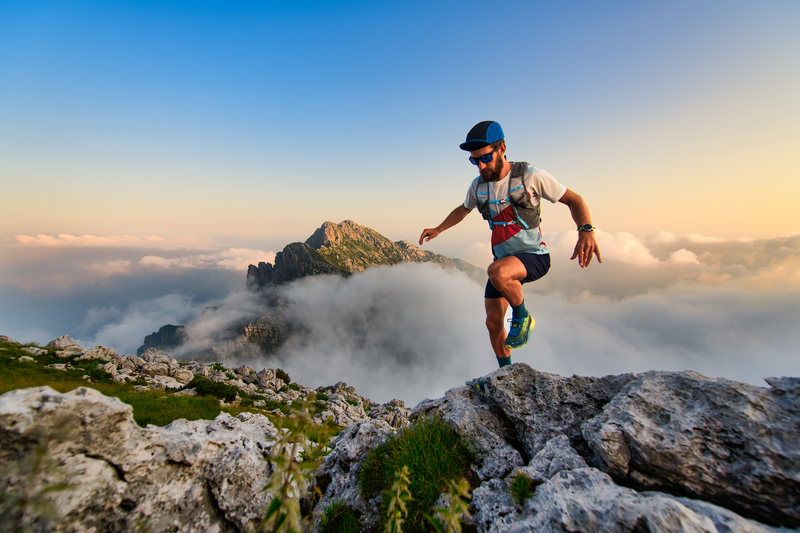
95% of researchers rate our articles as excellent or good
Learn more about the work of our research integrity team to safeguard the quality of each article we publish.
Find out more
ORIGINAL RESEARCH article
Front. Mar. Sci. , 06 December 2024
Sec. Ocean Observation
Volume 11 - 2024 | https://doi.org/10.3389/fmars.2024.1503177
This article is part of the Research Topic Advances in Remote Sensing Technologies for Ocean Ecology and Carbon Cycle View all 5 articles
Transparency () can most intuitively reflect changes in marine ecosystems, therefore; the data of is crucial to protect marine ecosystems. However, there is still a relative lack of long-term sequence data on for coastal turbid waters. Satellite remote sensing inversion provides an efficient means of obtaining long-term, large-scale . The method proposed by Lee et al. is currently one of the most widely used methods, which is divided into clear water and turbid water models based on the 670 nm remote sensing reflectance (). In this study, we employed an improved model building upon the aforementioned method. The improved model simulates the continuous transition from clear to turbid water, which allows for automatic adjustment of model weights based on a logistic curve. Utilizing this improved model, this study inverts within 100 km of the eastern coast of China from 2003 to 2023 using MODIS Aqua Level 2 data. shows an increasing trend with the distance from the coast, with high in the northern Yellow Sea, the southern Shandong Peninsula, and the far shore of the East China Sea, and the low in the coast of Bohai Sea and northern Jiangsu. As for the long-term changes, the number of pixels with significantly increased and those with significantly decreased and no significant changes accounted for 20.84%, 1.14%, and 78.02%, respectively. The order of seasonal is summer > autumn > spring > winter, and the seasonal variability amplitude increases synchronously with the offshore distance of seawater on the whole. Interestingly, the correlation between and the annual runoff of rivers exhibits spatial differentiation among six typical estuaries: there are positive correlations in northern, whereas negative correlations in the south. Additionally, the in five of six estuaries have negative correlations with annual sediment transport. Overall, this study not only provides more accurate and continuous data of for nearshore turbid waters compared to those obtained by the original model, but also offers valuable insights on the spatiotemporal variation in the of large-scale seawater.
Global climate change and human activities have increasingly threatened the security of marine ecosystems, particularly in the coastal regions (Cael et al., 2023; Dai et al., 2023). The Bohai Sea (BS), Yellow Sea (YS), and East China Sea (ECS), situated along the edge of the northwest Pacific Ocean, face intensified environmental pressures due to pollutant and sediment inputs from major rivers in China (Shi and Wang, 2012). Although the stricter environmental protection regulations have been executed in recent years, uncertainties remain regarding the response and long-term ecological trends of marine ecosystems (Wang et al., 2023b). Fortunately, transparency, as a critical indicator of water quality, effectively indicates changes in marine environments. Thus, research on nearshore water transparency is crucial for evaluating marine ecosystem health and developing effective marine protection regulations (Basset et al., 2001; Melo et al., 2009). At present, transparency can be measured by the Secchi disk depth (). This method requires consumption of massive time and resources although it can obtain accurate results, which makes it difficult for us to obtain long-term and large-scale data (Lee et al., 2016; Qing et al., 2021). In contrast, remote sensing technology has the advantages of wide monitoring area and fast data collection, which can facilitate convenient services for long-term and large-scale transparency data (Guo et al., 2022; Molner et al., 2023; Guo et al., 2023b). Over the past four decades, satellite remote sensing has been demonstrated to be an effective means of monitoring global and regional water environments (Feng et al., 2019; Jiang et al., 2019). Also, remote sensing has been successfully applied to the prediction of water (Feng et al., 2019; Guo et al., 2022).
In general, the commonly used methods for retrieving in remote sensing data include empirical algorithms and semi-analytical algorithms (Jiang et al., 2019). The former estimates using a simple regression analysis between remote sensing data and in-situ measurements of . This approach offers several advantages, including simplicity, convenience, and high accuracy. However, it is susceptible to limitations due to regional and temporal constraints. Differently, the latter is based on underwater visibility theory for inversion (Msusa et al., 2022), which is a more commonly used method (Zhao et al., 2022). However, most of these algorithms have been designed for the ocean and do not consider the local characteristics of the ocean (He et al., 2004; Lee et al., 2015; Mao et al., 2018). For example, the semi-analytic algorithm (QAA) developed by Lee et al., and it is currently one of the most commonly used mechanism models (Lee et al., 2015). This model can derive the total absorption coefficient (, m-1) and the total backscattering coefficient (, m-1) from the remote sensing reflectance (, sr-1). Based on this, the minimum diffusion attenuation coefficient (, m-1) within 400 - 700 nm can be calculated to ultimately determine . Following the naming convention proposed by Xiang et al., we refer to the algorithm developed by Lee et al. in 2015 as “Lee15” in this study (Xiang et al., 2023). The Lee15 divided the model into clear water () and turbid water () models based on the value of . This method is well applied in clear water, but may generate inaccurate results when applied to highly turbid water (Lee et al., 2015; Yin et al., 2021). In fact, the ocean is usually divided into Class I water bodies (ocean) and Class II water bodies (heavily influenced by humans) (Gordon and Morel, 1983). This results in a limited practical application scope of previous methods, especially for coastal turbid waters (Mao et al., 2018; Jiang et al., 2019; Zhao et al., 2022). The in complex coastal waters is more unstable compared to oceanic water which greatly increases the difficulty of monitoring.
To accurately invert the for complex water, various inversion methods based on water body classification have been proposed in previous studies. For instance, Liu et al. and Xiang et al. classified water into clear to moderately turbid water and highly turbid water, and clear, moderately turbid, and highly turbid water based on remote sensing reflectance band ratios, respectively, and used corresponding algorithms to estimate (Liu et al., 2022; Xiang et al., 2023); Jia et al. established an inversion method for water with different nutrient states based on the fuzzy logic optical water type scheme (Jia et al., 2022). These methods essentially rely on strict thresholds partitioning, although they perform well in obtaining of turbid water. In fact, the boundary between clarity and turbidity in complex water is not very clear, and threshold division may result in discontinuity of estimation results near the threshold (Yu et al., 2019). For this reason, we improved the semi-analytical model (Lee15) by leveraging and to simulate the continuous changes in water , thereby avoiding the constraints of fixed thresholds and ensuring data continuity (Lee et al., 2015; Chen et al., 2022). Given the complexity and continuous variability of nearshore water, we have chosen the improved model for inversion in order to obtain more accurate information.
