- 1School of Life Sciences and Technologies, Sanquan College of Xinxiang Medical University, Xinxiang, China
- 2Experimental Teaching Center of Biology and Basic Medicine Sciences, Sanquan College of Xinxiang Medical University, Xinxiang, China
- 3Shandong Key Laboratory of Eco-Environmental Science for the Yellow River Delta, Shandong University of Aeronautics, Binzhou, Shandong, China
- 4Key Laboratory of Humid Subtropical Eco-geographical Process of Ministry of Education, College of Geographical Sciences, Fujian Normal University, Fuzhou, China
- 5State Key Laboratory of Marine Resource Utilization in South China Sea, Hainan University, Haikou, Hainan, China
- 6College of Life Sciences, Yangtze River Delta Institute of Biodiversity Conservation and Utilization, China Jiliang University, Hangzhou, China
- 7College of Geographic Science and Tourism, Xinjiang Normal University, Urumqi, China
Introduction: Archaeal communities play a crucial role in marine ecosystems, yet our understanding of their ecological and functional traits remains incomplete. This study focuses on northern Hangzhou Bay to fill gaps in knowledge regarding the biogeography and functionality of archaeal groups.
Methods: We utilized a high-throughput sequencing dataset based on the 16S rRNA gene to characterize the archaeal community, aiming to identify biogeographic patterns and assess the influence of environmental factors on community structure.
Results: The predominant phyla identified were Woesearchaeota, Thaumarchaeota, Euryarchaeota, and Crenarchaeota. Archaeal community structure in sediments showed a geographical pattern along the environmental gradient, influenced by factors such as salinity, ammonium, total phosphorus, pH, and total nitrogen content. Network analysis revealed nonrandom co-occurrence patterns, with associations changing along the salinity gradient. Additionally, this study directly proved the existence of dispersal limitation in this strongly connected marine ecological system through null model analyses. Variation in the archaeal community was attributed to both environmental constraints and stochastic processes due to dispersal limitation. Furthermore, our results revealed that the key biogeochemical functions of the archaeal community also exhibited a clear salinity gradient, the functional differences appear to be influenced by salinity, and the critical roles of archaeal diversity were highlighted.
Discussion: All these findings enhance our understanding of microbial ecology and element transformation in estuarine environments. The highlighted roles of archaeal diversity and the influence of environmental factors on community structure and function underscore the complexity of marine microbial ecosystems.
1 Introduction
Microbial remarkably affect the redox reaction and the organic remineralization, thereby promoting the biogeochemical cycle (Sørensen, 1982; Ducklow, 2000). Particularly, in a dynamic coastal water environment, as rivers bring in allochthonous substrates and particle resuspension increases, microbial activity is enhanced by such intense chemical and physical processes (Santos et al., 2014). Organic matters as well as nutrients brought about by freshwater discharge, shallow sediments, and terrestrial environment enhance the primary as well as secondary production in the water environment. These organisms and resources are exported offshore, which increases the geographic effect imposed by highly productive zones out of the coastline (Fortunato et al., 2012; Satinsky et al., 2017). Estuary spanning brackish or freshwater and the marine environment make the microbial community pattern complicated to interpret, hence the salinity gradient usually dominates the formation of microbial community pattern (Satinsky et al., 2017; Zhang et al., 2023a, b), demonstrating salinity as an essential component for influencing the microbial distribution structure (Lozupone and Knight, 2007). Nevertheless, recent research suggests that microorganisms non-randomly distribute in coastal environments are also due to the geographic distance (Xiong et al., 2014; Wang et al., 2015; Zhang et al., 2023a). Spatial distance is capable of limiting microorganism spread as well as accelerating speciation (Diniz-Filho and Telles, 2000). In coastal oceans, there is some evidence shows a strong gradient in the nearshore to offshore microbial community composition which might be shaped by distance, suggesting the unignorable influence of the stochastic process on the variation of microbial distribution (Fortunato et al., 2012; Wang et al., 2019).
The tidal movement in Hangzhou Bay and adjacent sea areas are mainly in the form of past recirculation, and the terrestrial materials carried by rivers are continuously engaged in a series of activities such as material exchange, precipitation enrichment, dilution, and diffusion as different water masses gather in Hangzhou Bay (Liu et al., 2018; Chen et al., 1999). The regions harbor a complicated hydrological environment formed by the Taiwan warm current (south) together with the Yellow Sea coastal water (north). Besides, the intertidal ecological system here is subjected to sewage discharge and pollutants from urban river runoff (Liu et al., 2001; Kuotung et al., 2009; Shi et al., 2014). More and more studies focus on the microbial ecology in these areas (Zhang and Jiao, 2007; Feng et al., 2009; Sun et al., 2015; Liu et al., 2015; Ye et al., 2016; Guo et al., 2017; Sunagawa et al., 2015). Yet, those studies paid more attention to the environmental factors affecting, which related to the marine microbial community, and were concerned less about the deeper complicated promoting factors (such as assembly processes) for the microbial community pattern from nearshore to the open ocean. Deterministic and stochastic processes were two different processes in the assembly of microbial communities, representing the niche and neutral perspectives in community ecology, separately (Hubbell, 2001; Leibold and Mcpeek, 2006). The microbial community assembly processes have been investigated in numerous habitats, and the contributions of these two processes for microbial assembly vary in different ecosystems (Jiao et al., 2019a, b; Liu et al., 2020).
A considerable proportion of the microbial in intertidal ecological system are archaea, which can account for up to 90% of the total prokaryotic population (Biddle et al., 2006). Recent advances in genome-centric metagenomics and single-cell genomics have significantly broadened our understanding of the phylogenetic diversity and genetic capabilities of archaea (Spang et al., 2015; Liu et al., 2020). These studies have revealed the functional diversity of archaea, including their roles in ammonia oxidation, methane metabolism, and the degradation of organic matter. The discovery of new archaeal phyla with unique metabolic traits continues to expand our knowledge. For instance, Nezhaarchaeota and Helarchaeota, identified in 2019, have been shown to possess the potential for anaerobic short-chain hydrocarbon cycling (Seitz et al., 2019). Despite the growing insights into the composition and metabolic capabilities of archaeal communities, there remains a considerable gap in our knowledge regarding the spatiotemporal dynamics of their distribution in sediments, the mechanisms underlying their assembly, and the interactions among different taxa. So far, we remain unclear on the balance of different assembly processes for the archaeal community in this region. Additionally, microorganisms are capable of forming a complicated interaction network in a certain ecological niche (Faust and Raes, 2012). Co-occurrence network analysis can help us to better understand the structure possessed by complicated microbial communities as well as various functional interactions among microorganisms (Barberán et al., 2012). Planktonic archaea in the ocean are key drivers of biogeochemical cycles and an important component of the microbial food web. The horizontal spatial distribution characteristics of archaea in surface seawater have also gradually attracted attention (Wang et al., 2019). However, there is still a lack of understanding regarding the spatial distribution characteristics, influencing factors, and symbiotic relationships of planktonic archaea in the Hangzhou Bay to the East China Sea.
Additionally, we know less about the functional potential of archaea in these places. In this century, eutrophication of coastal water bodies has been identified as a major environmental issue, due mainly to the widespread application of artificial N fertilizers and excessive combustion of fossil fuels (Gruber and Galloway, 2008; Kim et al., 2014; Hou et al., 2015; Lin and Lin, 2022; Liu et al., 2022). Much of the anthropogenic carbon (C), nitrogen (N), and phosphorus (P) are delivered to the estuarine and coastal areas through river flow, groundwater discharge, and atmospheric deposition (Seitzinger, 2008; Diaz and Rosenberg, 2008; Kim et al., 2014), consequently exerting a serious threat to these aquatic ecosystems. Archaeal communities mediated C, N, and P recycling processes such as nitrification occur simultaneously with the removal processes of denitrification and anaerobic ammonium oxidation (anammox) in estuarine waters (Lisa et al., 2015). Therefore, understanding the key elements removal processes and related microbial mechanisms is important for developing management strategies to protect estuary ecosystems.
In this study, 16S rRNA gene amplicon was adopted for analyzing the community structure of archaea, considering the environmental factors together with spatial factors. Then we evaluate the relative importance of species sorting and dispersal limitation in shaping sediment microbial communities across different salinity regions in estuary ecosystems. The null model was used to discern the relative importance of niche and neutral processes that structure community composition. Additionally, the co-occurrence patterns exhibited by archaeal taxa in coastal water systems were also explored. Meanwhile, to enhance our understanding of the biogeochemical functions of archaea, especially the translocation processes of C, N, and P elements in coastal ecosystems, functional prediction was utilized to uncover the key elemental metabolic pathways along the estuarine salinity gradient.
2 Materials and methods
2.1 Field sampling and environmental characteristics
In June 2022, 22 stations were selected from inshore to ocean near the northern Hangzhou Bay (Figure 1). Surface water samples at 0.5 m depth were collected at each site. Approximately 500 ml of water sample from each site was filtered onto a 0.2 μm polycarbonate membrane (Millipore, USA), and immediately stored in a tank filled with liquid nitrogen before DNA extraction. We imported the global positioning system (GPS) coordinates that were recorded at every sampling point into the NOAA website (http://www.nhc.noaa.gov/gccalc.shtml) for calculating the pairwise geographic distance between samples.
