilized to strengthen the comprehensive research of the land and sea disaster chain. Simultaneously, multi-objective optimization is utilized to determine the future production layout, resource allocation, and coastal safety management measures in disaster-prone areas.
Data availability statement
The raw data supporting the conclusions of this article will be made available by the authors, without undue reservation.
Author contributions
HG: Writing – review & editing, Visualization, Software, Writing – original draft, Methodology, Formal analysis, Data curation, Conceptualization. CH: Writing – review & editing, Supervision, Software, Project administration, Methodology, Funding acquisition, Data curation. CZ: Writing – review & editing, Visualization, Validation, Software, Methodology. QS: Writing – review & editing, Writing – original draft, Visualization, Validation, Software, Methodology, Formal analysis, Data curation.
Funding
The author(s) declare that financial support was received for the research, authorship, and/or publication of this article. This work was supported by the National Social Science Found General Projects of China (grant no. 23BGL031), the Natural Science Foundation Youth Program of Shandong, China (grant no. ZR2023QG040), and the China Postdoctoral Science Foundation General project (grant no. 2023M742051).
Acknowledgments
The authors appreciate the valuable suggestions and objective comments made by the reviewers.
Conflict of interest
The authors declare that the research was conducted in the absence of any commercial or financial relationships that could be construed as a potential conflict of interest.
Generative AI statement
The author(s) declare that no Generative AI was used in the creation of this manuscript.
Publisher’s note
All claims expressed in this article are solely those of the authors and do not necessarily represent those of their affiliated organizations, or those of the publisher, the editors and the reviewers. Any product that may be evaluated in this article, or claim that may be made by its manufacturer, is not guaranteed or endorsed by the publisher.
References
Amin S., Tamima U., Amador-Jiménez L. E. (2019). Optimal pavement management: Resilient roads in support of emergency response of cyclone affected coastal areas. Transport. Res. Part A: Policy Pract. 119, 45–61. doi: 10.1016/j.tra.2018.11.001
Androulidakis Y., Makris C., Mallios Z., Pytharoulis I., Baltikas V., Krestenitis Y., et al. (2023). Storm surges and coastal inundation during extreme events in the Mediterranean Sea: the IANOS Medicane. Natural Hazards. 117, 939–978. doi: 10.1007/s11069-023-05890-6
Arianos S., Bompard E., Carbone A., Xue F. (2009). Power grid vulnerability: a complex network approach. Chaos 19, 1–16. doi: 10.1063/1.3077229
Artameeyanant P., Sultornsanee S., Chamnongthai K. (2017). Electroencephalograp-hy-based feature extraction using complex network for automated epileptic seizure detection. Expert Syst. 34, 70–83. doi: 10.1111/exsy.12211
Chao W. T., Young C. C., Hsu T. W., Liu W. C., Liu C. Y. (2020). Long-lead-time prediction of storm surge using artificial neural networks and effective typhoon parameters: revisit and deeper insight. Water 12, 1–8. doi: 10.3390/w12092394
Chen X., Cui Y. (2017). The formation of the Wulipo landslide and the resulting debris flow in Dujiangyan City, China. J. Mount. Sci. 14, 1100–1112. doi: 10.1007/s11629-017-4392-1
Chen H., Zhang L., Ran L. (2021). Vulnerability modeling and assessment in urban transit systems considering disaster chains: a weighted complex network approach. Int. J. Disaster Risk Reduct. 54, 102033. doi: 10.1016/j.ijdrr.2020.102033
Chen Y., Zhang J., Zhou A., Yin B. (2018). A modeling method for a disaster chain–taking the coal mining subsidence chain as an example. Hum. Ecol. Risk Assess. 24, 1–21. doi: 10.1080/10807039.2017.1412820