Moreover, current studies primarily focus on the inversion of characteristics in inland lakes, open oceans, and coastal bays (Shi and Wang, 2012; Mao et al., 2018; Zhou et al., 2021). Studies specifically addressing turbid coastal waters with varying optical properties are relatively scarce, particularly concerning long-term series analysis (Shang et al., 2016; Guo et al., 2022, 2023a). To advance our understanding of long-term changes in complex nearshore waters, we generate daily data with spatial resolution of 1 km by inverting the within 100 km of coastal waters in eastern China from 2003 to 2023 using an improved algorithm based on modified secondary water bodies. The principal objectives of this research are as follows: (1) to reveal the long-term spatiotemporal distribution pattern of within 100 km of coastal waters in eastern China; (2) to elucidate the spatiotemporal distribution characteristics and key influencing factors of in typical estuaries in eastern China. These results not only provide high-resolution data for the analysis of long-term changes in coastal waters of China, but also offer a novel perspective for a deeper understanding of the spatiotemporal variation in seawater .
The study area extends 100 km inland from the eastern coast waters of China, encompassing the BS, the YS, and the northern area of the ECS (Figure 1). It spans latitudes 25.3° to 41.0°N and longitudes 117.6° to 125.4°E, covering a total area of approximately 376,937 km² with an average depth of 31.55 m. The coastal waters serve as recipients for numerous rivers originating from the mainland, which consistently transport sediment and other nutrients to the ocean (Dai et al., 2018). Among these rivers, the Yangtze River stands as China’s largest river, annually conveying approximately 5.0× 108 million tons of sediment to the ECS. Additionally, the Yellow River ranks as China’s second largest river, transporting around 1.1×109 tons of sediment to the BS each year (Milliman and Meade, 1983). Other major rivers, including the Liaohe, Qiantang, Haihe, and Minjiang rivers, also contribute substantial quantities of sediment to these three seas (Shi and Wang, 2012). The impact of these sediments on nearshore is of significant consequence. A substantial quantity of sediment from the Liaohe and Yellow Rivers has been transported and deposited in the BS, resulting in the formation of two delta wetlands: the Liao River Estuary and the Yellow River Estuary. The identification of sediments from the ancient Yellow River as a contributing factor in the formation of the Subei Shoal, situated in the vicinity of Jiangsu Province, China, has been made in the YS (Shi and Wang, 2012). Concurrently, a considerable quantity of sediment from the Yangtze River and the Qiantang River, in conjunction with the river’s interaction with the sea, has resulted in the formation of a substantial tidal delta and a trumpet-shaped Hangzhou Bay on the west coast waters of the ECS (Hori et al., 2002). As the largest mountain river on the southeast coast, the Minjiang River contributes a significant amount of sediment to the region, forming a medium-sized high-tide estuarine system and a delta with distinct sedimentary zones (Wu et al., 2023).
Figure 1. Study area. ELHR, the Estuary of Liaohe River; EHHR, the Estuary of Haihe River; EYR, the Estuary of Yellow River; DZBC, The Dingzi Bay and Coast; HZBC, The Haizhou Bay and Coast; EYTR, the Estuary of Yangtze River; EQHB, the Estuary of Qiantang River and Hangzhou Bay; EMJR, the Estuary of Minjiang River.
The data at 412 nm, 443 nm, 488 nm, 531 nm, 547 nm, 555 nm, and 667 nm, taken at a spatial resolution of 1 km × 1 km with daily temporal resolution, were derived from the MODIS-Aqua level 2 data of the NASA Ocean Biology Processing Group (OBPG, https://oceancolor.gsfc.nasa.gov/), covering the period from 2003 to 2023. To enhance data quality, we performed rigorous quality control on all MODIS data, including using quality assurance (QA) bands to remove interference from factors such as clouds and aerosol particles, and removing negative values. The bathymetric data were derived from ETOPO 2022 (https://www.ncei.noaa.gov/products/etopo-global-relief-model), a global bathymetric topographic elevation dataset released by the National Environmental Information Center of the United States in 2022.
The dataset of in situ was obtained from the National Field Scientific Observation and Research Station of the Jiaozhou Bay Marine Ecosystem in Shandong Province (http://jzb.cern.ac.cn/), the National Earth System Science Data Center (https://www.geodata.cn/main/), and relevant references (Zheng et al., 2014; Fang et al., 2021; Zhan et al., 2021; Zhang et al., 2022). The dataset includes 225 sets of matched data from 84 sampling stations, spanning from 2005 to 2020, with locations ranging from 120.79°E to 123.80°E and 26.39°N to 36.77°N (Figure 1). The data on annual runoff and sediment transport volume for the Liao River, Haihe River, Yellow River, Yangtze River, Qiantang River, and Minjiang River were obtained from the River Sediment Bulletin of China (2003-2023, China Water Power Press) (http://www.mwr.gov.cn/sj/tjgb/zghlnsgb/). The data presented here were obtained from representative hydrographic stations located in close proximity to the estuaries and are detailed in Supplementary Table S1.
Lee et al. developed a quasi-analytical algorithm for retrieving the inherent optical properties of water (Zhan et al., 2020). They subsequently refined the algorithm multiple times for different types of water, resulting in the successive proposals of QAA-v4, QAA-v5, and QAA-v6 (Zhan et al., 2020). Proposed in 2015, QAA-v6 is a semi-analytic algorithm developed based on a new underwater visibility theory for the and of water from visible spectra (Lee et al., 2015). Then, the ) was estimated from and with Lee’s model (Lee et al., 2015; Xiang et al., 2023). A total of seven bands in the visible spectrum of the MODIS sensor were selected for the estimation of in coastal waters in this study. These include the following wavelengths: 412 nm, 443 nm, 488 nm, 531 nm, 547 nm, 555 nm, and 667 nm. Of these, 555 nm was chosen as the reference band. The estimate of can be expressed as (Lee et al., 2015):
Where is the diffuse attenuation coefficient; is the absorption coefficient of the total; is the backscattering coefficient of total and is the backscattering coefficient of pure seawater; is the solar zenith angle.
Then, the was estimated from and using the following formula (Lee et al., 2015):
where represents the minimum diffuse attenuation coefficient of the 412 nm, 443 nm, 488 nm, 531 nm, 547 nm, 555 nm, and 667 nm bands in coastal waters. denotes the remote sensing reflectance in the same band as the minimum .
In accordance with the value of the water , QAA-v6 subdivides the water into two models for calculation, which are the clear water model () and the turbid water model () (Lee et al., 2015). Most coastal waters in the BS, YS, and ECS are classified as Class II water bodies. may underestimate the of some clear water, while may overestimate the of some turbid water (Feng et al., 2019). Consequently, for turbidity and fast-changing Class II water bodies, the threshold of can be adjusted in order to circumvent the problem of underestimation in clear water and overestimation in turbidity water (Qiu et al., 2023). To estimate the of coastal waters with greater accuracy, our improved model based on Lee15 was performed (Chen et al., 2022). The improved model postulates that the is uniform between the turbid and clear states. According to the characteristics of this process, we fitted the model with a binary logistic regression model (Chen et al., 2022). When is extremely low, the ratio of is 0; In turn, when is extremely high, the ratio of is 1. Moreover, the coefficient of is used to construct a logistic function. It can be expressed as:
where and are the proportion and the estimation of , k and are the steepness and the x value of the midpoint of the curve, and the sum of the proportion of and should be one.
Subsequently, we refined the parameters by fitting the curve using the least squares method based on the of 106 matched field measurements and spectral estimates. After that, by calculating the curvature of the curve to find the position of the threshold, and use Formula 3 and Lee15 to derive and . The coefficients of the two models used in this study are from our previous research (Chen et al., 2022). Finally, the can be expressed as follows:
where and are the coefficients of and ; and are the estimations of Formula 2.
