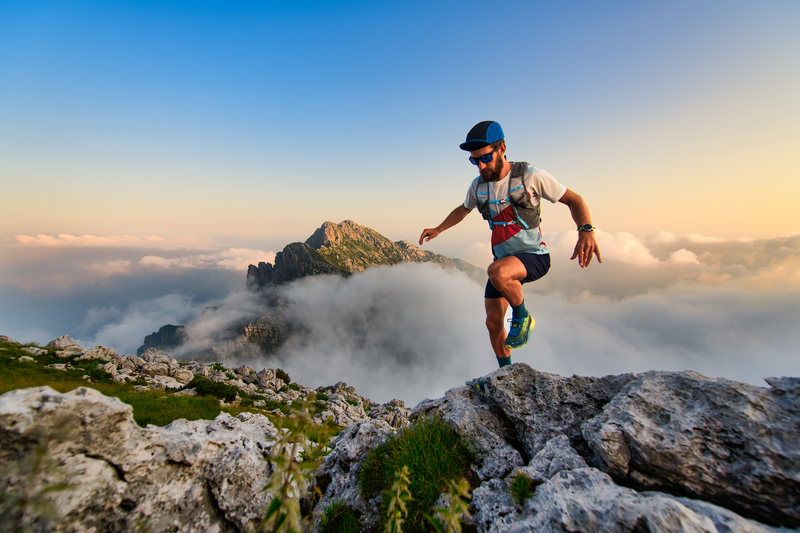
95% of researchers rate our articles as excellent or good
Learn more about the work of our research integrity team to safeguard the quality of each article we publish.
Find out more
ORIGINAL RESEARCH article
Front. Mar. Sci. , 04 September 2024
Sec. Aquatic Microbiology
Volume 11 - 2024 | https://doi.org/10.3389/fmars.2024.1458853
This article is part of the Research Topic Biological Invasions in Aquatic Ecosystems: Detection, Assessment and Countermeasures View all 12 articles
Achieving carbon neutrality in wastewater treatment plants relies heavily on mainstream anaerobic ammonia oxidation. However, the stability of this process is often compromised, largely due to the significant influence of microbial morphology. This study analyzed 208 microbial samples using bioinformatics and machine learning (ML) across four different morphologies: Suspended Sludge (SS), Biofilm, Granular Sludge (GS) and the Integrated Fixed-film Activated Sludge process (IFAS). The results revealed IFAS’s notably complex and stable community structure, along with the identification of endemic genera and common genera among the four microbial morphologies. Through co-occurrence network analysis, the interaction between microorganisms of various genera was displayed. Utilizing the Extreme Gradient Boosting (XGBoost) model, a ML modeling framework based on microbiome data was developed. The ML-based feature importance analysis identified LD-RB-34 as a key organism in SS and BSV26 was an important bacterium in IFAS. Additionally, functional bacteria KF-JG30-C25 occupied a higher proportion in GS, and Unclassified Brocadiaceae occupied a higher proportion in Biofilm. Furthermore, dissolved oxygen, temperature and pH were identified as the primary factors determining microbial communities and influencing anammox activity. Overall, this study deepens our understanding of bacterial communities to enhance the mainstream anammox nitrogen removal.
Conventional biological nitrogen removal technologies necessitate significant energy inputs for oxygen supply during nitrification and frequently rely on supplementary organic matter to enable denitrification (Rahimi et al., 2020). Employing conventional nitrification-denitrification for wastewater treatment with limited organic carbon proves to be economically burdensome due to the significant energy demands of aeration and the necessity for added chemical oxygen demand (COD) to achieve for denitrification. Consequently, the development of new nitrogen removal technologies that can conserve both energy and carbon sources is of significant practical importance (Sun et al., 2018). In contrast, the anaerobic ammonia oxidation (anammox) process is acknowledged as a more efficient and energy-conserving method for wastewater treatment (Lackner et al., 2014; Wu et al., 2022). Functioning under anaerobic or oxygen-limited conditions, this process utilizes nitrite nitrogen as an electron acceptor and employs anammox bacteria (AnAOB) to directly convert ammonia nitrogen to nitrogen (Lawson et al., 2017; Zhu et al., 2023). Owing to the autotrophic nature and slow growth rate of AnAOB, additional carbon sources are not required in the nitrification reaction. Consequently, the anammox process demonstrates energy-saving characteristics and significantly reduces sludge production (Yin et al., 2022; Li et al., 2024). To meet the demand for increasingly stringent standards in an environment-friendly manner, the application of anammox in municipal wastewater treatment has become a focal point of research (Lotti et al., 2015; Laureni et al., 2016).