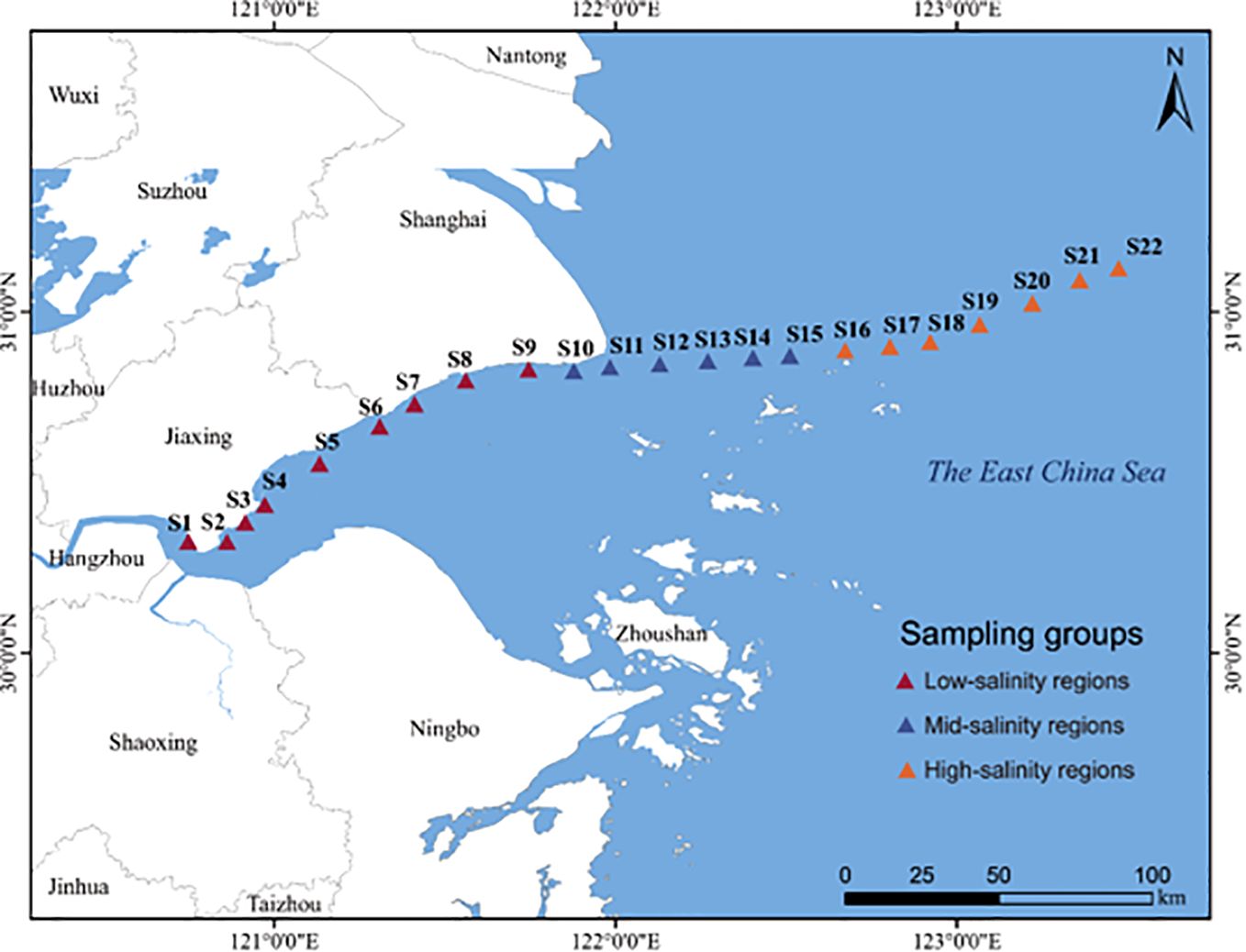
Figure 1. Study area. The figure shows the location of the Northern Hangzhou Bay and the sampling sites during field investigations.
Table 1 lists the water chemical parameters, including salinity, pH, exchangeable nitrite (NO2–N), nitrate (NO3–N), ammonium (NH4+-N), Fe2+ and Fe3+ concentration, total organic carbon (TOC), total phosphorus (TP), total nitrogen (TN) and Sulfide concentration. A portable water quality analyzer (HQ 40d, HACH, USA) assisted in measuring the pH and salinity. The continuous-flow injection analysis was used to measure the NH4+, NO3-, and NO2- concentration (Skalar Analytical SAN+ +, Netherlands) (Hu et al., 2017; Lin and Lin, 2022). Measurement of Fe2+ and Fe3+ concentration followed the previous literature (Zheng et al., 2019) together with their references (Roden and Lovley, 1993). The methylene blue spectrophotometric method assisted in analyzing the sample sulfide (Cline, 1969). Vario EL CN Elemental Analyzer (Elementar, Germany) assisted in measuring the TOC and TN (Zhang et al., 2015). And the ascorbic acid-molybdate blue method for assaying TP (Hou et al., 2013). All of these physiochemical parameters were in triplicate.
2.2 DNA extraction and gene amplification
Plankton-archaea microorganisms were collected by filtering about 500 mL of water samples with a 0.2µm pore size polycarbonate membrane (Millipore, USA). Power Soil DNA Isolation Kit (MO BIO, USA) assisted in extracting community DNA from polycarbonate membranes in line with the protocol of the manufacturer. The primer set 524F/958R was used for archaeal 16S rRNA gene amplification (Shen et al., 2017), modifying the forward primer to contain a unique 8 nt barcode at the 5’ end. Gene amplification was conducted in a 20-μL reaction system containing 4 μL of FastPfu Buffer (5×), 2 μL of dNTP mix (2.5 mM), 0.8 μL of each primer (5 μM), 0.4 μL of Fastpfu polymerase, 10 ng of template DNA, and 0.2 μL of BSA. The PCR parameters were 96°C for 5 min, followed by 35 cycles of 96°C for 35 s, 56°C for 30 s, and 72°C for 40 s, with a final extension at 72°C for 5 min. We employed electrophoresis to identify the PCR products, which were located in the distinct band range of 400-450 base pairs (bp), within a 2% (w/v) agarose gel. These PCR products were then combined in equal proportions and subjected to purification using the GeneJET Gel Extraction Kit from Thermo Scientific (Jiao et al., 2016). Subsequently, four replicate PCR products derived from a single DNA sample were consolidated and purified using a DNA gel extraction kit from Axygen, USA, following the manufacturer’s guidelines. The concentration and purity of the extracted DNA were ascertained utilizing a NanoDrop spectrophotometer. Subsequently, we mixed three parallel PCR products in each subsample to sequence on an Illumina platform.
2.3 Sequence analysis, null model, and network analysis
FLASH (V1.2.7, http://ccb.jhu.edu/software/FLASH/) assisted in merging the paired-end reads, and the quality of reads was filtered based on the literature (Caporaso et al., 2011). USEARCH software was applied to the detection and removal of acquired sequences following the UCHIME algorithm (Edgar et al., 2011). These sequences had their barcodes during the assignation to each sample. UPARSE software package was applied to the sequence analysis by the UPARSE-OTU algorithm. We assigned sequences with a similarity of 97% to the same OTU. The RDP classifier assisted in assigning the sequences representing each OTU to the taxonomic groups (Caporaso et al., 2011).
Null model analysis was carried out using the framework described by Stegen et al. (2013), to classify community pairs into underlying drivers of deterministic process and stochastic process based on the β-nearest taxon index (βNTI). Deterministic processes including heterogeneous selection (βNTI<−2) and homogeneous selection (βNTI> 2), and the stochastic processes including homogenizing dispersal (mass effect), dispersal limitation, and drift were distinguished by the Raup-Crick metric (RCbray) as described by Liu et al. (2020). The null model expectation was generated using 999 randomizations. Network assisted in exploring the co-occurrence pattern exhibited by microbial taxa, selecting OTUs with top 500 relative abundances. A Spearman’s correlation between 2 OTUs presented statistical robustness with a coefficient over 0.6 and a P-value less than 0.01 (Barberán et al., 2012; Jiao et al., 2016). The interactive platform Gephi assisted in visualizing the network (Bastian et al., 2009).
2.4 Data analyses
QIIME (http://qiime.org/index.html) was employed for calculating the Alpha and Beta diversity, and the minimum sequence number used for normalizing the sequencing depth difference. The potential for the cycling of C, N, and P elements within microbial communities was forecasted based on the composition of the 16S rRNA gene library, using the FAPROTAX software version 1.2.1. Differences in environmental variations, community diversity, abundances, and functional profiles among microbial groups were compared via the Kruskal-Wallis H test and One-way analysis of variance (ANOVA), respectively. We confirmed the relation of geographical coordinates or the environmental factor (the Euclid distance based on environmental factors) with the sample ordination on the taxonomic (Bray-Curtis). The Mantel test assisted in checking the effect of environmental and spatial dissimilarity on taxonomy. Nonmetric multidimensional scaling (NMDS) was used to evaluate the structure and function differences of microbial communities (Caporaso et al., 2010; Legendre and Legendre, 1998).
Two-way analysis assisted in comparing the spatial variations of environmental variables. Based on the values of lengths of gradient, we applied to the redundancy analysis (RDA) examining the relationships between microbial taxonomic and functional structure and environmental indices in Canoco (version 4.5) software, respectively (Danovaro and Gambi, 2002). The linear trend together with the principle coordinates of neighbor matrices (PCNM) procedure assisted in deriving the spatial variable from the geographic coordinates (Griffith and Peresneto, 2006), thereby minimizing the association of spatial distance with environmental factors, and capturing all spatial scales detected in the dataset. Then adjusted R2 was used in the analysis of variation partitioning, to determine the different effects of spatial factor and environmental factors on the microbial community variation, as well as the combined effect of the two factors.
Except as described above, the rest of the data analysis processing is done in R environment (http://www.r-project.org). The raw reads of high-throughput sequences have been deposited in the NCBI SRA database.
3 Results
3.1 Geochemical characteristics of waters
There was an obvious salinity gradient along the transect of samples (Table 1). The salinity distribution was considered to divide these study sites into high-salinity regions (22.60–35.50 ppt, S16 to S22), mid-salinity regions (11.30–19.70 ppt, S10 to S15), and low-salinity regions (1.60-8.90 ppt, S1 to S9). All samples were alkalescent and pH ranged from 8.00 to 8.73. Water nutrients NH4+ showed significant variations among three salinity gradients (two-way ANOVA, P = 0.02). The values of Fe2+ and Fe3+ were highest at low-salinity regions (two-way ANOVA, P < 0.05). Concentrations of water sulfide also showed substantial variability across the salinity zones (two-way ANOVA, P < 0.001), with the highest levels observed in the high-salinity areas. Nevertheless, the variations in other water environmental parameters did not reach statistical significance, implying that the observed changes in water geochemical characteristics are likely due to specific biogeochemical processes rather than water salinity shifts.
