- Shanghai Key Laboratory for Molecular Engineering of Chiral Drugs, School of Pharmacy, Shanghai Jiao Tong University, Shanghai, China
The rise of bacterial infections and increasing antibiotic resistance underscores an urgent need for new, effective antimicrobial agents with marine bacteria offering a unique and promising source for novel antibiotic compounds to combat persistent and emerging pathogens. In this research, five compounds were achieved from marine Streptomyces sp., C2-13, and their yield was enhanced with the addition of zinc sulfate at 0.5 mM. All compounds have been evaluated for their antibacterial activity against multiple pathogens, among which good activity was achieved against S. aureus, while potent activity was achieved against Riemerella anatipestifer with its IC50 value at 200 µm and bactericidal effect at 300 µm. Among all compounds, 4 was more active against both pathogens. A transcriptome analysis of active compound 4 showed its antibacterial effect on R. anatipestifer by inhibiting 30S and 50S ribosomal subunits, resistance mechanisms, and gliding motility proteins IX secretion system (T9SS) and interfering with protein translations process, secretion system, defense and resistance mechanisms, ultimately resulting in effective inhibition of normal bacterial growth and its motility. To investigate the anti-bacterial mechanism, all compounds were docked with two enzymes and TLR4 protein for predicting the vaccine construct, and the best docking score was achieved against RMFP with the highest score of -12.9 for compound 4. In silico cloning was carried out to ensure the expression of proteins generated and were cloned using E. coli as a host. The simulation studies have shown that both compound 4–RMFP and TLR4–RMFP complex are stable with the system. To the best of our knowledge, this is the first study investigating marine bacterial metabolites against R. anatipestifer with their anti-bacterial mechanism and enhancing their yield through the addition of zinc sulfate ions.
1 Introduction
Evolved over eons to encode nature’s own schematics, natural products have long been the bedrock of medicinal discovery, offering an enduring dawn for drug innovation. Marine environments are a rich reservoir of unique bioactive compounds with vast potential for pharmaceutical applications, particularly in antibiotic discovery (ul Hassan et al., 2022). Among these marine-derived compounds, those produced by marine bacteria have drawn significant attention for their potent antimicrobial properties (Carroll et al., 2024). Marine bacteria, especially Streptomyces species, are known for their exceptional biosynthetic abilities, creating diverse secondary metabolites with unique structural features and potent biological activities. The genus Streptomyces, well established as a major source of antibiotics in terrestrial environments, has demonstrated even greater chemical diversity in marine habitats, where adaptations to extreme conditions yield novel compounds not found elsewhere. This adaptability has led to the discovery of various antibiotics with activity against multidrug-resistant bacteria, offering promising solutions to combat the rising threat of antibiotic resistance. Research on marine-derived Streptomyces has revealed complex molecules with distinct mechanisms of action, often targeting bacterial pathways that differ from those affected by conventional antibiotics (Pulat et al., 2024).
Marine microorganisms exhibit diverse responses to heavy metals, adapting to high concentrations of these elements often found in marine environments. Exposure to heavy metals like zinc, copper, and cadmium can act as stressors, triggering defensive mechanisms in marine microbes that lead to enhanced production of secondary metabolites (Shams et al., 2022). These bioactive compounds, including antibiotics, pigments, and antioxidants, play crucial roles in microbial survival, offering protection against oxidative stress and cellular damage caused by metal ions. Interestingly, the presence of heavy metals stimulates complex biosynthetic pathways, leading to the synthesis of novel metabolites that may hold significant pharmacological potential, particularly in drug discovery and biotechnological applications (Ul Hassan et al., 2021).
Bacterial infections remain a serious health concern, with Staphylococcus aureus and Riemerella anatipestifer among the pathogens responsible for significant morbidity and economic losses. S. aureus, notorious for its role in hospital-acquired infections, exhibits high resistance to multiple antibiotics, making it particularly challenging to treat (Baindara et al., 2024; Yang et al., 2024). Similarly, R. anatipestifer impacts poultry, leading to high mortality rates and affecting the agricultural industry (Yang et al., 2024). The rising resistance in these pathogens intensifies the need for new antibiotics, driving interest toward natural products from marine sources.
Omics technologies have transformed drug discovery by enabling comprehensive analyses of biological systems at molecular levels. Among these, transcriptomics studies the complete set of RNA transcripts in cells, revealing gene expression patterns and uncovering potential drug targets (Wu et al., 2024). Complementing this, chemoinformatics uses computational tools to model and predict chemical interactions, optimizing compound selection and reducing experimental workload. Molecular docking further enhances these efforts by simulating the binding of small molecules to target proteins, allowing researchers to predict drug efficacy and specificity. These approaches together streamline drug development, accelerating the discovery of safe and effective therapeutics for various diseases (Khan et al., 2022).
In our research, we have isolated five new antibiotics from marine Streptomyces sp. The compounds’ production was enhanced by adding different concentrations of zinc sulfate in the medium. The antibacterial activity of all the compounds was tested in vitro against multiple gram-positive and gram-negative pathogens. Transcriptomic analysis was performed for the best compound to evaluate its in-depth mechanism behind anti-bacterial activity and further chemoinformatics approach targeting molecular docking, and simulations were also performed to determine the best compounds.
2 Materials and methods
2.1 General
High-performance liquid chromatography (HPLC) system was used with a set of Waters 996 Photodiode Array Detector and Waters 717 plus Autosampler (SHIMADZU LC-2010AHT, 1290 infinity II HDR-DAD Agilent), U.V. (SHIMADZU UV-2550). On a Bruker AVANCE DRX 500 NMR spectrometer with TMS as an internal standard, 1H NMR (500 MHz), 13C NMR (125 MHz), and DEPT (135) spectra were measured at 25°C. The heavy metals were purchased from Sangon Biotech, Shanghai, China.
2.2 Soil sample collection
The marine sediment samples were procured from locations near Ningbo City, Fenghua, in Zhejiang sea shores, approximately 100–200 m inland. These samples were gathered from both polluted and unpolluted sites along the coast. After collection, immediate isolation work began in the lab, with the remaining samples stored at 4°C in a refrigerator.
2.3 Isolation and storage of strains
In this study, a 10-1 dilution of a fresh soil sample labeled C2, weighing approximately 2 to 3 g, was prepared by adding simulated seawater to pre-sterilized glass vials. The mixture underwent 1 min of sonication to detach microorganisms from the soil particles, followed by 15 min of shaking at room temperature. Subsequently, serial dilutions up to 10-2, 10-3, and 10-4 were made, and nystatin (0.05 g/L) was added to the pre-prepared ISP2 medium to inhibit fungal growth. Aliquots of 100 μL from each dilution were inoculated onto the medium and spread with sterilized spreaders. The plates were then incubated at 28°C for 2 to 3 weeks, and purified colonies of bacteria were collected and stored on agar media at 4°C.
2.4 Molecular characterization
DNA extraction was performed using the Omega Mag-Bind Soil DNA kit (M5635-02), following the manufacturer’s instructions. Subsequently, the concentration and purity of the DNA were evaluated using electrophoresis on 1% agarose gel. The V3–V4 variable region of the 16S rDNA gene was amplified using the universal primers 338F (5′-ACTCCTACGGGAGGCAGCA-3′) and 806R (5′-GGACTACHVGGGTWTCTAAT-3′) [9] and paired-end sequenced at Sangon Biotech (Shanghai).
2.5 Metal-induced stress conditions and standard cultivation
The growth of the bacterial strains, C2-13, was tested with and without heavy metals in 500-mL Erlenmeyer flasks containing 250 mL ISP media and maintained at 28°C on a rotatory shaker operating at 180 rpm for a duration of 14 days. As a control, two flasks underwent standard batch growth of the variants as mentioned above to ensure the accuracy of the results. The heavy-metal-inoculated medium for C2-13 was supplemented with different heavy metals, including zinc sulfate, cupper chloride, nickel chloride, and cobalt chloride with initial concentrations of 0.5–4 mM, respectively.
2.6 HPLC analysis and purification of heavy-metal-induced metabolites
Reversed-phase HPLC-UV analysis was conducted using an HPLC-dad system equipped with a C18 column to isolate the secondary metabolites. The HPLC method employed wavelengths of 210 and 254 nm. The gradient elution consisted of a mixture of H2O and MeOH, ranging from 10% to 100% MeOH over 0 to 30 min, followed by 100% MeOH from 30 to 40 min. The flow rate was maintained at 0.1 mL/min throughout the analysis. Subsequently, the stress-induced metabolite was isolated using purification-HPLC, operated at a constant flow rate of 10 mL/min with a consistent mobile phase composition.
2.7 Extraction and isolation
Ethyl acetate (EtOAc) was used to extract the 40-L fermentation broth of C2-13. After evaporating the solvent, the resulting crude extract was dissolved in methanol and centrifuged at 120,000 rpm for 15 min prior to analytical HPLC analysis. During the initial screening, HPLC analysis was carried out using a gradient of H2O and MeOH, increasing from 10% to 100% over 30 min. The target compounds were then purified using preparative HPLC, employing a constant mobile phase of a selected methanol–water mixture. For structural characterization, the purified compound was subjected to 1D NMR analysis, including ¹H NMR, ¹³C NMR, and DEPT135.
2.8 Antibacterial assay
The antibacterial assay of all metabolites was initially evaluated against 10 pathogenic gram-positive and gram-negative bacteria, among which best activity was achieved against two pathogens including S. aureus ATCC29213 and Riemerella anatipestifer WH59 (clinical strain). The bacterial suspension was shaken to the logarithmic growth phase and diluted to 0.5 McFarland standard, with an OD value of approximately 0.1. The compound concentration was 10 mg/mL, and the experimental compound concentration was 400 μg/mL with 8 μL of the compound added. First, 92 μL of MH broth was added to each well of a 96-well plate, followed by 8 μL of the compound in order. Finally, 100 μL of the diluted bacterial suspension was added and mixed well. Each strain was tested in duplicate with each compound. The plate was incubated at 37°C for approximately 24 h in an incubator. The absorbance was measured at 600 nm using an ELISA reader. The positive control drug was ciprofloxacin with a concentration of 6 μg/mL.
2.9 Transcriptome analysis
2.9.1 RNA sequencing
RA bacterial cells were treated with compound 4, followed by a subsequent collection for transcriptomic analysis. Following an incubation period of 60 min at 37°C, the cells were centrifugated. Total RNA extraction, removal of ribosomal RNA, cDNA synthesis, RNA library preparation, transcriptome sequencing, and bioinformatic data analysis were conducted by employing the Illumina HiSeq platform.
2.9.2 DEGs screening and bioinformatics analysis
The procedure for DEGs screening and bioinformatics analysis was performed similarly as in our recent article with slight changes (Wu et al., 2024).
2.10 Molecular docking
2.10.1 Ligand and protein preparation
The ligand and proteins were prepared using the same procedure as per our recent article with slight changes (Wu et al., 2024). The docking studies of all compounds were carried out through the PyRX software. For anti-bacterial potential against R. anatipestifer, two proteins were selected from Uniprot as tRNA N6-adenosine threonylcarbamoyltransferase Q9F0V0 and RIEAN Multifunctional fusion protein. As in PDB, no data was available for this pathogen.
2.10.2 Protein–protein docking
Human Toll-like receptors (TLRs), including the three-dimensional structure of TLR4, were obtained from the Protein Data Bank (PDB ID: 2z65). Refinement of the receptor model was carried out using the Galaxy refine server, with subsequent removal or exclusion of any ligands associated with the retrieved structures. Molecular docking analysis was conducted using the HADDOCK 2.0 protein–protein docking server to explore the interaction profile between the vaccine and TLRs. This server generates cluster scores based on rigid docking, aiming to minimize pairwise RMSD energy between conformations. The final version of the vaccine–TLRs complex model was selected based on the lowest energy weight score and its constituents. Subsequently, this model was visualized using the Chimera 1.10.1 system.
