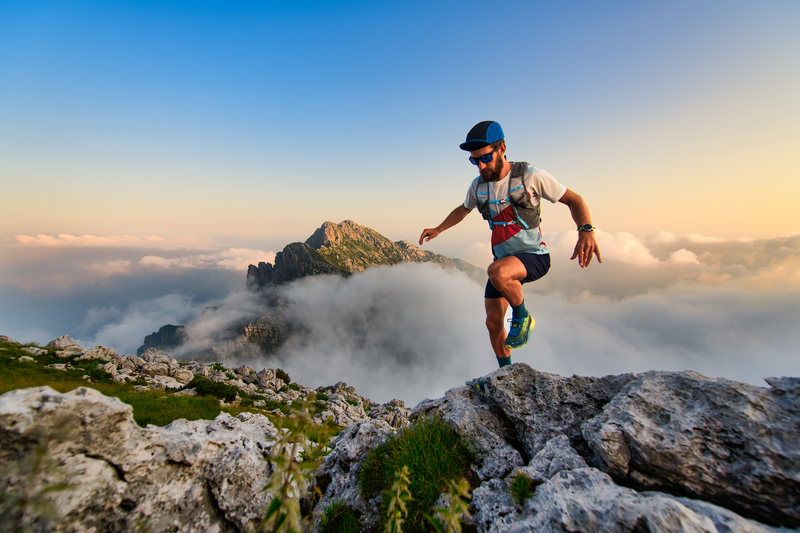
94% of researchers rate our articles as excellent or good
Learn more about the work of our research integrity team to safeguard the quality of each article we publish.
Find out more
ORIGINAL RESEARCH article
Front. Mar. Sci. , 20 December 2024
Sec. Aquatic Microbiology
Volume 11 - 2024 | https://doi.org/10.3389/fmars.2024.1462432
Mastering the characteristics of planktonic microbial communities under the influence of basin-scale environmental factors can provide scientific basis to develop more targeted protection and restoration measures. Here, an environmental DNA metabarcoding technology was applied to investigate the phytoplankton and microzooplankton simultaneously along the whole basin of Jialing River, one of the most important rivers in Southwest China with large scale altitude gradient and water temperature discrepancy. Along the river-way from upper to lower reach, an upward trend in richness of both phytoplankton and microzooplankton was observed with significant positive and negative correlations to water temperature and altitude (Spearman correlation, p < 0.05), respectively. Significant variations in their compositions along the river were also uncovered with Chrysophyta and Foraminifera was potential keystones recognized by the co-occurrence network analysis. Additionally, exponential distance-decay of similarities were detected for both phytoplankton and microzooplankton, which were stronger for phytoplankton. Based on the results of variation partitioning analysis and null model, variations in phytoplankton communities were more governed by environmental selection than those in microzooplankton. Furthermore, the co-occurrence network showed a declining trend in the complexity and stability from the upper to lower reaches. These findings could serve to establish a basis for the investigation of phytoplankton and microzooplankton communities in rivers with multiple artificial modifications.
River ecosystem is a key factor in maintaining the ecological balance of a region and provide numerous ecological services to human beings, such as water supply, irrigation, climate regulation and water conservation (Sabater et al., 2023). The protection and restoration of river ecosystems is essential for sustainable development (Huang et al., 2022). The Jialing River is one of the most important rivers in Southwest China, which plays an important role in the water supply and water quality regulation of the Yangtze River. The river originates from the Qinling Moutains, specifically at an elevation of about 2,800 m, and flows approximately 1,120 km to join the Yangtze River at Chongqing, which only have an elevation of about 400 m. In recent years, in order to meet the economic development and energy demand, some water conservancy projects have been constructed in the Jialing River basin, such as the Ningxia Water Conservancy Hub Project and the Chaotian Water Conservancy Hub Project, based on the large scale altitude gradient (Guo et al., 2020). The altitude gradient can significantly influence river ecosystems, affecting biodiversity, water quality, and ecological dynamics (Huang et al., 2024). High-altitude reservoirs can create more stable flow conditions, which may enhance the habitat for various macroinvertebrate families (Petruzziello et al., 2021). In addition, studies show that while high-altitude reservoirs do not significantly alter the breakdown rates of coarse particulate organic matter, they increase its availability compared to pristine sites (García et al., 2023). Moreover, high-altitude reservoirs tend to mitigate atmospheric influences on water temperature, leading to more stable thermal conditions compared to stretches affected solely by meteorological factors (Körner, 2007). The altered thermal regimes could have significant implications for aquatic species by affecting their metabolism and reproductive cycles (Bonacina et al., 2023). Besides, higher altitudes may experience more pronounced biotic responses due to increased temperatures and altered precipitation patterns from climate change, which can lead to shifts in species distributions and community compositions within river ecosystems (Naud et al., 2019). In summary, investigating these dynamics is crucial for effective conservation and management strategies aimed at preserving biodiversity in highland and pre-highland environments.
In contrast to the significant impacts of altitude gradients on large organisms, such as fishes and macroinvertebrates (Askeyev et al., 2017; Bruno et al., 2019), knowledge of its influences on planktonic microbial communities are still relatively shallow. Phytoplankton and microzooplankton are the basis of aquatic ecosystems and are primary producers and consumers in the food chain, respectively (Gusha et al., 2019). They perform key functions in the water column such as photosynthesis, provision of dissolved oxygen and participation in the material cycle (Lobus and Kulikovskiy, 2023). They also have sensitive responses to water quality and nutrient status of water bodies, inducing them as indicator organisms of a good water environment (Singh et al., 2013; Costa et al., 2016). Understanding the ecological impacts of altitude gradients on planktonic microbes is crucial for developing strategies to maintain healthy, functioning river ecosystems.
Planktonic microorganisms play a crucial role in the aquatic food chain, participating in the cycling of nutrients in water bodies (Yang et al., 2021). They can quickly respond to environmental changes, basin scale studies can reveal the dynamics of planktonic microbial communities among different regions, which helps predict the response of aquatic ecosystems to climate change and human activities (Isabwe et al., 2018). Previous studies have reported the variations in the phytoplankton and microzooplankton communities in the Jialing River for assessing the water quality. One such study highlighted the changes in the phytoplankton community after the impoundment of the Caojie Reservoir in the Jialing River, which suggested a state of medium eutrophication and light to moderate pollution levels (Yang et al., 2015). Another study investigated the seasonal variations in microzooplankton communities of Jialing River in Nanchong, and determined a moderate pollution (Ren et al., 2011). These findings are crucial as they provide insights into how human activities and environmental management practices, like reservoir impoundment, can influence aquatic ecosystems in significant ways, impacting biodiversity and water quality in river systems such as the Jialing River. However, these studies only focus on a certain section of the Jialing River, and to the best of knowledge, the present study is the first research to investigate the phytoplankton and microzooplankton communities in the Jialing River at the basin scale.
Here, water samples covering the whole Jialing River were obtained and the planktonic microbial communities were investigated by an environmental DNA (eDNA) metabarcoding sequencing technology. Through the deep analysis of the obtained data, including diversity, composition, assembly mechanisms, and co-occurrence network, the planktonic microbial communities on this important river were more fully described. Meanwhile, variations of these characteristics of planktonic microbial communities among different reaches along the Jialing River were explored. The findings of this study provide a richer context on planktonic microbes and the effects of altitude gradient on them in the Jialing River basin, which could give a solid foundation for further research.