3.2 Diversity and richness of planktonic archaeal communities
The Miseq sequencing platform assisted in identifying the structure of the archaeal community regarding all water samples. We obtained a data set containing 1551070 quality sequences from the 22 samples. And the total OTU number was 7456 defined by 97% sequence similarity. Table 2 lists the archaeal community abundance and diversity in sediments along the salinity gradient from inshore to ocean near northern Hangzhou Bay. All of the coverage of the archaeal sequencing library reached 100%, indicating that the primers used in the study effectively reflect the diversity of archaeal populations in the samples. The Shannon indices were in the range of 0.130 to 3.714, and the highest values were seen at mid-salinity sites S14. Chao 1 and ACE indices ranged from 253.240 to 1089.500 and 262.860 to 1683.239, respectively. Moreover, the highest values of these two richness indices appear at mid-salinity sites S10. According to the results of the alpha diversities index, although the difference was not significant, archaeal communities at high-salinity regions presented relatively higher diversity, with the average values of the Shannon index in high-, middle- and low-salinity regions were 1.740, 1.040, and 1.470, respectively. However, the richness was highest in high-salinity regions. The average values of Chao 1 and ACE indexes are the highest in the mid-salinity regions, which are 665.909 and 875.850 respectively (one-way ANOVA, P < 0.05).
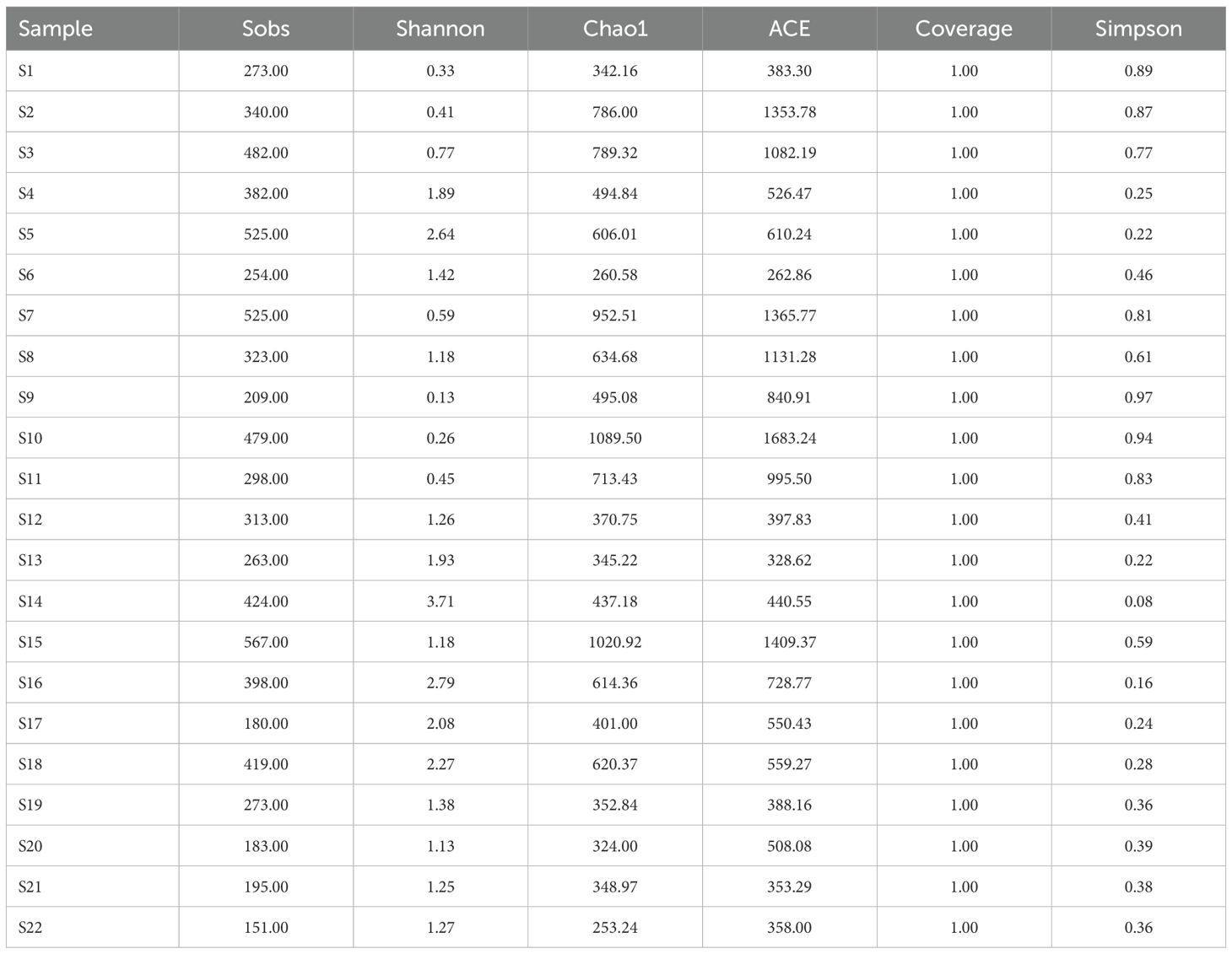
Table 2. Diversity characteristics of microbial communities from Northern Hangzhou Bay to the East China Sea.
3.3 Archaeal geographic patterns along the salinity gradient
Most sequences (99.84%) were included in the phyla of Woesearchaeota, Thaumarchaeota, Euryarchaeota, and Crenarchaeota (relative abundance > 2%), occupying 99.28% of the total sequences (Figure 2A). As revealed by NMDS analysis, the planktonic archaeal community distribution exhibited a distinct geographical specificity along the salinity gradient. According to the first two axes of NMDS, there were 3 groups (low-, middle- and high-salinity regions) of microbial assemblages along the salinity gradient (Figure 2B). An analysis of similarity made a further conformation on these patterns, ANOSIM result indicating the vital significance of sampling sites for determining the community composition (the mantel test, R = 0.792, P < 0.001). Based on all these results, archaeal composition presented a dynamic spatial pattern along the salinity gradient.
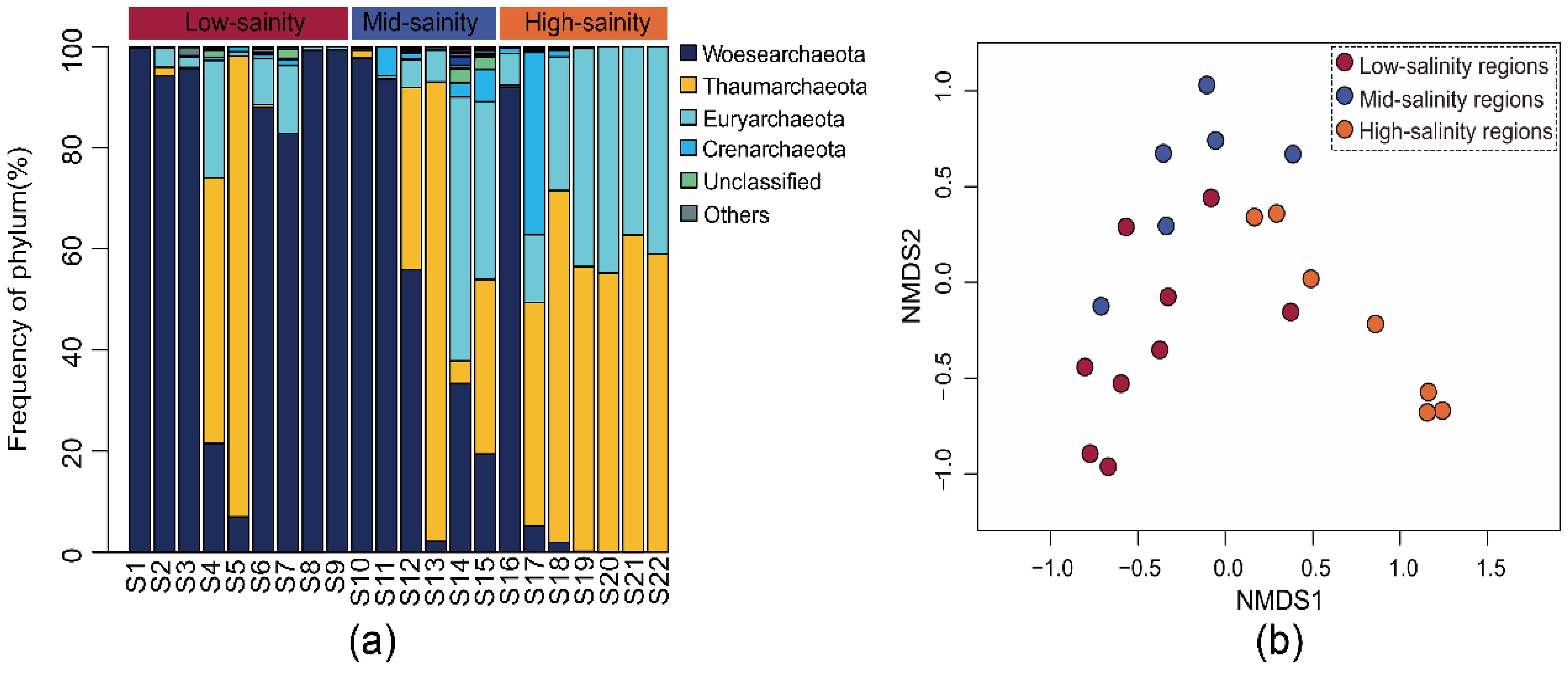
Figure 2. The (A) community composition in Phylum-level and (B) nonmetric multidimensional scaling (NMDS) analysis of the archaeal community revealed by the 16S rRNA gene sequences.