2.10.3 In silico cloning and codon optimization
Codon optimization is a technique used to enhance the efficiency of translating foreign genes in a host organism when there are differences in codon usage between the two organisms. Codon optimization was carried out, followed by in silico cloning, after the careful evaluation of RIEAN Multifunctional fusion protein properties and immune response. To make this method consistent with the commonly used prokaryotic expression system, E. coli K12, the java codon adaptation tool (http://www.jcat.de/) was used for codon optimization. The sticky ends of HindIII and BamHI restriction enzymes were incorporated to facilitate restriction and cloning at the modified target sequence’s start/N terminal and end/C terminal, respectively. Additionally, the modified nucleotide sequence of the target sequence was cloned into the S. aureus strain USA300 pET30a (+)-Tev and pCC1 vectors using the SnapGene tool (https://www.snapgene.com/) to ensure its in vitro expression.
2.11 Molecular docking simulations
Molecular dynamics simulation (MDS) analysis was used with the same protocol as in our previous articles (Muhammad et al., 2024; Wu et al., 2024) with slight modifications.
3 Results
3.1 Molecular characterization of C2-13
The molecular characterization of the bacterial strain C2-13 was characterized by Sangon Biotech (Shanghai). Initially, agarose gel electrophoresis of a PCR-amplified bacterial strain was performed (Supplementary Figure S1). After that, according to 16S rDNA sequencing, multiple phyla were initially detected in the strain, and a single-sample multi-level species composition map visually displayed the annotation results of the strain C2-13 at the taxonomic level (Figure 1A). Based on the taxonomic comparison results of C2-13, only the classification of dominant species were selected. The genus post-hoc of actinobacteria was with 95% confidence (Figure 1B) and the final genus was selected as Streptomyces sp. (Figure 1C). The phylogenetic tree is mentioned in Figure 1.
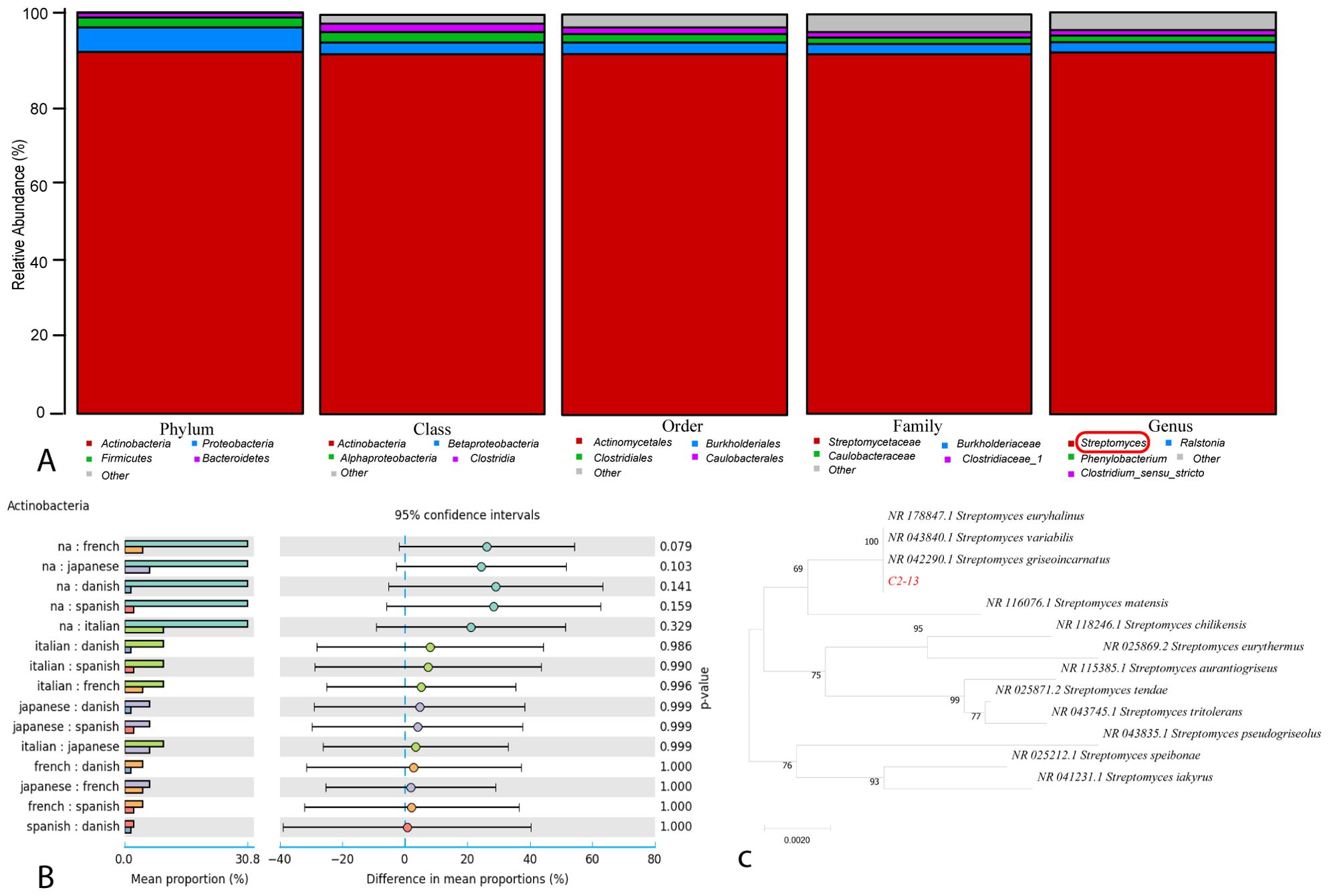
Figure 1. (A) Relative abundance of bacterial strain C2-13 with regards to phylum, class, order, family, and genus. (B) Genus_ postHoc of actinobacteria. (C) Phylogenetic tree of C2-13.
3.2 Zinc-sulfate-induced and standard cultivation through HPLC
The impacts of elution mode, mobile phase, detection wavelength, and column temperature were conducted to determine the ideal HPLC setting for purification of the metal-induced metabolites of C2-13. The C2-13 strain was cultured in the normal and zinc-sulfate-fermented medium, and based on the previous literature search and studies, we selected four initial elicitors premised on their previous ability to produce secondary metabolites in microbes (zinc sulfate, copper chloride, nickel chloride, and cobalt chloride). All four metals with their four initial concentrations of 0.5, 1, 2, and 4 mM were chosen as initial elicitors to evaluate the bacterial strain C2-13 potential against these metals in ISP medium at 180 rpm for 14 days. The alterations in the metabolic profile after subjecting the metal ions were validated by establishing one medium without metal ions as a blank, one medium without bacteria as a metal control, and four groups of media with distinct ionic concentrations (0.5 to 4 mM). Except zinc sulfate, none of the heavy metals showed any kind of peak alterations. The best culture condition was selected as 1 mM for bulk culture of 40 L (Figure 2A). During the addition of zinc sulfate, five peaks were elicited at all concentrations at different retention times which were available in a minute quantity in the normal culture and also declined at other concentrations (Figure 2A). A total number of five zinc-sulfate-induced compounds were purified and identified as compound 1 (tR = 14.6 min), compound 2 (tR = 15.3 min), compound 3 (tR = 17.1 min), n-octacos-9-enyl propionate (compound 4; tR = 20.2 min), and 9-octadecenoic acid (compound 5; tR = 25.7 min) (Figure 2B).
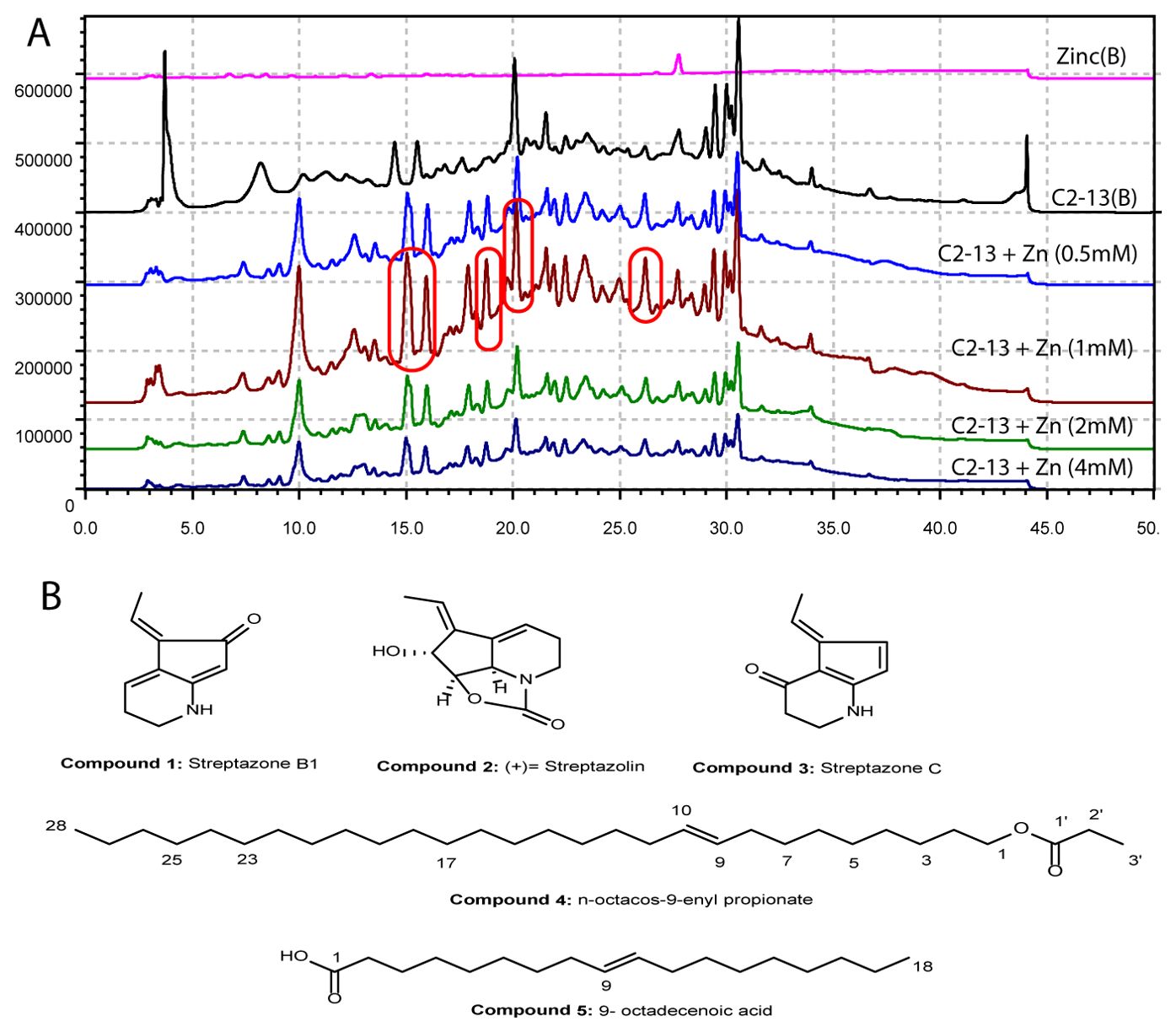
Figure 2. (A) HPLC chromatogram of zinc-sulfate-induced C2-13 strain compounds. (B) Chemical structures of the compounds.