As the largest tributary in the upper reach of the Yangtze River, The Jialing River originates from the Minshan Mountain Range at the easternmost tip of the Tibetan Plateau with a drainage area of approximately 160,000 km2. The regional climate of Jialing River is complex and diverse with both climatic characteristics of temperate, subtropical and tropical zones (Wang et al., 2023). It is rich in water resources, mineral resources and biological resources, and is an important agricultural, industrial and energy base in China (Shao et al., 2021). In addition, the Jialing River basin is home to many rare and endangered species under national protection, and is an important biodiversity reserve in the southwestern part of China (Qin et al., 2023). The Jialing River has a total length of 1,120 km, which has been divided into three reaches based on the geomorphological characteristics (Figure 1A). The upper reach covers an area of 60,000 km2 and is approximately 357 km long with a natural gradient of 3.8‰. The middle reach is approximately 633 km long and covers an area of approximately 105,000 km2 with only 0.3‰ gradient. The lower reach only covers an area of about 3,600 km2 with 96 km long. Since 1970, multiple dams have been established on the Jialing River, causing significant changes to the original aquatic ecological conditions of the Jialing River (Qin et al., 2023).
Figure 1. (A) Map of sample collection. Each sampling station is marked as a black point. (B) Variations in the environmental variables among different reaches of the Jialing River. Different lowercase letters above each box in the same sub-figure represent significant differences among samples from different reaches (Tukey’s HSD test, p < 0.05). [ASL, above sea level (m); WT, water temperature (°C); DO, dissolved oxygen (mg/L); EC, electrical conductivity (μs/cm); FV, flow velocity (m/s); SD, secchi depth (m); TDS, total dissolved solids (ppm); Chl_a, chlorophyll a (μg/L); TOC, total organic carbon (mg/L); COD, chemical oxygen demand (mg/L); TN, total nitrogen (mg/L); TP, total phosphorus (mg/L)] (C) Phylogenetic tree of top 100 abundant ASVs for phytoplankton (left) and microzooplankton (right), respectively.
According to the geomorphological characteristics and dams, the Jialing River can be separated into 20 sections, including 4 sections in the upper reach, 14 sections in the middle reach, and 2 sections in the lower reach. Three surface water samples were collected from each section, with one sample each from the upper, middle, and lower locations of the section (Figure 1). A total of 12, 42, and 6 sites were determined in the upper, middle, and lower reaches of the Jialing River, respectively, for sampling. Due to the large geographical area, all samples for this study were collected from August 1, 2023, to August 6, 2023. The summer temperatures were high, and there was no rainfall during the six days of collection; however, there was substantial rainfall in July, resulting in higher river flow. About 2 L of surface water was sampling from each site with a specialized water sampler, and then divided it equally in two parts. The water samples were placed in disinfected polyethylene bottles. One of them was stored at 4°C for measurements of environmental variables. Another part water samples were immediately filtered through a 0.22 μm pore size polycarbonate membranes (142 mm diameter, Millipore, USA) with a peristaltic pump. The filters were immediately preserved in liquid nitrogen, transported to our lab, and stored at -80°C until DNA extraction.
During the sampling, we simultaneously measured a series of environmental indicators. The longitude, latitude, and altitude of sampling sites were observed using a GPS device. The water temperature (WT), dissolved oxygen (DO), pH, and electrical conductivity (EC), total dissolved solids (TDS), chemical oxygen demand (COD), and total organic carbon (TOC) of water samples were monitored by LS310 multi-parameters water quality analyzer (Shenzhen Linshang Technology Co.,Ltd., Shenzhen, China) (Hou et al., 2013). In addition, the Secchi depth (SD) and flow velocity (FV) were measured by Setter disk (JCT-8S, Juchuang Group Co., Ltd., Qingdao, China) and DJS flow meter (CSDIHO, Shanghai, China), respectively. Total nitrogen (TN) and total phosphorus (TP) were determined using Cleverchem 380 fully automatic intermittent chemical analyzer (DeChem Tech. GmbH, Hamburg, Germany) (Hou et al., 2013). The concentration of chlorophyll a (Chl_a) and ammonia-nitrogen was measured using TE-700PLUS multi-parameters water quality analyzer (TIANER, Tianjin, China) (Yang et al., 2011). All instruments used to record environmental variables are calibrated and verified before use.
Total DNA for each water sample was extracted from the filters using a PowerWater DNA isolation kit (QIAGEN, CA, USA) according to the manufacturer’s instructions. After the quality measurements by agarose gel electrophoresis (1% concentration) and NanoDrop ND-1000 Spectrophotometer (NanoDrop, USA), the qualified DNA were amplified by the primers 1389F (TCCCTGCCHTTTGTACACAC) and 1510R (CCTTCYGCAGGTTCACCTAC) to obtain the eukaryotic 18S rRNA V9 region (Amaral-Zettler et al., 2009). The detailed processes of PCR amplified and library construction complied and implemented by a previous study (Zhao et al., 2022a). Subsequently, the libraries of all samples were sequenced using an Illumina NovaSeq 6000 platform at Shanghai BIOZERON Co., Ltd (Shanghai, China).
The raw sequences were firstly performed the quality control process to obtain clean reads, including removed reads with an average Q score < 20 and deleted reads containing ambiguous bases or mismatches in primers (Bokulich et al., 2013). Then, remaining high-quality reads were clustered into amplicon sequence variants (ASVs) using the DADA2 algorithm in QIIME2 software (Bokulich et al., 2018). To eliminate the effects of differences in sequencing depth, the read size of all samples was rarefied to 500,000 reads. SILVA 138 release (Yilmaz et al., 2014) was used as the reference database for taxonomic annotation of each ASVs. Following the analysis strategy outlined by Shen et al. (2024), all ASVs classified as fungi and metazoans were removed based on their taxonomy before identifying phytoplankton and microzooplankton. ASVs belonging to phytoplankton and microzooplankton were then assigned using a self-written R script according to the method described by Sun et al. (2023). Some categories here identified as phytoplankton include autotrophic, mixotrophic, and heterotrophic species (e.g., Pyrrophyta). However, due to the enormous diversity of data that are obtained through eDNA sequencing and the lack of comprehensive information on the nutritive metabolism of each species, most current studies are unable to discriminate such traits at the species level. Therefore, in the present context we followed a pragmatic approach we use the term phytoplankton to encompass eight categories traditionally regarded as part of this assemblage, which include Cryptophyta, Chlorophyta, Chrysophyta, Bacillariophyta, Pyrrophyta, Euglenophyta, Rhodophyta, and Xanthophyta. The remaining ASVs not assigned to phytoplankton were considered as microzooplankton. Finally, to reduce the potential sequencing error, ASVs that only detected in one sample and the read number lower than 10 were removed before further analyses.
All data analyses were performed by R v4.2.2 and the results were visualized by the “ggplot2” package. Four alpha-diversity indices, Chao1 (richness), Pd_faith (evolution), Shannon (diversity), and Pielou_J (evenness), of phytoplankton and microzooplankton communities were respectively calculated using the “vegan” package. Differences in the alpha diversity between phytoplankton and microzooplankton were assessed by the wilcox rank-sum test. In addition, variations in the environmental variables of water samples and alpha-diversity indices of phytoplankton or microzooplankton communities among different reaches were determined by the Tukey’s HSD test (“multcomp” package). Variations in the relative abundances of phytoplankton and microzooplankton phyla among different reaches were also tested by the Tukey’s HSD test. The Bray-Curtis distance for phytoplankton or microzooplankton between each of two samples were respectively estimated based on the “vegan” package. Then, variations in phytoplankton and microzooplankton community structures among different reaches were evaluated by principal coordinate analysis (PCoA) and adonis test by the “ape” and “vegan” packages. Moreover, random forest (RF) models were constructed based on the relative abundances of phytoplankton or microzooplankton phyla to distinguish the sample sources and identify the keytaxa for reach distinguishing by the “RandomForest” package. Subsequently, spearman correlation was used to explore the correlations between environmental variables with alpha diversity indices and keytaxa of phytoplankton and microzooplankton.