Figure 3 displays the impact of environmental factors on the structure of archaeal communities along various salinity gradients. The clusters based on the water environment factor in the right diagram showed a similar cluster to the microbial community structure in the left diagram, confirming the obvious association between them (the mantel test, R = 0.792, P < 0.001) (Figure 3A). RDA analysis assisted in exploring the effect of environmental factors on archaeal communities (Figure 3B). The first 2 RDA axes accounted for 43.96% of the cumulative variation regarding the relation between them. In which, microbial community distribution and structure were affected by salinity (P = 0.001), NH4+ (P = 0.003), TP concentration (P = 0.008), pH (P = 0.009), TN (P = 0.011) as well as spatial factors (PCNM 1 and PCNM 2, P < 0.05). The variance partitioning analysis (VPA) was further used to quantify the effect of environmental and spatial factors on the variation of the archaeal community. The effect of the two factors accounted for 38.66% of the community variation. The pure effect of environmental factors explained 12.11% of the variation. While the PCNM purely explained 6.62% of the variation. Also, the combined effect of PCNM and environmental variables accounted for 19.93% of the variation, demonstrating the mutual dependence of the two factors.
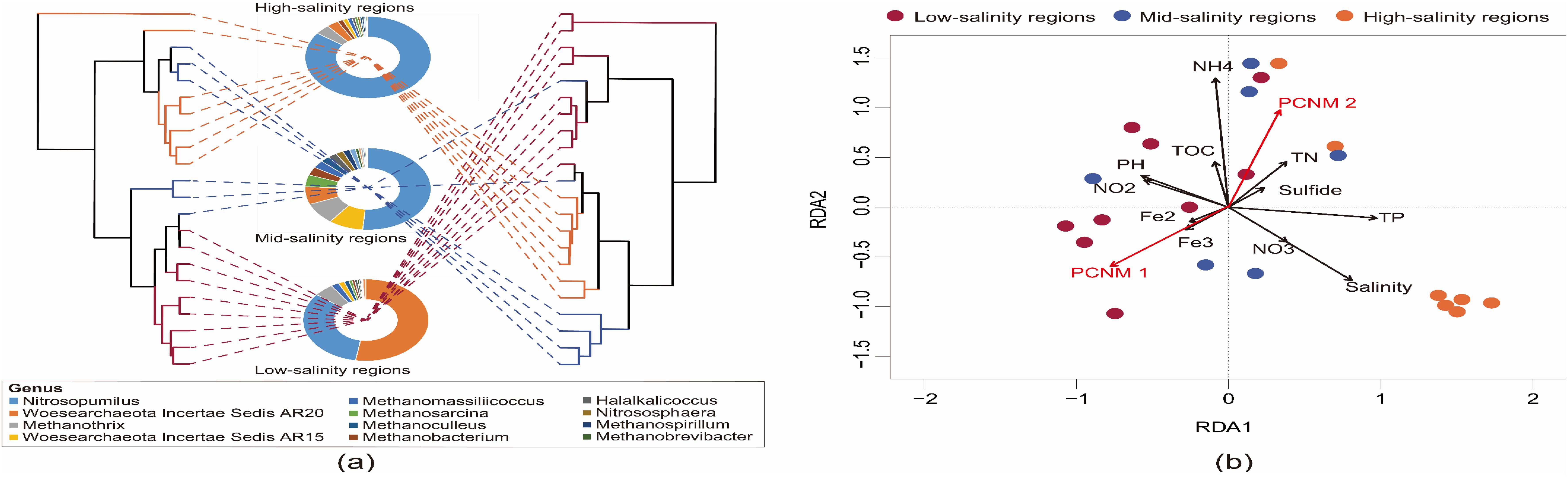
Figure 3. Dendrogram (A) of BrayeCurtis distance based on archaeal communities and Euclid distance based on environmental factors, branches are colored by the sample sites that they represent; Sunburst charts showing the hierarchical taxonomic composition of a salinity group. The colors are assigned automatically to distinguish taxa. (B) The redundancy analysis (RDA) biplot of the distribution of bacterial communities with environmental factors. PH, Fe2, Fe3, NO2, NO3 and NH4 represent pH, Fe2+, Fe3+, NO2–N, NO3–N and NH4+-N, respectively.
3.4 Ecological assembly process and planktonic archaeal network
To explore community assembly mechanisms under the observed geographic pattern, the relative roles of niche and neutral processes in community assembly were analyzed. Across all samples, stochastic processes (-2< βNTI <2) explained a slightly higher proportion than deterministic processes (2< |βNTI|) of the archaeal community variation, and the balance of these ecological processes varied greatly across the salinity gradient (Figure 4A). Our result shows that the deterministic processes explained a lower proportion (16.67%) of the archaeal community variation in middle-salinity regions than in the low- and high-salinity regions (34.57% and 36.74%, respectively). In addition, the effect of homogenous selection (βNTI> 2) on community assembly in the middle-salinity areas was 0%. For the stochastic processes, the result showed that drift was the most important process, accounting for 33.33%, 50.00%, and 34.69% of the community variation in high-, middle- and low-salinity regions, respectively. Then was followed by dispersal limitation, which respectively explained 9.88%, 27.78%, and 24.49% of the community variation in high-, middle- and low-salinity regions (Figure 4B).
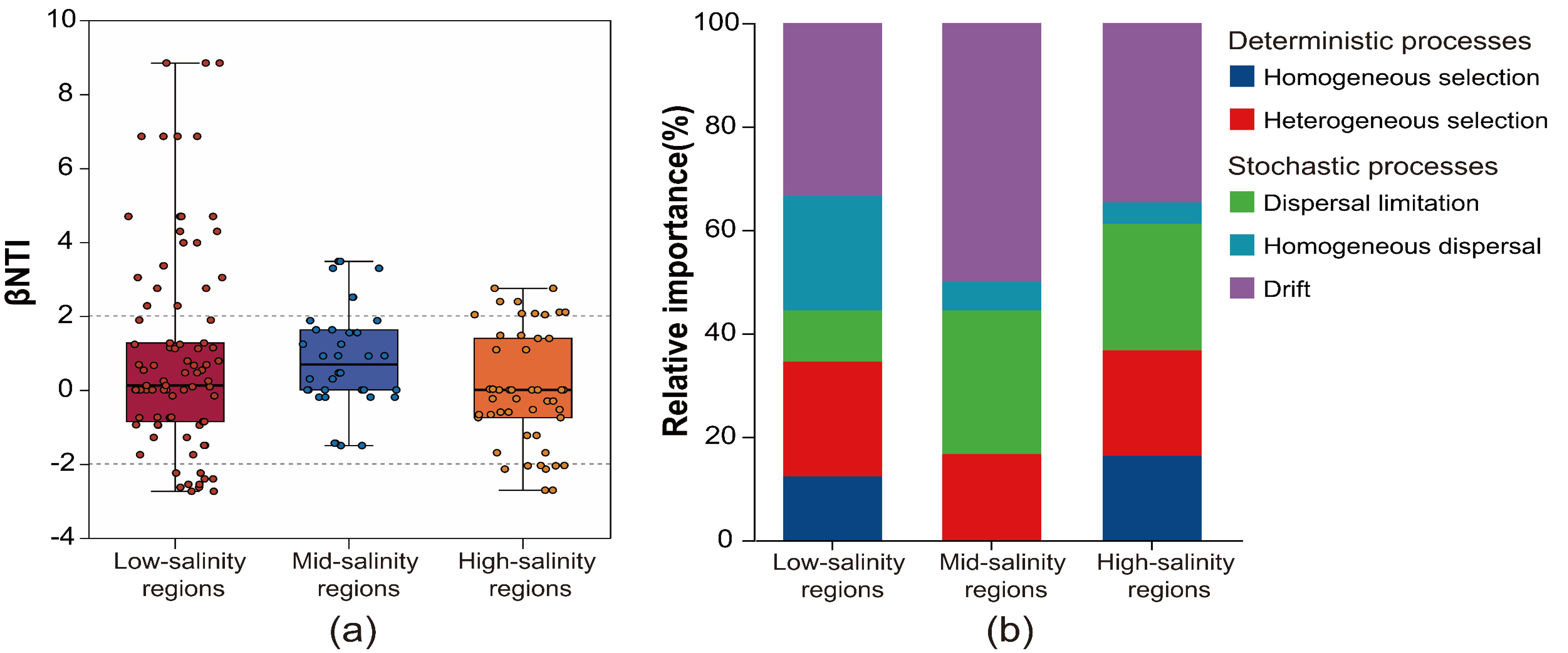
Figure 4. The (A) β-nearest taxon index (βNTI) revealing the assembly mechanism of the archaeal community in salinity gradient. And (B) the percent of determinism and stochasticity process based on null modelus.
The co-occurrence network results indicate that compared to areas with low and medium salinity, the archaeal co-occurrence networks in high salinity areas have significantly higher avgK and avgCC (Figure 5A). The results of the node-level topological properties of the co-occurrence network show that archaeal communities have a significantly higher node degree, node CC, and degree centrality indices, as well as a notably lower betweenness centrality index in high salinity areas (Figure 5B), suggesting that the archaeal community in high salinity areas has more complex interaction relationships. Furthermore, our results show that from areas with low salinity to those with high salinity, there is a clear gradient change in the network-level properties (such as avgK and avgCC), node-level properties (such as node degree, node CC, and betweenness centrality), and modularity of the archaeal symbiotic network, implying a key regulatory role of water salinity on the co-occur relationships of archaeal communities.