3.3 Characterization of compounds
• Compound 1
Compound 1 was isolated as a colorless oil. The molecular formula C10H11NO was established by ESI-MS m/z 184 [M+Na] +. 1H NMR (CD3OD-d4, 700 MHz): The proton NMR showed a total number of six signals at δH 6.31 (1H, td, J = 4.4, 1.3 Hz, H-4), δH 6.25 (1H, q, J = 7.5 Hz, H-8), δH 5.18 (1H, s, H-7), δH 3.37 (2H, m, H-2), δH 2.48 (2H, td, J = 7.0, 4.4 Hz, H-3), δH 2.23 (3H, d, J = 7.5 Hz, H-9). 13C NMR (CD3OD-d4, 176 MHz): The 13C NMR spectrum showed the presence of a total of 10 signals, including one carbonyl signal at δC 193.9 (C-6), three quaternary carbons at δC 164.5 (C-7a), δC 131.1 (C-4a), and δC 130.9 (C-5), three methane carbons at δC 125.3 (C-8), δC 117.7(C-4), and δC 101.0 (C-7), two methylene signals at δC 38.5 (C-2) and δC 22.7 (C-3), and one methyl signal at δC 12.1 (C-9) (Supplementary Table S1). From the NMR data, compound 1 was identified as a known compound streptazone B1 (Puder et al., 2000) (Figure 2; Supplementary Figures S2–S5).
• Compound 2
Compound 2 was isolated as a light yellow oil. The molecular formula C11H13 NO3 was established by ESI-MS m/z 230 [M+Na] +.1H NMR (CD3OD-d4, 700 MHz): The proton NMR showed a total number of eight signals at δH 6.21 (1H, q, J = 7.3 Hz, H-10), δH 6.09 (1H, dt, J = 6.7, 2.9 Hz, H-9), δH 4.73 (1H, s, H-6), δH 4.69 (1H, d, J = 6.7 Hz, H-2), δH 4.37 (1H, d, J = 6.8 Hz, H-3), δH 3.59 (2H, m, H-7), δH 2.48 (1H, dtd, J = 17.2, 7.2, 3.2 Hz, H-8a), δH 2.20 (1H, m, H-8b), and δH 1.88 (3H, d, J = 6.8 Hz, H-11). 13C NMR (CD3OD-d4, 176 MHz): The 13C NMR spectrum showed the presence of 11 signals, including three quaternary carbons at δC 160.2 (C-1), δC 143.3 (C-5), and δC 138.4 (C-4). DEPT135 shows a total number of eight signals, including one methyl (CH3) group at δC 13.6 (C-11), five methine (CH) group at δC 123.1 (C-10), δC 117.7 (C-9), δC 82.5 (C-2), δC 73.7 (C-3), and δC 58.9(C-6), and two methylene (CH2) groups at δC 39.6 (C-7) and δC 22.2 (C-8) (Supplementary Table S2). From the NMR data, stress metabolite compound 2 was identified as a known compound (+)-streptazolin (Mothana et al., 2022) (Figure 2; Supplementary Figures S6–S9).
• Compound 3
Compound 3 was isolated as a colorless oil. The molecular formula C10H11NO was established by ESI-MS m/z 184 [M+Na] +. 1H NMR (CD3OD-d4, 700 MHz): The proton NMR showed a total number of six signals at δH 7.21 (1H, d, J = 5.5 Hz, H-6), δH 6.87 (1H, q, J = 7.6 Hz, H-8), δH 6.29 (1H, m, H-7), δH 3.59 (2H, m, H-2), δH 2.40 (2H, t, J = 7.7 Hz, H-3), and δH 2.05 (3H, d, J = 7.6 Hz, H-9). 13C NMR (CD3OD-d4, 176 MHz): The 13C NMR spectrum showed the presence of a total of 10 signals, including one carbonyl signal at δC 185.6 (C-4), two quaternary carbons at δC 168.9 (C-7a) and δC 102.4 (C-4a), four methine carbons at δC 139.3 (C-5), δC 134.7 (C-6), δC 129.6 (C-8), and δC 122.8 (C-7), two methylene signals at δC 41.9 (C-2) and δC 35.4 (C-3), and one methyl signal at δC 14.3 (C-9) (Supplementary Table S3). From the NMR data, stress metabolite compound 3 was identified as a known compound streptazone C (Puder et al., 2000) (Figure 2; Supplementary Figures S10–S13).
• Compound 4
Compound 4 was isolated as a light yellow oily liquid. The molecular formula C31H60O2 was established by ESI-MS m/z 487 [M+Na] +. 1H NMR (CD3OD-d4, 700 MHz) showed a total number of seven signals, of which one signal δ5.34 (2H, t, J = 4.9 Hz, H-9, H-10) was for vinylic hydrogens, one signal was for oxygenated methylene at δH 3.66 (2H, m, H-1), δH 2.19 (6H, m, H-2’, H-11, H-8), δH 2.03 (4H, dd, J = 7.6, 4.3 Hz, H-2, H-3), δH 1.60 (4H, m, H-7, H-12), δH 1.31 (36H, m, H-4,H-5, H-6, H-13-27), and δH 0.90 (6H, t, J = 6.8 Hz, H-28, H-3’). 13C NMR (CD3OD-d4, 176 MHz): The 13C NMR spectrum showed the presence of 23 signals, of which there was one signal for ester carbon at δC 177.9 (C-1′), one oxygenated methylene carbon at δC 64.6 (C-1), vinylic carbons at δC 129.5 (C-9, C-10), methylene carbons at δC 35.1 (C-2′), δC 31.7 (C-2), δC 29.4 (C-11), δC 29.4 (C-8), δC 29.4 (C-6), δC 29.3 (C-3, C-7), δC 29.3 (C-4, C-5), δC 29.2 (C-25), δC 29.2 (C-26, C-12), δC 29.1 (C-13), δC 29.0 (C-15), δC 28.9 (C-14), δC 28.9 (C-16, C-17), δC 28.9 (C-18, C-20), δC 28.8 (C-19), δC 26.7 (C-21, C-23), δC 26.7 (C-22), δC 25.5 (C-24), and δC 22.3 (C-27), and one methyl carbon at δC 13.0 (C-28, C-3′) (Supplementary Table S4). From the NMR data, stress metabolite compound 4 was identified as a known compound n-octacos-9-enyl propionate (Chung et al., 2007) (Figure 2; Supplementary Figures S14–S17).
• Compound 5
Compound 5 was isolated as a light yellow oily liquid. The molecular formula C18H34O2 was established by ESI-MS m/z 305 [M+Na] +. 1H NMR (CD3OD-d4, 700 MHz) showed a total number of eight signals at δH 5.34 (1H, t, J = 4.9 Hz, H-9), δH 3.65 (1H, m, H-10), δH 2.31 (2H, m, H-2), δH 2.19 (2H, t, J = 7.6 Hz, H-3), δH 2.03 (2H, dd, J = 7.7, 4.3 Hz, H-11), δH 1.60 (2H, t, J = 7.6 Hz, H-5), δH 1.33 (10H, m, H-13, H-14, H-15, H-16, H-17), δH 1.29 (10H, m, H-12, H-4, H-6, H-7, H-8), and δH 0.90 (3H, t, J = 6.9 Hz, H-18). 13C NMR (CD3OD-d4, 176 MHz): The 13C NMR and DEPT135 spectrum showed the presence of 16 signals, of which there was one carboxyl signal at δC 177.9 (C-1), two ethylene signals at δC 129.5 (C-9) and δC 129.4 (C-10), 14 methylene signals showing the presence of aliphatic groups at δC 35.1 (C-4), δC 31.7 (C-2), δC 29.4 (C-13), δC 29.4 (C-5, C-6), δC 29.2 (C-7), δC 29.0 (C-12), δC 28.9 (C-15), δC 28.9 (C-16), δC 28.8 (C-8, C-11), δC 26.7 (C-17), δC 25.5 (C-3), and δC 22.3 (C-14), and one methyl signal at δC 13.0 (C-18) (Supplementary Table S5). From the NMR data, stress metabolite compound 5 was identified as a known compound 9-octadecenoic acid (Figure 2; Supplementary Figures S18–S21).
3.4 Omics analysis for C2-13 strain
3.4.1 COG analysis
COG (Clusters of Orthologous Groups) analysis predicts gene functions by categorizing genes into functional groups, enabling insights into microbial metabolic pathways and adaptive responses under specific conditions, such as heavy metal exposure (Tatusov et al., 2003). In our results (Figure 3A), we have seen some responsible COG catalogs for heavy metals (Supplementary Table S6). As heavy metals can indeed influence secondary metabolite production in bacteria, this response is often a survival strategy where bacteria produce bioactive compounds as part of their defense against the stress caused by toxic metal exposure. Here is how the listed categories contribute to this process (Figure 3A).
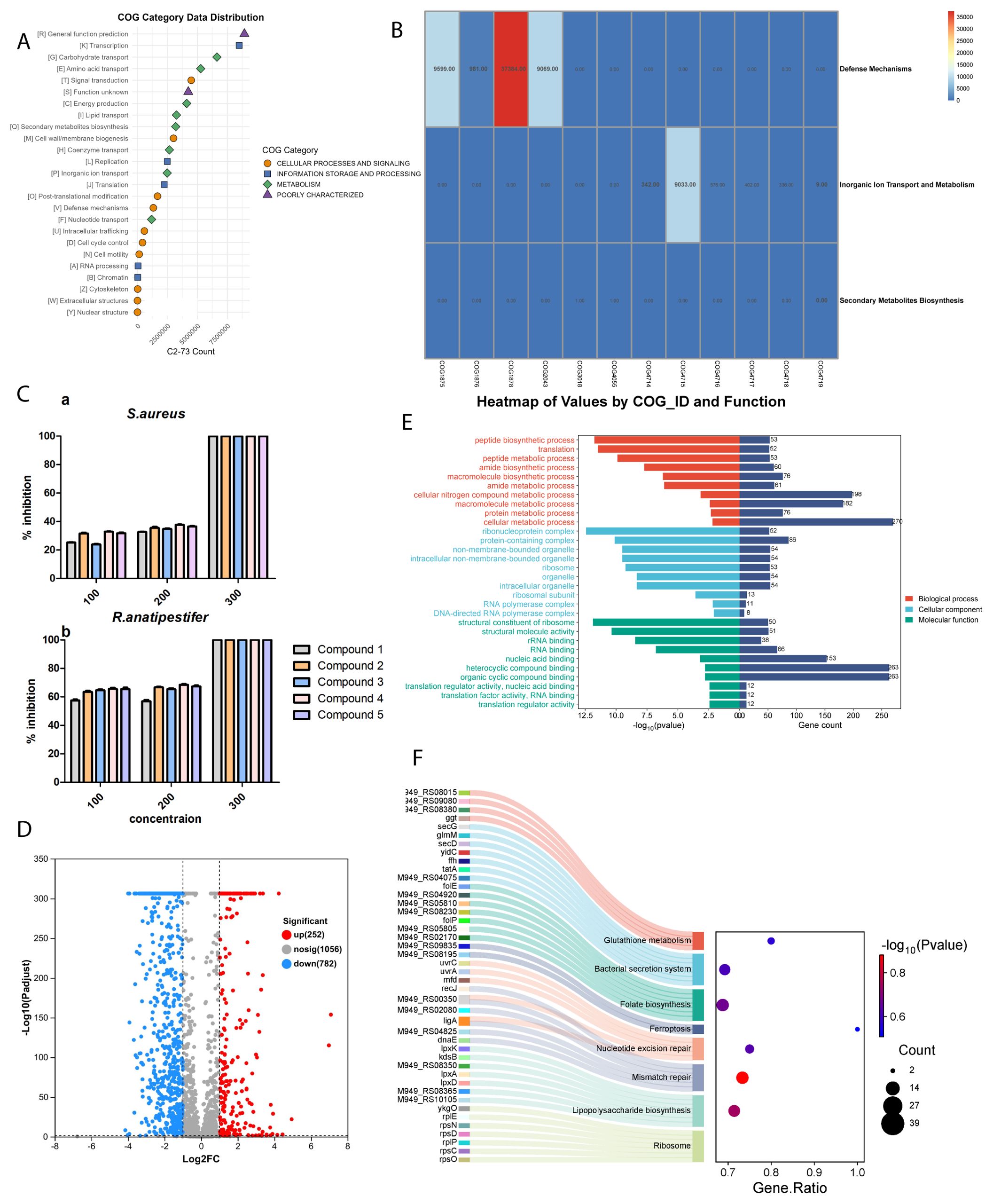
Figure 3. (A) COG category data distribution for C2-13 strain. (B) COG gene analysis for C2-13 strain. (C) Antibacterial activity of compounds. (D) Volcano plot of DEGs. (E) GO enrichment analysis of DEGs. Red involves biological process processes, blue involves cellular component processes, and green involves molecular function processes. (F) KEGG enrichment analysis of downregulated DEGs.