In this study, the accuracy verification process is as follows: (1) we matched and compared the obtained by using Lee15 and the improved model with the measured data, respectively. (2) in order to obtain more matched data, we set the time window of daily data to 24 h and defined the spatial window as the of the pixel in which the station is located or eight adjacent pixels, resulting in a total of 225 pairs of matched data. In addition, the accuracy tests conducted in this study employed the following indices: R² (Coefficient of Determination), Root Mean Square Error (RMSE) and Mean Absolute Error (MAE). The RMSE and MAE are calculated using the following formulas:
In these formulas, and represent the measured and estimated values of the sample i. N represents the total number of samples, which in this study is equal to 225.
The Mann-Kendall trend test is a nonparametric test model widely employed in the analysis of time series for trends and abrupt changes (Subash et al., 2011). This test method does not require a specific sequence distribution and is not sensitive to anomalies. The process of the Mann-Kendall trend test is as follows: for the sequence Xi = (x1, x2,…, xn), the size relationship between xi and xj in all dual values (xi, xj, j > i) (set as S) must first be determined, and then a significance test must be performed on it. The test statistic formulas are as follows:
In addition, the choice of significance test statistics is contingent on the length of the time series. Given that the time series in question has a length of 21 years (2003-2023), it can be reasonably assumed that the statistical statistic S approximately follows a standard normal distribution. Accordingly, the significance test is performed using the test statistic Z, which is calculated in accordance with Formulas 9 and 10.
In Formulas 9 and 10, n represents the number of data points in the sequence, m denotes the number of knots (repeated data groups) in the sequence, is the width of the knots (number of repeated data points in the group i), and Var(S) is the variance of S. During the test, the significance level is taken as α = 0.05, and . When , the trend is not considered to be significant; if , the trend is considered to be significant. Moreover, if , the sequence is considered to have an upward trend, and if , the sequence is considered to have a downward trend.
Sen’s slope, also known as Sen’s slope estimation, represents a nonparametric approach to computing the trend slope. The slope indicates the amplitude of the variability in the of the series. The Sen’s trend represents the median of the calculated sequence, which effectively reduces the impact of noise and is not affected by the singular values of the sequence. The Sen’s slope is an effective method for reflecting the extent of change in the trend of a sequence. However, it is not a standalone indicator of trend significance and can be assessed in conjunction with the Mann-Kendall trend test method (Burn and Elnur, 2002), which has a wide range of applications in the analysis of hydrometeorological data (da Silva et al., 2015). For a time series Xi = (x1, x2,…, xn), the Sen’s slope estimates of the trend β are calculated as follows:
The median is the median function, and when β>0, the time series shows an upward trend; When β<0, the time series shows a downward trend.
The temporal and spatial means and anomalies of various properties are commonly employed to elucidate spatio-temporal variations in geophysical properties (Shang et al., 2011). This approach allows us to calculate the monthly, quarterly, annual, and multi-year spatial mean and temporal anomaly of , respectively. The exact calculation is as follows: for a given pixel i of an extracted month in an extracted year, the monthly average of is calculated by adding all valid values for each day of the month and dividing them by the number of valid days. The calculation of annual average is the sum of the average values of all valid months in a year, and then divided by the number of months with valid values. Similarly, the multi-year monthly average is the sum of the monthly average effective value of for pixel i from 2003 to 2023 divided by the number of months in which the effective value exists. Multi-year quarterly average is obtained from quarterly statistics based on the multi-year monthly average in a similar way. Time anomalies count the difference between a month in a given year and the long-term average for that month. This can reflect the anomaly of that month in a given year with respect to the long-term average. The methods employed to calculate these averages and anomalies are outlined in detail in Shang et al. (2011).
Following a series of processing steps, including projection, clipping and quality control band screening, all MODIS data are calculated in Matlab using the Lee15 and the improved model, with the objective of obtaining the daily of the area within 100 km of the east coast of China. After the data cleaning process, a total of 225 pairs of data were used for accuracy verification. The results demonstrate that the RMSE and MAE of the improved algorithm have decreased from 1.49 to 1.32 and from 0.96 to 0.94 when compared to the Lee15, respectively (Figures 2A, C). More importantly, the coefficient of determination R² increased from 0.63 to 0.70 (Figures 2A, C). The improvement of these indicators indicates that our model has better adaptability when dealing with high turbidity water. Moreover, to demonstrate the strength of the improved model, we compare it with the new three-class model, using the similarity principle (Xiang et al., 2023). This new three-class model, which classifies water as clear, extremely turbid, and moderately turbid water, employs the Lee15, simple semi-analytical, and weighted average methods, respectively. The accuracy of this model is still inferior to ours, with an R2 of 0.65, RMSE of 1.69 and MAE of 1.11 (Figure 2B). These results further solidify the reliability of our improved model for handling complex water. Consequently, the improved algorithm exhibits superior performance, whereas the Lee15 may have erroneously classified turbid water as clear water in the estimation, resulting in an overestimation of .
Figure 2. (A) In situ compared to derived from MODIS using the Lee15. (B) In situ compared to derived from MODIS using the new three-class model. (C) In situ compared to derived from MODIS using the improved model and estimated (bias corrected).
Furthermore, Figure 2B illustrates that the in situ measured appears to be consistently lower than the estimated . The slope is 1.04, which is close to 1, indicating a discrepancy between the fitted line and 1:1 line. This discrepancy may be attributed to differences in sample size and measurement time (Feng et al., 2019). Therefore, the correlation between the two variables can be used to adjust the bias in the estimate. The correlation can be expressed as follows:
It can be further expressed as follows:
Following calculation, the adjusted model has a MAE of 0.88, RMSE of 1.24, and R2 of 0.70, indicating an improvement in accuracy compared to the before adjustment model. Therefore, this study used the improved model to invert and adjusted the results according to Formula 13, thereby making them more closely aligned with the true .
To elucidate the long-term spatial variation of within 100 km coast waters of eastern China, we calculated the annual average based on the retrieved daily , with spatial resolution of 1 km (Figure 3). The results indicated that the average was 1.32 m from 2003 to 2023. On the whole, showed an increasing trend with the increase of coastal distance. In particular, regions with high values of were concentrated in the north of the YS, south of Shandong Peninsula, and the far coast of the ECS, while regions with low values of were mainly in the BS, the northern coastal zone of Jiangsu (NJCZ), and the nearshore of the ECS. Moreover, seawater adjacent to the shoreline has a consistently lower due to its greater impact from runoff inputs, more complex hydrodynamics, and stronger human activity compared to the ocean, resulting in a higher probability of sediment resuspension (Zhao et al., 2023). Meanwhile, the in the ECS exhibited typical circular distribution characteristics, and the in the NJCZ did not show considerable variation with increasing distance from the coast, forming a tongue-shaped low area.
Figure 3. Spatial distribution of annual within 100 km of the eastern coast of China from 2003 to 2023.
Sen’s slope estimation and Mann Kendall significance test were performed to explore the interannual trend of in the study. The results indicated that the number of pixels with significant increase, significant decrease, and no significant variation in from 2003 to 2023 accounted for 20.84%, 1.14%, and 78.02% of the study area, respectively (Figure 4; Supplementary Table S2). This indicates that has remained relatively stable over the past 21 years for most of the area within 100 km of the eastern coast of China, and that regions with significantly increased are much larger than those with significantly decreased . Specifically, the regions with significantly increased mainly belong to northern China, including the coastal waters of the BS and the northern YS, while the regions with significantly decreased are the DZBC and HZBC of the YS. In contrast, the sea regions with insignificant variation in include the ECS, the southern and central YS, and the central and northern BS (Figures 4A, B). These variations in in coastal waters further indicate a trend of improving water quality along the northern coast of China during the period 2003 to 2023.
Figure 4. The distribution of annual variation trend of within 100 km of the eastern coast of China (A). The distribution of annual significance test of the within 100 km of the eastern coast of China (B).