While the fundamental reaction mechanism of anammox has been established and its feasibility in sewage treatment has been demonstrated, the understanding of microbial interactions within anaerobic anammox systems remains significantly limited (Laureni et al., 2015; Li et al., 2019). AnAOB engage in quorum sensing by secreting and sensing specific signaling molecules such as N-acyl homoserine lactones (AHLs). These molecules can activate or inhibit the expression of particular genes at specific concentrations, thereby regulating the metabolic activity and growth state of the bacteria. Additionally, different morphological forms of AnAOB have been observed in biological treatment systems, exhibiting varying nitrogen removal performances and microbial community structures (Yue et al., 2019). Although the performance of various anammox sludge morphologies formed in numerous independent systems or under different operating conditions has been studied, the differences in their microbial community composition have not been comprehensively compared under these different morphological conditions.
The differences in stability, treatment efficiency and microbial diversity of different microbial morphology might have important effects on process optimization. Research on the four microbial morphologies can provide a deeper understanding of the mechanisms of these influencing factors, thereby utilizing the research findings to optimize the anammox process and enhance nitrogen removal efficiency in wastewater treatment. This study revealed variations in microbial flora among different morphologies during the anammox process through comparative analysis and employed bioinformatics methodologies to investigate the distinctions in bacterial communities across four microbial morphologies. Through systematic analysis of 16S rRNA gene sequences from 208 extracted samples of different microbial morphologies, common bacterial phylum and genus were identified, elucidating the interrelationships among various taxa. Machine Learning (ML) techniques were employed to identify key microbial species in various microbial morphologies and to study the contribution of bacterial abundance to different microbial forms under mainstream conditions. Additionally, the XGBoost model was leveraged to highlight microbial communities with significant contributions in various microbial morphologies within the anammox process and to delineate the influential environmental factors (e.g., COD, temperature, pH, dissolved oxygen) shaping bacterial community structures. Multiple analytical methods and the use of machine learning algorithms to analyze high-throughput sequencing data contributed to a deeper understanding of microorganisms in anaerobic anammox systems. These findings aimed at enhancing our understanding of bacterial communities in anammox systems, developing and evaluating machine learning models based on microbial community data and environmental conditions, to improve the accuracy and efficiency of classification of microbial morphology, and further provide insights for improving nitrogen removal efficiency in wastewater treatment.
To obtain 16S rRNA gene sequencing data from four different morphologies of bacterial communities in biological wastewater treatment systems, the Web of Science database was used to retrieve published studies, and additional data were sourced from the NCBI Sequence Read Archive (Sayers et al., 2022). A total of 208 samples from 2017 to 2023 were included, comprising SS (42 samples), GS (30 samples), biofilm (103 samples), and IFAS (33 samples). Detailed information and sequencing data accession numbers for the four different morphologies of bacterial communities in biological wastewater treatment systems were provided in Supplementary Tables S1 and S2.
All 16S rRNA gene sequences were processed and analyzed using USEARCH (v.10.0.240) (Edgar, 2010). The parameters –fastq_minlen 400 and –fastq_trunclen 400 were applied to remove primer binding, and low-quality reads were filtered using –fastq_maxee 1.0 (Kuczynski et al., 2011). Following quality control, the representative sequence of each OTU was taxonomically classified using the Silva 138 Classifier, and operational taxonomic unit (OTU) clustering was performed at a 97% identity threshold (Wu et al., 2019). The categorical distribution profiles of bacterial at the genus level across individual samples were aggregated for comprehensive statistical assessment and visual representation. The resultant OTU count table served as the foundation for subsequent ML modeling efforts. To characterize the incidence and abundance of distinct bacterial genera, several descriptive terms were introduced: “frequently observed genera” were defined as those observed in the top 50% of all samples; “frequent abundance genera” were defined as those with a relative abundance of > 5% in the top 50% of samples; and “frequently observed but low abundance genera” were defined as those with a relative abundance of < 2% in the top 50% of the samples.