The environmental similarities based on the Euclidean distance and geographic distances (linear distance on a flat projection) between each of two sampling sites were calculated firstly using the “vegan” and “geosphere” packages, respectively. Then, partial least squares regression was applied to evaluate the distance-decay of community similarity (DDCS) between phytoplankton or microzooplankton (1 - Bray-Cutis distance) with environmental similarities and geographic distance, respectively. In addition, variation partitioning analysis (VPA) was completed to determine the relative importance of environmental and spatial factors in shaping phytoplankton and microzooplankton communities. The pure environmental variation without a spatial component represents the strength of species sorting; the pure spatial variation without an environmental component is interpreted as dispersal limitation. Moreover, a null model method reported by Stegen et al. (2012) was used to investigate the assembly processes of abundant and rare sub-communities. Based on the value of beta nearest taxon index (betaNTI) and Bray-Curtis-based Raup-Crick metric obtained from the null model, the relative importance of ecological processes for community assembly were estimated (Zhou and Ning, 2017).
The co-occurrence network of phytoplankton and microzooplankton in the upper, middle, and lower reaches were constructed respectively based on the Spearman rank correlations between the relative abundance of each ASV. Only ASVs that detected in more than 60% of samples within the same substance and the average relative abundance higher than 0.05% were remained to perform correlation analysis (“psych” package). Co-occurrence events were identified with statistically robust correlations (|correlation coefficient| > 0.6 with Benjamini-Hochberg adjusted p-value < 0.05) (Steinhauser et al., 2008). Network graphs were visualized using the Gephi interactive platform (Bastian et al., 2009). The topological roles of individual nodes in networks were evaluated by the threshold values of Pi (measuring how well a node was connected to nodes in different modules) and Zi (measuring how well a node was connected to other nodes in its own module) (Zhao et al., 2022b). Based on the Pi and Zi scores, nodes can be defined as network hubs (Zi > 2.5; Pi > 0.6), module hubs (Zi > 2.5; Pi < 0.6), connectors (Zi < 2.5; Pi > 0.6) and peripherals (Zi < 2.5; Pi < 0.6) (Poudel et al., 2016). In addition, robustness and vulnerability for co-occurrence networks were calculated to evaluate the network stability according to a previous study (Yuan et al., 2021). Changes in the robustness of co-occurrence networks between different reaches were also analyzed by the Tukey’s HSD test.
A total of 14 environmental variables of the water samples were measured and their differences among different reaches were compared (Figure 1B). Based on the comparison results, the above sea level (ASL) for the upper reach of the Jialing River was significantly higher than that for the middle and lower reaches (Tukey’s HSD test, p < 0.05), while the WT, EC and TDS were significantly increased in the middle and lower reaches (Tukey’s HSD test, p < 0.05). In addition, The SD content in the middle reach was significantly higher than that in upper and lower reaches (Tukey’s HSD test, p < 0.05), while the concentrations of TOC, COD, and TN were significantly reduced in the middle reach (Tukey’s HSD test, p < 0.05). Moreover, the FV was also found to be highest in the upper reach, followed by the lower reach, and lowest in the middle reach. Besides, the water pH and contents of Chl-a, ammonia and TP were not significantly varied among different reaches (Tukey’s HSD test, p > 0.05).
A total of 37,493,751 high quality sequences for 18S rRNA V9 region were obtained from 60 water samples, ranging from 557,053 to 698,869 sequences per sample (Supplementary Table S1). Among them, average 250,664 and 74,029 sequences were assigned to phytoplankton and microzooplankton, respectively (Supplementary Table S1), which were respectively clustered into 14,492 and 5,264 ASVs (Supplementary Table S2). For phytoplankton, 199 genera were uncovered with 78.46% ASV annotation rate, which belonged to 70 families and 8 phyla (Supplementary Table S2). On the other hand, the ASV annotation rate for microzooplankton was 59.06%, which obtained 162 genera belonged to 69 families and 16 phyla (Supplementary Table S2). The rarefaction and species accumulation curves for both phytoplankton and microzooplankton showed sufficient sequencing depth and sample size to capture the complete communities (Supplementary Figure S1). The top 100 abundant phytoplankton ASVs belonged to several phyla, including Bacillariophyta, Chlorophyta, Chrysophyta, Crytophyta, and Pyrrophyta, in contrast, the dominated microzooplankton ASVs mainly belonged to Ciliophora and Stramenopiles (Figure 1C).
Differences in the alpha diversity between phytoplankton and microzooplankton in the Jialing River were first explored, and the results showed significantly higher Chao1, Pd_faith, and Shannon indices of phytoplankton than those of microzooplankton (Wilcox rank-sum test, p < 0.05, Figure 2A). Furthermore, variations in the alpha diversity of phytoplankton and microzooplankton among different reaches of the Jialing River were investigated, respectively (Figure 2B). The Chao1 index of both phytoplankton and microzooplankton were showed to be significantly lower in upper reach than those in middle and lower reaches (Tukey’s HSD test, p < 0.05). The Pd_faith index of phytoplankton was also significantly lower in upper reach (Tukey’s HSD test, p < 0.05), while it was no obviously variations for microzooplankton. In addition, an increased trend was observed for the Shannon index of phytoplankton along the Jialing River from upper to lower reaches (Tukey’s HSD test, p < 0.05). In contrast, the trend for Shannon and Pielou_J indices of microzooplankton from the upper to lower reaches of Jialing River was declining (Tukey’s HSD test, p < 0.05). Moreover, PCoA plots based on the Bray-Curtis distance showed obviously separated clusters of both phytoplankton and microzooplankton among different reaches, especially between the upper reach to others (Figure 2C). Adonis test further indicated significant effects of reach division on the structure of phytoplankton and microzooplankton communities (p < 0.05).
Figure 2. (A) Differences in the alpha diversity between phytoplankton and microzooplankton. Different lowercase letters above each box in the same sub- figure. represent significant difference (Wilcox rank-sum test, p < 0.05). (B) Variations in the alpha diversity of phytoplankton and microzooplankton among different reaches from the Jialing River, respectively. Different lowercase letters above each box in the same sub- figure represent significant differences among samples from different reaches (Tukey’s HSD test, p < 0.05). (C) Principal coordinate analysis (PCoA) and adonis test of phytoplankton and microzooplankton communities based on the Bray-Curtis distance, respectively. The arrow path points the samples from upper to lower reaches.
In the Jialing River, Crytophyta, Chlorophyta, Chrysophyta, Bacillariophyta, and Pyrrophyta occupied the most of the phytoplankton communities (Figure 3A). The relative abundance of Crytophyta showed an increase first and then decrease trend along the Jialing River, which was significantly higher in the middle reach compared to the upper reach (Tukey’s HSD test, p < 0.05, Supplementary Figure S2A). Chrysophyta was more abundant in the upper reach, while Bacillariophyta and Pyrrophyta were enriched in the lower reach (Tukey’s HSD test, p < 0.05, Supplementary Figure S2A). The relative abundance of Chlorophyta showed an obvious fluctuation along the Jialing River, but it was no significant variation among different reaches (Tukey’s HSD test, p > 0.05, Supplementary Figure S2A). A RF model based on the relative abundance of the phytoplanktonic phyla showed a 98.33% accuracy for distinguishing the water from different reaches (Figure 3C). Chrysophyta and Euglenophyta were recognized based on the RF model as the keytaxa for reach differentiation (Figure 3D).