Furthermore, we calculated the anomalies of in three sea areas to investigate whether there is a periodic pattern of variations. The results showed that the anomalies of in the BS and YS exhibited a significant increase trend in the past 21 years (p = 0.002 and p = 0.025, respectively), with an average increase of 0.009 m/year and 0.007 m/year, respectively (Figures 5A, B). However, some anomalies in the ECS demonstrated an insignificant change (p = 0.188; Figure 5C). Concurrently, the anomalies of in different seas showed a phased pattern of change. In detail, the BS can be divided into three stages, with significantly decreasing from January 2003 to August 2007 (p = 0.001), while there was no significant variation from September 2007 to December 2015 (p = 0.381), and significantly increasing from January 2016 to December 2023 (p < 0.0001) (Figure 5A). The YS can be divided into two stages, with no significant change in between January 2003 and July 2009 (p = 0.498), and a significant increase between August 2009 and December 2023 (p < 0.0001) (Figure 5B). Although there has been a slight increase in in the ECS on the whole, there has not been a significant variation (p = 0.188; Figure 5C), which is due to the large fluctuations in during the year. Overall, the anomalies in the BS and YS have increased significantly over the past 21 years, while there is no significant variation in the ECS, and the anomalies in in different seas exhibit different phases of the variability pattern.
Figure 5. The time series and corresponding linear trends of the spatially averaged monthly anomalies for the coastal areas within 100 km of the BS (A), YS (B), and ECS (C).
To clarify the long-term seasonal variability trend of , this study calculated the monthly and quarterly average for a 100 km sea area along the eastern coast of China from 2003 to 2023 based on the daily product. As can be seen in Figure 6, the of coastal waters had a conspicuous seasonal variation, with the performance being summer (mean = 1.81 m) > autumn (mean = 1.33 m) > spring (mean = 1.21 m) > winter (mean = 0.87 m). Specifically, in spring, the of the BS, YS and ECS coastlines, as well as NJCZ, was lower than 4 m, while the of the northern YS, southern Shandong Peninsula, and far shore of the ECS was higher, reaching 12 m. By summer, increased compared to spring, with the highest values of reaching 8 m, 12 m and 16 m in the BS, YS, and ECS, respectively. Subsequently, the of seawater began to decrease in autumn. In winter, the of all sea areas reached the lowest value of the year, with the smallest spatial differences and the highest in the BS, YS, and ECS was less than 3 m, 9 m, and 12 m, respectively (Figure 6; Supplementary Table S1).
Figure 6. Spatial distribution of seasonal of within 100 km of the eastern coast of China from 2003 to 2023.
We further analyzed the monthly distribution of the maximum and minimum for each pixel within 100 km of the eastern coast of China based on the monthly average products from 2003 to 2023 to gain a deeper understanding of the monthly variability of . The results showed that the time interval between the maximum and minimum of is usually ~6 months. Overall, mainly reached maximum in June, July, or August (Supplementary Figure S2A), while reaching minimum in December, January, and February (Supplementary Figure S2B). Specifically, the maximum and minimum of in the southern of the Shandong Peninsula appeared earliest in June and December, respectively (Supplementary Figure S2). Subsequently, the maximum of in the coastal waters of the BS and the northern of the YS mainly occurred in July, while the minimum was in February. The maximum and minimum of were both one month later than those in the central BS. Furthermore, the maximum in the central and northern YS generally occurred in August, while the minimum occurred in January. As for the ECS, the maximum of occurred in June, August, or September, while the minimum was in January (Supplementary Figure S2).
Estuaries are important channels for the ocean to receive runoff and sediment, and their is strongly influenced by the interaction between the ocean and the land environment (An et al., 2023). The rivers corresponding to ELHR, EHHR, EYR, EYTR, EQHB, and EMJR are the main rivers in China, spanning different climate zones and geological conditions along the eastern coast of China, as well as in the areas with the highest intensity of human activities. In addition, these estuaries receive 69% of the runoff and 78% of the sediment input from China’s land to the ocean. Therefore, these six estuaries have typical representativeness and the area with 32 km x 32 km of them were used to analyze the spatiotemporal variations of (Figure 1). In order to explore the long-term trend of in these estuaries, we calculated the annual average of each pixel from 2003 to 2023 and defined valid pixels as pixels with an average greater than 0 and sample size of no less than 15. The results showed that the EMJR had the highest average (2.64 m) of from 2003 to 2023, followed by the EYR (1.65 m), EHHR (1.13 m), ELHR (0.73 m), and EQHB (0.66 m), while the average of the EYTR was the lowest, at 0.64 m (Figure 7). On a long-term trend, the of ELHR, EHHR, EYR, EQHB, and EMJR showed a significant increase, while there was no significant change in EYTR (Figure 7). However, the corresponding R2 in EQHB and EMJR is at an extremely low level (R2< 0.02), although the change trends of their is significant (p< 0.05; Figures 7E, F), which means that the actual explanation of time on variation is weak, leading to statistically false positive results (Edwards et al., 2008). Therefore, the of EQHB and EMJR is likely to have insignificant trend of change and belong to normal hydrological and meteorological fluctuations.
Figure 7. The interannual variations and linear trends of each pixel in the estuarine regions. Each line represents a single pixel, with darker colors indicating higher latitudes and lighter colors indicating lower latitudes. (A–F) represent ELHR, EHHR, EYR, EYTR, EQHB, and EMJR, respectively.
Furthermore, we calculated the monthly average for each estuary from 2003 to 2023 to further understand the annual variation characteristics of . The results showed that the of ELHR, EHHR, EYR, and EMJR were the highest during the summer months of the year, with a single peak trend. By comparison, the EYTR and EQHB exhibited a bimodal pattern with peaks in autumn and spring, with the highest occurring in autumn. Moreover, the of ELHR, EYTR, and EQHB fluctuated less within the year (Figures 8A, D, E), while the of EHHR, EYR, and EMJR showed obvious seasonality and instability (Figures 8B, C, F).
Figure 8. The of the six estuaries changes during the year. (A–F) represent ELHR, EHHR, EYR, EYTR, EQHB, and EMJR, respectively.
, as a significant indicator reflecting variation in water quality (Guo et al., 2023a), its attenuation process from top to bottom in natural water bodies is mainly dominated by the inherent optical properties (IOP) of water, including absorption coefficient and scattering coefficient. Under natural conditions, the main substances that affect two coefficients include colored dissolved organic matter (CDOM), suspended particulate matter (SPM), and phytoplankton (Shao et al., 2017; Zhou et al., 2021). Therefore, the reasons for variation of can essentially be attributed to the effects of climate change, seawater dynamics, and human activities exerted through CDOM, SPM, and phytoplankton (Harvey et al., 2019; Zhou et al., 2021). Among them, phytoplankton is considered one of the most crucial influencing factors (Shao et al., 2017; Zhou et al., 2021). However, there is usually the highest density of phytoplankton in the summer (Gong et al., 2003; Yamaguchi et al., 2012), whereas the highest remains at the same season (Figure 6), as well as in studies for seas of China (Guo et al., 2022, 2023a). Similarly, the lowest values of phytoplankton density and seawater are observed simultaneously in winter (Figure 6) (Guo et al., 2022; Wang et al., 2023a). This phenomenon suggests that the driving effect of phytoplankton in coastal waters on long-term changes in is secondary, while CDOM and SPM are dominant. The same phenomenon has also been observed in the Bothnian Sea, Baltic Sea, and Skagerrak (Harvey et al., 2019).
Our results suggest that the of different seas in eastern China exhibited distinct change trends from 2003 to 2023, with a significant increase in in the coastal waters of the BS and the north YS, while in the DZBC and HZBC of the YS declined significantly, with no significant changes in other regions (Figures 4A, B). In the BS, the significant increase in was related to the decline in SPM to a large extent, as in the past 21 years, the SPM of surface seawater in the BS had shown a decreasing trend against the background of weakened wind and wave intensity (Li et al., 2022), particularly in the southern region (Zhao et al., 2022). This phenomenon is in accordance with our results.