3.4.2 Inorganic ion transport and metabolism
Bacteria adapt to metal stress primarily through the regulation of inorganic ion transport (Silver and Phung, 2005). Selective transport systems, as represented by COG4719 through COG4714, enable bacteria to control metal ion levels and prevent toxic accumulation (Figure 3B). The presence of heavy metals, such as zinc, can trigger a stress response that activates pathways associated with secondary metabolite production. For instance, in our study, a 1-mM concentration of zinc sulfate was found to be optimal for the bacterial strain C2-13. Higher concentrations, however, created a toxic environment that adversely affected bacterial growth (Figure 2). This observation underscores the delicate balance bacteria must maintain regarding metal ion concentrations to promote survival and metabolite production.
3.4.3 Defense mechanisms
Under conditions of heavy metal stress, bacteria activate defense-related genes represented by COG2043 and COG1878, leading to the production of secondary metabolites with antioxidative and metal-chelating effects. Compounds such as exopolysaccharides and biosurfactants, linked to COG1876 and COG1875, are produced in higher quantities in response to heavy metal exposure (Figure 3B). These substances facilitate biofilm formation and help trap metals outside the cell, effectively reducing intracellular metal concentration (Gupta and Diwan, 2017). In our study, compound 5 functions as a biosurfactant with a hydrophobic tail and a carboxylic acid group. This defense strategy highlights the role of secondary metabolites not only as a means of coping with metal stress but also as protective agents that enhance bacterial survival.
3.5 Antibacterial activity
The antibacterial activity of all compounds was initially conducted against 10 pathogenic microbes, but only two pathogens have shown interesting results, including S. aureus and R. anatipestifer. Among all compounds, 4 and 5 have more interesting results compared to others. All of the compounds were re-evaluated against these two pathogenic microbes with three different concentrations: 100, 200, and 300 µM. For S. aureus, all of the compounds at 100 µM showed weak inhibition and at 200 µM showed moderate inhibition, while at 300 µM all of the compounds showed 100% inhibition (Figure 3C). For R. anatipestifer, the compounds have a more potent effect compared to S. aureus and achieved its IC50 values at 100 µM (Figure 3C). Overall, compound 4 has shown potent results among all compounds, and further in-depth analysis was performed only for compound 4.
3.6 Transcriptome analysis unveiling antimicrobial mechanisms
3.6.1 Differentially expressed genes
The preceding investigations provided compelling evidence for the remarkable antibacterial efficacy exhibited by compound 4 against R. anatipestifer, and transcriptomic analysis was performed to gain deeper insights into the underlying mechanism. The RNA samples obtained from compound 4 treatment group and the control group were of high quality following the filtration of raw sequencing data, and the resulting sequencing data exhibited a Q30 trimming percentage of 95%, surpassing the threshold of 90% (Supplementary Table S7). Furthermore, a total of 71 differentially expressed genes (DEGs) were identified, with 43 genes upregulated and 28 genes downregulated (Figure 3D), with the detailed information of DEGs described in Supplementary Tables S8 and S9.
3.6.2 Functional annotation analysis of DEGs
The DEGs were subjected to annotation and enrichment analysis utilizing the Gene Ontology (GO) database, a globally recognized system for classifying gene functions into three categories: biological process (BP), cellular component (CC), and molecular function (MF). The results of the GO classifications and functional enrichment analysis for the DEGs are presented in Figure 3. The volcano plot here showed that the total number of significant genes’ count was 1,034, among which 252 genes were upregulated and 782 were downregulated (Figure 3D). The Gene Ontology (GO) analysis results indicate that treatment with compound 4 predominantly affects the biological process (BP) category, with a significant number of DEGs linked to macromolecule biosynthesis, especially proteins. These DEGs are notably involved in various protein-related processes, including peptide biosynthesis, translation, protein folding, as well as peptide and protein metabolism (Figure 3E). In the cellular component (CC) category, the most substantial enrichment of DEGs is observed in ribosomes, ribonucleoprotein complexes, and ribosomal subunits, with additional enrichment found in organelle components, cellular structures, and ribosome composition. For the molecular function (MF) category, the DEGs are associated with roles in ribosomal structural components, ATP-dependent protein-folding chaperones, and the binding of rRNA and RNA. This suggests that the structural integrity and functional dynamics of Riemerella anatipestifer ribosomes might be compromised, potentially impairing protein biological functions.
KEGG enrichment analysis was conducted to comprehensively investigate the functional roles of the DEGs, aiming to classify the DEGs into distinct pathways by utilizing bioinformatic databases. Multiple upregulated pathways closely associated with antibacterial processes were discovered, revealing the underlying mechanism of R. anatipestifer cells with the treatment of compound 4 (Figure 3F). In the ribosome pathway category, the highest number of DEGs (gene count: 52) was significantly enriched in the translation process, and 11 DEGs were enriched in the mismatch repair and folate biosynthesis process, indicating that compound 4 exerted its antimicrobial effect by inhibiting the translation, replication, and DNA repair process of proteins in R. anatipestifer. Furthermore, enrichment was observed within lipopolysaccharide biosynthesis, nucleotide excision repair, folate biosynthesis, and bacterial secretion system with the high DEGs (Figure 3F). This implied that compound 4 may exert its antimicrobial effects by actively engaging in and influencing various processes within bacterial cells. These results enhance our understanding of the molecular mechanisms that underpin the antimicrobial actions of compound 4.
3.6.3 Core DEGs related to antibacterial mechanism
The ribosome is a critical pillar in bacteria, where it plays an essential role in protein synthesis, focusing the first target with compound 4 in R. anatipestifer. The bacterial ribosome is composed of two subunits: the 30S (small) subunit and the 50S (large) subunit. These subunits are made up of ribosomal RNA (rRNA) and proteins, and together they catalyze the translation of mRNA into proteins, which are necessary for cell function, growth, and reproduction (Poehlsgaard and Douthwaite, 2005). In our study, significant downregulation was observed in genes encoding 30S ribosomal proteins (rpsD, rpsO, rpsC, and rpsN) and 50S ribosomal proteins (rplP and rpsD) upon exposure to compound 4, as indicated in Figure 3F and Supplementary Table S8. The inhibition of these genes disrupts the fundamental process of protein synthesis in bacteria that encode essential ribosomal proteins. This causes bacterial growth inhibition, misfolded proteins, and ultimately bacterial death. Since ribosomes are vital for cell survival, compound 4 acts as a ribosomal inhibitor, targeting R. anatipestifer ribosomal subunits or proteins for its antibacterial effect.
Gliding motility proteins like GldM, GldL, GldK, GldN, and sprA are integral to the type IX secretion system (T9SS), which is essential in bacteria like R. anatipestifer. These proteins facilitate the secretion of enzymes and adhesins that are critical for motility, surface attachment, and colonization. In terms of antibacterial mechanisms, mutations or downregulation of these proteins impair the secretion system, reducing bacterial motility and biofilm formation, which, in turn, decreases their pathogenicity and resistance to host immune defense. However, if the T9SS pathway is disrupted, it can reduce bacterial survival under stressful conditions, indirectly lowering their resistance to antibiotics (Chen et al., 2019).
Resistance mechanism is also one of the important ways to target bacteria. By targeting multiple essential pathways, it demonstrates a multi-pronged antibacterial mechanism, which not only inhibits growth but also prevents the bacteria from adapting or developing resistance easily. DEGs like dapA, dnaA, and yidC interfere with three critical aspects of bacterial survival. dapA weakens structural defense, making the bacteria more susceptible to lysis, and plays a major role on cell wall integrity. dnaA, which plays a major role in replication, disrupts the bacterial cell cycle and population growth, and yidC is responsible for membrane functionality, impairing essential processes like energy production, transport, and protein secretion (Figure 3F and Supplementary Table S8). Our compound downregulated all of these three DEGS, showing the broad mechanism that is highly effective against Riemerella anatipestifer and proving to be a potent antibacterial agent. As we can see, our compound 4 downregulated these proteins (Supplementary Table S8), which hereby shows that our compound also shows an antibacterial effect by impairing the secretion system and minimizing the bacterial motility and biofilm formation, thereby reducing the resistance toward the host immune system. Thus, understanding this system offers potential therapeutic avenues for targeting bacteria by inhibiting their motility and biofilm-forming capabilities.
3.7 Molecular docking
3.7.1 Ligand and protein preparation
The in vitro antibacterial assays indicated that all compounds showed best activity against S. aureus and potent activity against R. anatipestifer. Thus, we have considered to take R. anatipestifer for in-depth analysis, and to further validate the transcriptome studies, we have initially considered two proteins for R. anatipestifer. The proteins selected for R. anatipestifer based on literature search were obtained from Uniprot as tRNA N6-adenosine threonylcarbamoyltransferase Q9F0V0 and RIEAN Multifunctional fusion protein.
3.7.2 Structure evaluation
The structure of the RIEAN Multifunctional fusion protein was further evaluated to predict the quality of the protein structure. Multiple methods were employed for validation of the 3D models. Initially, PROCHECK server was used for the structural quality assessment of the modeled structure. The predicted model evaluation of the 3D protein model demonstrated that nearly 94.3% of the residues was in the core regions, 5.1% was in allowed, and 0.4% was in disallowed regions, thus confirming that the predicted model is of high quality. VERIFY 3D predicted that, in the RIEAN model, 78.07% of the residues had averaged 3D–1D score. ERRAT, a so-called quality factor, predicted the quality score of the RIEAN model as 97.87%. The higher the score, the more significant the quality of the 3D model. These findings demonstrate that the refined model is of high quality. The Pro-SA-web server was employed to double-check the quality of the 3D models. The Z-scores, a parametric quantity representing the overall quality of the model, was 10.82 for RIEAN, respectively. Furthermore, the Ramachandran plot shows the statistical distribution of the combinations of the backbone dihedral angles ϕ and ψ. In theory, the allowed regions of the Ramachandran plot show which values of the Phi/Psi angles are possible for an amino acid, X, in an ala-X-ala tripeptide as the plot indicates a good average score as mentioned in Figure 4. Supplementary Table S9 and Figure 4 summarize the findings of these four programs, indicating the high quality of the models.