Alpha diversity analysis of various microbial morphologies was conducted using the ggpubr and ggplot2 packages in R, enabling the visualization and comparison of species richness and Simpson’s diversity. The Mann-Whitney U test was applied to discern significant differences. Bray-Curtis distance-based Principal Coordinates Analysis (PCoA) and Adonis analysis were performed in R utilizing the vegan package. The abundance distribution of predominant genera within the various microbial morphologies was visually represented using violin plots, with the PERMANOVA employed to assess the statistical significance of differences among the four microbial morphologies. Exploration of inter-genus correlations within different microbial morphologies was facilitated through co-occurrence network analysis using R and Gephi. Spearman’s correlation coefficient ρ > 0.7 and significance level p < 0.05 were utilized as criteria to discern strong correlations and statistically significant relationships.
ML methodologies have emerged as powerful tools for discerning microbial community variations and investigating the impact of diverse environmental factors on bacterial populations. In this study, relative abundance data from microbial samples at the genus level were subjected to ML training and modeling processes using four distinct models: Logistic Regression (LR), Support Vector Machine with Linear Kernel (SVML), Support Vector Machine with Radial Basis Function Kernel (SVMRBF), and Extreme Gradient Boosting (XGBoost) (Cai et al., 2019). To optimize the performance of the ML models, this study employed the holdout method to segment the data set (randomly dividing it into an 80:20 training-to-testing ratio). GridSearchCV was utilized for hyperparameter tuning. GridSearchCV is a universal hyperparameter searcher which can automate the search for optimal parameters using an exhaustive search method, ensuring that no potential combinations are missed and thereby guaranteeing the identification of the global optimal solution. This approach saved time and effort compared to manual hyperparameter tuning. Subsequently, five-fold cross-validation was implemented to compute the average accuracy and Area Under the Curve (AUC) metrics for model evaluation and facilitate visual analysis (Figure 1A). Five-fold cross-validation fully utilized the data, reduced random error, and enhanced the stability and reliability of model performance evaluation. Among these, SVML and LR were linear models, XGBoost was a decision tree-based model, and SVMRBF was based on the radial basis function (RBF) methodology. SVMRBF uses the same support vector machine as SVML but is based on the radial basis function (RBF) methodology. The results of these models would be discussed in detail in the results section, especially in identifying key microorganisms in different morphologies. All four models were suitable for various data scenarios, and thus, the most appropriate model should be determined based on the accuracy and AUC values derived from the classification predictions of microbial morphology facilitated by each feature. The AUC was derived from the area under the ROC curves of each model through integration, which were plotted using the true positive rate (TPR) and false positive rate (FPR).
Figure 1. The performance of ML models utilizes microbiome data to determine the significance of each feature. (A) Classification prediction accuracy and AUC of the four ML models; (B) ROC curves of the four ML models. The confusion matrix illustrates the prediction accuracy of the four ML models (C). LR, (D) SVML, (E) SVMRBF, (F) XGBoost).
Additionally, feature importance in the classification predictions of all models was calculated using SHapley Additive exPlanations (SHAP). SHAP provided a unified framework that can explain the outputs of any machine learning model due to its model-agnostic applicability, and capability to provide both local and global interpretability, as previously described (Lundberg et al., 2020). SHAP calculated the marginal contribution of each feature to the prediction output in different combinations and finally derived the average contribution value of each feature. After obtaining the SHAP values, SHAP v0.45.1 (https://github.com/shap/shap) was used to generate dot plots. These plots illustrated feature importance and the magnitude of positive and negative contributions of each feature by showing the SHAP values for different samples in each model. In the plots, each point represented a SHAP value of a specific feature in a sample, red dots indicated positive contributions, while blue dots represented negative contributions. Finally, the XGBoost algorithm was employed to assess the individual contributions of bacterial taxa to the classification outcomes for distinct microbial morphologies (SS, GS, Biofilm, IFAS), pinpointing the most influential bacteria driving the classification results for each morphological group. Furthermore, feature importance analysis of environmental factors impacting different microbial morphologies was conducted using the XGBoost Classifier.
An analysis of bacterial communities within four distinct microbial morphologies was performed by examining 208 16S rRNA gene sequencing datasets sourced from 34 studies. As shown in Figure 2, the Simpson Diversity index of IFAS systems was significantly higher than that of other systems, indicating higher diversity and complexity in microbial community composition.
Figure 2. This study conducted a comprehensive analysis of four microbial morphologies. (A) Comparison of the Simpson index among the four microbial morphologies; (B) Comparison of species richness (Richness Index) among the four microbial morphologies; (C) PCoA of four microbial morphologies based on phylum level Bray-Curtis distance; (D) PCoA of four microbial morphologies based on genus level Bray-Curtis distance.