In the YS, the long-term variation of in different regions exhibit different characteristics (Figures 4A, B). Among the regions, the northern region exhibits a significant increase in , while the southwest regions of HZBC and DZBC exhibit a significant decrease. This is in line with the research results of Zhou et al. (2021) and Dong et al. (2011). The decrease in of HZBC and DZBC from 2003 to 2023 can be attributed to sediment input and environmental pollution (Gao et al., 2023; Liang et al., 2023; Wang et al., 2024). Indeed, the excrement from industrial and agricultural production and aquaculture in the vicinity of these two areas has been continuously increasing since the 21st century (Pan et al., 2014; Liang et al., 2023). This puts tremendous pressure on the seawater. In one respect, the abundant nutrients can induce outbreaks of red and green tides, which can impede the penetration of light into seawater and reduce (Liu et al., 2009). In another respect, a large amount of microplastics, as one of the suspended pollutants, can not only enrich CDOM and SPM, but also absorb and scatter light (Hoellein et al., 2019; Gao et al., 2023), this can all lead to a decrease in . In addition, the DZBC is located in a small muddy sediment area in the northwest of the South YS (Zhang et al., 2016), and recent coastal projects such as dredging and sand disposal can also promote further elevate the concentration of suspended sediments. It is noteworthy that, despite the seawater along NJCZ exhibiting minimal change in , this has been the case for a long time. In truth, this region is situated between the old channel of the Yellow River Delta and the Yangtze River Delta, and a substantial amount of sediment accumulated in these deltas can significantly reduce after suspension and diffusion (Rao et al., 2017; Su et al., 2017). At the same time, sediment from the Huai River can also contribute to the reduction of (An et al., 2023). As a result, this area has become one of the most turbid coastal waters in China and has formed the famous Radial Sand Ridge (RSR), keeping its at a low level year-round.
In terms of long-term seasonal variability, the of coastal seawater is the highest in summer, followed by autumn and spring, and the lowest in winter. This change can mainly be attributed to seasonal variation patterns of temperature and wind. In summer, the increase in thermocline thickness caused by high temperatures enhances the vertical stability of water bodies, and the weak monsoon makes it difficult for the upper and lower layers of seawater to mix (Guo et al., 2023c), resulting in high . In contrast, the thickness of the thermocline in winter decreases and the monsoon strengthens, which can promote the enhancement of vertical convection in seawater, thereby increasing the concentration of suspended solids (Shang et al., 2016; Mao et al., 2018; Guo et al., 2022), resulting in low . Spring and autumn seasons are a transitional period between summer and winter, with relatively moderate temperature and monsoon intensity, so is usually at the intermediate level. Furthermore, the seasonal variability of shows an increasing trend with increasing offshore distance. This is due to the fact that coastal water bodies are more strongly affected by land runoff inputs, seawater dynamics (e.g., tides, waves, and coastal currents), and sedimentary suspension and transportation than far shore water bodies (Lewis et al., 1988; Megard and Berman, 1989; Idris et al., 2022).
In natural water bodies, CDOM and SPM are the two most important direct factors affecting (Shao et al., 2017; Harvey et al., 2019; Zhou et al., 2021). However, due to the difficulty in obtaining large-scale and long-term CDOM and SPM data, we chose the sediment transport volume and runoff to indicate CDOM and SPM to reveal their effects on variation in the six typical estuaries from 2003 to 2023. The results showed that there is spatial variation in the correlations between annual runoff and to some extent: the of estuaries in the northern region (ELHR, EHHR, and EYR) had a positive relationship with annual runoff (Figures 9A, C, E); while a negative relationship occurred in the southern region (EYTR, EQHB, and EMJR) (Figures 9G, I, K). Among them, only significantly increases with the increase of annual runoff at the EHHR (Figure 9C), but significantly decreases with the increase of annual runoff at the EMJR (Figure 9K). This phenomenon cannot be separated from the increased intensity of human activity on the estuary. Since the 21st century, northern China has vigorously developed vegetation restoration projects and reservoir construction projects (Li et al., 2016). These ecological projects have the potential to effectively reduce the sediment transport of rivers (Wang et al., 2016). As the annual runoff increases, the water entering the estuary will be replaced more frequently, which helps to maintain the cleanliness of the water body and increase . In contrast, South China has stronger industrial activity and denser population than north China (Liang et al., 2021), which means that rivers in the South will accept more pollutants and suspended solids and bring them into the estuary, leading to a decrease in in the estuary.
Figure 9. Correlations of estuary with annual runoff and sediment transport volume in ELHR (A, B), EHHR (C, D), EYR (E, F), EYTR (G, H), EQHB (I, J), and EMJR (K, L).
For the relationship of annual sediment discharge and , the of EHHR, EYR, EYTR, EQHB, and EMJR were negatively correlated with their sediment transport volume, while a positive relationship was observed in ELHR (Figure 9). This result serves to reinforce the conclusion that an increase in sediment transport volume results in a reduction in the of seawater. Notably, the annual runoff and sediment transport volume has a significant negatively correlation with in EMJR (p = 0.0056, p< 0.0001) (Figures 9K, L). We believe that this phenomenon is mainly attributable to the relatively clear water of the EMJR (Figure 7). The concentration of SPM in the EMJR is lower than other turbid estuaries. The increase in EMJR sediment input significantly increased the concentration of SPM in the estuary, leading to a rapid decrease in of EMJR with increasing annual runoff and sediment transport volume. However, the SPM concentration and eutrophication in turbid estuaries are usually at high levels, and the reduction of QAQ requires more sediment and pollutants than in clean estuaries. Thus, the correlation between and estuarine runoff as well as sediment transport is closely linked to the long-term cleanliness of estuarine water quality.
Additionally, we also observed that the of the estuary shows a certain trend of variation with latitude (Figure 8). Hence, we further calculated their correlations. The results showed that the of ELHR and EQHB significantly decreased with increasing latitude (all p values< 0.0001; Supplementary Figures S3A, E), whereas the of EHHR, EYR, and EYTR were significantly increased with latitude (all p values< 0.0001; Supplementary Figures S3B-D), and the of EMJR had no significant calculation with latitude (p = 0.0503; Supplementary Figure S3F). It is worth noting that although the relationship between and latitude of EHHR and EQHB is significant (p< 0.0001), their corresponding R2 is at a lower level (all R2 values = 0.0283; Supplementary Figures S3B, E). This means that the effect of latitude on the of EHHR and EQHB probably be weak. Actually, the effect of latitude on is still dominated by the offshore distance of the seawater. On the one side, the sediment transport direction at the ELHR is from north to south (Figure 1), which means that the higher the latitude of the seawater, the closer it is to the shore, thereby synchronously reducing . On the other side, the EYR we have chosen is located on the north bank of the estuary (Figure 1), so the higher the latitude, the farther offshore the seawater is, and the corresponding increase in . In addition, the selected EYTR gradually moves away from Shanghai (China’s economic center) from south to north (Shi and Liu, 2018), resulting in the increased .
The deteriorating ecological health of coastal waters has become one of the obstacles to sustainable development in China. In this study, an improved model was used to invert and analyze the and its spatiotemporal variations in coastal waters within a 100 km of eastern China from 2003 to 2023. Our results provide more accurate and continuous estimates of for nearshore turbid waters compared to those obtained by the original model, so the dataset also has important application value in marine ecological protection and fishery production. In addition, this study has achieved considerable advance in both spatiotemporal resolution and time series length than the previous studies. In particular, the daily data with a spatial resolution of 1km we obtained provides a reliable basis for in-depth research on the dynamic changes of nearshore water bodies. Furthermore, considering the estuary as the intersection of ocean and land, our analysis of the changes and driving factors of typical estuaries can provide novel insights for scientific management and ecological restoration of estuaries at different latitudes.
However, there are still some limitations to this study. For example, although the data we use can cover the major area, there is a possibility of atmospheric correction failure in waters with an extremely high turbidity, resulting in a smaller amount of effective data being obtained (Guo et al., 2023a). Therefore, the improvement of atmospheric correction algorithms, the utilization of hyperspectral and multispectral data, and the fusion of multi-source spatiotemporal data should be the priority in the future. In addition, the of seawater is not only directly affected by CDOM, SPM, and phytoplankton, but also indirectly disturbed by hydrodynamic and climatic factors (Lewis et al., 1988; Megard and Berman, 1989; Idris et al., 2022). Nevertheless, this study only quantificationally analyzed the effects of these direct influencing factors on , making it difficult for us to fully comprehend the spatiotemporal variability pattern of seawater . Consequently, we should consider the coupling relationship among multiple factors to further clarify the inherent mechanism of variation in seawater .
