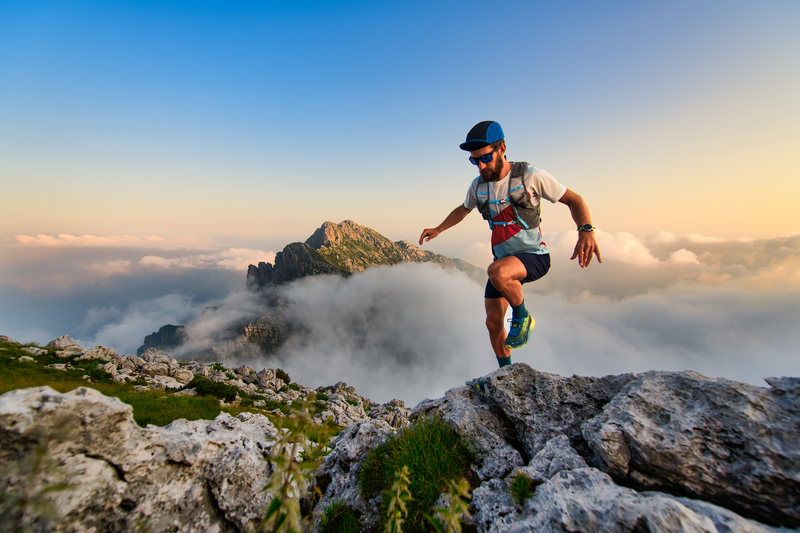
95% of researchers rate our articles as excellent or good
Learn more about the work of our research integrity team to safeguard the quality of each article we publish.
Find out more
ORIGINAL RESEARCH article
Front. Mar. Sci. , 15 October 2024
Sec. Marine Ecosystem Ecology
Volume 11 - 2024 | https://doi.org/10.3389/fmars.2024.1467371
Urbanization is particularly prevalent along the coast, causing a considerable change in the ecology of the habitats found there. Ports, docks and all the structures linked to this anthropization modify the coastal environment by providing new niches, but also new constraints. Thus, ports are ecosystems in their own right, although they are rarely studied as such. In Europe’s largest marina (La Rochelle, France), among the multitude of organisms inhabiting it, four taxa seem particularly interesting to study: Mytilidae, Ostreïdae, Pectinidae and ascidiacea. Because these taxa, which belong to the bivalve and tunicate groups, are the stewards of the health of the port environment both as bio-indicators and as engineering species. The establishment of a systematic and regular census allows us to study the evolution of their populations and to determine what influences their distribution. To have as less impact as possible on the fauna studied, the census was carried out by underwater photography. The study shows that the populations are partly conditioned by the hydrodynamics of the environment and by the anthropic activity which is carried out there. Indeed, this study, which was carried out in a particular context (before and after the COVID-19 health crisis), shows the importance of anthropic pressure, particularly on the bivalve communities. A large amount of data is needed to understand what precisely governs bivalve and tunicate populations. Therefore, an innovative method, using artificial intelligence to automate the analyses, was tested in this study. This promising method should facilitate the census by reducing the analysis time.
Coastal environments are biodiversity hotspots and provide refuge, food and nursery for many organisms (Henseler et al., 2019). They are also the site of significant human activity. Today, more than 20% of the world’s population lives within 30 km of the coast and 60%, or nearly 3.8 billion, live within 150 km in the greater coastal zone (Small and Nicholls, 2003; Béoutis et al., 2009). This hyper-anthropization has significantly changed coastal ecosystems (Le Berre et al., 2017). More and more structures, such as ports, are emerging and establishing new ecosystems in their own right. In these highly anthropized environments, life seem to have no place. However, significant communities grow, out of sight, protected by the maze of pontoons that the port ecosystem offers (Connell and Glasby, 1999; Glasby, 1999; Connell, 2000).
Studies in Sydney’s harbor (Australia) show that the abundance and diversity of subtidal epibiota is even increased by the presence of structures such as pontoons and pilings (Connell and Glasby, 1999; Connell, 2000). These structures offer new habitats that are easily colonizable by epibiota species such as mussels, ascidians, bryozoans, hydroids and sponges (Connell and Glasby, 1999; Connell, 2000). As Connell (2000) highlights, ports are indeed ecosystems in their own right because the communities present on their structures are not comparable to those present in the surrounding areas. La Rochelle marina (France) is the largest marina in Europe with 70 hectares and some 5,000 moorings spread over more than 60 pontoons. This makes it an ideal study site for studying port’s ecosystems.
Among the multitude of species that inhabit this marina, three groups seem particularly abundant: bivalves, tunicates and bryozoans. Indeed, after numerous scientific dives and several samples taken in La Rochelle marina, bivalves [mainly: Mytilus edulis (Linnaeus, 1758), Modiolus barbatus (Linnaeus, 1758), Crassostrea gigas (Thunberg, 1793) and Mimachlamys varia (Linnaeus, 1758)], tunicates [mainly: Ciona intestinalis (Linnaeus, 1767) and Clavelina sp (Müller, 1776)] and bryozoans [mainly: Bugula sp (Oken, 1815)] seem to cover every square centimeter of pontoon. These organisms, known as filter feeders, have the particularity of filtering water from the environment in order to extract their food and live anchored to their substrates for part of their lives. Because of their way of life, these filter feeders have a strong interaction with their environment, which makes them particularly interesting to study. Indeed, by remaining anchored to the structures, they are constantly subjected to the environmental factors that characterize the port (anthropogenic or not) and their feeding behavior leads them to be in contact with a large quantity of water charged with various elements (food, trace elements, sediments etc.). This way of life makes them good bioindicators, especially because some species such as scallops are sensitive and have a high bioaccumulation potential towards trace elements (Ag, As, Cd, Co, Cr, Cu, Fe, Mn, Ni, Pb, Se, Sn, V and Zn) very present in port areas (Breitwieser et al., 2017). Therefore, scallops (Mimachlamys varia) and oysters (Crassostrea gigas) are used as an ecotoxicological biomonitoring model for marine environments, especially in La Rochelle marina (Bustamante and Miramand, 2005; Milinkovitch et al., 2015; Breitwieser et al., 2018, 2020).
Their feeding behavior, their propensity to bioaccumulate and their potential as engineer species make these filter feeders key models for eco-engineering. According to several studies, they can clean up their environments by capturing and accumulating pollutants, particularly trace elements, present in the water column (Kim et al., 2017; McCann and May, 2018; Vozzo et al., 2021). A project called the “Billion Oyster Project” was initiated in 2014 in New York. This project aims to reduce trace elements such as mercury in New York port through the filtration and bioaccumulation of oysters (Kim et al., 2017; McCann and May, 2018). Bivalves are also used to improve the ecological value of artificial structures (Bradford et al., 2020; Strain et al., 2018). These engineer species provide habitats for other mobile invertebrates and are a food source for many predators (Gutiérrez et al., 2003; Bradford et al., 2020; Strain et al., 2018). Bradford et al. (2020) show by seeding Saccostrea cuccullata oysters that their presence on artificial structures significantly increases their specific richness.