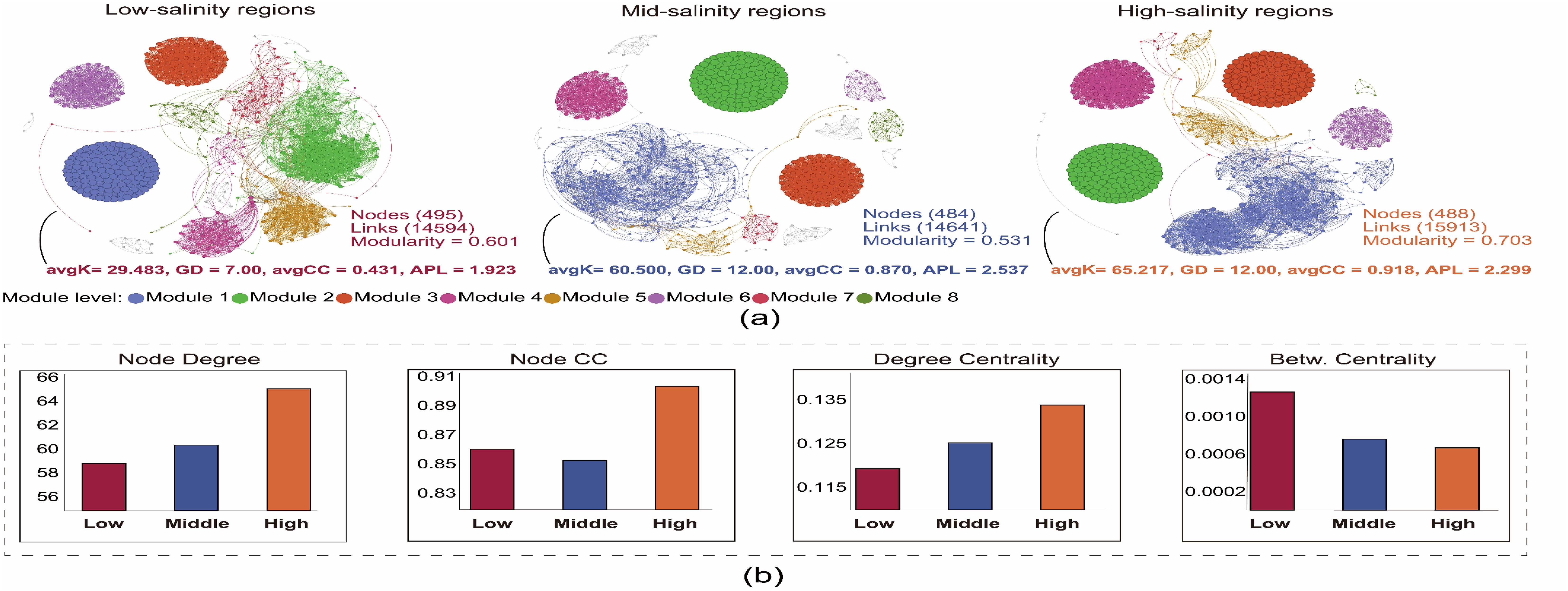
Figure 5. The (A) network of co-occurring OTUs based on correlation analysis. A connection stands for a strong (Spearman’s r > 0.6) and significant (P < 0.01) correlation. The size of each node is proportional to the relative abundance; the thickness of each connection between two nodes (edge) is proportional to the value of Spearman’s correlation coefficients. (B) Node-level topological properties among three salinity groups, Low: Low-salinity regions; Middle: Middle-salinity regions; High: High-salinity regions.
3.5 Potential metabolic functions of planktonic archaeal communities
The prediction results from FAPROTAX indicate that the main metabolic mode of the archaeal community in the study area is aerobic ammonia oxidation, followed by nitrification, methanogenesis, and hydrogenotrophic methanogenesis. Both aerobic ammonia oxidation and nitrification processes are predicted to be present in all samples, suggesting a key impact of archaeal communities on the biogeochemical nitrogen cycle (Figure 6A). Additionally, microbial groups involved in methanol oxidation, anammox, nitrogen fixation, aromatic compound degradation, and iron respiration have been detected (Figure 6A). Beyond these, enzymatic processes such as chitinolysis, cellulolysis, xylanolysis, and ureolysis are also present (Figure 6A; Supplementary Table S1). This indicates that the archaeal communities in the study area are involved in a variety of biogeochemical cycles or metabolic processes of organic and inorganic substances. Among the nutritional types, chemoheterotrophy is the dominant type of microorganism, with high abundance in all three salinity zones and peaking in the medium salinity area; photoautotrophs and photoheterotrophs are also distributed, mainly in the medium salinity area (Figure 6A; Supplementary Table S1). Furthermore, from low to high salinity areas, functions such as aerobic ammonia oxidation and nitrification significantly increase, while functions like Methylotrophy, Methanogenesis by reduction of methyl compounds with H2, and Aerobic chemoheterotrophy show a significant decrease (Figure 6B).
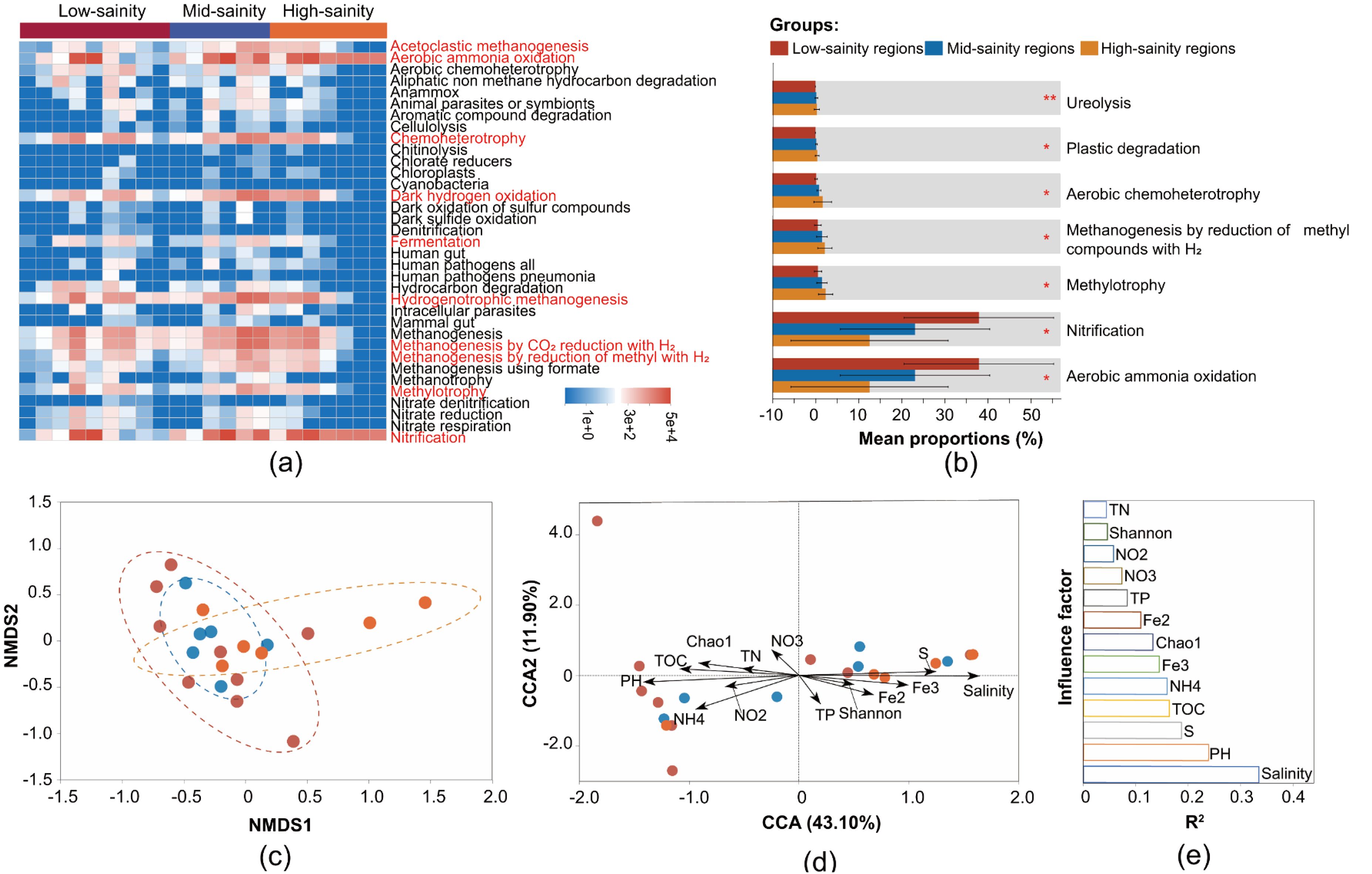
Figure 6. Genetic potential for the metabolic functions in different salinity sediment. The (A) relative abundance and (B) composition of metabolic functions among three salinity groups. The (C) nonmetric multidimensional scaling (NMDS) analysis and (D) redundancy analysis (RDA) of the distribution of archaeal founctional characteircs with environmental and microbiological factors, and the (E) rank the importance of each influence factor. PH, Fe2, Fe3, NO2, NO3 and NH4 represent pH, Fe2+, Fe3+, NO2–N, NO3–N and NH4+-N, respectively.
The NMDS results indicate that the functional characteristics of the archaeal community do not show significant variation across the salinity gradient. (ANOSIM, R = 0.012, P = 0.241) (Figure 6C), CCA was used to explore environmental and biological effects on the observed functional pattern (Figure 6D). The CCA result showed that the environmental factors including salinity and pH were the most powerful predictors separating functional patterns along the salinity gradients (P < 0.05) (Figure 6E). In addition, according to fitting analyses, significant correlations were confirmed between salinity and functional β diversity (P < 0.001, R2 = 0.34), as well as microbial richness (Chao 1 index) and functional β diversity (P = 0.029, R2 = 0.28).
4 Discussion
This study focuses on discussing the characterization of the archaeal community, and the functional profiles of key element cycles along the salinity gradient from the Qiantang River to the adjacent marine areas along the salinity gradient. Additionally, this study pays attention to the microbial interaction and assembly process in water ecological systems with strong connectedness.
4.1 Factors influencing planktonic archaeal community structure
The archaeal communities were under the dominance of the phyla of Woesearchaeota, Thaumarchaeota, Euryarchaeota, and Crenarchaeota. This pattern presents a similarity to the patterns of other marine waters in China. It has been reported the temporal distribution of archaeal communities in the Changjiang Estuary and the adjacent East China Sea was examined, revealing that Euryarchaeota and Crenarchaeota were the predominant groups, with salinity identified as a key factor influencing their distribution (Liu et al., 2011). Our findings align with their observation of salinity’s impact; However, we also detected a more diverse range of archaeal phyla, suggesting a broader response of archaeal communities to the complex interplay of environmental factors in our study area.