In this study, we employed an improved model based on Class II water bodies to first invert the of the coastal waters within 100 km of eastern China from 2003 to 2023 with a spatial resolution of 1 km. Our results achieve a higher accuracy in calculating for offshore seas than those derived from the Lee15. On the whole, showed an increasing trend with increasing coastal distance. The high were located in the northern Yellow Sea, the southern Shandong Peninsula, and the far shore of the East China Sea, while the low were located in the coastal areas of the Bohai Sea and northern Jiangsu. Concerning long-term variations, of partial regions (20.84%) exhibited significant increases, particularly in the Bohai Sea and the northern Yellow Sea. Only a few areas (1.14%) showed significant decreases, including Dingzi Bay and Haizhou Bay in the Yellow Sea. In terms of seasonal changes, the highest occurred in summer, followed by autumn, spring, and winter, and the extend of seasonal variation tends to increase with greater offshore distance. Furthermore, there is a spatial differentiation in the correlations between and the annual runoff of rivers. The positive correlations are observed in the north and negative correlations in the south, contrasting with the negative correlations between and annual sediment transport. The findings of this study provide valuable data that can be used to gain insight into long-term changes in the coastal environment of China, which offers novel insights for us to deeply understand the spatiotemporal changes in seawater .
The raw data supporting the conclusions of this article will be made available by the authors, without undue reservation.
SC: Conceptualization, Data curation, Formal analysis, Investigation, Methodology, Resources, Software, Validation, Visualization, Writing – original draft, Writing – review & editing. FX: Conceptualization, Funding acquisition, Investigation, Methodology, Project administration, Resources, Supervision, Validation, Visualization, Writing – review & editing. MC: Methodology, Writing – review & editing, Conceptualization, Formal Analysis. ZW: Methodology, Writing – review & editing, Conceptualization, Data curation. JL: Writing – review & editing, Conceptualization, Data curation. YD: Writing – review & editing, Conceptualization, Supervision.
The author(s) declare financial support was received for the research, authorship, and/or publication of this article. This study was supported by the Key R & D projects in Hubei Province (NO: 2023BCB104), the Key Laboratory for Environment and Disaster Monitoring and Evaluation of Hubei, the Innovation Academy for Precision Measurement Science and Technology, and Chinese Academy of Sciences.
We thank the NASA for providing MODIS data, and we also thank the National Field Science Observation and Research Station of Jiaozhou Bay Marine Ecosystem in Shandong Province and the National Earth System Science Data Center for providing transparency measurement data.
The authors declare that the research was conducted in the absence of any commercial or financial relationships that could be construed as a potential conflict of interest.
The authors declare that no Generative AI was used in the creation of this manuscript.
All claims expressed in this article are solely those of the authors and do not necessarily represent those of their affiliated organizations, or those of the publisher, the editors and the reviewers. Any product that may be evaluated in this article, or claim that may be made by its manufacturer, is not guaranteed or endorsed by the publisher.
The Supplementary Material for this article can be found online at: https://www.frontiersin.org/articles/10.3389/fmars.2024.1503177/full#supplementary-material
An Y. H., Feng X. L., Liu J., Saito Y., Qiu J. D., Zhang X., et al. (2023). Development of a Middle-Late Holocene subaqueous clinoform in the northern Jiangsu coastal zone, western South Yellow Sea. Geomorphology 439, 108853. doi: 10.1016/j.geomorph.2023.108853
Basset A., Carlucci D., Fiocca A., Vignes F. (2001). Water transparency and health of coastal salt marshes: simple enclosure experiments on nutrient dynamics. Aquat. Conserv. Mar. Freshw. Ecosyst. 11, 273–279. doi: 10.1002/aqc.455
Burn D. H., Elnur M. A. H. (2002). Detection of hydrologic trends and variability. J. Hydrol. 255, 107–122. doi: 10.1016/S0022-1694(01)00514-5
Cael B. B., Bisson K., Boss E., Dutkiewicz S., Henson S. (2023). Global climate-change trends detected in indicators of ocean ecology. Nature 619, 551–554. doi: 10.1038/s41586-023-06321-z
Chen M., Xiao F., Wang Z., Feng Q., Ban X., Zhou Y., et al. (2022). An improved QAA-based method for monitoring water clarity of Honghu lake using landsat TM, ETM+ and OLI data. Remote Sens. 14 (15), 3798. doi: 10.3390/rs14153798
Dai Y., Yang S., Zhao D., Hu C., Xu W., Anderson D. M., et al. (2023). Coastal phytoplankton blooms expand and intensify in the 21st century. Nature 615, 280–284. doi: 10.1038/s41586-023-05760-y
Dai Z. J., Mei X. F., Darby S. E., Lou Y. Y., Li W. H. (2018). Fluvial sediment transfer in the Changjiang (Yangtze) river-estuary depositional system. J. Hydrol. 566, 719–734. doi: 10.1016/j.jhydrol.2018.09.019
da Silva R. M., Santos C. A. G., Moreira M., Corte-Real J., Silva V. C. L., Medeiros I. C. (2015). Rainfall and river flow trends using Mann-Kendall and Sen’s slope estimator statistical tests in the Cobres River basin. Nat. Hazards 77, 1205–1221. doi: 10.1007/s11069-015-1644-7
Dong L. X., Guan W. B., Chen Q., Li X. H., Liu X. H., Zeng X. M. (2011). Sediment transport in the Yellow Sea and East China Sea. Estuar. Coast. Shelf Sci. 93, 248–258. doi: 10.1016/j.ecss.2011.04.003
Edwards L. J., Muller K. E., Wolfinger R. D., Qaqish B. F., Schabenberger O. (2008). An R2 statistic for fixed effects in the linear mixed model. Stat. Med. 27, 6137–6157. doi: 10.1002/sim.3429
Fang G., Yu H., Sheng H., Tang Y., Liang Z. (2021). Comparative analysis of microbial communities between water and sediment in Laoshan Bay marine ranching with varied aquaculture activities. Mar. pollut. Bull. 173, 112990. doi: 10.1016/j.marpolbul.2021.112990
Feng L., Hou X. J., Zheng Y. (2019). Monitoring and understanding the water transparency changes of fifty large lakes on the Yangtze Plain based on long-term MODIS observations. Remote Sens. Environ. 221, 675–686. doi: 10.1016/j.rse.2018.12.007
Gao S. K., Yan K., Liang B. G., Shu R. L., Wang N., Zhang S. (2023). The different ways microplastics from the water column and sediment accumulate in fish in Haizhou Bay. Sci. Total Env. 854, 158575. doi: 10.1016/j.scitotenv.2022.158575
Gong G.-C., Wen Y.-H., Wang B.-W., Liu G.-J. (2003). Seasonal variation of chlorophyll a concentration, primary production and environmental conditions in the subtropical East China Sea. Deep Sea Res. Part II Top. Stud. Oceanogr. 50, 1219–1236. doi: 10.1016/S0967-0645(03)00019-5
Gordon H. R., Morel A. Y. (1983). “In — Water Algorithms,” in Remote Assessment of Ocean Color for Interpretation of Satellite Visible Imagery: A Review. Eds. Gordon H. R., Morel A. Y. (Springer US, New York, NY), 24–67.