These organisms are all the more interesting to study because their feeding behavior leads them to be in contact with part of the sediments present in the water column. The sediments sucked up by these filter feeders are grouped during digestion in the feces or upstream by the mucus in the pseudo-feces. These rejects, called bioproducts, are involved in the composition of the mud (Walker et al., 2014; Zúñiga et al., 2014; Hamani et al., 2021). An experimental study conducted in La Rochelle marina shows that on the scale of this port (70ha) these organisms would be able to reject approximately 3.2 tons of bioproducts per day (Hamani et al., 2021). Studies carried out on oyster and mussel farms show that organisms such as mussels, oysters and tunicates have an impact on the quantity of surrounding mud, the sedimentation rate of suspended particles, the distribution, structure and composition of the mud (Mckindsey et al., 2011; Walker et al., 2014; Wang et al., 2018). These organisms are therefore not to be neglected in an environment, such as ports, where the mud must be regularly dredged to allow navigation.
With all involvements previously explained, bivalves and tunicates appear to be key actors of port ecosystem. Then, to better understand the port environment, it seems important to know the current state of their populations and what conditions them. However, to our knowledge, no recent study has defined the state of their populations in port environments. The objective of the present study is to investigate the abundance and distribution of filter feeders (bivalves and tunicates) in Europe’s largest marina, while proving an innovative recognition method: artificial intelligence (AI) image analysis. As filter feeders are omnipresent engineer species in the port area, their study should enable us to describe an important part of the biocenosis of this ecosystem and should reveal the biological richness and disparity of this environment. In addition, as a relevant bioindicator, the study of the distribution of these organisms should provide a better understanding of physico-chemical factors and thus enable us to characterize the biotope of this ecosystem. Finally, as filter feeders seem to have an impact on mud behavior, their study will provide new insights into the factors governing siltation in port area.
This study was carried out in a particular context: the first sampling campaign was carried out in June 2019, i.e. one year before the sanitary crisis caused by COVID-19, and the second campaign in June 2020, i.e. one month after the crisis. During this sanitary crisis, a total confinement, including a ban on navigation, was applied from March to May 2020, strongly reducing anthropic pressure on the study area (Portlarochelle.com, 2020a, b, c). This study thus provides an overview of the effect of a major change in anthropogenic activity on model communities such as bivalves and tunicates.
In order to apply a non-destructive sampling method that is repeatable from year to year, the population survey was carried out using underwater photography. In a port of 70 hectares like La Rochelle marina, an efficient estimation of the populations requires a very large amount of data, especially if we want to include a time scale in our censuses. The automation of the analysis of photos has therefore become necessary. Artificial intelligence (AI) seems to be the ideal tool for this. Several studies, notably on coral reefs, show the relevance of this tool for detecting and identifying species in large image sets (González-Rivero et al., 2020; Hamylton et al., 2020; Nunes et al., 2020). González-Rivero et al. (2020) even state that when the computer has learned, the AI identifies corals 200 times faster than an operator and is much less expensive. This tool would therefore seem to be ideal for establishing regular, long-term monitoring of bivalves and tunicates in La Rochelle marina. However, ports are very dynamic environments and the turbidity of the water and therefore the quality of the photos can change quickly. In addition, the diversity of shape and the complexity of the entanglements that individuals may have under the pontoons should not be neglected. To ensure that the AI was able to overcome these difficulties and to prove its relevance, we propose here to present the first results of the survey of bivalves and tunicates carried out by an operator in La Rochelle marina and to compare them with those obtained by the AI.
La Rochelle marina is in the inland part of the Pertuis d’Antioche bay, along the French Atlantic coast, in the central part of the Bay of Biscay (Figure 1). The port is one of the largest in the world. It is divided into 4 basins: the Lazaret basin, the Tamaris basin, the Bout Blanc basin and the Marillac basin (Figure 1). Each basin is characterized by very distinct environmental conditions (hydro-sedimentary in particular, Huguet et al., 2020). The port has 63 pontoons of varying lengths, with a total of 15 km of pontoons.
Figure 1. Study area, La Rochelle marina (Les Minimes). Surrounded by red: the 4 basins which compose it.
The study will only focus on bivalves and tunicates. In La Rochelle marina, the most encountered family and species are:
- Mytilidae: Mytilus edulis (Linnaeus, 1758) and Modiolus barbatus (Linnaeus, 1758).
- Ostreidae: Crassostrea gigas (Thunberg, 1793) and Ostrea edulis (Linnaeus, 1758).
- Pectinidae: Mimachlamys varia (Linnaeus, 1758).
- Acidiacea: Ciona intestinalis (Linnaeus, 1767) and Clavelina sp (Müller, 1776).
As identification by species rank on the images is not possible for all these taxa, we have chosen to group them by family.
Two sampling campaigns were carried out during the summer of 2019 and the summer of 2020. During the 2019 campaign, only three of the four marina basins (Marillac, Bout Blanc and Tamaris) were sampled, i.e., 19 pontoons for a total of 1457 images. During the second campaign, all the basins were sampled, i.e., 27 pontoons for a total of 2331 images.
Systematic sampling by successive images was carried out. For each basin, one in two pontoons was sampled. For each pontoon, a sampling point is defined every 5m (Figure 2). For each of these points, four photos were taken across the width of the pontoon, to cover the entire pontoon (Figure 2). Photographic sampling is spaced to avoid overlapping, thus avoiding overestimation of abundance. However, it is distributed over the entire width and length of the pontoons to consider the heterogeneity of the environment.
The tangle of organisms is often complex and some hide others. Some organisms are even attached to other organisms. This makes them difficult to detect in a photograph. As the organisms are entangled, the estimation by photo tends to underestimate their abundance. To improve the quality of the abundance estimates, a second sampling by collection was conducted in parallel with the photographic sampling. This second sampling was carried out by a scientific diver. A floating quadra of 0.64m2 was placed under the pontoon, the entire quadra was photographed and then the organisms present in the quadra were physically sampled (Table 1). 27 collections of organisms were carried out in 2019 and 36 in 2020, i.e., 9 per basin (3 per pontoon on 3 separate pontoons). The organisms collected by the diver were counted and measured in the laboratory (Table 1). These measurements (Width*Length*Height) provide additional information on the state of the harbor’s filter feeder populations (Table 1).
Table 1. Estimation of the average number of individuals per square meter and the size of individuals by taxon and by basin, made by collection; and average number of individuals per square meter estimated from photographs taken in the same area, for the 2019 and 2020 campaigns.