It is noteworthy that the high abundance of Woesearchaeota in our study is a striking finding that contrasts with some previous reports. However, recent research has begun to uncover the ecological preferences and adaptive strategies of this archaeal group, which may explain their success in specific environments (Liu et al., 2021). In the context of our study, the high salinity and nutrient-rich conditions of the coastal waters, influenced by the estuarine environment, may favor the proliferation of Woesearchaeota. Similar findings have been reported, such as in the Qiantang River estuaries where Woesearchaeota were found to be dominant, suggesting that the dynamic physicochemical characteristics of the estuarine mixing zone may provide a suitable habitat for this group (Liu et al., 2020). Another possible explanation is that Woesearchaeota widely distributed in terrestrial ecosystems (Wei et al., 2016). The runoff from rivers may carry a large number of Woesearchaeota cells/species into the estuarine water bodies, promoting the high enrichment of this species. Thaumarchaeota and Euryarchaeota dominate in marine water bodies far from the estuary, where the sources of chemical energy differ from those in estuarine water bodies, such as the lack of available organic matter, which may lead to the adaptation and dominance of specific microbial groups. Thaumarchaeota and Euryarchaeota can utilize dissolved organic carbon through chemosynthesis or obtain energy through ammonia oxidation, ensuring their survival and proliferation in the marine environment, thereby occupying a dominant position in marine water bodies far from the estuary. Furthermore, Thaumarchaeota plays an important role in the marine nitrogen cycle, capable of performing ammonia oxidation in the deep waters of the ocean. The distribution pattern of Thaumarchaeota in the deep sea in this study is consistent with previous studies (Baker et al., 2020), indicating that they play a significant role in the biogeochemical cycles of the deep sea. Additionally, we found that Thaumarchaeota is also abundant in some water bodies from the estuarine area, which may be related to the high nitrogen load in the estuarine region.
From the Northern Hangzhou Bay to the East China Sea, the spatial differentiation exhibited by samples presented an obvious correlation with the salinity, and it seems that these factors pose a dramatic impact on archaeal community composition. As shown in several studies of coastal zone, salinity mainly affects the microbial diversity variation (Fortunato et al., 2012; Wang et al., 2015; Liu et al., 2018). Salinity, as an environmental factor, mainly determines the community composition in soil, sediment, freshwater, and marine, and exerts a more important effect compared with pH, temperature, etc (Lozupone and Knight, 2007; Liu et al., 2015). Besides, regarding the coastal environment, salinity is likely to cause a density gradient that separates the residential microbial community in water masses (Fortunato et al., 2012). Here, we also found the importance of nutrient-related variables (primarily TN, TP, and NH4+) and pH also affected the archaeal community. Nutrients remarkably affect the composition and growth of bacteria in the environment, including nitrogen, carbon, and iron in the bioavailable form, which may be fully utilized by microorganisms and closely related to the composition of the archaeal community (He et al., 2016). In conclusion, a deterministic process (environment filtering) assisted in shaping the archaeal community from Hangzhou bay to the adjacent sea.
4.2 Ecological processes governing the planktonic archaeal communities’ assembly
In river estuary and its adjacent areas, water flows together with tidal activity mainly dominate the microbiota spread as well as the microbial transportation to the benthic zone (Augspurger et al., 2010). Therefore, because the dispersal rate (mass effect) is high, the archaeal composition may be randomly distributed within this highly connective ecological system. On that account, geographic distance may slightly affect the taxon diversification in the zone. Nonetheless, based on the null model, a stronger role of stochastic processes rather than deterministic processes in governing the archaeal community assembly was detected. In the middle-salinity samples, the null model revealed that stochastic processes were overwhelming. In contrast in low- and high-salinity sites, the influence of stochastic processes was weak. This was consistent with the result of CCA, where the spacial factor (PCNM) plays a more important role in structuring the microbial community in middle-salinity sites than in other regions. A potential explanation is that middle-salinity regions have lower regional connectivity, Due to the interactive effects between rivers and oceans (Zinger et al., 2014), in the present study, the water bodies in the medium-salinity area exhibit less restricted diffusion and mixed archaeal communities from both rivers and oceans, allowing the stochastic process to dominate the community assembly.
Here we found that the stochasticity of the archaeal community was mainly controlled by dispersal limitation and drift. The result is consistent with Wu and Huang (2019) reported that dispersal limitation and drift accounted for ~50% of the protist community turnover in the South China Sea and homogeneous dispersal explained 0%. In addition, these indicators have obvious dynamic changes along the salinity gradient. The influences of dispersal limitation had the lowest proportion in low salinity sites, this might be due to the strong runoff effect of the Qiantang River, allowing archaeal communities to disperse randomly. Therefore, environmental gradients (such as salinity gradient) and environmental conditions (such as hydrological conditions) might determine the balance of determinism and stochasticity, and thus construct the geographical pattern of archaeal community structure from Northern Hangzhou Bay to the East China Sea.
A microbial community network sees the occurrence of complicated interactions, which could be reflected in the co-occurrence networks to some extent (Faust and Raes, 2012; Liu et al., 2019). In this study, the archaeal communities exhibited a connectedness as well as a non-random co-occurrence. In addition, the network structure was significantly subjected to salinity, which further demonstrated the impact of the salinity (or other environmental factors of water) on the community structure, possibly because of the co-occurrence of dominant taxa with other taxa which had the same requirement for habitat (Delgado-Baquerizo et al., 2018). Functions possessed by nodes in different modules were different (Newman, 2006), and taxas strongly linked in ecology could cluster as one module. The network characteristics of archaeal communities within the same module exhibit noticeable variations across different salinity zones, thus, our results suggest that community compositions and ecological functions might be interconnected along the salinity gradient of the water. Also, it is necessary to pay attention to the case that the estimated co-occurrence pattern variation obtained by using a system method based on topology was incapable of reflecting the actual correlation among taxa (Ma et al., 2016).
4.3 Functional potential of planktonic archaeal community
We further reconstructed microbial biogeochemical processes in this salinity gradient region based on the FAPROTAX predictions. The ubiquity of aerobic ammonia oxidation and nitrification highlights the central role of archaea in the nitrogen cycle. This finding is consistent with previous studies on the importance of archaea in nitrogen transformation processes, further emphasizing their role in the global nitrogen cycle (Baker et al., 2020). Particularly in the medium salinity zone, the abundance of chemoheterotrophic microorganisms reaches the highest, which may be related to the specific environmental conditions of this area (such as salinity, temperature, and the availability of organic matter) as well as the ecological characteristics of the archaeal community (such as a lower deterministic process). This diversity and abundance distribution of trophic types may have profound effects on the function and stability of the ecosystem, as they affect energy flow and material cycling (Escalas et al., 2019; Lu et al., 2023). Moreover, from low to high salinity regions, the enhancement of aerobic ammonia oxidation and nitrification functions may be related to the increase in environmental stress, such as the rise in salinity that may promote the microbial adaptability of these processes. In contrast, the reduction of methylotrophy and methanogenesis may reflect the decreased energy efficiency of these metabolic pathways under high salinity conditions. This trend is significant for understanding the adaptive strategies of microbial communities in extreme environments. It is also worth noting that the detection of various enzymatic processes, such as chitinolysis, cellulolysis, and xylanolysis, indicates that archaeal communities play a key role in the decomposition of complex organic matter. These processes are crucial for maintaining the ecosystem’s carbon cycle and may respond to global climate change.
In summary, this study provides an in-depth understanding of the metabolic patterns of archaeal communities in the study area and emphasizes their key role in biogeochemical cycles. These findings lay the foundation for further exploration of the functions of archaea in ecosystem services and their responses to environmental changes. Additionally, in this study, spatial gradients were not found in archaeal community functional characteristics. This suggested that the geochemical functions of the archaeal communities are not influenced by the gradients of environmental factors in water. Furthermore, considering that nitrogen cycling pathways in sediments are mediated by a series of microorganisms occupying different taxonomic levels (Marcel et al., 2018), thus the taxonomic characteristics change might affect the potential of nitrogen cycling functions. Here, our results showed that the archaeal diversity characteristics (e.g. Chao 1 index) affect the spatial pattern of functional characteristics, except with the environmental factors. Suggested the unneglectable influences of microorganisms in the key element transformation in marine ecosystems. Furthermore, although further research is needed, we suggest that the balance of niche and neutral processes within microbial communities might influence their functional characteristics.
To sum up, based on the study results, the spatial distribution exhibited by archaeal community and function is subject to the direct control of environmental factors and the indirect control of the stochastic genetic drift and dispersal limitation. Future study is suggested to conduct sampling in time series and at least in the seasonal scale, for predicting dynamic archaeal assembly patterns on the time scale. It is necessary to integrate more detailed physiochemical information as well as hydrologic conditions with the functional information and taxonomy of microbe, for better characterizing the biogeographic community distribution as well as the functional structure. Although the predicted microbial function based on 16S rRNA do not reflect true abundance and are not always expressed in proportion, the annotated gene abundance seems to demonstrate the potential biogeochemical process instead of the microbiome-mediated actual biogeochemical process. Multi-omic profiling is needed to gain holistic insights into the genetic potential and main elements (eg: nitrogen, sulfur, and carbon) metabolic coupling of marine water microbial communities in future research. Anyway, our study provides an overall insight into archaeal diversity and functionality in this coastal ecosystem. This might provide essential basic data for the protection and restoration of estuarine and seawater ecosystems.
Data availability statement
The datasets presented in this study can be found in online repositories. The names of the repository/repositories and accession number(s) can be found below: https://www.ncbi.nlm.nih.gov/bioproject/PRJNA1159009.
Author contributions
CT: Writing – original draft. XH: Writing – original draft, Writing – review & editing. HZ: Data curation, Methodology, Writing – original draft. CL: Investigation, Software, Writing – review & editing. DG: Supervision, Writing – review & editing. YC: Supervision, Writing – review & editing. SZ: Supervision, Writing – review & editing. WZ: Writing – review & editing. ZZ: Validation, Writing – original draft.
Funding
The author(s) declare financial support was received for the research, authorship, and/or publication of this article. This work was jointly supported by Natural Science Foundation of China (42206237 and 32401322), Shandong Provincial Natural Science Foundation (ZR2023QD103), and the Guangdong Basic and Applied Basic Research Foundation (2023A1515110368). It was also funded by Backbone Teachers Program of Sanquan College of Xinxiang Medical University (SQ2023GGJS03), and the Excellent Young Teachers Training Program of Sanquan College of Xinxiang Medical University (SQ2022YQJH12).