Guo J., Nie Y., Sun B., Lv X. (2022). Remote sensing of transparency in the China seas from the ESA-OC-CCI data. Estuar. Coast. Shelf Sci. 264, 107693. doi: 10.1016/j.ecss.2021.107693
Guo J., Pan H., Cao R., Wang J., Lv X. (2023a). Multiple timescale variations in water transparency in the eastern China seas over the period 1997–2019. J. Geophys. Res. Oceans 128 (4), e2022JC019170. doi: 10.1029/2022jc019170
Guo J. T., Pan H. D., Cao R. C., Wang J. F., Lv X. Q. (2023b). Multiple timescale variations in water transparency in the eastern China seas over the period 1997-2019. J. Geophys. Res.-Oceans 128, e2022JC019170. doi: 10.1029/2022JC019170
Guo J., Zhao Z., Cao R., Lv X. (2023c). Regional to global assessments of ocean transparency dynamics from 1997 to 2019. Prog. Oceanogr. 219, 103165. doi: 10.1016/j.pocean.2023.103165
Harvey E. T., Walve J., Andersson A., Karlson B., Kratzer S. (2019). The effect of optical properties on secchi depth and implications for eutrophication management. Front. Mar. Sci. 5. doi: 10.3389/fmars.2018.00496
He X., Pan D., Mao Z. (2004). Water-transparency (Secchi Depth) monitoring in the China Sea with the SeaWiFS satellite sensor (SPIE), 5568. doi: 10.1117/12.564605
Hoellein T. J., Shogren A. J., Tank J. L., Risteca P., Kelly J. J. (2019). Microplastic deposition velocity in streams follows patterns for naturally occurring allochthonous particles. Sci. Rep. 9, 3740. doi: 10.1038/s41598-019-40126-3
Hori K., Saito Y., Zhao Q. H., Wang P. X. (2002). Architecture and evolution of the tide-dominated Changjiang (Yangtze) River delta, China. Sediment. Geol. 146, 249–264. doi: 10.1016/S0037-0738(01)00122-1
Idris M. S., Siang H. L., Amin R. M., Sidik M. J. (2022). Two-decade dynamics of MODIS-derived Secchi depth in Peninsula Malaysia waters. J. Mar. Syst. 236, 103799. doi: 10.1016/j.jmarsys.2022.103799
Jia T., Zhang Y., Weng C., Dong R. (2022). Improving remote sensing retrieval of global ocean transparency with optical water classification. Ecol. Indic. 143, 109359. doi: 10.1016/j.ecolind.2022.109359
Jiang D. L., Matsushita B., Setiawan F., Vundo A. (2019). An improved algorithm for estimating the Secchi disk depth from remote sensing data based on the new underwater visibility theory. ISPRS J. Photogramm. Remote Sens. 152, 13–23. doi: 10.1016/j.isprsjprs.2019.04.002
Lee Z., Shang S., Hu C., Du K., Weidemann A., Hou W., et al. (2015). Secchi disk depth: A new theory and mechanistic model for underwater visibility. Remote Sens. Environ. 169, 139–149. doi: 10.1016/j.rse.2015.08.002
Lee Z., Shang S., Qi L., Yan J., Lin G. (2016). A semi-analytical scheme to estimate Secchi-disk depth from Landsat-8 measurements. Remote Sens. Environ. 177, 101–106. doi: 10.1016/j.rse.2016.02.033
Lewis M. R., Kuring N., Yentsch C. (1988). Global patterns of ocean transparency - implications for the new production of the open ocean. J. Geophys. Res.-Oceans 93, 6847. doi: 10.1029/JC093iC06p06847
Li P., Chen S., Ke Y., Ji H., Li P., Fan Y. (2022). Spatiotemporal dynamics of suspended particulate matter in the Bohai Sea, China over the past decade from the space perspective. Sci. Total Environ. 851, 158210. doi: 10.1016/j.scitotenv.2022.158210
Li S., Liang W., Fu B., Lü Y., Fu S., Wang S., et al. (2016). Vegetation changes in recent large-scale ecological restoration projects and subsequent impact on water resources in China’s Loess Plateau. Sci. Total Environ. 569–570, 1032–1039. doi: 10.1016/j.scitotenv.2016.06.141
Liang L., Chen M., Luo X., Xian Y. (2021). Changes pattern in the population and economic gravity centers since the Reform and Opening up in China: The widening gaps between the South and North. J. Clean. Prod. 310, 127379. doi: 10.1016/j.jclepro.2021.127379
Liang B. G., Gao S. K., Wang Z. Y., Shu R. L., Wang N., Tan W. J., et al. (2023). Spatial distribution characteristics of microplastics in the seawater column and sediments of the artificial reef area and adjacent water in Haizhou Bay. Sci. Total Env. 900, 166236. doi: 10.1016/j.scitotenv.2023.166236
Liu D., Keesing J. K., Xing Q., Shi P. (2009). World’s largest macroalgal bloom caused by expansion of seaweed aquaculture in China. Mar. pollut. Bull. 58, 888–895. doi: 10.1016/j.marpolbul.2009.01.013
Liu Y., Li J., Xiao C., Zhang F., Wang S., Yin Z., et al. (2022). A classification-based, semianalytical approach for estimating water clarity from a hyperspectral sensor onboard the ZY1-02D satellite. IEEE Trans. Geosci. Remote Sens. 60, 1–14. doi: 10.1109/TGRS.2022.3161651
Mao Y., Wang S. Q., Qiu Z. F., Sun D. Y., Bilal M. (2018). Variations of transparency derived from GOCI in the Bohai Sea and the Yellow Sea. Opt Express 26, 12191–12209. doi: 10.1364/Oe.26.012191
Megard R. O., Berman T. (1989). Effects of algae on the secchi transparency of the Southeastern Mediterranean-sea. Limnol. Oceanogr. 34, 1640–1655. doi: 10.4319/lo.1989.34.8.1640
Melo C. E., Lima J. D., da Silva E. F. (2009). Relationships between water transparency and abundance of Cynodontidae species in the Bananal floodplain, Mato Grosso, Brazil. Neotrop. Ichthyol. 7, 251–256. doi: 10.1590/S1679-62252009000200017
Milliman J. D., Meade R. H. (1983). World-wide delivery of river sediment to the oceans. J. Geol. 91, 1–21. doi: 10.1086/628741
Molner J. V., Soria J. M., Pérez-González R., Sòria-Perpinyà X. (2023). Estimating water transparency using sentinel-2 images in a shallow hypertrophic lagoon (The Albufera of Valencia, Spain). Water 15 (20), 3669. doi: 10.3390/w15203669
Msusa A. D., Jiang D. L., Matsushita B. (2022). A semianalytical algorithm for estimating water transparency in different optical water types from MERIS data. Remote Sens. 14, 868. doi: 10.3390/rs14040868
Pan J., Pan J.-F., Wang M. (2014). Trace elements distribution and ecological risk assessment of seawater and sediments from Dingzi Bay, Shandong Peninsula, North China. Mar. pollut. Bull. 89, 427–434. doi: 10.1016/j.marpolbul.2014.10.022
Qing S., Cui T. W., Lai Q., Bao Y. H., Diao R. X., Yue Y. L., et al. (2021). Improving remote sensing retrieval of water clarity in complex coastal and inland waters with modified absorption estimation and optical water classification using Sentinel-2 MSI. Int. J. Appl. Earth Obs. Geoinform. 102, 102377. doi: 10.1016/j.jag.2021.102377
Qiu R. T., Wang S. L., Shi J. K., Shen W., Zhang W. Z., Zhang F. F., et al. (2023). Sentinel-2 MSI observations of water clarity in Inland waters across Hainan island and implications for SDG 6.3.2 evaluation. Remote Sens. 15, 1600. doi: 10.3390/rs15061600
Rao W. B., Mao C. P., Wang Y. G., Huang H. M., Ji J. F. (2017). Using Nd-Sr isotopes and rare earth elements to study sediment provenance of the modern radial sand ridges in the Southwestern Yellow Sea. Appl. Geochem. 81, 23–35. doi: 10.1016/j.apgeochem.2017.03.011
Shang S., Dong Q., Lee Z., Li Y., Xie Y., Behrenfeld M. (2011). MODIS observed phytoplankton dynamics in the Taiwan Strait: an absorption-based analysis. Biogeosciences 8, 841–850. doi: 10.5194/bg-8-841-2011