Chen C., Zhao D., He F., Sun F. (2023). A comprehensive multi-hazard risk assessment model for an urban agglomeration with multiple factors. J. Saf. Sci. Resil. 4, 43–51. doi: 10.1016/j.jnlssr.2022.09.008
Choi J. H., Lee Y., Kwak Y. H. (2020). A socioeconomic ripple effect analysis of integrative national construction standards codification efforts: system dynamics approach. IEEE Trans. Eng. Manage. 69, 2959–2975. doi: 10.1109/TEM.2020.3025326
Cui Y. L., Hu J. H., Xu C., Zheng J., Wei J. B. (2021). A catastrophic natural disaster chain of typhoon-rainstorm-landslide-barrier lake-flooding in Zhejiang province. China. J. Mt Sci. 18, 2108–2119. doi: 10.1007/s11629-020-6513-5
Dalton K., Skrobe M., Bell H., Kantner B., Berndtson D., Gerhardinger L. C., et al. (2020). Marine-related learning networks: shifting the paradigm toward collaborative ocean governance. Front. Mar. Sci. 7. doi: 10.3389/FMARS.2020.595054
Du W., Sheng Q., Fu X., Chen J., Zhou Y. (2022). A TPDP-MPM-based approach to understanding the evolution mechanism of landslide-induced disaster chain. J. Rock Mech. Geotech. Eng. 14, 1200–1209. doi: 10.1016/j.jrmge.2022.03.004
Fiore M., D’Onofrio E. E., Pousa J. L., Schnack E. J., Bértola G. R. (2009). Storm surges and coastal impacts at Mar del Plata, Argentina. Continent. Shelf Res. 29, 1643–1649. doi: 10.1016/j.csr.2009.05.004
Ge F., Beullens P., Hudson D. A. (2021). Optimal economic ship speeds, the chain effect, and future profit potential. Transport. Res. Part B Methodol. 147, 168–196. doi: 10.1016/j.trb.2021.03.008
Guo H., Yin K., Huang C. (2022). Modeling of direct economic losses of storm surge disasters based on a novel hybrid forecasting system. Front. Mar. Sci. 8. doi: 10.3389/fmars.2021.804541
Hffken J., Vafeidis A. T., Macpherson L. R., Dangendorf S. (2020). Effects of the temporal variability of storm surges on coastal flooding. Front. Mar. Sci. 7. doi: 10.3389/fmars.2020.00098
Hisamatsu R., Tabeta S., Kim S., Mizuno K. (2020). Storm surge risk assessment for the insurance system: a case study in Tokyo bay, Japan. Ocean Coast. Manage. 189, 1–13. doi: 10.1016/j.ocecoaman.2020.105147
Hosseini S., Ivanov D. (2019). A new resilience measure for supply networks with the ripple effect considerations: a bayesian network approach. Ann. Operat. Res. 319, 581–607. doi: 10.1007/s10479-019-03350-8
Hou W., Chen Z. Q., Zuo D. D., Feng G. L. (2019). Drought loss assessment model for southwest China based on a hyperbolic tangent function. Int. J. Disaster Risk Reduct. 33, 477–484. doi: 10.1016/j.ijdrr.2018.01.017
Huang L., Chen T., Deng Q., Zhou Y. (2023). Reasoning disaster chains with bayesian network estimated under expert prior knowledge. Int. J. Disaster Risk Sci. 6, 1011–1028. doi: 10.1007/s13753-023-00530-w
Jager W. S., Christie E. K., Hanea A. M., Den Heijer C., Spencer T. (2017). A bayesian network approach for coastal risk analysis and decision making. Coast. Eng. 134, 48–61. doi: 10.1016/j.coastaleng.2017.05.004
Jiao L., Luo Q., Lu H., Huo X., Zhang Y., Wu Y. (2024). Research on the urban rail transit disaster chain: Critical nodes, edge vulnerability and breaking strategy. Int. J. Disaster Risk Reduct. 102, 1–15. doi: 10.1016/j.ijdrr.2024.104258
Kiri H., Kubota T., Tanji H., Nakay T. (2016). Characteristics of storm surge disaster due to typhoon 9918 in farmlands in the yatsushiro sea coastal area. J. Agric. Meteorol. 60, 359–364. doi: 10.2480/agrmet.359
Li K., Li G. S. (2013). Risk assessment on storm surges in the coastal area of Guangd-ong Province. Natural Hazards 68, 1129–1139. doi: 10.1007/s11069-013-0682-2
Li H. C., Wu T., Wei H. P., Shih H. J., Chao Y. C. (2017). Basinwide disaster loss assessments under extreme climate scenarios: a case study of the Kaoping River basin. Natural hazards. 86, 1039–1058. doi: 10.1007/s11069-016-2729-7
Li W., Zhao W., Mou X. (2009). “A technique for network modeling and reliability evaluation of complex radial distribution systems,” in 2009 IEEE Power and Energy Society General Meeting, (Canada: IEEE Press), Vol. 10, 1–5. doi: 10.1109/PES.2009.5275389