Conflict of interest
The handling editor XL declared a past co-authorship with the author CL.
The remaining authors declare that the research was conducted in the absence of any commercial or financial relationships that could be construed as a potential conflict of interest.
Publisher’s note
All claims expressed in this article are solely those of the authors and do not necessarily represent those of their affiliated organizations, or those of the publisher, the editors and the reviewers. Any product that may be evaluated in this article, or claim that may be made by its manufacturer, is not guaranteed or endorsed by the publisher.
Supplementary material
The Supplementary Material for this article can be found online at: https://www.frontiersin.org/articles/10.3389/fmars.2024.1469717/full#supplementary-material
References
Augspurger C., Karwautz C., Musmann M., Daims H., Battin T. J. (2010). Drivers of bacterial colonization patterns in stream biofilms. FEMS Microbiol. Ecol. 72, 47–57. doi: 10.1111/j.1574-6941.2009.00830.x
Baker B. J., Anda V. D., Seita K. W., Dombrowski N., Santoro A. E., Lloyd K. G. (2020). Diversity, ecology and evolution of Archaea. Nat. Microbiol. 5, 887–900. doi: 10.1038/s41564-020-0715-z
Barberán A., Bates S. T., Casamayor E. O., Fierer N. (2012). Using network analysis to explore co-occurrence patterns in soil microbial communities. ISME J. 6, 343–351. doi: 10.1038/ismej.2011.119
Bastian M., Heymann S., Jacomy M. (2009). Gephi: an open source software for exploring and manipulating networks. ICWSM. 3, 361–362. doi: 10.1609/icwsm.v3i1.13937
Biddle J. F., Lipp J. S., Lever M. A., Lloyd K. G., Sørensen K. B., Anderson R., et al. (2006). Heterotrophic Archaea dominate sedimentary subsurface ecosystems off Peru. Proc. Natl. Acad. Sci. U. S. A. 103, 3846–3851. doi: 10.1073/pnas.0600035103
Caporaso J. G., Kuczynski J., Stombaugh J., Bittinger K., Bushman F. D., Costello E. K., et al. (2010). QIIME allows analysis of high-throughput community sequencing data. Nat. Methods 7, 335–336. doi: 10.1038/nmeth.f.303
Caporaso J. G., Lauber C. L., Walters W. A., Berg-Lyons D., Lozupone C. A., Turnbaugh P. J., et al. (2011). Global patterns of 16S rRNA diversity at a depth of millions of sequences per sample. Proc. Natl. Acad. Sci. U. S. A. 108, 4516–4522. doi: 10.1073/pnas.1000080107
Chen J., Li D., Chen B., Hu F., Zhu H., Liu C. (1999). The processes of dynamic sedimentation in the Changjiang Estuary. J. Sea Res. 41, 129–140. doi: 10.1016/s1385-1101(98)00047-1
Cline J. D. (1969). Spectrophotometric determination of hydrogen sulfide in natural waters. Limnol. Oceanogr. 14, 454–458. doi: 10.4319/lo.1969.14.3.045
Danovaro R., Gambi C. (2002). Biodiversity and trophic structure of nematode assemblages in seagrass systems: evidence for a coupling with changes in food availability. Mar. Biol. 141, 667–677. doi: 10.1007/s00227-002-0857-y
Delgado-Baquerizo M., Oliverio A. M., Brewer T. E., Benavent-González A., Eldridge D. J., Bardgett R. D., et al. (2018). A global atlas of the dominant bacteria found in soil. Science. 359, 320–325. doi: 10.1126/science.aap9516
Diaz R. J., Rosenberg R. (2008). Spreading dead zones and consequences for marine ecosystem. Science. 321, 926–929. doi: 10.1126/science.1156401
Diniz-Filho J. A., Telles M. P. (2000). Spatial pattern and genetic diversity estimates are linked in stochastic models of population differentiation. Genet. Mol. Biol. 23, 541–544. doi: 10.1590/s1415-47572000000300007
Ducklow H. (2000). Bacterial production and biomass in the oceans. In Microbial Ecology of the Oceans, 1nd ed. Kirchman D.. (ed). (Wiley), 85–120.
Edgar R. C., Haas B. J., Clemente J. C., Quince C., Knight R. (2011). UCHIME improves sensitivity and speed of chimera detection. Bioinformatics. 27, 2194–2200. doi: 10.1093/bioinformatics/btr381
Escalas A., Hale L., Voordeckers J. W., Yang Y., Firestone M. K., Alvarez-Cohen L., et al. (2019). Microbial functional diversity: From concepts to applications. Ecol. Evol. 9, 12000–12016. doi: 10.1002/ece3.5670
Faust K., Raes J. (2012). Microbial interactions: from networks to models. Nat. Rev. Microbiol. 10, 538–550. doi: 10.1038/nrmicro2832
Feng B. W., Li X. R., Wang J. H., Hu Z. Y., Meng H., Xiang L. Y., et al. (2009). Bacterial diversity of water and sediment in the Changjiang estuary and coastal area of the East China Sea. FEMS Microbiol. Ecol. 70, 236–248. doi: 10.1111/j.1574-6941.2009.00772.x
Fortunato C. S., Herfort L., Zuber P., Baptista A. M., Crump B. C. (2012). Spatial variability overwhelms seasonal patterns in bacterioplankton communities across a river to ocean gradient. ISME J. 6, 554–563. doi: 10.1038/ismej.2011.135
Griffith D. A., Peresneto P. R. (2006). Spatial modeling in ecology: the flexibility of eigenfunction spatial analyses. Ecology. 87, 2603–2613. doi: 10.1890/0012-9658(2006)87[2603:smietf]2.0.co;2
Gruber N., Galloway J. N. (2008). An Earth-system perspective of the global nitrogen cycle. Nature. 451, 293–296. doi: 10.1038/nature06592
Guo X. P., Niu Z. S., Lu D. P., Feng J. N., Chen Y. R., Tou F. Y., et al. (2017). Bacterial community structure in the intertidal biofilm along the Yangtze Estuary, China. Mar. Pollut. Bull. 124, 314–320. doi: 10.1016/j.marpolbul.2017.07.051
He Y., Li. M., Perumal V., Feng X., Fang J., Xie J., et al. (2016). Genomic and enzymatic evidence for acetogenesis among multiple lineages of the archaeal phylum Bathyarchaeota widespread in marine sediments. Nat. Microbiol. 1, 16035. doi: 10.1038/nmicrobiol.2016.35
Hou L., Zheng Y., Liu M., Gong J., Zhang X., Yin G., et al. (2013). Anaerobic ammonium oxidation (anammox) bacterial diversity, abundance, and activity in marsh sediments of the Yangtze Estuary. J. Geophys. Res. 118, 1237–1246. doi: 10.1002/jgrg.20108
Hou L., Zheng Y., Liu M., Li X., Lin X., Yin G., et al. (2015). Anaerobic ammonium oxidation and its contribution to nitrogen removal in China’s coastal wetlands. Sci. Rep. 5, 15621–15621. doi: 10.1038/srep15621
Hu M., Wilson B. J., Sun Z., Ren P., Tong C. (2017). Effects of the addition of nitrogen and sulfate on CH4 and CO2 emissions, soil, and pore water chemistry in a high marsh of the Min River estuary in southeastern China. Sci. Total Environ. 579, 292–304. doi: 10.1016/j.scitotenv.2016.11.103
Hubbell S. P. (2001). The unified neutral theory of biodiversity and biogeography (Princeton: Princeton University Press).
Jiao S., Liu Z., Lin Y., Yang J., Chen W., Wei G. (2016). Bacterial communities in oil contaminated soils: Biogeography and co-occurrence patterns. Soil Biol. Biochem. 98, 64–73. doi: 10.1016/j.soilbio.2016.04.005
Jiao S., Xu Y., Zhang J., Lu Y. (2019a). Environmental filtering drives distinct continental atlases of soil archaea between dryland and wetland agricultural ecosystems. Microbiome. 7, 1–13. doi: 10.1186/s40168-019-0630-9
Jiao S., Yang Y., Xu Y., Zhang J., Lu Y. (2019b). Balance between community assembly processes mediates species coexistence in agricultural soil microbiomes across eastern China. ISME J. 14, 1–15. doi: 10.1038/s41396-019-0522-9
Kim I. N., Lee K., Gruber N., Karl D. M., Bullister J. L., Yang S., et al. (2014). Increasing anthropogenic nitrogen in the North Pacific Ocean. Science. 346, 1102–1106. doi: 10.1126/science.1258396
Kuotung J., Liangsaw W., Gwoching G., et al. (2009). Distribution and behaviors of Cd, Cu, and Ni in the East China Sea surface water off the Changjiang estuary. Terr. Atmos. Ocean. Sci. 20, 433–443.