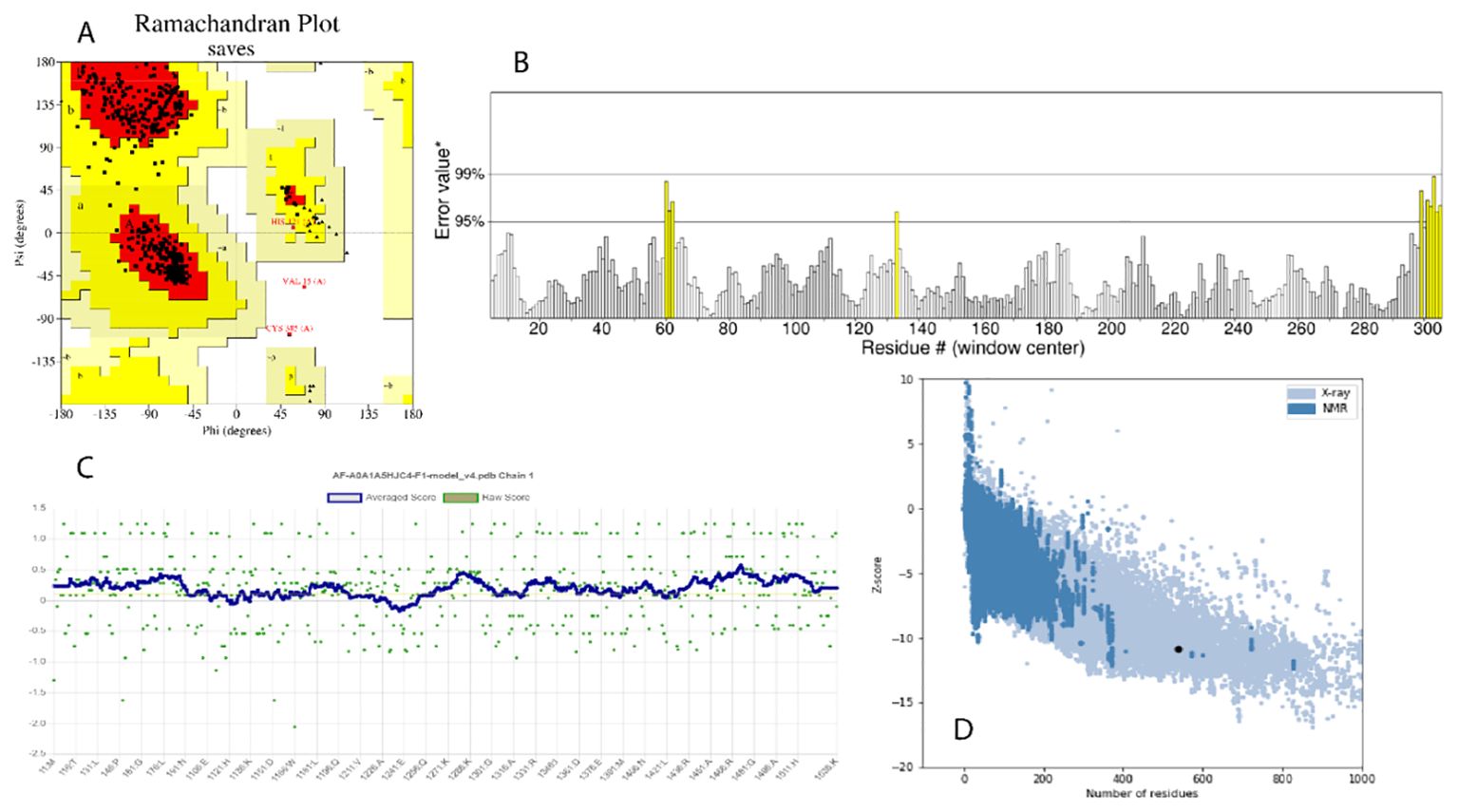
Figure 4. (A) Ramachandran plot. (B) ERRAT crystallography picture for protein. (C) Z-score value for the recognition of errors in three-dimensional structures of protein. (D) Best Z-score value through X-ray and NMR.
3.7.3 Ligand–protein docking
Through systematic analysis, two protein targets of R. anatipestifer were selected for molecular docking. Docking scores, alongside binding energy values, served as primary criteria for subsequent molecular dynamics simulations (Table 1; Figure 5). The compounds have shown best binding toward both selected target proteins, but the highest binding was achieved against RIEAN Multifunctional fusion protein (RMFP). The interacting residues for all compounds against RMFP and tRNA N6-adenosine threonylcarbamoyltransferase in molecular docking analysis 3D figures are demonstrated in Figure 5. Each compound interaction with the receptor proteins, including hydrogen bonds, Pi–pi-stacked interactions, and van der Waals interactions, which are depicted by dotted lines, are evaluated in 10 different pockets, and the best pocket with the highest binding score was recorded (Figure 5). Among all compounds, compound 4 achieved the highest binding score against RMFP at -12.8 kcal/mol. Additionally, the presence of hydrogen bond interactions with receptor proteins is specifically highlighted in Figure 5 and Supplementary Figures S22–S31.
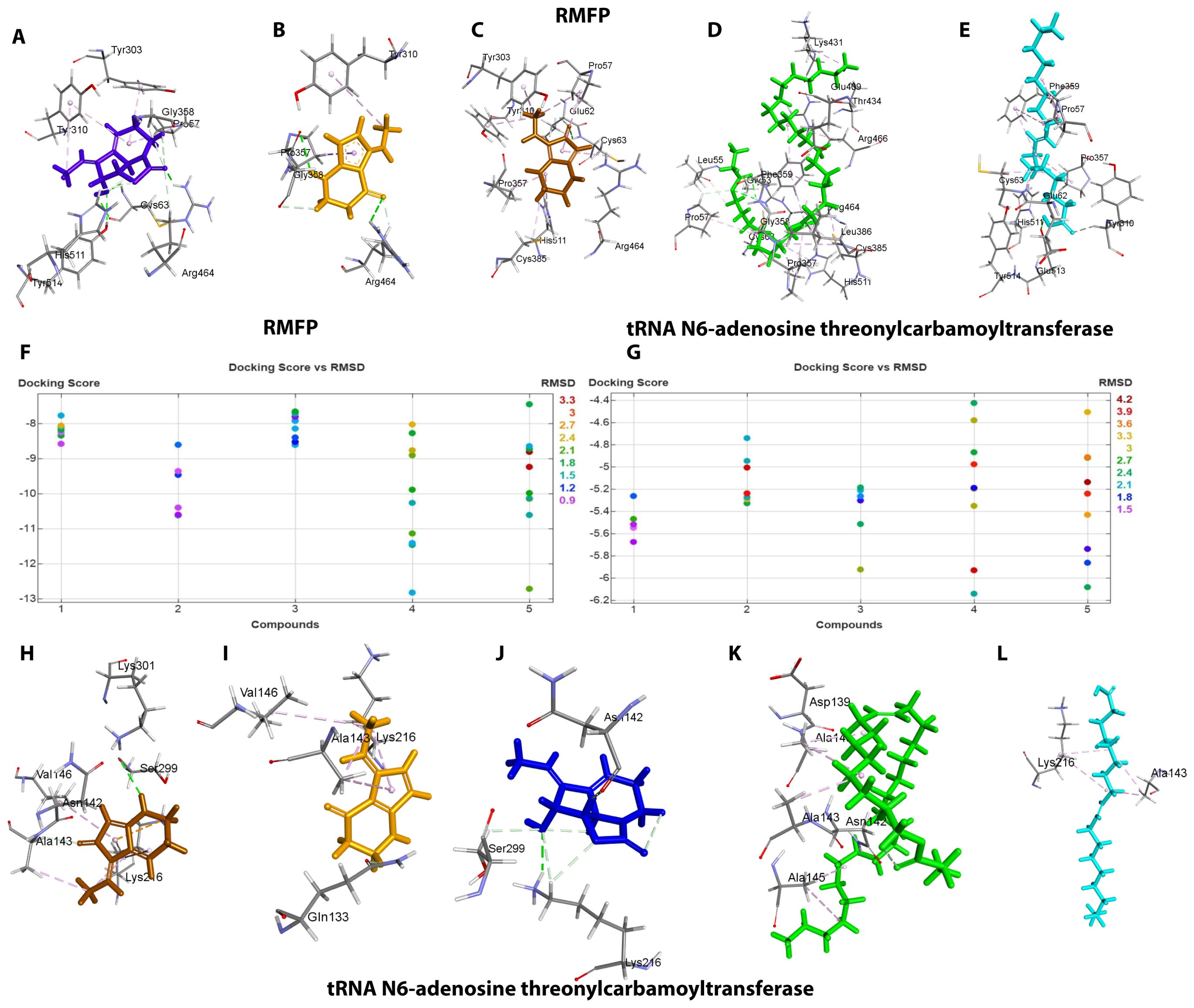
Figure 5. (A–F) 3D vision of complex for compounds 1–5 with RMFP. (G) Docking score and RMSD values of compounds with RMFP. (H) Docking score and RMSD values of compounds with tRNA N6-adenosine threonylcarbamoyltransferase. (I–L) 3D vision of complex for compounds 1–5 with tRNA N6-adenosine threonylcarbamoyltransferase.
For the protein RMFP, all compounds’ binding complex can be observed in Figures 5A–E and Supplementary Figures S22–S26. Compound 1 was observed to bind with three hydrogen bonds at ARG A: 464, TYR A: 514, and CYS A: 63 with a binding score of -8.7 and a RMSD value of 0.9 (Figure 5A; Supplementary Figure S22). Compound 2 was observed to bind with two hydrogen bonds at ARG A: 464 and PRO A: 357 with a binding score of -10.7 and a RMSD value of 0.9 (Figure 5B; Supplementary Figure S23). Compound 3 was observed to bind with one hydrogen bond at CYS A: 63 with a binding score of -8.6 and a RMSD value of 1.2 (Figure 5C, Supplementary Figure S24). Compound 4 was observed to bind with one hydrogen bond at ARG A: 464 with the highest binding score of -12.9 and RMSD value of 1.5 (Figure 5D; Supplementary Figure S25). Compound 5 was observed to bind with one hydrogen bond at GLU A: 513 with a binding score of -12.7 and a RMSD value of 1.8 (Figure 5E, Supplementary Figure S26). For the protein tRNA N6-adenosine threonylcarbamoyltransferase, all compounds’ binding complex can be observed in Figures 5H–L and Supplementary Figures S27–S31. Compound 1 was observed to bind with one hydrogen bond at SER B: 299 with a binding score of -5.7 and a RMSD value of 1.5 (Figure 5H; Supplementary Figure S27). Compounds 2, 4, and 5 have no hydrogen bonds with binding scores of -5.3, -6.1, and 6 (Figures 5I, K, L, Supplementary Figures S28, S30, S31). Compound 3 was also observed to bind with one hydrogen bond at LYS B: 63 with a binding score of -5.9 and a RMSD value of 2.4 (Figure 5J; Supplementary Figure S29).
3.7.4 Protein–protein docking
Using HADDOCK 2.0 in conjunction with the PDBsum database, we conducted simulations to analyze both stable and dynamic interactions between the multi-epitope vaccine (acting as the ligand) and three innate immune receptors. Among the models generated by HADDOCK 2.0, the TLR4–RMFP complex exhibited the lowest binding energy score of 16.9 ± 11.2 kcal/mol, indicating a high level of affinity and stability for the docked complexes, as outlined in Table 2.
Consequently, a considerable number of clearly defined molecular interactions have been predicted between the envisaged vaccine construct and the TLR4 receptor. The interface statistics for TLR4 receptors are depicted in Figure 6A.