Liu A. H., Chao W. U. (2015). Research on risk assessment method of disaster chain based on complex network. Sys. Eng. Theory Pract. 35, 466–472. doi: 10.12011/1000-6788(2015)2-466
Liu Y., Lu C., Yang X., Wang Z., Liu B. (2020). Fine-scale coastal storm surge disaster vulnerability and risk assessment model: A case study of Laizhou bay, China. Remote Sens. 12, 1301. doi: 10.3390/rs12081301
Liu Y., Tang W., Zhang W., Zhang X., Niu S. (2021). Overview of flood disaster risk analysis based on disaster chain. Water Resour. Protect. 37, 20–27. doi: 10.3880/j.issn.1004-6933.2021.01.004
Liu C., Wang Z., Ma C., Wang X. (2020). Influencing factors of the chain effect of spherical gas cloud explosion. Process Saf. Environ. Protect. 142, 359–369. doi: 10.1016/j.psep.2020.06.007
Liu X. J., Zhong D. H., Tong D. W., Zhou Z. Y., Ao X. F., Li W. Q. (2016). Dynamic visualisation of storm surge flood routing based on three-dimensional numerical simulation. J. Flood Risk Manage. 11, 729–749. doi: 10.1111/jfr3.12252
Ma C., Qi W., Xu H., Zhao K. (2022). An integrated quantitative framework to assess the impacts of disaster-inducing factors on causing urban flood. Nat. Hazards 113, 1903–1924. doi: 10.1007/s11069-022-05375-y
Moradi S., Heinze T., Budler J., Gunatilake T., Kemna A., Huisman J. A. (2021). Combining site characterization, monitoring and hydromechanical modeling for assessing slope stability. Land 10, 1–23. doi: 10.3390/land10040423
Morone F., Makse H. A. (2015). Influence maximization in complex networks through optimal percolation. Nature 524, 65–68. doi: 10.1038/nature14604
Nazempour R., Monfared M. A. S., Zio. E. (2018). A complex network theory approach for optimizing contamination warning sensor location in water distribution networks. Int. J. Disaster Risk Reduct. 30, 225–234. doi: 10.1016/j.ijdrr.2018.04.029
Oluwajana S. D., Aderinlewo O. O., Owolabi A. O., Croope S. V. (2013). Assessment of centrality properties of akure road network. Int. J. Traffic Transp. Eng. 3, 82–94. doi: 10.1016/j.ijdrr.2018.04.029
Oumeraci H., Kortenhaus A., Burzel A., Naulin M., Dassanayake D. R., Jensen J., et al. (2018). Xtremrisk — integrated flood risk analysis for extreme storm surges at open coasts and in estuaries: methodology, key results and lessons learned. Coast. Eng. J. 53, 1–23. doi: 10.1142/s057856341540001x
Pan A. (2020). Study on the decision-making behavior of evacuation for coastal residents under typhoon storm surge disaster. Int. J. Disaster Risk Reduct. 45, 101522. doi: 10.1016/j.ijdrr.2020.101522
Pan J., Wang S., Sun T., Chen M., Wang D. (2019). Experimental study on inner slope failure mechanism of seawall by coupling effect of storm surge and wave. J. Mar. Limnol. 37, 1912–1920. doi: 10.1007/s00343-019-8236-4
Papathoma-Koehle M., Zischg A., Fuchs S., Glade T., Keiler M. (2015). Loss estimation for landslides in mountain areas - An integrated toolbox for vulnerability assessment and damage documentation. Environ. Model. Software 63, 156–169. doi: 10.1016/j.envsoft.2014.10.003
Sarwar B., Bajwa I., Ramzan S., Kausar M. (2018). Design and application of fuzzy logic based fire monitoring and warning systems for smart buildings. Symmetry 10, 615. doi: 10.3390/sym10110615
Sawik T., Lev B. (2022). Stochastic optimization of supply chain resilience under ripple effect: a covid-19 pandemic related study. Omega 109, 102596. doi: 10.1016/j.omega.2022.102596
Sun L., Huang Y., Chen Y., Yao L. (2018). Vulnerability assessment of urban rail transit based on multi-static weighted method in Beijing, China. Transport. research Part A. Policy pract. 108, 12–24. doi: 10.1016/j.tra.2017.12.008
Suzuki T., Shibaki H., Suzuyama K. (2014). Prediciton of inundation disaster due to storm surge under global warming. Agric. Sys. 1, 1–8. doi: 10.9753/icce.v34.management.32