Figure 3. Relative abundance (%) of top 10 abundant phyla for phytoplankton (A) and microzooplankton (B), respectively, along the Jialing River. Accuracy of the random model (C) based on the relative abundances of phytoplanktonic phyla for reach differentiation of water samples and their keytaxa (D). Accuracy of the random model (E) based on the relative abundances of phytoplanktonic phyla for reach differentiation of water samples and their keytaxa (F).
Although the top abundant microzooplanktonic ASVs were belonged to Ciliophora and Stramenopiles (Figure 1C), the most abundant microzooplanktonic phylum was Intramacronucleata (Figure 3B). The relative abundance of Intramacronucleata in the upper reach was significantly lower than that in the middle and lower reaches, while Stramenopiles and Cercozoa were more abundant in the upper reach (Tukey’s HSD test, p < 0.05, Supplementary Figure S2B). The RF model based on the relative abundance of the microzooplanktonic phyla obtained 95% accuracy (Figure 3E), with four phyla (Foraminifera, Intramacronucleata, Kinetoplastea, and Thecofilosea) as the bioindicators (Figure 3F).
Several significant correlations were found between the alpha diversity of phytoplankton with environmental variables (Figure 4A). Particularly, the Chao1 and Pd_faith indices of phytoplankton were negatively correlated to ASL and TN, but positively correlated to WT, EC, and TDS (Spearman correlation, p < 0.05). In addition, negatively correlations were observed between the ASL with Shannon and Pielou_J indices of phytoplankton, while they were positively correlated to EC (Spearman correlation, p < 0.05). For keytaxa of phytoplankton, Chrysophyta was positively correlated to ASL, but negatively correlated to WT, EC, and TDS (Spearman correlation, p < 0.05). In contrast, Euglenophyta was positively correlated to TOC, COD, and TN, but negatively correlated to WT (Spearman correlation, p < 0.05). For microzooplankton, the Chao1 index was found to be positively correlated to WT but negatively correlated to TOC and COD (Spearman correlation, p < 0.05). In addition, significantly positive correlations between the Pielou_J index of microzooplankton with ASL and COD were observed (Spearman correlation, p < 0.05). Moreover, the relative abundances of microzooplankton (Foraminifera and Kinetoplastea) were negatively correlated to WT and SD, but positively correlated to TOC and COD (Spearman correlation, p < 0.05).
Figure 4. (A) Spearman correlations between environmental variables with alpha diversity indices and keytaxa of phytoplankton and microzooplankton. The color of block represents the correlation coefficient. “++” and “+” represent the p-value lower than 0.01 and 0.05, respectively. Distance-decay of similarities between the community similarity of phytoplankton and microzooplankton with environmental similarity (B) or geographic distance (C) between each of two sampling sites. (D) Variation partitioning analysis (VPA) quantitates the contributions of environmental and spatial factors to variations of phytoplankton and microzooplankton, respectively.
Unlike the commonly found linear DDCS, the DDCSs of the phytoplankton and microzooplankton in this study were exponentially correlated with environmental and geographical distances (Figures 4B, C). In addition, the strength of DDCSs to both environmental and geographical distances were slightly higher for phytoplankton than those for microzooplankton (Figures 4B, C). The VPA indicated that spatial effect was stronger than environmental effect on both phytoplankton and microzooplankton (Figure 4D). Moreover, variation of phytoplankton was mainly driven by the interaction of environmental and spatial factors, rather than pure effects of environmental and spatial factors for microzooplankton (Figure 4D).
The DDCS of phytoplankton and microzooplankton in different reaches were further investigated (Supplementary Figure S3). The strength of DDCS between both of phytoplankton and microzooplankton with environmental similarity gradually increased from the upper to lower reaches, while no obvious variations in the strength of DDCS between them with geographic distance (Supplementary Figure S3). These results reflected that the exponential DDCS of environmental similarity along the overall river could be a combination of linear dependency relationships of different reaches, while the exponential DDCS of geographic distance could be its inherent characteristic. In addition, the correlation coefficient of the DDCS between phytoplankton with environmental similarity in the middle reach was weakest, but was highest for DDCS of geographic distance (Supplementary Figure S3A). For microzooplankton, the correlation coefficient in DDCS of environmental similarity and geographic distance were both showed a decreased trend from the upper to lower reaches (Supplementary Figure S3B).
The values of betaNTI indicated that deterministic processes controlled the assembly of phytoplankton and microzooplankton in the Jialing River, and it was stronger for phytoplankton and microzooplankton (Supplementary Figure S4A). Furthermore, heterogeneous selection governed the assembly of phytoplankton (64.58%) and homogenizing dispersal was the most dominant stochastic process (18.59%) (Supplementary Figure S4B). In contrast, heterogeneous selection (35.82%), homogenizing dispersal (32.32%), and drift (30.56%) contributed similar to the assembly of microzooplankton (Supplementary Figure S4B). Based on these results, the contribution of environmental filtering for assembly of phytoplankton and microzooplankton was stronger than that of dispersal, which was also confirmed by the VPA (Figure 5A) and null model (Figure 5B).
Figure 5. (A) Ratio of the pure effects of environmental and spatial factors for variations of phytoplankton and microzooplankton. The pure environmental variation without a spatial component represents the strength of species sorting; the pure spatial variation without an environmental component is interpreted as dispersal limitation. The values for calculation were obtained from the Figure 4D. (B) Ratio of the contributions of deterministic and stochastic processes for assembly of phytoplankton and microzooplankton. (C) Differences in the betaNTI of phytoplankton and microzooplankton among different reaches. (D) Contributions of different ecological processes for assembly of phytoplankton and microzooplankton in different reaches.
The assembly mechanisms of phytoplankton and microzooplankton among different reaches were further explored (Figures 5C, D). In the upper reach, the assembly of phytoplankton and microzooplankton were respectively governed by deterministic (heterogeneous selection) and stochastic (drift) processes. The assembly of both phytoplankton and microzooplankton in the middle reach turned to stochastic-dominated with homogenizing dispersal and drift as the main processes. In the lower reach, the assembly of phytoplankton and microzooplankton further turned to stochastic- (drift) and deterministic- (heterogeneous selection) dominated, respectively.
The complex of co-occurrence network of phytoplankton and microzooplankton gradually decreased from the upper to lower reaches, especially for network in the lower reach, which only contained very few taxa (Figure 6A). The number of phytoplankton nodes in the upper and middle reaches was higher than microzooplankton nodes, but it was lower in the lower reach (Figure 6B). In upper reach, the edge between phytoplankton was the most, followed by interaction between phytoplankton and microzooplankton, and lowest from microzooplankton edges (Figure 6C). In contrast, the most dominated edge was the interaction between phytoplankton and microzooplankton (Figure 6C). Moreover, three connectors (one Bacillariophyta, and two Intramacroucleata) were recognized from the network of the upper and middle reaches (Figure 6D) based on the zi-pi analysis. In contrast, no keystone was observed in the network of the lower reach due to the its limited complexity (Supplementary Figure S5). Finally, the stability of co-occurrence network exhibited a decreased trend from the upper to lower reaches based on the robustness and vulnerability (Figure 6E).