Leibold M. A., Mcpeek M. A. (2006). Coexistence of the niche and neutral perspectives in community ecology. Ecology. 87, 1399–1410. doi: 10.1890/0012-9658(2006)87[1399:cotnan]2.0.co;2
Lin G., Lin X. (2022). Bait input altered microbial community structure and increased greenhouse gases production in coastal wetland sediment. Water Res. 218, 118520. doi: 10.1016/j.watres.2022.118520
Lisa J. A., Song B., Tobias C. R., Hines D. E. (2015). Genetic and biogeochemical investigation of sedimentary nitrogen cycling communities responding to tidal and seasonal dynamics in Cape Fear River Estuary. Estuarine Coast. Shelf Sci. 167, 313–323. doi: 10.1016/j.ecss.2015.09.008
Liu J., Liu X., Wang M., Qiao Y., Zheng Y., Zhang X. H. (2015). Bacterial and archaeal communities in sediments of the north chinese marginal seas. Microb. Ecol. 70, 105–117. doi: 10.1007/s00248-014-0553-8
Liu J., Meng Z., Liu X., Zhang X. (2019). Microbial assembly, interaction, functioning, activity and diversification: a review derived from community compositional data. Mar. Life Sci. Technol. 1, 112–128. doi: 10.1007/s42995-019-00004-3
Liu M., Hou L. J., Yang Y., Zou H., Lu J., Wang X. (2001). Distribution and sources of polycyclic aromatic hydrocarbons in intertidal flat surface sediments from the Yangtze estuary, China. Environ. Geol. 41, 90–95. doi: 10.1007/s002540100347
Liu X., Wang Y., Gu J. D. (2021). Ecological distribution and potential roles of woesearchaeota in anaerobic biogeochemical cycling unveiled by genomic analysis. Comput. Struct. Biotec. 19, 794–800. doi: 10.1016/j.csbj.2021.01.013
Liu Q., Wu Y., Liao L., Zhang D., Yuan Y., Wang Z. A., et al. (2018). Shift of bacterial community structures in sediments from the changjiang (Yangtze river) estuary to the east China sea linked to environmental gradients. Geomicrobiol. J. 35, 898–907. doi: 10.1080/01490451.2018.1489914
Liu C., Xia J., Cui Q., Zhang D., Liu M., Hou L., et al. (2022). Crab bioturbation affects competition between microbial nitrogen removal and retention in estuarine and coastal wetlands. Environ. Res. 215, 114280. doi: 10.1016/j.envres.2022.114280
Liu M., Xiao T., Wu Y., Zhou F., Zhang W. (2011). Temporal distribution of the archaeal community in the Changjiang Estuary hypoxia area and the adjacent East China Sea as determined by denaturing gradient gel electrophoresis and multivariate analysis. Can. J. Microbiol. 57, 504–513. doi: 10.1139/w11-037
Liu J., Zhu S., Liu X., Yao P., Ge T., Zhang X. H. (2020). Spatiotemporal dynamics of the archaeal community in coastal sediments: assembly process and co-occurrence relationship. ISME J. 14, 1–16. doi: 10.1038/s41396-020-0621-7
Lozupone C. A., Knight R. (2007). Global patterns in bacterial diversity. Proc. Natl. Acad. Sci. U. S. A. 104, 11436–11440. doi: 10.1073/pnas.0611525104
Lu L., Zhao D., Li Z., Fernández L. D. (2023). Editorial: Microbial diversity and ecosystem functioning in fragmented rivers worldwide. Front. Microbiol. 14, 1253190. doi: 10.3389/fmicb.2023.1253190
Ma B., Wang H., Dsouza M., Lou J., He Y., Dai Z., et al. (2016). Geographic patterns of co-occurrence network topological features for soil microbiota at continental scale in eastern China. ISME J. 10, 1891–1901. doi: 10.1038/ismej.2015.261
Marcel M. M. K., Hannah K., Boran K. (2018). The microbial nitrogen-cycling network. Nat. Rev. Microbiol. 16, 263–276.
Newman M. E. (2006). Modularity and community structure in networks. Proc. Natl. Acad. Sci. U. S. A. 103, 8577–8582. doi: 10.1073/pnas.0601602103
Roden E. E., Lovley D. R. (1993). Evaluation of 55Fe as a tracer of Fe(III) reduction in aquatic sediments. Geomicrobiol. J. 11, 49–56. doi: 10.1080/01490459309377931
Santos L., Vaz L., Gomes N. C., Vaz N., Dias J. M., Cunha A., et al. (2014). Impact of freshwater inflow on bacterial abundance and activity in the estuarine system Ria de Aveiro. Estuarine Coast. Shelf Sci. 138, 107–120. doi: 10.1016/j.ecss.2013.12.021
Satinsky B. M., Smith C. B., Sharma S., Landa M., Medeiros P. M., Coles V. J., et al. (2017). Expression patterns of elemental cycling genes in the Amazon River Plume. ISME J. 11, 1852–1864. doi: 10.1038/ismej.2017.46
Seitz K. W., Dombrowski N., Eme L., Spang A., Lombard J., Sieber J. R., et al. (2019). Asgard archaea capable of anaerobic hydrocarbon cycling. Nat. Commun. 10, 1822. doi: 10.1038/s41467-019-09364-x
Shen L. D., Wu H. S., Liu X., Li J. (2017). Cooccurrence and potential role of nitrite- and nitrate-dependent methanotrophs in freshwater marsh sediments. Water Res. 123, 162–172. doi: 10.1016/j.watres.2017.06.075
Shi H., Yang Y., Liu M., Yan C., Yue H., Zhou J. (2014). Occurrence and distribution of antibiotics in the surface sediments of the Yangtze Estuary and nearby coastal areas. Mar. Pollut. Bull. 83, 317–323. doi: 10.1016/j.marpolbul.2014.04.034
Sørensen J. T. (1982). Reduction of ferric iron in anaerobic, marine sediment and interaction with reduction of nitrate and sulfate. Appl. Environ. Microbiol. 43, 319–324. doi: 10.1128/aem.43.2.319-324.1982
Spang A., Saw J. H., Jørgensen S. L., Zaremba-Niedzwiedzka K., Martijn J., Lind A. E., et al. (2015). Complex archaea that bridge the gap between prokaryotes and eukaryotes. Nature. 521, 173–179. doi: 10.1038/nature14447
Stegen J. C., Lin X., Fredrickson J. K., Chen X., Kennedy D. W., Murray C. J., et al. (2013). Quantifying community assembly processes and identifying features that impose them. ISME J. 7, 2069–2079. doi: 10.1038/ismej.2013.93
Sun Z., Li G., Wang. C., Jing Y., Zhu Y., Zhang S., et al. (2015). Community dynamics of prokaryotic and eukaryotic microbes in an estuary reservoir. Sci. Rep. 4, 6966–6966. doi: 10.1038/srep06966
Sunagawa S., Coelho L. P., Chaffron S., Kultima J. R., Labadie K., Salazar G., et al. (2015). Structure and function of the global ocean microbiome. Science. 348, 1261359–1261359. doi: 10.1126/science.1261359
Wang Z., Juarez D. L., Pan J., Blinebry S. K., Gronniger J., Clark J. S., et al. (2019). Microbial communities across nearshore to offshore coastal transects are primarily shaped by distance and temperature. Environ. Microbiol. 21, 3862–3872. doi: 10.1111/1462-2920.14734
Wang K., Ye X., Chen H., Zhao Q., Hu C., He J., et al. (2015). Bacterial biogeography in the coastal waters of northern Zhejiang, East China Sea is highly controlled by spatially structured environmental gradients. Environ. Microbiol. 17, 3898–3913. doi: 10.1111/1462-2920.12884
Wei G., Li M., Li F., Li H., Gao Z. (2016). Distinct distribution patterns of prokaryotes between sediment and water in the Yellow River estuary. Appl. Microbiol. Biotechnol. 100, 9683–9697. doi: 10.1007/s00253-016-7802-3
Wu W., Huang B. (2019). Protist diversity and community assembly in surface sediments of the South China Sea. MicrobiologyOpen. 8, e891. doi: 10.1002/mbo3.891
Xiong J., Ye X., Wang K., Chen H., Hu C., Zhu J., et al. (2014). Biogeography of the sediment bacterial community responds to a nitrogen pollution gradient in the East China Sea. Appl. Environ. Microbiol. 80, 1919–1925. doi: 10.1128/aem.03731-13
Ye Q., Wu Y., Zhu Z., Wang X., Li Z., Zhang J. (2016). Bacterial diversity in the surface sediments of the hypoxic zone near the Changjiang Estuary and in the East China Sea. MicrobiologyOpen 5, 323–339. doi: 10.1002/mbo3.330
Zhang Z., Han P., Jiao S., Jiao S., Dong H., Liang X., et al. (2023a). Spatiotemporal dynamics of bacterial taxonomic and functional profiles in estuarine intertidal soils of China coastal zone. Microbial Ecol. 85, 383–399. doi: 10.1007/s00248-022-01996-9
Zhang Y., Jiao N. (2007). Dynamics of aerobic anoxygenic phototrophic bacteria in the East China Sea. FEMS Microbiol. Ecol. 61, 459–469. doi: 10.1111/j.1574-6941.2007.00355.x
Zhang X., Yao C., Zhang B., Tan W., Gong J., Wang G. Y., et al. (2023b). Dynamics of benthic nitrate reduction pathways and associated microbial communities responding to the development of seasonal deoxygenation in a coastal mariculture zone. Environ. Sci. Technol. 57, 15014–15025. doi: 10.1021/acs.est.3c03994
Zhang W., Zeng C., Tong C., Zhai S., Lin X., Gao D. (2015). Spatial distribution of phosphorus speciation in marsh sediments along a hydrologic gradient in a subtropical estuarine wetland, China. Estuarine Coast. Shelf Sci. 154, 30–38. doi: 10.1016/j.ecss.2014.12.023
Zheng Y., Hou L., Chen F., Zhou J., Liu M., Yin G., et al. (2019). Denitrifying anaerobic methane oxidation in intertidal marsh soils: Occurrence and environmental significance. Geoderma. 357, 113943. doi: 10.1016/j.geoderma.2019.113943
Keywords: marine water, archaeal community, assembly processes, co-occurrence patterns, biogeochemical function
Citation: Tian C, He X, Zhou H, Liu C, Gao D, Chang Y, Zhao S, Zhang W and Zhang Z (2024) Archaeal community from the Northern Hangzhou Bay to the East China Sea: biogeography, ecological processes, and functional potential. Front. Mar. Sci. 11:1469717. doi: 10.3389/fmars.2024.1469717
Received: 24 July 2024; Accepted: 16 September 2024;
Published: 08 October 2024.
Edited by:
Xianbiao Lin, Ocean University of China, ChinaReviewed by:
Wei Xie, Sun Yat-sen University, ChinaSujata Dabolkar, Government College of Arts, Science and Commerce, Quepem, India
Copyright © 2024 Tian, He, Zhou, Liu, Gao, Chang, Zhao, Zhang and Zhang. This is an open-access article distributed under the terms of the Creative Commons Attribution License (CC BY). The use, distribution or reproduction in other forums is permitted, provided the original author(s) and the copyright owner(s) are credited and that the original publication in this journal is cited, in accordance with accepted academic practice. No use, distribution or reproduction is permitted which does not comply with these terms.
*Correspondence: Zongxiao Zhang, zhangzx@sustech.edu.cn
†These authors have contributed equally to this work