Shang S. L., Lee Z., Shi L. H., Lin G., Wei G. M., Li X. D. (2016). Changes in water clarity of the Bohai Sea: Observations from MODIS. Remote Sens. Environ. 186, 22–31. doi: 10.1016/j.rse.2016.08.020
Shao T., Zheng H., Song K., Zhao Y., Zhang B. (2017). Influence of environmental factors on absorption characteristics of suspended particulate matter and CDOM in Liaohe River watershed, northeast China. Environ. Sci. pollut. Res. 24, 19322–19337. doi: 10.1007/s11356-017-9480-9
Shi Y., Liu D. (2018). Excessive urbanization and rejuvenation in the metropolitan city center in Shanghai, China. J. Urban Plan. Dev. 144, 05018020. doi: 10.1061/(ASCE)UP.1943-5444.0000486
Shi W., Wang M. H. (2012). Satellite views of the Bohai sea, yellow sea, and East China sea. Prog. Oceanogr. 104, 30–45. doi: 10.1016/j.pocean.2012.05.001
Su M., Yao P., Wang Z. B., Zhang C. K., Stive M. J. F. (2017). Exploratory morphodynamic modeling of the evolution of the Jiangsu coast, China, since 1855: Contributions of old Yellow River-derived sediment. Mar. Geol. 390, 306–320. doi: 10.1016/j.margeo.2016.10.013
Subash N., Singh S. S., Priya N. (2011). Variability of rainfall and effective onset and length of the monsoon season over a sub-humid climatic environment. Atmos. Res. 99, 479–487. doi: 10.1016/j.atmosres.2010.11.020
Wang S., Fu B., Piao S., Lü Y., Ciais P., Feng X., et al. (2016). Reduced sediment transport in the Yellow River due to anthropogenic changes. Nat. Geosci. 9, 38–41. doi: 10.1038/ngeo2602
Wang C., Li E., Zhang L., Wei H., Zhang L., Wang Z. (2023a). Long-term succession characteristics and driving factors of zooplankton communities in a typical subtropical shallow lake, central China. Environ. Sci. pollut. Res. 30, 49435–49449. doi: 10.1007/s11356-023-25782-3
Wang C., Mao Y., Zhang L., Wei H., Wang Z. (2024). Insight into environmental adaptability of antibiotic resistome from surface water to deep sediments in anthropogenic lakes by metagenomics. Water Res. 256, 121583. doi: 10.1016/j.watres.2024.121583
Wang Q., Xu H., Yin J., Du S., Liu C., Li J. (2023b). Significance of the great protection of the Yangtze River: Riverine input contributes primarily to the presence of PAHs and HMs in its estuary and the adjacent sea. Mar. pollut. Bull. 186, 114366. doi: 10.1016/j.marpolbul.2022.114366
Wu S. L., Tao S. Q., Ye X., Wang A. J., Liu Z. T., Ran C., et al. (2023). Characteristics of sedimentary organic matter in tidal estuaries: A case study from the Minjiang river estuary. Water 15, 1682. doi: 10.3390/w15091682
Xiang J., Cui T., Qing S., Liu R., Chen Y., Mu B., et al. (2023). Remote sensing retrieval of water clarity in clear oceanic to extremely turbid coastal waters from multiple spaceborne sensors. IEEE Trans. Geosci. Remote Sens. 61, 1–18. doi: 10.1109/TGRS.2023.3318590
Yamaguchi H., Kim H.-C., Son Y. B., Kim S. W., Okamura K., Kiyomoto Y., et al. (2012). Seasonal and summer interannual variations of SeaWiFS chlorophyll a in the Yellow Sea and East China Sea. Prog. Oceanogr. 105, 22–29. doi: 10.1016/j.pocean.2012.04.004
Yin Z., Li J., Liu Y., Xie Y., Zhang F., Wang S., et al. (2021). Water clarity changes in Lake Taihu over 36 years based on Landsat TM and OLI observations. Int. J. Appl. Earth Obs. Geoinform. 102, 102457. doi: 10.1016/j.jag.2021.102457
Yu X., Lee Z., Shen F., Wang M., Wei J., Jiang L., et al. (2019). An empirical algorithm to seamlessly retrieve the concentration of suspended particulate matter from water color across ocean to turbid river mouths. Remote Sens. Environ. 235, 111491. doi: 10.1016/j.rse.2019.111491
Zhan J., Zhang D. J., Zhang G. Y., Wang C. X., Zhou G. Q. (2020). Estimation of optical properties using qaa-v6 model based on modis data. Int. Arch. Photogr. Remote Sens Spat. Inf Sci. 42, 937–940. doi: 10.5194/isprs-archives-XLII-3-W10-937-2020
Zhan J., Zhang D., Zhou G., Zhang G., Cao L., Guo Q. (2021). MODIS-based research on secchi disk depth using an improved semianalytical algorithm in the yellow sea. IEEE J. Sel. Top. Appl. Earth Obs. Remote Sens. 14, 5964–5972. doi: 10.1109/JSTARS.2021.3085556
Zhang X. B., Bi S. P., Zhang Y., Yang Y., Liu S. S., Kong X. H., et al. (2016). Provenance analysis of surface sediments in the Holocene mud area of the southern coastal waters off Shandong Peninsula, China. Acta Oceanol. Sin. 35, 124–133. doi: 10.1007/s13131-016-0852-7
Zhang D., Jia G., Chen L., Jin H., Wang Z., Feng W., et al. (2022). Seasonal succession and spatial heterogeneity of the nekton community associated with environmental factors in Hangzhou Bay, China. Reg. Stud. Mar. Sci. 49, 102108. doi: 10.1016/j.rsma.2021.102108
Zhao G., Jiang W., Wang T., Chen S., Bian C. (2022). Decadal variation and regulation mechanisms of the suspended sediment concentration in the Bohai sea, China. J. Geophys. Res. Oceans 127, e2021JC017699. doi: 10.1029/2021JC017699
Zhao L., Song C., Fang C., Xu Y., Xin Z., Liu Z., et al. (2023). Spatiotemporal variation of long-term surface and vertical suspended particulate matter in the Liaohe estuary, China. Ecol. Indic. 151, 110288. doi: 10.1016/j.ecolind.2023.110288
Zheng X., Fu Z., Xi Y., Mu J., Li Y. (2014). Grey relationship analysis for the environmental factors affecting the Noctiluca scintillans density in Qinhuangdao coastal area. Prog. Fish. Sci. 35, 8–15.
Keywords: transparency, remote sensing, coastal waters, inversion, spatiotemporal variability
Citation: Cao S, Xiao F, Chen M, Wang Z, Luo J and Du Y (2024) Inversion and analysis of transparency changes in the eastern coastal waters of China from 2003 to 2023 by an improved QAA-based method. Front. Mar. Sci. 11:1503177. doi: 10.3389/fmars.2024.1503177
Received: 28 September 2024; Accepted: 22 November 2024;
Published: 06 December 2024.
Edited by:
Qingjun Song, Ministry of Natural Resources, ChinaReviewed by:
Deyong Sun, Nanjing University of Information Science and Technology, ChinaCopyright © 2024 Cao, Xiao, Chen, Wang, Luo and Du. This is an open-access article distributed under the terms of the Creative Commons Attribution License (CC BY). The use, distribution or reproduction in other forums is permitted, provided the original author(s) and the copyright owner(s) are credited and that the original publication in this journal is cited, in accordance with accepted academic practice. No use, distribution or reproduction is permitted which does not comply with these terms.
*Correspondence: Fei Xiao, eGlhb2ZAd2hpZ2cuYWMuY24=
Disclaimer: All claims expressed in this article are solely those of the authors and do not necessarily represent those of their affiliated organizations, or those of the publisher, the editors and the reviewers. Any product that may be evaluated in this article or claim that may be made by its manufacturer is not guaranteed or endorsed by the publisher.
Research integrity at Frontiers
Learn more about the work of our research integrity team to safeguard the quality of each article we publish.