Figure 6. (A) Co-occurrence network of phytoplankton and microzooplankton in different reaches. Statistics of nodes (B) and edges (C) of the co-occurrence networks in different reaches. Green and blue in sub-figure b represent nodes of phytoplankton and microzooplankton, respectively. Green, blue, and orange in sub-figure c represent the edges between two phytoplankton, two microzooplankton, and one phytoplankton and one microzooplankton, respectively. (D) The Zi-Pi plot for co-occurrence networks in the upper and middle reaches. The threshold values of Zi and Pi used for categorizing were 2.5 and 0.62, respectively. (E) Differences in the robustness and vulnerability of co-occurrence networks among different reaches. Different lowercase letters above each box in the same sub- figure. represent significant differences among co-occurrence networks from different reaches (Tukey’s HSD test, p < 0.05).
The variations in the diversity of planktonic microbial communities along a river are influenced by a complex interplay of environmental factors. Among them, climate is one of the key factors influencing phytoplankton diversity in rivers (Rusanov et al., 2022). Temperature have been found to contribute significantly to phytoplankton community changes, with greater temperatures often leading to increased richness and abundance of phytoplankton (Ge et al., 2022). The geographical characteristics of the Jialing River result in an altitude difference of over a kilometer between its downstream and upstream, leading to significant temperature variations. The positive correlations between the alpha diversity of phytoplankton and WT observed in this study (Figure 4A) further supported the important roles of climate on the phytoplankton communities. In addition, the geographical- and climate-driven changes in river flow and nutrient availability can also impact phytoplankton diversity (Gao et al., 2024). Contradicting to the negative relationships between TDS and EC with phytoplankton diversity in rivers (Inyang and Wang, 2020; Khatun and Rashidul Alam, 2020; Mohammed and Mahran, 2022), results of the present study revealed significant positive relationships (Figure 4A). This unique correlation may be influenced by specific human activities in the watershed, warranting further in-depth research to explore its underlying causes. In contrast to the same trend of phytoplankton diversity, the increased richness but decreased evenness of microzooplankton was observed in the lower reach of Jialing River (Figure 2B), indicating few species occupied the most of community. The higher temperature in the lower reach could also be the driver for the increased microzooplankton richness (Figure 4A), while the higher organic carbon concentrations (TOC and DOC) could selectively enrich specific microzooplankton species, such as Foraminifera and Kinetoplastea (Figure 4A). Although there are currently differences in the classification information of some taxa here collectively termed phytoplankton in terms of nutritional types (autotrophic, mixotrophic and heterotrophic), we believe the trend of spatial richness differences between phytoplankton and microplankton species discussed below is still credible, but should be revisited in the future when more information is available. In summary, the variations in phytoplankton and microzooplankton diversity along a river are influenced by a complex interplay of environmental factors. Understanding these factors and their interactions is crucial for effective biological assessments and the management of riverine aquatic ecosystems. Therefore, further in-depth research is needed to explore the processes and mechanisms through which key environmental factors impact the diversity of phytoplankton and microzooplankton at the watershed level.
Typically, planktonic communities can serve as indicators of broader biogeographic patterns and dispersal mechanisms for aquatic organisms (Zhang et al., 2023a). In this study, we have recognized some potential keytaxa that can distinguish the water sourced from different regions of the Jialing River (Figure 3). Especially, two representative phytoplankton phyla, Chrysophyta and Euglenophyta, were found more abundant in the upper reach. Both Chrysophyta and Euglenotohyta play a role in aquatic ecosystems as primary producers through photosynthesis, forming a crucial part of the food web (Modigh and Franzè, 2009). However, they are characterized by the ability to perform both photosynthesis and heterotrophy, resulting they thrive in nutrient-rich freshwater habitats by absorbing organic compounds form their surroundings (Bock et al., 2022; Prokopchuk et al., 2022). These results were further supported by the higher concentration of COD, TOC and TN at the upper reach (Figure 1B) as well as positive correlations between them with Euglenophyta (Figure 4A). On the other hand, microzooplankton are heterotrophic organisms, meaning they consume other organisms for their nutrition (Safi et al., 2023). The dominant keytaxa of microzooplankton, Intramacronucleata, has been reported to be sensitive to changes in their environment, including fluctuations in organic matter levels (Naumova et al., 2023). Specific Intramacronucleata are associated with varying levels of organic pollution, categorized into zones like polysaprobity (very polluted) and oligosaprobity (clean) (Dias et al., 2008). In summary, these findings supported the ecological roles of keytaxa recognized here and their utility as bioindicators in the Jialing River.
Moreover, understanding the spatial patterns and connectivity of planktonic communities can guide the design of conservation efforts aimed at preserving the health and resilience of aquatic ecosystems (Dias et al., 2021). Studies have shown that phytoplankton exhibit a stronger DDCS compared to microzooplankton (Jin et al., 2022; Sun et al., 2023), indicating that environmental factors play a more significant role in shaping phytoplankton communities (Zhang et al., 2023b). In contrast, microzooplankton are generally more resilient to environmental changes and exhibit a lesser DDCS compared to phytoplankton (Anderson and Harvey, 2019). This is because microzooplankton are able to adapt to changing environmental conditions through their ability to migrate and adjust their grazing rates (López-Abbate, 2021). Additionally, microzooplankton are more diverse and can occupy a wider range of ecological niches, making them more resistant to environmental changes (Novotny et al., 2021). The results of this study also obtained similar results with relatively stronger DDCS for phytoplankton compared to microzooplankton in the Jialing River (Figures 4B, C) with more deterministic assembly by heterogeneous selection (Figure 5). In addition, it is necessary to distinguish the relative importance of environmental and spatial factors and their potential interplay in shaping plankton community composition (Zeng et al., 2024). The results showed a more spatial effects on both phytoplankton and microzooplankton in the Jialing River (Figure 4D), which could be associated to the physically block during the river due to the cascade dams (Lehner et al., 2024; Shen et al., 2022; Watters, 1996). In contrast, the obvious higher contribution of the interaction of environmental and spatial factors for phytoplankton assembly (Figure 4D) further indicated that the phytoplankton could more influenced by environmental factors (Ding et al., 2021).
The co-occurrence network of phytoplankton and microzooplankton in rivers is a complex system where the interactions between these two groups of organisms play a crucial role in shaping the dynamics of aquatic ecosystems (Hou et al., 2020). In the Jialing River, the complex and stability of co-occurrence network of phytoplankton and microzooplankton continued to reduce (Figure 6). Especially for the upper and middle reaches, they had similar nodes but obviously lower edges in the middle reach (Figures 6B, C), which turned the network from a phytoplankton-centered to a relatively loose pattern connected by some phytoplankton and microzooplankton (Figure 6D). Phytoplankton, as primary producers, form the base of the aquatic food web, while microzooplankton, as herbivores, feed on phytoplankton and are an essential link between primary production and higher trophic levels (Ger et al., 2019). Thus, microzooplankton play a crucial role in the aquatic food web as both grazers and predators (Zhao et al., 2020). Changes in microzooplankton trophic activities, such as shifts in their grazing preferences or predator-prey interactions, can have significant impacts on the co-occurrence network of phytoplankton and microzooplankton (Xue et al., 2018). Therefore, the top-down control microzooplankton in the co-occurrence network of the middle reach of Jialing River recognized in this study should receive more attention. Future research should focus on developing more comprehensive models that incorporate the complex interactions between phytoplankton, microzooplankton, and other components of the aquatic food web (Osakpolor et al., 2021). Additionally, development of tools to allow for a more detailed classification of nutritional types, and comparative analysis research may also be very important research directions. Such knowledge is essential for predicting how changes in environmental factors might impact the aquatic food web and the overall health of the ecosystem (Giraldo et al., 2024).