The collections of organisms allowed to formally estimate the number of individuals. In order to improve the estimates obtained by the photographic analysis, a corrective factor relating to the average number of organisms collected per basin is applied, per taxon, to each photo of the corresponding basin. This correction factor is an adaptation of the methods used to correct an estimate when the probability of detection of individuals is not certain (Burnham and Overton, 1978; Otis et al., 1978; Gatti et al., 2011) This corrective factor is calculated for each basin as follows: Average number of organisms per square meter of a taxon, obtained by sampling/Average number of organisms per square meter of a taxon, obtained by photographic analysis. Only the photos corresponding to the collections are used to calculate this corrective factor. For example: For the Tamaris basin, in 2020, the average number of Mytilidae obtained per sampling is 157.55/m2 (Table 1). The average number of Mytilidae estimated on the corresponding photos is 72.71/m2 (Table 1). The correction factor is 157.55/72.71 = 2.16. Thus, for all photos of the Tamaris basin the number of Mytilidae per square meter is multiplied by 2.16. All the results presented below are corrected by this method.
The images were obtained using a GoPro® HeRO8 black placed on a measuring rod to standardize the position of the camera and the image shooting (Figure 2). As the port environment is often turbid, image feedback is necessary to ensure the quality of the image before shooting. A coaxial cable is added to the set-up to provide a WIFI link between the operator and the camera (Figure 2). Each shot provides a 33.41 × 25.06 cm photoquadrate. Each image is geo-referenced according to the EPSG/3857-WGS84/Pseudo-Mercator system.
All image analysis is carried out using the Ikomia® software. The images are corrected by a color balance for a better reading. The individuals are then counted by an operator. For this, each individual recognized in an image is surrounded by a box corresponding to its taxon in the software (Figure 3). A txt file is then extracted for each photo with the labels and size of each identified individual.
Figure 3. Example of image analyzed by operator, with the box corresponding to the different taxon identified.
Artificial intelligence is used in this study to automate the identification of individuals in images. This method is based on the concept of deep learning, a subcategory of machine learning, using neural network architectures which are inspired by the structure of the human brain. It works as a system of layers that analyzes the image one after other, usually the more layers there are, the more networks are able to learn. The raw data is input into the network and will be processed by each layer in a specific way. The result of this first processing will inform the next layer, which will in turn process the dataset until the last layer. Each layer corresponds to a piece of information useful for the recognition of the object.
The model used for this study was the fourth version of the Yolo model: Yolo-v4 model (Bochkovskiy et al., 2020). The two main advantages of this model are its accuracy and speed of execution. The first Yolo (You only Look Once) model was implemented in 2015 (Redmon et al., 2016; Bochkovskiy et al., 2020). Yolo model allowed an increase in the speed of program execution by processing the data in one go (the so-called one-pass approach) and not in two goes as previous models (Faster R-CNN, Mask R-CNN,…) did. For example, on an image where different communities of organisms are present, the Yolo model allows the identification of the areas where the species are found as well as their identification at the same time for each layer.
Yolo-V4 model works in three main steps (Figure 4):
- The image is sent to the first layer of the “backbone”, which will allow an initial simplistic identification of the objects (by their shape, color, etc.). The successive layers making up the “backbone” will extract maps indicating the location of the objects in the image.
- This data is sent to the “neck”, which will sort out the relevant maps, extract them and combine them into useful images. These new images will allow a fine detection of all the objects present (from the simplest to the most complex objects, from low to high resolution images).
- Finally, the “head” or “dense prediction” will collect the information provided by the “neck” and conclude on the detection of objects. These objects will be boxed, and their nature will be determined.
Figure 4. Architecture of a “one-pass” network presented as Backbone, Neck and Dense Prediction (head). The input image is provided to the first layer of the backbone, and the head part returns the detections in the form of bounding boxes. (Source: Bochkovskiy et al., 2020).
AI analysis is carried out in two stages, learning and analysis/validation; and these are carried out for each taxon. The first step consists in training the neural network to extract the relevant features in the image in order to recognize objects. To do this, images already annotated by an operator are presented to the network. It will use these annotations to adapt its own parameters to provide the good output, the recognition of the expected objects. The more images (samples) are presented to the system, the more it learns and becomes accurate. The second step consists of presenting images without annotations to the network and comparing the results obtained with those obtained by an operator on these same images. This step allows the method to be validated and the system error rate to be estimated.
The analysis by artificial intelligence was carried out on the Ikomia® software, by implementing the Darknet framework (https://github.com/AlexeyAB/darknet), according to the protocol of Bochkovskiy et al. (2020). Only the resolution and the batch size were modified to correspond to the needs of the study; from 416 x 416 to 1536 x 1536 pixels for the resolution and 32 to 128 for the batch size. These modifications improve the detection quality, as the objects to be detected are small compared to the image size and improve the convergence of the algorithm. The validation was carried out on the two most abundant taxa, Ascidiacea and Mytilidae, the other taxa are not present enough for the model to learn to recognize them. The training was carried out on all the images (year 2019 and 2020) containing these taxa, i.e. 3553 images for Ascidiacea and 502 images for Mytilidae. The validation was carried out on a sample of 100 images, randomly selected and not used for training. An operator manually re-analyzed these photos to estimate the rate and type of errors made by the software.
The abundance and distribution of organisms are compared spatially at the scale of the basins but also between pontoons and within pontoons; and temporally by comparing the 2 sampling campaigns. The data are presented in number of individuals per square meter (ind./m2). All the statistical tests have been carried out using the software R® (Version 3.6.3 “Holding the Windsock”) with a rejection threshold of 5%. The data do not comply with any of the conditions of application of the parametric tests. The Wilcoxon-Mann-Whitney test is used for simple comparisons. For multiple comparisons, the Kruskal Wallis test and the Wilcoxon Pairwise post-hoc test are used. The distributions are mapped using QGIS® version 3.22., in EPSG/3857-WGS84/Pseudo-Mercator projection. Only data collected by an operator are used to analyze the abundance and distribution of organisms.
In 2019, Mytilidae were absent from the Bout Blanc basin and were few presents in the Marillac basin (1.57 ± 34.03 ind./m2). Mytilidae were statistically more abundant in the Tamaris basin (p-value<0.05), i.e., 80 times more than in the two other basins (127.19 ± 639.25 ind./m2). The Mytilidae are distributed homogeneously between the pontoons of the three basins sampled except for the most northeastern pontoon of the Tamaris basin (p-value<0.05), which has a larger population of Mytilidae with average densities of over 1500 individuals per square meter (Figure 5A; Table 2).
Figure 5. Distribution and abundance maps of Mytilidae (A), ascidiacea (B), Ostreïdae (C) and Pectinidae (D) for the 2019 campaign in La Rochelle marina. Average densities (ind./m2) are estimated for each 20m of pontoon. In order not to mask the distribution of the least abundant taxa, the average density bubbles are separated into 10 classes with density values specific to each taxon.
Table 2. Average number of individuals per square meter and standard deviation, by basin and by taxon.
Ascidiacea are statistically more present in the Tamaris basin than in the Marillac basin (p-value<0.05) and in the Bout blanc basin (p-value<0.05) (Tamaris > Marillac > Bout blanc). They are eight times more present in the Tamaris basin (9228.49 ± 5678.06 ind./m2) than in the Marillac basin (1199.50 ± 1288.31 ind./m2) which is itself three times more densely populated than the Bout blanc basin (401.47 ± 376.24 ind./m2; p-value<0.05). The ascidiacea have clear distributions between the basins. However, the pontoons of the same basin have comparable densities (Figure 5B; Table 2).