Takayabu I., Hibino K., Sasaki H., Shiogama H., Mori N., Shibutani Y., et al. (2015). Climate change effects on the worst-case storm surge: a case study of typhoon Haiyan. Environ. Res. Lett. 10, 1–10. doi: 10.1088/1748-9326/10/6/064011
Wang J., Gu X., Huang T. (2013). Using bayesian networks in analyzing powerful earthquake disaster chains. Natural Hazards. 68, 509–527. doi: 10.1007/s11069-013-0631-0
Wang S., Mu L., Qi M., Yu Z., Zhao E. (2021). Quantitative risk assessment of storm surge using gis techniques and open data: a case study of Daya bay zone, China. J. Environ. Manage. 289, 112514. doi: 10.1016/j.jenvman.2021.112514
Wei L., Chen H. (2010). “The ripple effect of natural disaster on the agricultural industry chain,” in Fourth International Conference on Management of E-commerce and E-government, Vol. 2010, 85–89 (China: IEEE). doi: 10.1109/ICMeCG.2010.25
Wen F., Shuhan M. A., Hanxue Y. E., Yue Q. I., Zuo B., Psychology, S. O (2020). psychological typhoon eye effect” and “ripple effect”: double perspective test of risk perception and anxiety characteristics of people in different covid-19 severity regions. Acta Psychol. Sinica. 52, 1087–1104. doi: 10.3724/SP.J.1041.2020.01087
Wu J., Li W. (2013). The ripple effect from destroyed attractions to undestroyed attractionsafter natural disasters: example of attractions in Sichuan province after the Wenchuan great earthquake. Tour. Tribune. 117, 489–507. doi: 10.1021/jp404248z
Xiao F. W., Chen G. (2003). Complex networks: small-world, scale-free and beyond. IEEE Circuits Syst. Mag. 3, 6–20. doi: 10.1109/MCAS.2003.1228503
Xiao Z., Jin G., Zheng L., Li S. (2017). “Research on fault risk analyzing method of satellite power system based on ripple effect,” in 2017 2nd International Conference on System Reliability and Safety (ICSRS), (Italy: IEEE Press), 91–98. doi: 10.1109/ICSRS.2017.8272802
Xu Z. D., Xu W., Sun B., Liu X., Xu D. (2022). Analysis on the disaster chain evolution from gas leak to explosion in urban utility tunnels. Eng. failure anal. 140, 106609–106624. doi: 10.1016/j.engfailanal.2022.106609
Yan B., Liu J., Qi Q., Liu W., Li X. (2022). Study on chain relationship and risk assessment model of coal mine geological disasters. Arab. J. Geosci. 15, 1–12. doi: 10.1007/s12517-022-10184-5
Yang S., Liu X., Liu Q. (2016). A storm surge projection and disaster risk assessment model for China coastal areas. Natural Hazards. 84, 649–667. doi: 10.1007/s11069-0162447-1
Zheng L., Wang F., Zheng X. (2017). Complex network construction method to extract the nature disaster chain based on data mining//IEEE International Conference on Electronics Information and Emergency Communication. IEEE 7, 602–605. doi: 10.1109/ICEIEC.2017.8076504
Keywords: storm surge, disaster chain, complex network, chain effect, chain-breaking and disaster mitigation
Citation: Guo H, Huang C, Zhang C and Shao Q (2024) A novel comprehensive system for analyzing and evaluating storm surge disaster chains based on complex networks. Front. Mar. Sci. 11:1510791. doi: 10.3389/fmars.2024.1510791
Received: 13 October 2024; Accepted: 14 November 2024;
Published: 19 December 2024.
Edited by:
Xuemei Li, Ocean University of China, ChinaReviewed by:
Bai Jing, Yanshan University, ChinaJin Cancan, Nanjing University of Finance and Economics, China
Shuangxi Miao, China Agricultural University, China
Copyright © 2024 Guo, Huang, Zhang and Shao. This is an open-access article distributed under the terms of the Creative Commons Attribution License (CC BY). The use, distribution or reproduction in other forums is permitted, provided the original author(s) and the copyright owner(s) are credited and that the original publication in this journal is cited, in accordance with accepted academic practice. No use, distribution or reproduction is permitted which does not comply with these terms.
*Correspondence: Qinglong Shao, c2hhb3FsQGFpcmNhcy5hYy5jbg==