Along the whole Jialing River, significant variations in the diversity, compositions, assembly processes, and co-occurrence patterns of plankton microbial communities were observed from the upper to lower reaches. Water temperature was recognized as the main driver for richness of both phytoplankton and microzooplankton. Meanwhile, some reach-related indicator species have been identified and a more stochastic assembly of plankton microbial communities was found in the middle reach. Importantly, the complex and stability of co-occurrence network continued decrease along the Jialing River. The network of phytoplankton and microzooplankton turned to a multi-module pattern in the middle reach from a core-centered pattern in the upper reach, and then highly broken in the lower reach. Furthermore, the distinct assembly mechanisms between phytoplankton and microzooplankton were uncovered with stronger environmental filtering for phytoplankton. However, the deeper drivers of the phytoplankton assembly mechanism may need to be incorporated into more environmental factors, such as land use types, pollutant discharges, etc., to be further explored in depth in the future. In conclusions, these findings help to comprehensively evaluate the health status of the Jialing River and provide important basis for water environmental management and protection.
The original contributions presented in the study are included in the article/Supplementary Material, further inquiries can be directed to the corresponding author.
The animal study was approved by the Ethics Committee of Chongqing Normal University. The study was conducted in accordance with the local legislation and institutional requirements. This study did not involve any live animals. The species information was obtained through the analysis of river water samples collected using environmental DNA methods.
YS: Data curation, Formal analysis, Writing – review & editing. XZ: Data curation, Formal analysis, Writing – original draft. JZ: Data curation, Software, Writing – original draft. QL: Data curation, Software, Writing – original draft. YZ: Data curation, Investigation, Writing – original draft. QZ: Conceptualization, Writing – review & editing.
The author(s) declare financial support was received for the research, authorship, and/or publication of this article. This work was sponsored by the National Natural Science Foundation of China (Grant No. 32202939).
The authors declare that the research was conducted in the absence of any commercial or financial relationships that could be construed as a potential conflict of interest.
All claims expressed in this article are solely those of the authors and do not necessarily represent those of their affiliated organizations, or those of the publisher, the editors and the reviewers. Any product that may be evaluated in this article, or claim that may be made by its manufacturer, is not guaranteed or endorsed by the publisher.
The Supplementary Material for this article can be found online at: https://www.frontiersin.org/articles/10.3389/fmars.2024.1462432/full#supplementary-material
Amaral-Zettler L. A., McCliment E. A., Ducklow H. W., Huse S. M. (2009). A method for studying protistan diversity using massively parallel sequencing of V9 hypervariable regions of small-subunit ribosomal RNA genes. PloS One 4, e6372. doi: 10.1371/journal.pone.0006372
Anderson S. R., Harvey E. L. (2019). Seasonal variability and drivers of microzooplankton grazing and phytoplankton growth in a subtropical estuary. Front. Mar. Sci. 6. doi: 10.3389/fmars.2019.00174
Askeyev A., Askeyev O., Yanybaev N., Askeyev I., Monakhov S., Marić S., et al. (2017). River fish assemblages along an elevation gradient in the eastern extremity of Europe. Environ. Biol. Fishes 100, 585–596. doi: 10.1007/s10641-017-0588-z
Bastian M., Heymann S., Jacomy M. (2009). “Gephi: an open source software for exploring and manipulating networks,” in International AAAI Conference on Weblogs and Social Media: California, USA, vol. 3, 361–362. doi: 10.1609/icwsm.v3i1.13937
Bock C., Olefeld J. L., Vogt J. C., Albach D. C., Boenigk J. (2022). Phylogenetic and functional diversity of Chrysophyceae in inland waters. Organisms. Org. Divers. Evol. 22, 327–341. doi: 10.1007/s13127-022-00554-y
Bokulich N. A., Kaehler B. D., Rideout J. R., Dillon M., Bolyen E., Knight R., et al. (2018). Optimizing taxonomic classification of marker-gene amplicon sequences with qiime 2’s q2-feature-classifier plugin. Environ. Microbiomes 6, 90. doi: 10.1186/s40168-018-0470-z
Bokulich N. A., Subramanian S., Faith J. J., Gevers D., Gordon J. I., Knight R., et al. (2013). Quality-filtering vastly improves diversity estimates from Illumina amplicon sequencing. Nat. Methods 10, 57–59. doi: 10.1038/nmeth.2276
Bonacina L., Fasano F., Mezzanotte V., Fornaroli R. (2023). Effects of water temperature on freshwater macroinvertebrates: A systematic review. Biol. Rev. 98, 191–221. doi: 10.1111/brv.12903
Bruno D., Belmar O., Maire A., Morel A., Dumont B., Datry T. (2019). Structural and functional responses of invertebrate communities to climate change and flow regulation in alpine catchments. Global Change Biol. 25, 1612–1628. doi: 10.1111/gcb.14581
Costa B. N. S., Pinheiro S. C. C., Lima M. D. O., Amado L. L. (2016). Microzooplankton as an indicator of environmental quality at an industrial complex in the Brazilian Amazon. Ecol. Indic 66, 220–229. doi: 10.1016/j.ecolind.2016.01.033
Dias F. S., Betancourt M., Rodríguez-González P. M., Borda-de-Água L., Reports S. (2021). Analysing the distance decay of community similarity in river networks using Bayesian methods. Sci. Rep. 11, 21660. doi: 10.1038/s41598-021-01149-x
Dias R. J. P., Wieloch A. H., D’Agosto M. (2008). The influence of environmental characteristics on the distribution of ciliates (Protozoa, Ciliophora) in an urban stream of southeast Brazil. Braz. J. Biol. 68, 287–295. doi: 10.1590/s1519-69842008000200009
Ding Y., Pan B., Zhao G., Sun C., Han X., Li M. (2021). Geo-climatic factors weaken the effectiveness of phytoplankton diversity as a water quality indicator in a large sediment-laden river. Sci. Total Environ. 792, 148346. doi: 10.1016/j.scitotenv.2021.148346
Gao W., Xiong F., Lu Y., Xin W., Wang H., Feng G., et al. (2024). Water quality and habitat drive phytoplankton taxonomic and functional group patterns in the Yangtze River. Ecol. Processes 13, 11. doi: 10.1186/s13717-024-00489-6
García A. V. D., Mata-Campo M. P., Pla S., Vicente E., Prego R., Frugone-Álvarez M., et al. (2023). Unprecedented recent regional increase in organic carbon and lithogenic fluxes in high altitude Pyrenean lakes. Sci. Rep. 13, 8586. doi: 10.1038/s41598-023-35233-1
Ge F., Ma Z., Chen B., Wang Y., Lu X., An S., et al. (2022). Phytoplankton species diversity patterns and associated driving factors in China’s Jiulong River estuary: roles that nutrients and nutrient ratios play. Front. Mar. Sci. 9. doi: 10.3389/fmars.2022.829285
Ger K. A., Naus-Wiezer S., Meester L. D., Lürling M. (2019). Zooplankton grazing selectivity regulates herbivory and dominance of toxic phytoplankton over multiple prey generations. Limnol. Oceanogr 64, 1214–1227. doi: 10.1002/lno.11108
Giraldo C., Cresson P., Mackenzie K., Fontaine V., Loots C., Delegrange A., et al. (2024). Insights into planktonic food-web dynamics through the lens of size and season. Sci. Rep. 14, 1684. doi: 10.1038/s41598-024-52256-4