As for the ascidiacea, the Ostreïdae are statistically more present in the Tamaris basin than in the Marillac (p-value<0.05) and the Bout blanc basins (p-value<0.05) (Tamaris > Marillac > Bout blanc). Ostreïdae are more than five times more present in the Tamaris basin (119.39 ± 312.36 ind./m2) than in the Marillac basin (22.24 ± 110.06 ind./m2), which is itself three times more densely populated than the Bout blanc basin (12.92 ± 83.25 ind./m2; p-value<0.05). Ostreïdae are homogeneously distributed in the pontoons of the Marillac and Bout Blanc basins. However, like the Mytilidae, they are more concentrated to the north of the Tamaris basin (Figure 5C; Table 2).
Pectinidae are practically absent from the Bout blanc and Marillac basins with less than one individual per square meter. They are present in the Tamaris basin (3.14 ± 8.20 ind./m2). Even if their distribution is heterogeneous between the basins (p-value<0.05), their distribution is comparable between the pontoons of the same basin (Figure 5D; Table 2).
In 2020, Mytilidae are more present in the Tamaris basin than in the Lazaret basin (p-value<0.05) and in the Marillac and Bout blanc basins (p-value<0.05) (Tamaris > Lazaret > Marillac = Bout blanc). The average number of Mytilidae per square meter in the Tamaris basin (198.88 ± 308.57 ind./m2) is more than 50 times higher than in the Bout blanc basin (3.51 ± 27.82 ind./m2). The distribution of Mytilidae is homogeneous between the pontoons of the Tamaris, Marillac and Bout Blanc basins. Their distribution is more heterogeneous in the Lazaret basin where we find density peaks in the two easternmost pontoons (p-value<0.05) and in the northernmost pontoon (p-value<0.05) (Figure 6A; Table 2).
Figure 6. Distribution and abundance maps of Mytilidae (A), ascidiacea (B), Ostreïdae (C) and Pectinidae (D) for the 2020 campaign in La Rochelle marina. Average densities (ind./m2) are estimated for each 20m of pontoon. In order not to mask the distribution of the least abundant taxa, the average density bubbles are separated into 10 classes with density values specific to each taxon.
The ascidiacea have a statistically different distribution between all the basins (p-value<0.05), as for the Mytilidae, they are more present in the Tamaris (949.50 ± 552.02 ind./m2) and Lazaret basins (959.44 ± 1055.4774 ind./m2). They are less present in the Marillac basin (288.41 ± 315.90 ind./m2), 8 times less than in the Tamaris and Lazaret basins. The distribution of ascidiacea is heterogeneous between and within each pontoon (p-value<0.05). With densities ranging from 0-300 to over 2000 individuals per square meter on a single pontoon (Figure 6B; Table 2).
Ostreïdae are also mainly present in the Tamaris basin (230.16 ± 497.21 ind./m2; p-value<0.05). Their distribution does not show any statistical difference between other basins. The distribution of Ostreïdae is similar between the pontoons of the Marillac, Bout Blanc and Lazaret basins but is heterogeneous in the Tamaris basin (p-value<0.05). Dense populations of Ostreïdae are found on some pontoons in the north of the Tamaris basin (Figure 6C; Table 2).
In contrast, Pectinidae are almost absent from the Tamaris basin and are mainly present in the Lazaret (7.28 ± 30.19 ind./m2) and Marillac (7.27 ± 46.61 ind./m2) basins. They are 7 times more present in these two basins (p-value<0.05) than in the Bout blanc basin (1.71 ± 12.97 ind./m2). Pectinidae are mainly found in the pontoons to the north of the Lazaret basin, as well as in the pontoons to the north and in the center of the Marillac basin. The pontoons with the highest densities of Pectinidae are also those showing the greatest heterogeneity of distribution (Figure 6D; Table 2).
In 2019, the Tamaris basin has more ascidiacea (9228.49 ± 5678.06 ind./m2) than Mytilidae (127.19 ± 639.25 ind./m2; p-value<0.05), Ostreïdae (119.39 ± 312.36 ind./m2; p-value<0.05) or Pectinidae (3.14 ± 8.20 ind./m2; p-value<0.05) (ascidiacea > Mytilidae > Ostreïdae > Pectinidae). It is the most contrasted basin, all taxa are represented (Figures 7A, B; Table 2).
Figure 7. Relative abundance maps of tunicates (ascidiacea) and bivalves (Mytilidae, Ostreïdae and Pectinidae) for each portion of the pontoons (20m) of La Rochelle marina in 2019 (A) and 2020 (C). Maps of relative abundance of bivalves (Mytilidae, Ostreïdae and Pectinidae) for each portion of the pontoons (20m) of the La Rochelle marina in 2019 (B) and in 2020 (D).
The Marillac basin contains mainly ascidiacea (1199.50 ± 1288.31 ind./m2; p-value<0.05), Ostreïdae (22.24 ± 110.06 ind./m2; p-value<0.05) then Mytilidae (1.57 ± 34.03 ind./m2; p-value<0.05) and Pectinidae (0.29 ± 2.59 ind./m2; p-value<0.05) (ascidiacea > Ostreïdae > Mytilidae > Pectinidae). For bivalves, the Marillac basin is mainly represented by Ostreïdae (Figures 7A, B; Table 2).
The Bout blanc basin contains only ascidiacea (401.47± 376.24 ind./m2), Ostreïdae (12.92 ± 83.25 ind./m2) and Pectinidae (0.61 ± 4.13 ind./m2). Mytilidae are absent (Figure 6; Table 2).
For the whole marina, the ascidiacea are the most present in terms of number of individuals per square meter with densities ranging from 401.47 ± 376.24 ind./m2 to 1199.50 ± 1288.31 ind./m2. On the opposite, the Pectinidae are the least present. The Pectinidae are mainly present in the Tamaris basin with a density of 3.14 ± 8.20 ind./m2. Their density is less than one individual per square meter for the other basins (Figures 7A, B).
In 2020, whatever the basin, the ascidiacea are always the most present group in number of individuals per square meter with densities per basin ranging from 288.41 ± 315.90 ind./m2 to 959.44 ± 1055.47 ind./m2 (Figures 7C, D; Table 2).
The Pectinidae are, on the opposite, the least present group whatever the basin with densities per basin ranging from 0.13 ± 1.71 ind./m2 to 7.27 ± 46.61 ind./m2. At the scale of the marina the ascidiacea are 100 times more present than the Pectinidae with respective averages of 622.62 ± 640.66 ind./m2 and 3.37 ± 18.77 ind./m2 (Figures 7C, D; Table 2).