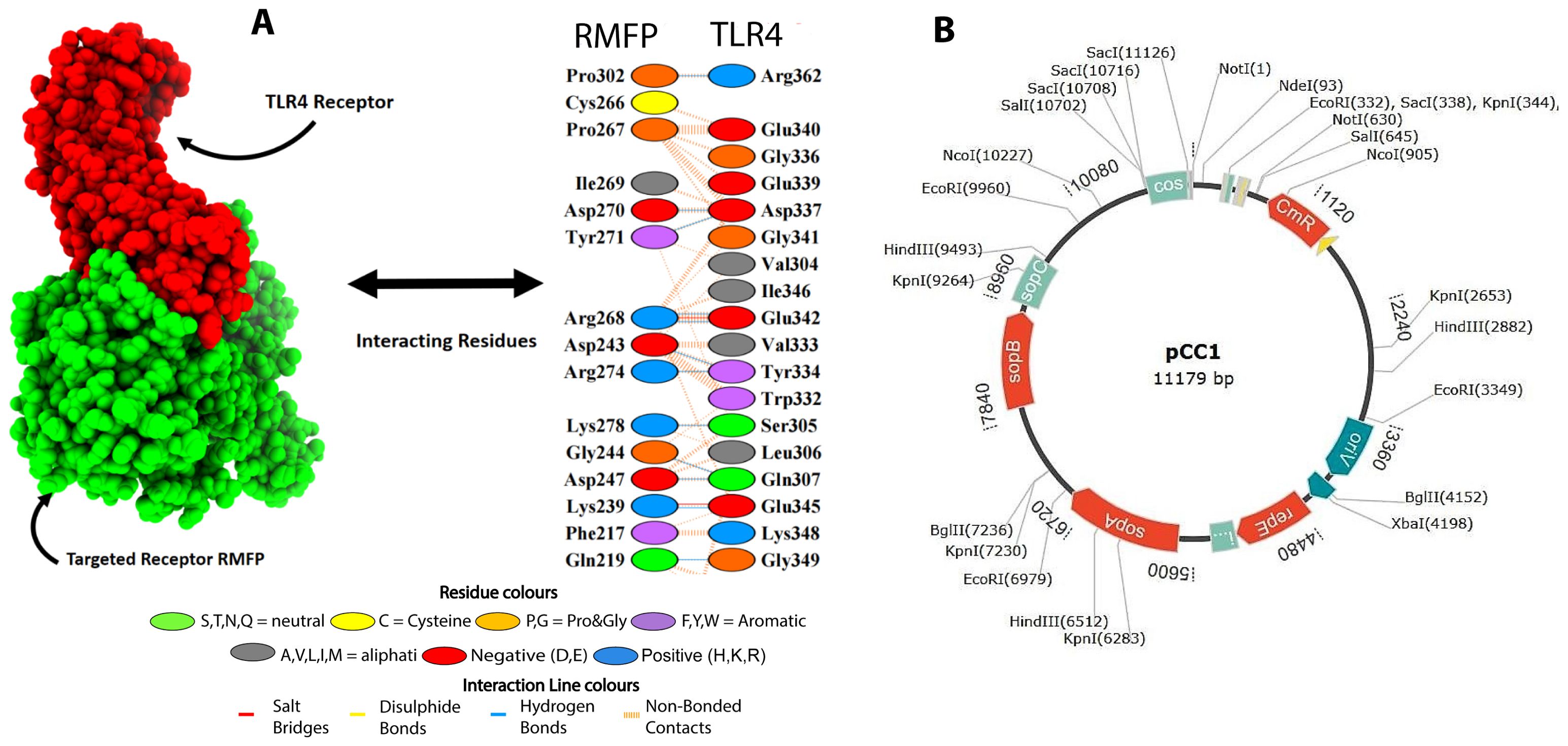
Figure 6. (A) Docked complex of TLR4–RMFP along interactions. (B) In silico cloning of codon-optimized RMFP into the E. coli K12 expression system. The plasmid backbone is kept in black, while the inserted DNA sequence is shown.
3.8 In silico cloning within E. coli system
In silico cloning was carried out to ensure the expression of proteins as per our previous protocols (Hassan et al., 2024) but with little changes as E. coli was considered as host. First, the RMFP was altered to use the E. coli expression system’s codon. The clone was 7.50 and 1.1 kb long, as mentioned in Figure 6B.
The plasmid map of pCC1 highlights its critical features for genetic engineering applications, including an origin of replication (oriV) near 3360 for plasmid propagation, a chloramphenicol resistance gene (CmR) 1120 for selection, and sop genes (sopA-6720, sopB-7840, and sopC-8960) for plasmid stability and segregation. Additionally, it contains multiple restriction enzyme sites (e.g., EcoRI, HindIII, SacI), facilitating gene cloning. The presence of the replication initiator protein (RepE) nearby 4480 further ensures efficient plasmid replication. This structure makes pCC1 an ideal vector for manipulating and expressing genes of interest, such as those encoding bioactive compounds (Figure 6B).
3.8.1 Overview of restriction sites and selection rationale
To optimize genetic manipulation and functional analysis, restriction sites were strategically assigned to specific genes. From our transcriptome studies (Supplementary Table S8), the DEGs such as rpsO and gldN can be linked to the EcoRI site at 9960, ensuring seamless integration into plasmids for co-expression studies. Similarly, rpsC and dapA can be positioned with HindIII at 2882 to facilitate dual enzyme cloning and collaborative analysis of ribosomal and biosynthetic pathways. rplP and dnaA can be aligned with SacI at 10708, enabling multi-cloning site integration without disrupting other genetic elements (Supplementary Table S10).
3.8.2 Functional clustering and experimental benefits
Genes involved in motility, such as gldM, gldL, and gldK, can be carefully assigned to distinct restriction sites to assess their individual and collective roles in T9SS interactions—for instance, gldM can be inserted at EcoRI (332), while gldL and gldK can be assigned to SacI (10716) and HindIII (6512), respectively, ensuring functional isolation. Meanwhile, motility-associated genes like sprA and rpsD can be grouped at EcoRI (3349) to support coordinated assays (Supplementary Table S10). These assignments reflect a systematic approach, balancing functional clustering with experimental independence, critical for studying pathways like replication, motility, and protein synthesis. The versatile pCC1 plasmid can accommodate all mentioned downregulated genes at its multiple restriction sites (EcoRI, HindIII, and SacI) for functional validation. The strategic placement of these genes ensures effective expression, enabling a detailed exploration of compound 4’s mechanism as an antibiotic targeting ribosome assembly, motility, secretion systems, and essential metabolic processes.
3.9 Molecular docking simulations
3.9.1 Ligand–protein simulations
After the molecular docking results, all the compounds with RIEAN Multifunctional fusion protein (RMFP) were further analyzed based on binding energy. RMSD, RMSF, SASA, and Rog were analyzed for the complex for 100 ns (Figure 7). The RMSD graph shows that the complex was stable with all compounds except compound 5. Little fluctuations were observed in that compound after 60 ns, but later it was stable until the end of the simulations (Figure 7A). Following that, fluctuations in RMSD values for the protein during the simulation period were inside the limit of 2 Å, which is perfectly acceptable. The result demonstrated stable ligand binding to the protein’s active binding region throughout the simulation tenure. RMSF and RoG were stable for all compounds (Figures 7B, C). In SASA, little fluctuations were observed for all compounds, but later they were stable in the system (Figure 7D). The hydrogen bond analysis for all compounds was also mentioned in Figures 7E–1, and the best binding poses during simulations were recorded for all compounds (Figures 7J–N). The result demonstrated stable ligand binding to the protein’s active binding region throughout the simulation tenure. The PCA analysis for MDS was also performed. Figure 8 shows scatter plots and explained variance charts for four different molecular dynamics analyses, projected onto the first two principal components (PC1 and PC2). Each row represents a specific analysis with a distinct color scheme (a1–d1). The first row, shown in orange, corresponds to root mean square deviation (RMSD) (a2–d2). The second row, in cyan, represents root mean square fluctuation (RMSF) (a3–d3). The third row, colored purple, depicts solvent accessible surface area (SASA) (a4–d4). The fourth row, displayed in black and using circular markers (dots), corresponds to hydrogen bonds (H-bonds).
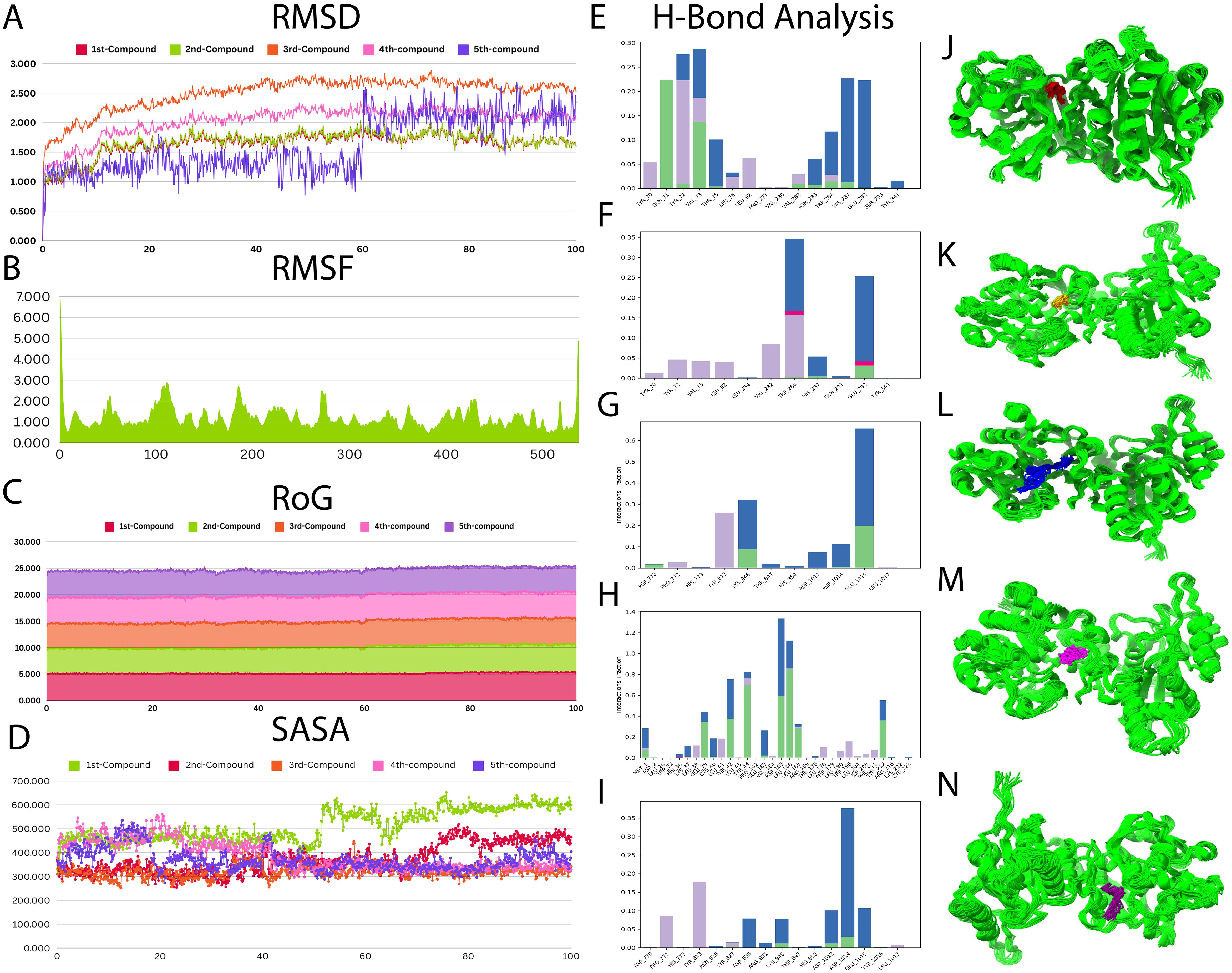
Figure 7. Molecular dynamic simulations for all compounds. (A) RMSD–RMFP complex for all compounds. (B) RMSF–RMFP complex for all compounds. (C) RoG for all compounds. (D) SASA for all compounds. (E–I) Hydrogen bond analysis for compounds 1–5. (J–N) Binding poses in the pocket during simulations for compounds 1–5.