Guo C., Jin Z., Guo L., Lu J., Ren S., Zhou Y. (2020). On the cumulative dam impact in the upper Changjiang River: Streamflow and sediment load changes. Catena 184, 104250. doi: 10.1016/j.catena.2019.104250
Gusha M. N. C., Dalu T., Wasserman R. J., McQuaid C. D. (2019). Zooplankton grazing pressure is insufficient for primary producer control under elevated warming and nutrient levels. Sci. Total Environ. 651, 410–418. doi: 10.1016/j.scitotenv.2018.09.132
Hou D., Zhang J., Chen L., Huang P., Zhang G. (2013). Water quality analysis by UV-Vis spectroscopy: A review of methodology and application. Spectrosc. Spect. Anal. 33, 1839–1844. doi: 10.3964/j.issn.1000-0593(2013)07-1839-06
Hou F. R., Zhang H. J., Xie W. J., Zhou X. Y., Zhu X. Y., Zhang D. M. (2020). Co-occurrence patterns and assembly processes of microeukaryotic communities in an early-spring diatom bloom. Sci. Total Environ. 711, 134624. doi: 10.1016/j.scitotenv.2019.134624
Huang R., Chen X., Hu Q., Jiang S., Dong J. (2024). Impacts of altitudinal ecohydrological dynamic changes on water balance under warming climate in a watershed of the Qilian Mountains, China. Sci. Total Environ. 908, 168070. doi: 10.1016/j.scitotenv.2023.168070
Huang L., Wang J., Chen X. (2022). Ecological infrastructure planning of large river basin to promote nature conservation and ecosystem functions. J. Environ. Manage 306, 114482. doi: 10.1016/j.jenvman.2022.114482
Inyang A. I., Wang Y. S. (2020). Phytoplankton diversity and community responses to physicochemical variables in mangrove zones of Guangzhou Province, China. Ecotoxicology 29, 650–668. doi: 10.1007/s10646-020-02209-0
Isabwe A., Yang J. R., Wang Y. M., Liu L. M., Chen H. H., Yang J. (2018). Community assembly processes underlying phytoplankton and bacterioplankton across a hydrologic change in a human-impacted river. Sci. Total Environ. 630, 658–667. doi: 10.1016/j.scitotenv.2018.02.210
Jin L., Chen H. H., Xue Y. Y., Soininen J., Yang J. (2022). The scale-dependence of spatial distribution of reservoir plankton communities in subtropical and tropical China. Sci. Total Environ. 845, 157179. doi: 10.1016/J.SCITOTENV.2022.157179
Khatun M., Rashidul Alam A. K. M. (2020). Phytoplankton assemblage with relation to water quality in Turag River of Bangladesh. Caspian J. Environ. Sci. 18, 31–45. doi: 10.22124/CJES.2020.3977
Körner C. (2007). The use of ‘altitude’ in ecological research. Trends Ecol. Evol. 22, 569–574. doi: 10.1016/j.tree.2007.09.006
Lehner B., Beames P., Mulligan M., Zarfl C., Felice D. L., Soesbergen V. A., et al. (2024). The Global Dam Watch database of river barrier and reservoir information for large-scale applications. Sci. Data. 11, 1069. doi: 10.1038/s41597-024-03752-9
Lobus N. V., Kulikovskiy M. S. (2023). The co-evolution aspects of the biogeochemical role of phytoplankton in aquatic ecosystems: A review. Biol 12, 92. doi: 10.3390/BIOLOGY12010092
López-Abbate M. C. (2021). Microzooplankton communities in a changing ocean: a risk assessment. Divers 13, 82. doi: 10.3390/D13020082
Modigh M., Franzè G. (2009). Changes in phytoplankton and microzooplankton populations during grazing experiments at a Mediterranean coastal site. J. Plankton Res. 31, 853–864. doi: 10.1093/plankt/fbp035
Mohammed K. A., Mahran B. N. (2022). Environmental studies on phytoplankton diversity in drainage water of main drains in Eastern Delta, case study. Egypt. J. Aquat. Res. 48, 211–216. doi: 10.1016/J.EJAR.2021.09.007
Naud L., Måsviken J., Freire S., Angerbjörn A., Dalén L., Dalerum F. (2019). Altitude effects on spatial components of vascular plant diversity in a subarctic mountain tundra. Ecol. Evol. 9, 4783–4795. doi: 10.1002/ece3.5081
Naumova N. B., Barsukov P. A., Baturina O. A., Kabilov M. R. (2023). Soil Alveolata diversity in the undisturbed steppe and wheat agrocenoses under different tillage. Vavilov J. Genet. Breed 27, 703. doi: 10.18699/VJGB-23-81
Novotny A., Zamora-Terol S., Winder M. (2021). DNA metabarcoding reveals trophic niche diversity of micro and mesozooplankton species. Proc. R. Soc B 288, 20210908. doi: 10.1098/RSPB.2021.0908
Osakpolor S. E., Kattwinkel M., Schirmel J., Feckler A., Manfrin A., Schäfer R. B. (2021). Mini-review of process-based food web models and their application in aquatic-terrestrial meta-ecosystems. Ecol. Modell 458, 109710. doi: 10.1016/J.ECOLMODEL.2021.109710
Petruzziello A., Bonacina L., Marazzi F., Zaupa S., Mezzanotte V., Fornaroli R. (2021). Effects of high-altitude reservoirs on the structure and function of lotic ecosystems: a case study in Italy. Hydrobiol 848, 1455–1474. doi: 10.1007/S10750-020-04510-9
Poudel R., Jumpponen A., Schlatter D., Paulitz T., Gardener B. M., Kinkel L., et al. (2016). Microbiome networks: a systems framework for identifying candidate microbial assemblages for disease management. Phytopathology 106, 1083–1096. doi: 10.1094/PHYTO-02-16-0058-FI
Prokopchuk G., Korytář T., Juricová V., Majstorović J., Horák A., Šimek K., et al. (2022). Trophic flexibility of marine diplonemids-switching from osmotrophy to bacterivory. ISME J. 16, 1409–1419. doi: 10.1038/S41396-022-01192-0
Qin Q., Xu J. H. Y., Zhang F. B., He S., Zhou T., Li S. Y., et al. (2023). Length–weight relationships and diversity status of fishes in the midstream of the Jialing River, a tributary of the upper Yangtze River, China. Divers 15, 561. doi: 10.3390/D15040561
Ren L. P., Zhang Z., Zeng X., Ma Y. H., Zeng Y., Zhou C. Q. (2011). Community structure of zooplankton and water quality assessment of Jialing River in Nan Chong. Proc. Environ. Sci. 10, 1321–1326. doi: 10.1016/j.proenv.2011.09.211
Rusanov A. G., Bíró T., Kiss K. T., Buczkó K., Grigorszky I., Hidas A., et al. (2022). Relative importance of climate and spatial processes in shaping species composition, functional structure and beta diversity of phytoplankton in a large river. Sci. Total Environ. 807, 150891. doi: 10.1016/J.SCITOTENV.2021.150891
Sabater S., Freixa A., Jiménez L., López-Doval J., Pace G., Pascoal C., et al. (2023). Extreme weather events threaten biodiversity and functions of river ecosystems: evidence from a meta-analysis. Biol. Rev. 98, 450–461. doi: 10.1111/BRV.12914
Safi K. A., Rodríguez A. G., Hall J. A., Pinkerton M. H. (2023). Phytoplankton dynamics, growth and microzooplankton grazing across the subtropical frontal zone, east of New Zealand. Deep Sea Res. Part II. 208, 105271. doi: 10.1016/j.dsr2.2023.105271
Shao Y. T., He Y., Mu X. M., Zhao G. J., Gao P., Sun W. Y. (2021). Contributions of climate change and human activities to runoff and sediment discharge reductions in the Jialing River, a main tributary of the upper Yangtze River, China. Theor. Appl. Climatol. 145, 1437–1450. doi: 10.1007/s00704-021-03682-1
Shen J. C., Qin G., Gu X., Liu Y., An S. Q., Liu R., et al. (2022). Effects of seasonal hydrological regulation of cascade dams on the functional diversity of zooplankton: Implications for the management of massive reservoirs and dams. J. Hydrol. 610, 127825. doi: 10.1016/j.jhydrol.2022.127825
Shen Y. J., Zhang Y. F., Zhou X. X., Li Q. H., Zhang J. M., Cheng R. L., et al. (2024). Environmental DNA metabarcoding revealing the distinct responses of phytoplankton and zooplankton to cascade dams along a river-way. Ecol. Ind. 166, 112545. doi: 10.1016/j.ecolind.2024.112545
Singh U. B., Ahluwalia A. S., Sharma C., Jindal R., Thakur R. K. (2013). Planktonic indicators: A promising tool for monitoring water quality (early-warning signals). Ecol. Environ. Conserv. 19, 793–800. Available at: https://www.researchgate.net/publication/261983329 (Accessed April 18, 2024).