The Tamaris and Bout Blanc basins have similar communities (ascidiacea > Ostreïdae > Mytilidae > Pectinidae; p-value<0.05) but in different proportions and distribution. The same applies to the Marillac and Lazaret basins (ascidiacea > Mytilidae > Ostreïdae > Pectinidae; p-value<0.05) (Figures 7C, D).
The communities change significantly between 2019 and 2020 (p-value<0.05). The average number of bivalves (Mytilidae, Ostreidae and Pectinidae) per square meter was significantly higher in 2020 than in 2019 for the Tamaris (from 83.24 ± 319.93 to 143.05 ± 239.16 ind./m2; p-value<0.05), Bout Blanc (from 4.51 ± 29.12 to 16.75 ± 64.82 ind./m2; p-value<0.05) and Marillac (from 8.03 ± 48.89 to 20.85 ± 84.23 ind./m2; p-value<0.05) basins.
Ascidiacea were more present in 2019 (p-value<0.05), except for the Bout Blanc basin where their average number per square meter doubled in 2020 (Figure 8; Table 2).
Figure 8. Average density and standard deviation (ind./m2) of Mytilidae, ascidiacea, Ostreïdae and Pectinidae per year and per basin.
In the Tamaris basin, the Mytilidae populations were supplanted by the Ostreïdae populations in terms of number of individuals per square meter in 2020 (Figure 8; Table 2).
In the Marillac basin it is the opposite, the Mytilidae populations have supplanted the Ostreïdae populations in terms of number of individuals per square metre. Pectinidae have also increased in the Marillac basin, whereas in 2019 they had less than one individual per square meter (Figure 8; Table 2).
In the Bout Blanc basin, the Mytilidae population, which was non-existent in 2019, has expanded (3.51 ± 27.82 ind./m2) and has surpassed the Pectinidae population (Figure 8; Table 2).
In the 100 photos analyzed for Mytilidae the operator detected 932 individuals. The AI detected 896 objects of which 774 were Mytilidae and 122 were detection errors. The AI was therefore 16.96% less efficient than the operator and made 13.61% identification errors for this taxon.
For the Ascidiacea, in the 100 photos analyzed the operator identified 3740 individuals. The AI identified 3206 objects of which 3167 were Ascidiacea and 39 were identification errors. The AI is therefore 15.33% less efficient than an operator, but only makes 1.21% errors in the identification of this taxon.
Bivalves and tunicates are two model groups at the center of the port environment. They are the guardian of its health as a bio-indicator and as an engineering species used in bio-engineering to limit the presence of metals in the water column (Milinkovitch et al., 2015; McCann and May, 2018; Breitwieser et al., 2020; Vozzo et al., 2021). They are also a refuge for many organisms and have a significant impact on the surrounding mud (Bradford et al., 2020; Strain et al., 2018; Zúñiga et al., 2014; Hamani et al., 2021). The establishment of a systematic and regular census allows us to study the evolution of their populations and what influences their distribution. The present study highlights the complexity of their communities and shows the speed of their evolution. A large amount of data is needed to understand what governs this complexity and dynamics; this is why an innovative method, using artificial intelligence, to automate the analyses, was tested during this study.
In order to have as less impact as possible on the communities studied, an underwater photographic survey is used. This method allows a systematic, standard and repeatable census. The main limitation of this method is that it leads to an underestimation of the populations. Indeed, photographs only allow a two-dimensional analysis, and individuals that are entangled or present under other organisms cannot be sampled by this method. This is the case for the estimation of Ostreïdae in particular, which are often at the base of communities, with other organisms coming to settle on them (observation by the scientific diver). This bias can be reduced by collecting samples to adjust the estimates. Photographic analysis allows rapid and complete sampling of very large areas. This is a significant advantage for accurate sampling of populations present in a large and diverse environment such as the La Rochelle marina.
The communities studied are complex, with variable distributions between taxa, between basins and between pontoons. This disparity would be linked to the fact that the port offers a fragmented discontinuous habitat where each pontoon can be considered as an island of habitat (Connell, 2000). Many other factors such as the type of materials used for the construction of pontoons (Connell and Glasby, 1999), the heterogeneity of the surface of the pontoons (Bradford et al., 2020) or interspecific competition (Shinen and Morgan, 2009; Bradford et al., 2020) could explain this disparity. Thus, it is very difficult in a complex and dynamic environment such as the marina of La Rochelle to discriminate the factors influencing the distribution of species as the environmental factors are so numerous and changing.
Nevertheless, the Tamaris basin is the basin with the highest density of organisms of all taxa in 2019 and 2020. This is all the more visible in 2019 when we observe density peaks for the four taxa studied, particularly in the north of this basin. These high densities can be related to the strong hydrodynamics of the basin. Huguet (2020) shows in a study of the hydro-sedimentary flows in the marina of La Rochelle that the Tamaris basin is one of the basins with the highest hydrodynamics. Indeed, this basin is the closest to the open sea and is bordered to the north and south by the two water entrances of La Rochelle marina (Huguet, 2020). The water coming from the open sea is rich in seston and therefore in food, which could explain the fact that this basin is the most densely populated. This hypothesis could also explain the high densities of ascidiacea, Pectinidae and Mytilidae in the southernmost pontoon of the Lazaret, which is also located close to the port’s entrance, in a zone of strong hydrodynamics (Huguet, 2020). The larvae of the four taxa studied are planktonic and their recruitment is influenced by the hydrodynamics of the environment (Martel, 1993; Gosling, 2015; Holland, 2016). It is also possible that the densities observed in the Tamaris basin reflect the transport of these larvae (Martel, 1993).
Ascidiacea is the predominant group in terms of density. They are present on all pontoons and in all basins in both 2019 and 2020. This predominance can be explained by their low sensitivity to anthropogenic pollutants and their great adaptability (Chebbi, 2010; Bouchard and Bouchard-Madrelle, 2018). Some species such as Ciona intestinalis, which is the predominant species in La Rochelle marina, have a high survival rate and a low malformation rate at the larval stage in presence of hydrocarbons and biocide such as tobacco tar (Bouchard and Bouchard-Madrelle, 2018).
In contrast, Pectinidae is the least present group in the marina of La Rochelle. Several studies propose Mimachlamys varia, a Pectinidae commonly found in the Atlantic, as a bio-indicator for its capacity to accumulate trace elements and for its physiological sensitivity towards these trace elements (Bustamante and Miramand, 2005; Milinkovitch et al., 2015; Breitwieser et al., 2018). This Pectinidae would be particularly sensitive to elements such as copper and zinc, which are elements linked to anthropic activity and common in port areas (Ory et al., 2021; Hamani et al., 2021). These elements at concentrations such as found in La Rochelle marina would impact several biological functions such as osmoregulation, oxidative stress, reproduction and energy metabolism (Ory et al., 2021; Hamani et al., 2021). This sensitivity to trace elements could influence the distribution of Pectinidae in the port area and their presence could be used as an indicator of trace element concentration in the environment. Long-term monitoring of their populations and trace element concentrations is necessary to validate this hypothesis.