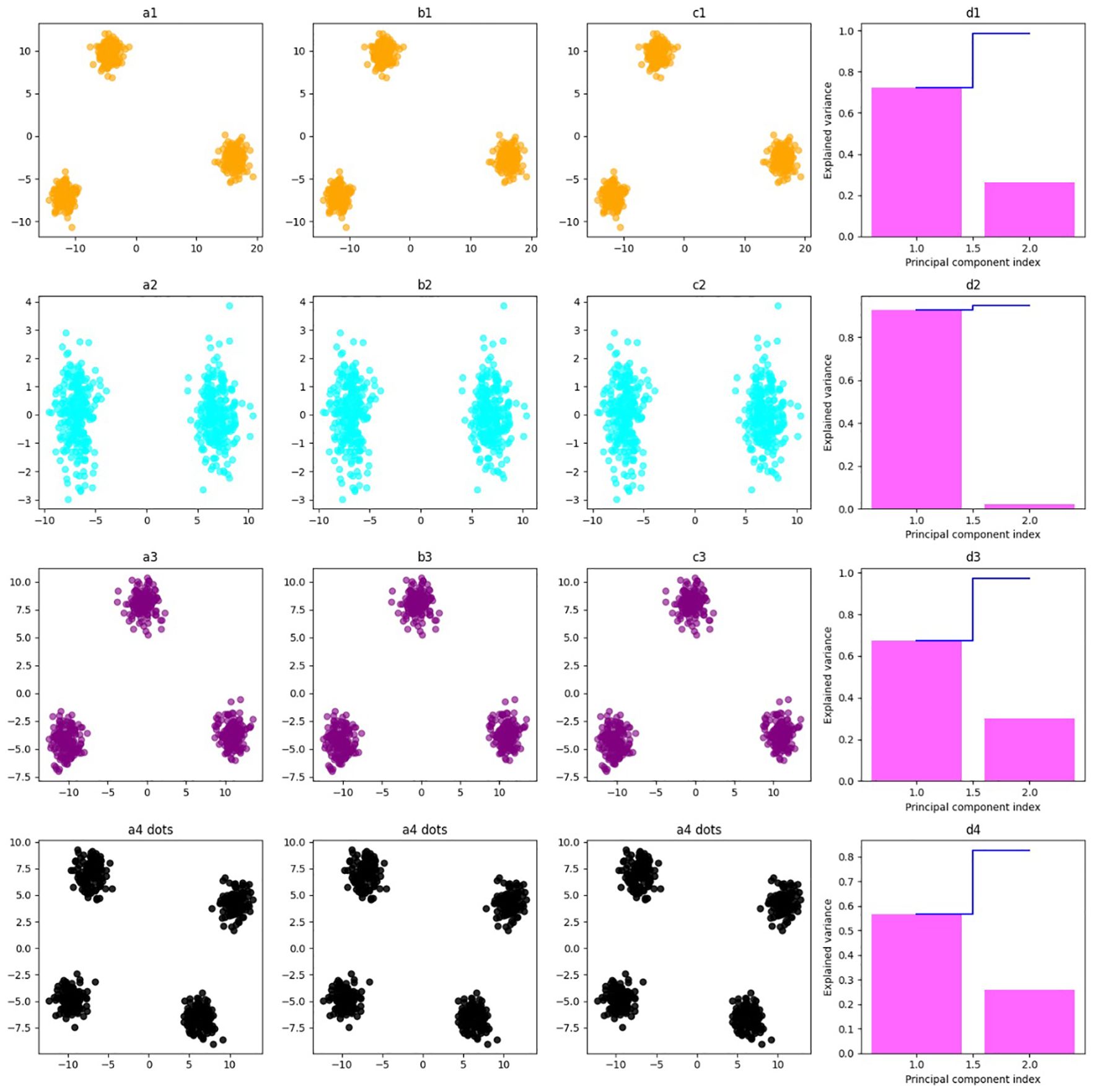
Figure 8. Scatter plots and explained variance charts for four different molecular dynamics analyses, projected onto the first two principal components (PC1 and PC2). Each row represents a specific analysis with a distinct color scheme. (a1–d1) The first row, shown in orange, corresponds to root mean square deviation (RMSD). (a2–d2) The second row, in cyan, represents root mean square fluctuation (RMSF). (a3–d3) The third row, colored purple, depicts solvent accessible surface area (SASA). (a4–d4) The fourth row, displayed in black and using circular markers (dots), corresponds to hydrogen bonds (H-bonds).
3.9.2 Protein–protein simulations
The MDS for protein–protein analysis was evaluated through publicly available online server IModS, which is widely used for structural analysis, that involves making changes to the complex’s force field at different time intervals. As a result, the model that comes out of this usually has less distortion in all of the capacities that the residues represent. Eigenvalue calculations reveal that the TLR4–RMFP complex has a value of 5.001838e-05. A low RMSD and a highly co-related region in the heat maps indicated that the individual residues interacted more effectively with one another. A comprehensive description of the results of the IModS molecular dynamics simulations is provided in Figure 9.
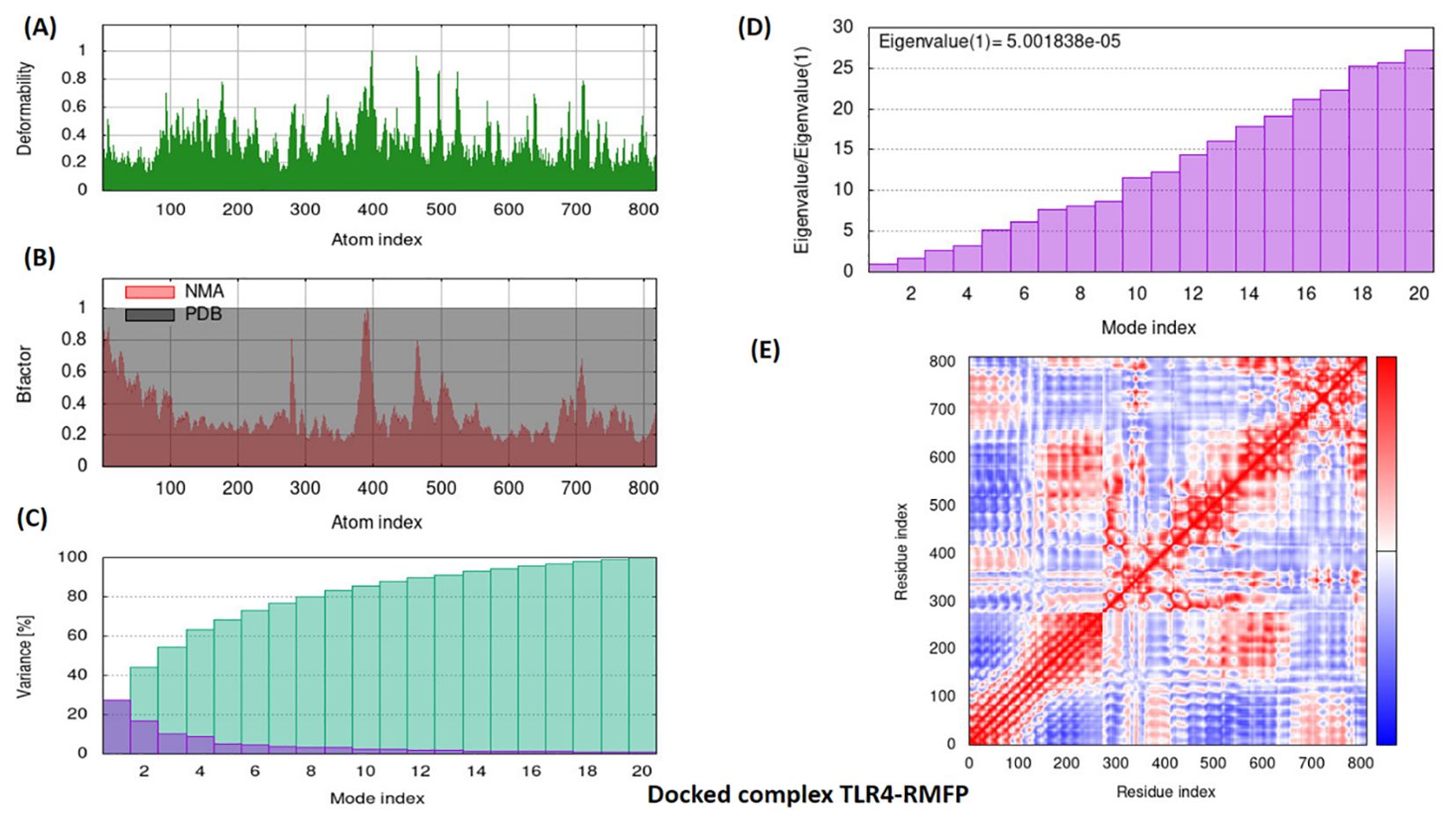
Figure 9. Simulation graphs of docked complex TLR4–RMFP. The iMOD server’s normal mode analysis (NMA) outputted the following plots: (A) Deformation plot, illustrating regions of significant structural flexibility, with values ranging from 0 to 0.6. (B) B-factor, representing atomic displacement parameters to indicate the dynamic behavior of atoms. (C) Variance plot, displaying the variance in atomic positions to highlight flexibility within the structure. (D) Eigenvalues, with a specific value of 5.001838e-05, reflecting the vibrational frequency and energy of the mode. (E) Covariance matrix plot, visualizing the correlated movements of atoms, with anticorrelated, uncorrelated, and correlated states of atomic motion represented by the blue, white, and red hues, respectively.
4 Discussion
This research focused on isolating secondary metabolites from marine Streptomyces sp. Furthermore, it evaluates the enhanced production of metabolites through heavy metals with various concentrations. The compounds (1–5) were evaluated for their antibacterial activity against gram-positive and gram-negative bacteria through in vitro assays. Further transcriptome analyses were performed to evaluate in depth the responsible genes for antibacterial mechanism. In silico studies of molecular docking and MD simulations were also performed for the active metabolites.
Using heavy metals as elicitors is an emerging approach to produce new or to enhance the production of secondary metabolites (Okem et al., 2015). Our previous studies have shown that compounds induced by metals typically do not appear under standard culture conditions but become detectable when abiotic stress, such as exposure to heavy metals, is applied (Shams et al., 2022; Hassan et al., 2024). Microorganisms exhibit varying levels of resistance or sensitivity to metals, which often requires adjustments in metal concentrations or combinations to activate dormant genetic pathways (Hassan et al., 2017; Shams ul Hassan et al., 2019; Ul Hassan et al., 2021). The effects of heavy metals on compound production can be both beneficial and detrimental, depending on the context. Metals like zinc and copper, in certain amounts, are essential for microbial growth and metabolism and can act as cofactors for enzymes involved in antibiotic synthesis and other drug production (Ye et al., 2014). In some microorganisms, the presence of heavy metals may trigger antibiotic production as a defense mechanism to compete with other organisms in metal-rich environments (Raytapadar et al., 1995). Our research indicates that Streptomyces sp. strains initially produced the compounds, but after adding zinc sulfate, the production of all compounds was totally enriched. As mentioned in Figure 3B, COG1878 was available with the highest quantity in the C2-13 bacterial strain, which is the main protein responsible for heavy metal attachment, which hereby also proves that our strain is sensitive to heavy metals and can respond well to any kind of heavy metal in their environment.
Natural products derived from plants, fungi, and bacteria have garnered significant interest as potential therapeutic agents to combat emerging antibiotic-resistant bacterial strains (Math et al., 2023). Marine-derived natural products, in particular, offer diverse structural frameworks with distinct mechanisms of action, making them promising candidates for developing novel anti-infective drugs capable of overcoming bacterial resistance mechanisms (Chakraborty et al., 2022b, a). Natural products generally have fewer side effects compared to synthetic drugs and are more easily biodegradable. Heavy metal-derived compounds are proven to have potent bioactivities as anticancer (Ye et al., 2014), anti-oxidant (Ul Hassan et al., 2021), anti-inflammatory (Shams et al., 2022), and antibacterial (Hassan et al., 2024). In our studies, the compounds (1–5) have shown the best antimicrobial activity against R. anatipestifer. All of the compounds (1–5) have achieved its IC50 value at 100 µM, which hereby proved that they are potent against R. anatipestifer.
Identifying genes with differential expression provides valuable insights into the molecular mechanisms and pathways at play, offering a foundation for future functional studies and the discovery of potential therapeutic targets (Wu et al., 2024). In our research, transcriptome analysis has given us a detailed understanding of gene expression changes under different conditions, shedding light on the complex regulatory networks driving cellular responses. Recent research has shown that gliding proteins are essential for anti-bacterial mechanism in R. anatipestifer as these proteins mutate the secretion system which, in turn, disturbs the bacterial normal growth process and results in cell lysis (Chen et al., 2019). In our studies, we also have proved that our compound 4 have shown an antibacterial effect by inhibiting the gliding proteins (Figure 3F) and also the ribosomal subunits. Additionally, we have conducted extensive molecular docking and simulation studies to assess the antibacterial activity against pathogenic bacteria. Two proteins for R. anatipestifer have been selected for docking, and all of the compounds have shown the best docking score against RMFP. Furthermore, MDS have also proved that compound 4 has good binding activity and has shown stability inside the protein core of the targeted proteins.