Stegen J. C., Lin X. J., Konopka A. E., Fredrickson J. K. (2012). Stochastic and deterministic assembly processes in subsurface microbial communities. ISME J. 6, 1653–1664. doi: 10.1038/ismej.2012.22
Steinhauser D., Krall L., Müssig C., Büssis D., Usadel B. (2008). “Correlation networks,” in Analysis of biological networks (John Wiley & Sons, New York), 305–333.
Sun Y., Li H. J., Wang X. C., Jin Y., Nagai S., Lin S. J. (2023). Phytoplankton and microzooplankton community structure and assembly mechanisms in northwestern Pacific Ocean estuaries with environmental heterogeneity and geographic segregation. Microbiol. Spectrum. 11, e04926–e04922. doi: 10.1128/spectrum.04926-22
Wang H. X., Ma Y. C., Yang H., Hong F. T., Guo W. X. (2023). Quantitative evaluation of the impact of climate change and human activities on Jialing river runoff changes in the past 60 years, China. J. Water Clim. Change. 14, 590–609. doi: 10.2166/wcc.2023.452
Watters G. T. (1996). Small dams as barriers to freshwater mussels (Bivalvia, Unionoida) and their hosts. Biol. Conserv. 75, 79–85. doi: 10.1016/0006-3207(95)00034-8
Xue Y. Y., Chen H. H., Yang J. R., Liu M., Huang B. Q., Yang J. (2018). Distinct patterns and processes of abundant and rare eukaryotic plankton communities following a reservoir cyanobacterial bloom. ISME J. 12, 2263–2277. doi: 10.1038/s41396-018-0159-0
Yang Y. Z., Xia W. M., Yang J., Wang T., Ma W. P. (2011). The research of methods of measuring chlorophyll a in water. Environ. Monit. China. 27, 24–27. doi: 10.19316/j.issn.1002-6002.2011.05.006
Yang M., Zhang S., Liu S. R. (2015). Phytoplankton community structure and water quality assessment in Jialing River after the impoundment of Caojie Reservoir. Environ. Sci. 36, 2480–2486. doi: 10.13227/j.hjkx.2015.07.021
Yang N., Zhang C., Wang L. Q., Li Y., Zhang W. L., Niu L. H., et al. (2021). Nitrogen cycling processes and the role of multi-trophic microbiota in dam-induced river-reservoir systems. Water Res. 206, 117730. doi: 10.1016/j.watres.2021.117730
Yilmaz P., Parfrey L. W., Yarza P., Gerken J., Pruesse E., Quast C., et al. (2014). The SILVA and “all-species living tree project (LTP)” taxonomic frameworks. Nucleic Acids Res. 42, D643–D648. doi: 10.1093/nar/gkt1209
Yuan M. M., Guo X., Wu L. W., Zhang Y. a., Xiao N. J., Ning D. L., et al. (2021). Climate warming enhances microbial network complexity and stability. Nat. Clim. Change 11, 343–348. doi: 10.1038/s41558-021-00989-9
Zeng L. P., Wen J., Huang B. J., Yang Y., Huang Z. W., Zeng F. T., et al. (2024). Environmental DNA metabarcoding reveals the effect of environmental selection on phytoplankton community structure along a subtropical river. Environ. Res. 243, 117708. doi: 10.1016/j.envres.2023.117708
Zhang G. C., Liu Z. S., Zhang Z. Y., Ding C. L., Sun J. (2023b). The impact of environmental factors on the phytoplankton communities in the Western Pacific Ocean: HPLC-CHEMTAX approach. Front. Mar. Sci. 10. doi: 10.3389/fmars.2023.1185939
Zhang Y., Qu Z. S., Li J. Q., Hu T., Chen C. Z., Lin X. F. (2023a). From river to ocean: Connectivity and heterogeneity of aquatic ecosystems depicted by planktonic microeukaryotes. Ecol. Indic. 148, 110136. doi: 10.1016/j.ecolind.2023.110136
Zhao Y., Dong Y., Li H. B., Lin S. Q., Huang L. F., Xiao T., et al. (2020). Grazing by microzooplankton and copepods on the microbial food web in spring in the southern Yellow Sea, China. Mar. Life Sci. Technol. 2, 442–455. doi: 10.1007/s42995-020-00047-x
Zhao Z. L., Li H. J., Sun Y., Shao K. S., Wang X. C., Ma X. D., et al. (2022a). How habitat heterogeneity shapes bacterial and protistan communities in temperate coastal areas near estuaries. Environ. Microbiol. 24, 1775–1789. doi: 10.1111/1462-2920.15892
Zhao Z. L., Li H. J., Sun Y., Zhan A. B., Lan W. L., Woo S. P., et al. (2022b). Bacteria versus fungi for predicting anthropogenic pollution in subtropical coastal sediments: assembly process and environmental response. Ecol. Indic. 134, 108484. doi: 10.1016/j.ecolind.2021.108484
Keywords: distance-decay of similarity, ecosystem stability, environmental DNA, environmental filtering, river ecosystem, temperature
Citation: Shen Y, Zhou X, Zhang J, Li Q, Zhang Y and Zuo Q (2024) Insights into the effects of environmental factors on phytoplankton and microzooplankton at a basin scale: diversity, assembly mechanisms, and co-occurrence networks. Front. Mar. Sci. 11:1462432. doi: 10.3389/fmars.2024.1462432
Received: 10 July 2024; Accepted: 29 November 2024;
Published: 20 December 2024.
Edited by:
Jin Zhou, Tsinghua University, ChinaReviewed by:
Si Tang, Tsinghua University, ChinaCopyright © 2024 Shen, Zhou, Zhang, Li, Zhang and Zuo. This is an open-access article distributed under the terms of the Creative Commons Attribution License (CC BY). The use, distribution or reproduction in other forums is permitted, provided the original author(s) and the copyright owner(s) are credited and that the original publication in this journal is cited, in accordance with accepted academic practice. No use, distribution or reproduction is permitted which does not comply with these terms.
*Correspondence: Yanjun Shen, c2hlbnlhbmp1bkBjcW51LmVkdS5jbg==; Qing Zuo, enVvcWluZzEwMUAxNjMuY29t
Disclaimer: All claims expressed in this article are solely those of the authors and do not necessarily represent those of their affiliated organizations, or those of the publisher, the editors and the reviewers. Any product that may be evaluated in this article or claim that may be made by its manufacturer is not guaranteed or endorsed by the publisher.
Research integrity at Frontiers
Learn more about the work of our research integrity team to safeguard the quality of each article we publish.