Between 2019 and 2020, bivalve densities increased in all basins. Populations of Mytilidae have appeared in the Bout Blanc basin and Pectinidae have increased by a factor of seven in the Marillac basin, whereas they were very low (less than one individual per square metre) in 2019. These taxa are sessile in the adult stage and have a lifetime of several years (Gosling, 2015; Holland, 2016). Inter-annual population changes are therefore related to larval recruitment and survival, but not to population movements.
This population increase occurs in a particular context. Indeed, the first sampling campaign was carried out in June 2019, i.e., one year before the health crisis caused by COVID-19, and the second campaign in June 2020, i.e., one month after the crisis. During this health crisis, a total containment, including a navigation ban, was applied from March to May 2020, strongly reducing the anthropic pressure on the study area. This increase in bivalve density could reflect reduced anthropogenic pressure. This hypothesis is reinforced by the fact that the recruitment period for the larvae of the three bivalve species present in the port of Les Minimes occurs mainly between March and July, i.e. during the period of the sanitary crisis (Bodoy et al., 1991; Arnaud et al., 2000; Bouquet et al., 2023). In addition, the development strategy of bivalves can be described as an ‘r strategy’, i.e. a large number of offspring and rapid growth to compensate for high mortality. For example, the mytilidae and pectinidae in our study have high growth rates and mature in less than a year (Bodoy et al., 1991; Soletchnik et al., 2013). It is during the early stages of their lives that bivalves are most sensitive to environmental stresses, such as anthropic pressure (Bernard, 2011; Toupoint, 2012; Gamain, 2016). A reduction in stress at the most critical stages of their development could therefore explain the rapid increase in their population. This hypothesis supports the one presented above on Pectinidae which assumes that their populations are strongly influenced by anthropogenic activity and reinforces the fact that these organisms could be used as relevant bioindicators. Long-term monitoring of their population, including precise data on water quality at a fine scale, could validate this hypothesis.
Image analysis using artificial intelligence allows rapid analysis of large datasets. Once trained, the software analyzed the 200 images in just a few minutes, whereas an operator would have done the work in several hours or even days.
This method of analysis is not yet fully optimized for data such as those in our study. Learning could only be performed on two of the four taxa of interest and the detection error rates were 13.63% for the Mytilidae. This is mainly explained by the fact that the organisms present under the pontoons are very entangled and take on very diverse postures and shapes, which makes learning more complex since the annotated data available does not sufficiently cover all these representations.
These results are nevertheless encouraging as the AI results are 98.79% accurate for ascidiacea. Moreover, the detection rates are equivalent to 83.04% for the Mytilidae and 84.67% for the Ascidiacea of those of an operator. The AI system will improve its learning with the number of available data, so the detection rates and accuracy should be improved with the new data collected at each campaign (if an operator has annotated them). If the AI can correctly identify two of the four taxa, the amount of work for the operators will be greatly reduced. However, in the future, the network could be trained to recognize more taxa. Trying out other models or creating a model specific to our needs are also solutions to consider improving the results of this tool.
The structures that make up a port provide a habitat for many organisms. In Europe’s largest marina, two groups of species, bivalves and tunicates, seem particularly interesting to study as they are abundant and play key roles, notably as engineer and bioindicator species. The present study shows the first results of the monitoring of their population.
The establishment of a systematic and regular census allows us to see the evolution of their populations and to study what influences their distribution. For this purpose, an underwater photographic census is used. This method allows a systematic, standard and repeatable census. A large amount of data is needed to understand what governs this complexity and dynamics; this is why an innovative method, using artificial intelligence, to automate the analyses, was tested during this study. This method allows the analysis of Mytilidae and Tunicates with detection rates of respectively 83.04% and 84.67% and with an associated error rate of 13.63% and 1.21% and is therefore not yet fully adapted to our research. However, analysis by artificial intelligence can be improved with more data available. Indeed, according to the concept of deep learning, the more the network is confronted with data, the more the network “learns” and improves its analyses. This promising method should make it possible in the future to simplify the overall monitoring of populations and to analyze larger datasets, thus providing the additional details needed to understand what conditions these communities, while saving considerable analysis time.
The study shows that the populations are partly conditioned by the hydrodynamics of the environment and by the anthropic activity which is carried out there. Results also suggests that to understand precisely what conditions the populations of bivalves and tunicates, further research is necessary as their distributions are so complex.
This study, carried out in a particular context (before and after the COVID-19 health crisis), seems to show the importance of anthropic pressure on these communities and would supports the fact that bivalves, particularly Pectinidae, are relevant bioindicators.
The raw data supporting the conclusions of this article will be made available by the authors, without undue reservation.
The manuscript presents research on animals that do not require ethical approval for their study.
VH: Conceptualization, Data curation, Formal analysis, Investigation, Methodology, Project administration, Resources, Software, Supervision, Validation, Visualization, Writing – original draft, Writing – review & editing. IB: Conceptualization, Funding acquisition, Investigation, Project administration, Resources, Supervision, Validation, Visualization, Writing – original draft, Writing – review & editing. OL: Data curation, Formal analysis, Investigation, Methodology, Software, Validation, Visualization, Writing – original draft, Writing – review & editing. GD: Data curation, Formal analysis, Investigation, Methodology, Software, Validation, Visualization, Writing – review & editing. J-CB: Data curation, Formal analysis, Funding acquisition, Investigation, Methodology, Resources, Software, Validation, Visualization, Writing – review & editing. LM: Conceptualization, Funding acquisition, Investigation, Methodology, Project administration, Resources, Supervision, Validation, Visualization, Writing – original draft, Writing – review & editing.
The author(s) declare financial support was received for the research, authorship, and/or publication of this article. Funding for this work came from the following separate sources: (i) Contrat de Plan Etat-Région FEDER and the CNRS ECONAT Axe 1-Ressources Marines Littorales: qualité et éco- valorisation and Axe 2-Gestion Intégrée des Zones Littorales et Portuaires (ii) French Ministry of Higher Education, Research and Innovation for doctoral grant of V. Hamani (Ministère de l’Enseignement supérieur, de la Recherche et de l’Innovation, MESRI).
We gratefully thank the Marina of La Rochelle for their assistance in particularly for the data transmission and the “Musée Mer Marine Bordeaux” for the involvement in the VH PhD. This work has also been supported by the French Ministry of Higher Education and Research, the European Regional Development Fund, the regional state plan, the National Centre for Scientific Research and the project Urban and Coastal Lab.
The authors declare that the research was conducted in the absence of any commercial or financial relationships that could be construed as a potential conflict of interest.
All claims expressed in this article are solely those of the authors and do not necessarily represent those of their affiliated organizations, or those of the publisher, the editors and the reviewers. Any product that may be evaluated in this article, or claim that may be made by its manufacturer, is not guaranteed or endorsed by the publisher.
Arnaud C., Faury N., Bouquet J. P., Guesdon S., Kantin R. (2000). Suivi de la reproduction de l'huître creuse Crassostrea gigas et de la moule Mytilus edulis dans le bassin de Marennes Oléron. Available online at: https://archimer.ifremer.fr/doc/00000/3883/ (accessed October 2024).