As demonstrated by our research, all compounds have exhibited promising outcomes in both antibacterial activity and protein binding through molecular docking.
5 Conclusion
The findings of this research highlight the significant potential of marine-derived compounds as novel sources of antibiotics, especially in the fight against antibiotic-resistant pathogens. Marine Streptomyces sp., C2-13, yielded five bioactive compounds, whose production was notably enhanced by the addition of zinc sulfate at a concentration of 0.5 mM. The antibacterial activities of these compounds were tested against various pathogens, with particularly promising results against Staphylococcus aureus and the less-studied pathogen Riemerella anatipestifer. The study’s comprehensive approach included transcriptomic analysis, which revealed that compound 4 disrupts bacterial growth by inhibiting the 30S and 50S ribosomal subunits and affecting the type IX secretion system (T9SS) crucial for protein translation and motility. This dual inhibition mechanism effectively stunts both growth and motility, offering a new avenue for antibacterial strategies. Additionally, molecular docking and in silico cloning further confirmed that compound 4 shows a high binding affinity to RMFP and TLR4 proteins, suggesting its potential role in immune modulation and vaccine development. This research provides a pioneering look into marine bacterial metabolites as novel antibacterial agents against R. anatipestifer, setting a foundation for future studies focused on optimizing yield and exploring broader clinical applications.
Data availability statement
The original contributions presented in the study are included in the article/Supplementary Material. Further inquiries can be directed to the corresponding authors.
Author contributions
SH: Conceptualization, Investigation, Methodology, Writing – original draft. HJ: Writing – review & editing, Project administration, Methodology, Validation.
Funding
The author(s) declare financial support was received for the research, authorship, and/or publication of this article. We are incredibly grateful to the NSFC (81973191), and the National Key Research and Development Program of China (2018YFE0192600) for providing financial support for the complete undertaking. This work was also supported by the modern plateau plant medicine research project of Shanghai Jiao Tong university (SA1700208).
Conflict of interest
The authors declare that the research was conducted in the absence of any commercial or financial relationships that could be construed as a potential conflict of interest.
Publisher’s note
All claims expressed in this article are solely those of the authors and do not necessarily represent those of their affiliated organizations, or those of the publisher, the editors and the reviewers. Any product that may be evaluated in this article, or claim that may be made by its manufacturer, is not guaranteed or endorsed by the publisher.
Supplementary material
The Supplementary Material for this article can be found online at: https://www.frontiersin.org/articles/10.3389/fmars.2024.1461607/full#supplementary-material
Abbreviations
RMFP, RIEAN Multifunctional fusion protein; TLR, Toll-like receptor; HPLC, high-performance liquid chromatography; NMR, nuclear magnetic resonance; DEG, differentially expressed genes; MDS, molecular docking simulations; RMSD, root mean square deviation; RMSF, root mean square fluctuations; RoG, radius of gyration; GO, Gene Ontology.
References
Baindara P., Roy D., Mandal S. M. (2024). CycP: A novel self-assembled vesicle-forming cyclic antimicrobial peptide to control drug-resistant S. aureus. Bioengineering 11, 855. doi: 10.3390/bioengineering11080855
Carroll A. R., Copp B. R., Grkovic T., Keyzers R. A., Prinsep M. R. (2024). Marine natural products. Nat. Prod. Rep. 41, 162–207. doi: 10.1039/d3np00061c
Chakraborty B., Kumar R. S., Almansour A. I., Gunasekaran P., Nayaka S. (2022a). Bioprospection and secondary metabolites profiling of marine Streptomyces levis strain KS46. Saudi. J. Biol. Sci. 29, 667–679. doi: 10.1016/j.sjbs.2021.11.055
Chakraborty B., Kumar R. S., Almansour A. I., Perumal K., Nayaka S., Brindhadevi K. (2022b). Streptomyces filamentosus strain KS17 isolated from microbiologically unexplored marine ecosystems exhibited a broad spectrum of antimicrobial activity against human pathogens. Process. Biochem. 117, 42–52. doi: 10.1016/j.procbio.2022.03.010
Chen Z., Wang X., Ren X., Han W., Malhi K. K., Ding C., et al. (2019). Riemerella anatipestifer GldM is required for bacterial gliding motility, protein secretion, and virulence. Vet. Res. 50, 1–12. doi: 10.1186/s13567-019-0660-0
Chung I. M., Ali M., Chun S. C., Jin C. W., Cho D. H., Hong S. B., et al. (2007). New aliphatic alcohol and ester constituents from rice hulls of Oryza sativa. Chin. J. Chem. 25, 843–848. doi: 10.1002/cjoc.200790155
Gupta P., Diwan B. (2017). Bacterial Exopolysaccharide mediated heavy metal removal: A Review on biosynthesis, mechanism and remediation strategies. Biotechnol. Rep. (Amsterdam. Netherlands). 13, 58–71. doi: 10.1016/j.btre.2016.12.006
Hassan S. S., Shah S. A. A., Pan C., Fu L., Cao X., Shi Y., et al. (2017). Production of an antibiotic enterocin from a marine actinobacteria strain H1003 by metal-stress technique with enhanced enrichment using response surface methodology. Pak. J. Pharm. Sci. 30, 313–324.
Hassan S. S. U., Wu J., Li T., Ye X., Rehman A., Yan S., et al. (2024). Unlocking marine microbial treasures: new PBP2a-targeted antibiotics elicited by metals and enhanced by RSM-driven transcriptomics and chemoinformatics. Microb. Cell Fact. 23, 303. doi: 10.1186/s12934-024-02573-0
Khan K., Sulaiman M., Alhar O., Abbas M. N., Abbas S. Q. (2022). Integrated bioinformatics-based subtractive genomics approach to decipher the therapeutic drug target and its possible intervention against brucellosis. Bioengineering 9, 633. doi: 10.3390/bioengineering9110633
Math H. H., Kumar R. S., Chakraborty B., Almansour A. I., Perumal K., Kantli G. B., et al. (2023). Antimicrobial efficacy of 7-hydroxyflavone derived from amycolatopsis sp. HSN-02 and its biocontrol potential on cercospora leaf spot disease in tomato plants. Antibiotics 12, 1175. doi: 10.3390/antibiotics12071175
Mothana A. A., Al-Shamahy H. A., Mothana R. A., Khaled J. M., Al-Rehaily A. J., Al-Mahdi A. Y., et al. (2022). Streptomyces sp. 1S1 isolated from Southern coast of the Red Sea as a renewable natural resource of several bioactive compounds. Saudi. Pharm. J. 30, 162–171. doi: 10.1016/j.jsps.2021.12.012
Muhammad I., Hassan S. S., Farooq M. A., Zhang H., Ali F., Xiao X., et al. (2024). Undescribed secondary metabolites derived from Cinnamomum migao H. W. Li, showcasing anti-inflammatory, antioxidant, and in silico properties. J. Mol. Struct. 1312, 138485. doi: 10.1016/j.molstruc.2024.138485
Okem A., Stirk W. A., Street R. A., Southway C., Finnie J. F., Van Staden J. (2015). Effects of Cd and Al stress on secondary metabolites, antioxidant and antibacterial activity of Hypoxis hemerocallidea Fisch. & C.A. Mey. Plant Physiol. Biochem. 97, 147–155. doi: 10.1016/j.plaphy.2015.09.015
Poehlsgaard J., Douthwaite S. (2005). The bacterial ribosome as a target for antibiotics. Nat. Rev. Microbiol. 3, 870–881. doi: 10.1038/nrmicro1265
Puder C., Krastel P., Zeeck A. (2000). Streptazones A, B 1, B 2, C, and D: new piperidine alkaloids from streptomycetes. J. Nat. Prod. 63, 1258–1260. doi: 10.1021/np0001373
Pulat S., Yang I., Lee J., Hwang S., Zhou R., Gamage C. D. B., et al. (2024). Anithiactin D, a phenylthiazole natural product from mudflat-derived streptomyces sp., suppresses motility of cancer cells. Mar. Drugs 22, 88. doi: 10.3390/md22020088
Raytapadar S., Datta R., Paul A. K. (1995). Effects of some heavy metals on growth, pigment and antibiotic production by Streptomyces galbus. Acta Microbiol. Immunol. Hung. 42, 171–177.
Shams S., Abbas S. Q., Muhammad I., Wu J., Yan S., Ali F., et al. (2022). Metals-triggered compound CDPDP exhibits anti-arthritic behavior by downregulating the in fl ammatory cytokines, and modulating the oxidative storm in mice models with extensive ADMET, docking and simulation studies. Front. Pharmacol. 13. doi: 10.3389/fphar.2022.1053744
Shams ul Hassan S., Jin H., Abu-Izneid T., Rauf A., Ishaq M., Suleria H. A. R. (2019). Stress-driven discovery in the natural products: A gateway towards new drugs. Biomed. Pharmacother. 109, 459–467. doi: 10.1016/j.biopha.2018.10.173
Silver S., Phung L. T. (2005). Genes and enzymes involved in bacterial oxidation and reduction of inorganic arsenic. Appl. Environ. Microbiol. 71, 599–608. doi: 10.1128/AEM.71.2.599-608.2005
Tatusov R. L., Fedorova N. D., Jackson J. D., Jacobs A. R., Kiryutin B., Koonin E. V., et al. (2003). The COG database: an updated version includes eukaryotes. BMC Bioinf. 4, 41. doi: 10.1186/1471-2105-4-41
ul Hassan S. S., Abdel-Daim M. M., Behl T., Bungau S. (2022). Natural products for chronic diseases: A ray of hope. Molecules 27, 5573. doi: 10.3390/molecules27175573
Ul Hassan S. S., Muhammad I., Abbas S. Q., Hassan M., Majid M., Jin H. Z., et al. (2021). Stress driven discovery of natural products from actinobacteria with anti-oxidant and cytotoxic activities including docking and admet properties. Int. J. Mol. Sci. 22, 11432. doi: 10.3390/ijms222111432
Wu J., ul Hassan S. S., Zhang X., Li T., Rehman A., Yan S., et al. (2024). Discovery of potent anti-MRSA components from Dalbergia odorifera through UPLC-Q-TOF-MS and targeting PBP2a protein through in-depth transcriptomic, in vitro, and in-silico studies. J. Pharm. Anal. 14, 100938. doi: 10.1016/j.jpha.2024.01.006
Yang Z., Yang X., Wang M., Jia R., Chen S., Liu M., et al. (2024). Genome-wide association study reveals serovar-associated genetic loci in Riemerella anatipestifer. BMC Genomics 25, 57. doi: 10.1186/s12864-024-09988-4
Keywords: anti-bacterial, Streptomyces, zinc sulfate, transcriptome, Staphylococcus aureus, Riemerella anatipestifer
Citation: Hassan SSu and Jin H (2025) Harnessing marine bacteria for next-gen antibiotics: potent inhibition of S. aureus and Riemerella anatipestifer through in vitro, omics, and chemoinformatics approach with enhanced production of secondary metabolites through zinc sulfate. Front. Mar. Sci. 11:1461607. doi: 10.3389/fmars.2024.1461607
Received: 08 July 2024; Accepted: 29 November 2024;
Published: 06 January 2025.
Edited by:
Syed Qamar Abbas, Sarhad University of Science & Information Technology (SUIT), PakistanReviewed by:
Aziz Eftekhari, Ege University, TürkiyeIsrar Fatima, Government College University, Faisalabad, Pakistan
Ata Ur Rehman, Duke University, United States
Copyright © 2025 Hassan and Jin. This is an open-access article distributed under the terms of the Creative Commons Attribution License (CC BY). The use, distribution or reproduction in other forums is permitted, provided the original author(s) and the copyright owner(s) are credited and that the original publication in this journal is cited, in accordance with accepted academic practice. No use, distribution or reproduction is permitted which does not comply with these terms.
*Correspondence: Syed Shams ul Hassan, U2hhbXMxMzI3QHlhaG9vLmNvbQ==; Huizi Jin, S2ltaHpAc2p0dS5lZHUuY24=