Béoutis A., Jean P., Colas S. (2009). L’Observatoire du littoral: démographie et économie du littoral (Insee, Montrouge, France: IFEN Les dossiers l’observatoire du littoral).
Bernard I. (2011). Écologie de la reproduction de l’huître creuse, Crassostrea gigas, sur les côtes atlantiques françaises. Vers une explication de la variabilité du captage. (Doctoral dissertation). La Rochelle University, France.
Bochkovskiy A., Wang C. Y., Liao H.-Y. M. (2020). Optimal speed and accuracy of object detection. arXiv preprint arXiv:2004.10934.
Bodoy A., Garnier J., Heurtebise S., Nollet V., Chagot D. (1991). Diversification des productions conchylicoles en Poitou-Charentes. L'élevage du pétoncle noir Chlamys Varia. Rapport IFREMER, RIDRV, 91–11.
Bouchard J., Bouchard-Madrelle C. (2018). [amp]]Eacute;tonnantes possibilités d ’adaptation de la larve d’ascidie en milieu pollué. Bull. la Socieíteí zoologique France 143, 3–47.
Bouquet A. L., Oudot G., Bodin P. (2023). Recrutement de la moule Mytilus edulis dans les pertuis charentais: suivi des larves de moules; Syntheìse annuelle (France: CAPENA), 15p.
Bradford T. E., Astudillo J. C., Lau E. T. C., Perkins M. J., Lo C. C., Li T. C. H., et al. (2020). Provision of refugia and seeding with native bivalves can enhance biodiversity on vertical seawalls. Mar. Pollut. Bull. 160. doi: 10.1016/j.marpolbul.2020.111578
Breitwieser M., Barbarin M., Plumejeaud-Perreau C., Dubillot E., Guyot T., Huet V., et al. (2020). Biomonitoring of Mimachlamys varia transplanted to areas impacted by human activities (La Rochelle Marina, France). Chemosphere 243. doi: 10.1016/j.chemosphere.2019.125199
Breitwieser M., Vigneau E., Viricel A., Becquet V., Lacroix C., Erb M., et al. (2018). What is the relationship between the bioaccumulation of chemical contaminants in the variegated scallop Mimachlamys varia and its health status? A study carried out on the French Atlantic coast using the Path ComDim model. Sci. Total Environ. 640–641. doi: 10.1016/j.scitotenv.2018.05.317
Breitwieser M., Viricel A., Churlaud C., Guillot B., Martin E., Stenger P. L., et al. (2017). First data on three bivalve species exposed to an intra-harbour polymetallic contamination (La Rochelle, France). Comp. Biochem. Physiol. Part - C Toxicol. Pharmacol. 199. doi: 10.1016/j.cbpc.2017.02.006
Burnham K. P., Overton W. S. (1978). Estimation of the size of a closed population when capture probabilities vary among animals. Biometrika 65. doi: 10.1093/biomet/65.3.625
Bustamante P., Miramand P. (2005). Evaluation of the variegated scallop Chlamys varia as a biomonitor of temporal trends of Cd, Cu, and Zn in the field. Environ. pollut. 138. doi: 10.1016/j.envpol.2005.02.014
Chebbi (2010). Etude systématique, bio-écologique et chimique des ascidies de Tunisie; Sciences de l’environnement (Cartage, Tunisia: Université du 7 Novembre à Carthage; Institut National Agronomique de Tunisie).
Connell S. D. (2000). Floating pontoons create novel habitats for subtidal epibiota. J. Exp. Mar. Bio. Ecol. 247. doi: 10.1016/S0022-0981(00)00147-7
Connell S. D., Glasby T. M. (1999). Do urban structures influence local abundance and diversity of subtidal epibiota? A case study from Sydney Harbour, Australia. Mar. Environ. Res. 47. doi: 10.1016/S0141-1136(98)00126-3
Gamain P. (2016). Impact des polluants et du changement climatique sur les capacités de reproduction et le développement embryo larvaire de l’huître creuse Crassostrea gigas dans le bassin d’Arcachon. (Doctoral dissertation). Bordeaux University, France.
Gatti S., Blanc L., Gimenez O., Marboutin E. (2011). Session intensive 2011 de piégeage photographique du Lynx ( Lynx lynx ) en Franche-Comté : estimation de densité sur deux sites de référence. 1–17. Available online at: https://professionnels.ofb.fr/sites/default/files/pdf/suivi/Rapport-Piegeagephoto_FrancheComte_Lynx-2011.pdf (accessed October 2024).
Glasby T. M. (1999). Differences between subtidal epibiota on pier pilings and rocky reefs at Marinas in Sydney, Australia. Estuar. Coast. Shelf Sci. 48. doi: 10.1006/ecss.1998.0417
González-Rivero M., Beijbom O., Rodriguez-Ramirez A., Bryant D. E. P., Ganase A., Gonzalez-Marrero Y., et al. (2020). Monitoring of coral reefs using artificial intelligence: A feasible and cost-effective approach. Remote Sens 12. doi: 10.3390/rs12030489
Gosling E. (2015). Marine Bivalve Molluscs: Second Edition, Marine Bivalve Molluscs: Second Edition. New York, USA: John Wiley & Sons. doi: 10.1002/9781119045212
Gutiérrez J. L., Jones C. G., Strayer D. L., Iribarne O. O. (2003). Mollusks as ecosystem engineers: The role of shell production in aquatic habitats. Oikos. 101 (1), 79–90. doi: 10.1034/j.1600-0706.2003.12322.x
Hamani V., Ory P., Bodet P., Murillo L. (2021). Untargeted metabolomics reveals a complex impact on different metabolic pathways in scallop Mimachlamys varia (Linnaeus, 1758) after short-term exposure to copper at environmental dose. Metabolites. 11 (12), 862. doi: 10.3390/metabo11120862
Hamylton S. M., Zhou Z., Wang L. (2020). What can artificial intelligence offer coral reef managers? Front. Mar. Sci. 7. doi: 10.3389/fmars.2020.603829
Henseler C., Nordström M. C., Törnroos A., Snickars M., Pecuchet L., Lindegren M., et al. (2019). Coastal habitats and their importance for the diversity of benthic communities: A species- and trait-based approach. Estuar. Coast. Shelf Sci. 226. doi: 10.1016/j.ecss.2019.106272
Huguet J. (2020). Dynamique Hydrosédimentaire en milieu portuaire : Application au port de plaisance de la Rochelle (Doctoral dissertation). La Rochelle University, France.
Huguet J. R., Brenon I., Coulombier T., Hamani V. (2020). Dynamics and management of siltation in a macro-tidal marina: The case of La rochelle marina, France. Ocean Coast. Manag 198. doi: 10.1016/j.ocecoaman.2020.105371
Kim M., Garcia A., Malinowski P., Park E. J. (2017). Mercury uptake by eastern oysters (Crassostrea virginica) in oyster restoration project of the New York Harbor. Int. J. Environ. Sci. Technol. 14. doi: 10.1007/s13762-017-1358-3
Le Berre I., Robert S. (2017). L’ urbanisation face à l’océan. L'océan découvert, 208–209. halshs-01626607 208–209.
Martel A. (1993). Dispersal and recruitment of zebra mussel (Dreissena polymorpha) in a nearshore area in west-central Lake Erie: the significance of postmetamorphic drifting. Can. J. Fish. Aquat. Sci. 50. doi: 10.1139/f93-001
McCann B. M., May P. D. (2018). New York City Oyster Monitoring Report : 2016 – 2017 A collaboration between The Nature Conservancy and Billion Oyster Project. New York, USA: Nature Conservancy, 2016–2017.
Mckindsey C. W., Archambault P., Callier M. D., Olivier F. (2011). Archimer. Can. J. Zool. 89, 622–646. doi: 10.1139/z11-037
Milinkovitch T., Bustamante P., Huet V., Reigner A., Churlaud C., Thomas-Guyon H. (2015). In situ evaluation of oxidative stress and immunological parameters as ecotoxicological biomarkers in a novel sentinel species (Mimachlamys varia). Aquat. Toxicol. 161, 170–175. doi: 10.1016/j.aquatox.2015.02.003
Nunes J. A. C. C., Cruz I. C. S., Nunes A., Pinheiro H. T. (2020). Speeding up coral reef conservation with AI-aided automated image analysis. Nat. Mach. Intell. 2. doi: 10.1038/s42256-020-0192-3
Ory P., Hamani V., Bodet P. E., Murillo L., Graber M. (2021). The variegated scallop, Mimachlamys varia, undergoes alterations in several of its metabolic pathways under short-term zinc exposure. Comp. Biochem. Physiol. - Part D Genomics Proteomics 37, 100779. doi: 10.1016/j.cbd.2020.100779
Otis D., Burnham K. P., White G. C., Anderson D. R. (1978). Statistical inference from capture data on closed animal populations. Wildl. Monogr. 62.
Portlarochelle.com (2020a). Avis au navigateur n°18.2020-Mesures faces au covid 19. Available online at: https://www.portlarochelle.com/avis_aux_navigateurs/mesures-face-au-covid-19/15/ (Accessed 28 December 2021).
Portlarochelle.com (2020b). Avis au navigateur n°18 bis-Mesures faces au covid 19-complément d’information. Available online at: https://www.portlarochelle.com/avis_aux_navigateurs/mesures-face-au-covid-19-complement-dinformations/15/ (Accessed 28 December 2021).
Portlarochelle.com (2020c). Avis au navigateur n°14/2020-fin de l’interdiction de naviguation. Available online at: https://www.portlarochelle.com/wp-content/uploads/2020/05/Avis-N°24.2020-Fin-de-linterdiction-de-navigation.pdf (Accessed 28 December 2021).
Redmon J., Divvala S., Girshick R., Farhadi A. (2016). “You only look once: Unified, real-time object detection,” in IEEE conference on computer vision and pattern recognition. (Las Vegas, USA: IEEEXplore), 779–788.
Shinen J. S., Morgan S. G. (2009). Mechanisms of invasion resistance: competition among intertidal mussels promotes establishment of invasive species and displacement of native species. Mar. Ecol. Prog. Ser. 383. doi: 10.3354/meps07982
Small C., Nicholls R. J. (2003). A global analysis of human settlement in coastal zones. J. Coast. Res. 19.
Soletchnik P., Robert S., Le Moine O. (2013). Suivi expérimental de la croissance de la moule, Mytilus edulis, sur les bouchots des Pertuis Charentais entre 2000 et 2010 (Ifremer, France: Étude des performances de croissance en liens avec l'environnement des élevages). Available online: https://archimer.ifremer.fr/doc/00120/23097/ (accessed October 2024).
Strain E. M. A., Olabarria C., Mayer-Pinto M., Cumbo V., Morris R. L., Bugnot A. B., et al. (2018). Eco-engineering urban infrastructure for marine and coastal biodiversity: Which interventions have the greatest ecological benefit? J. Appl. Ecol. 55 (1). doi: 10.1111/1365-2664.12961
Toupoint N. (2012). Le succès de recrutement de la moule bleue: influence de la qualité de la ressource trophique (Doctoral dissertation, Rimouski University, Quebec).
Vozzo M. L., Mayer-Pinto M., Bishop M. J., Cumbo V. R., Bugnot A. B., Dafforn K. A., et al. (2021). Making seawalls multifunctional: The positive effects of seeded bivalves and habitat structure on species diversity and filtration rates. Mar. Environ. Res. 165. doi: 10.1016/j.marenvres.2020.105243
Walker T. R., Grant J., Weise A. M., McKindsey C. W., Callier M. D., Richard M. (2014). Influence of suspended mussel lines on sediment erosion and resuspension in Lagune de la Grande Entrée, Îles-de-la-Madeleine, Québec, Canada. Aquaculture. 433. doi: 10.1016/j.aquaculture.2014.07.006
Wang F., Zhou Y., Wang P., Xu S., Liu P. (2018). Potential role of scallops Argopecten irradians in deposition of particulate nutrient and trace elements in a eutrophic estuary, northern China. Aquac. Environ. Interact. 10, 135–147. doi: 10.3354/aei00258
Keywords: bivalves, tunicates, bioindicator, port ecosystem, photographic survey, artificial intelligence
Citation: Hamani V, Brenon I, Lebon O, Demarcq G, Burie J-C and Murillo L (2024) What is hidden under our pontoons? Abundance and distribution of filter feeders (bivalves and tunicates) in the port area revealed. Artificial intelligence: an interesting analysis tool? Front. Mar. Sci. 11:1467371. doi: 10.3389/fmars.2024.1467371
Received: 19 July 2024; Accepted: 26 September 2024;
Published: 15 October 2024.
Edited by:
Iñigo Muxika, Technological Center Expert in Marine and Food Innovation (AZTI), SpainReviewed by:
Ganesh Thiruchitrambalam, Pondicherry University, IndiaCopyright © 2024 Hamani, Brenon, Lebon, Demarcq, Burie and Murillo. This is an open-access article distributed under the terms of the Creative Commons Attribution License (CC BY). The use, distribution or reproduction in other forums is permitted, provided the original author(s) and the copyright owner(s) are credited and that the original publication in this journal is cited, in accordance with accepted academic practice. No use, distribution or reproduction is permitted which does not comply with these terms.
*Correspondence: Vincent Hamani, dmluY2VudC5oYW1hbmlAdW5pdi1sci5mcg==
Disclaimer: All claims expressed in this article are solely those of the authors and do not necessarily represent those of their affiliated organizations, or those of the publisher, the editors and the reviewers. Any product that may be evaluated in this article or claim that may be made by its manufacturer is not guaranteed or endorsed by the publisher.
Research integrity at Frontiers
Learn more about the work of our research integrity team to safeguard the quality of each article we publish.