The richness index provided a more intuitive representation of biodiversity across the four microbial morphologies (Figure 2B). The presence of outliers in all groups indicated occasional significant deviations in species richness. Notably, the richness index distribution in the SS and biofilm groups showed a wide range, reflecting substantial variability in species abundance. In contrast, the IFAS group exhibited a higher and more stable richness index, indicating uniform numbers of species. The GS group exhibited the lowest richness index among the morphologies, with minimal variation, consistent with the results of the Simpson analysis.
Cluster analysis showed that differences in microbial community structure were not particularly significant at phylum and genus levels (Figures 2C, D). The two-dimensional distribution and clustering of samples in PCoA space indicated that the community structure was similar. IFAS samples demonstrated close relationships with each other, while SS samples exhibited greater dispersion, suggesting a higher degree of similarity within IFAS microbial communities. The variance interpretation ratio of PCoA at the genus level was lower than that at the phylum level, indicating higher community structure complexity at the genus level and more pronounced separation at the phylum level.
A comprehensive examination revealed a total of 1217, 693, 458, and 702 genera in SS, biofilm, GS, and IFAS samples, respectively (Figure 3A). Among them, SS had the highest number of unique bacterial genera at 453, while GS had the lowest at 29. The unique bacterial genera in biofilm and IFAS were 77 and 80, respectively. Additionally, 285 genera were identified in the overlapping central regions across all microbial morphologies, indicating their ubiquity within these systems. Conversely, fewer genera were shared between specific groups, highlighting the distinctiveness of microbial compositions in each morphology.
Figure 3. Comparison of bacteria genera across four distinct sludge types. (A) Venn diagram illustrating the shared and unique genus, (B) Total relative abundance of specific genus, (C) Number of frequently observed genus, categorized as frequently abundant, frequently observed but low abundance, and other genus, (D) Total relative abundance of frequently observed genus.
This study presented a detailed examination of the relative abundances of specific genera (Figure 3B) and compared the top 10 most endemic species among the four microbial morphologies (Supplementary Table S3). While the relative abundances of endemic species were generally low, biofilm and GS displayed significantly higher relative abundance values, suggesting a prevalence of certain endemic bacteria within these morphologies. Conversely, IFAS and SS exhibited lower relative abundance values with more concentrated distributions.
Furthermore, a survey of common genera based on their occurrence frequency across diverse morphologies was conducted, and stacked bar charts were used to visualize the distribution of genera within the four microbial morphologies (Figure 3C). The enrichment analysis of frequently observed genera in SS, biofilm, GS, and IFAS revealed that these genera accounted for 17.7%, 14.1%, 2.8%, and 27.2% of the total sequences, respectively (Figure 3D). The analysis highlighted the presence of genera frequently observed across different morphologies, albeit with low abundance. Noteworthy observations included the identification of specific abundant genera in each morphology, such as SBR1031 and Thauera in SS; Candidatus Brocadia, Nitrospira, and Truepera in biofilm, Denitratisoma, Nitrospira, and Truepera in GS; and Ignavibacterium and unclassified_Comamonadaceae in IFAS. Notably, Candidatus Brocadia, one of the main AnAOB in biofilms, utilizes ammonia and nitrite as substrates to convert them into nitrogen through the anammox process. This process helps reduce the nitrogen load in water bodies. Candidatus Brocadia often coexists with other microorganisms, and the formation of biofilms enhances its stability and survival in the environment (Okabe et al., 2021). These findings suggested that genus frequently enriched within microbial systems tend to exhibit higher relative abundances, potentially playing a dominant role in shaping the ecological dynamics of these systems. These discrepancies in microbial composition among different morphologies indicated significant impacts on the interactions and functions within the microbial systems.
To explore the intricate relationships among bacteria inhabiting different microbial morphologies, a detailed co-occurrence network analysis was conducted, signifying frequent interactions and interdependencies among different genera (Figure 4). The clustering of bacteria within the network indicated shared functional roles or cooperative dynamics among the co-occurring genera. Larger clusters indicated heightened complexity in interactions or a more extensive degree of connectivity within the corresponding group.
Figure 4. Co-occurrence analysis of frequently observed genus across different microbial morphologies. Each node represents a genus, with node size proportional to its degree and color indicating frequency of occurrence [(A) SS, (B) Biofilm, (C) IFAS].
Figure 4A provided a comprehensive visualization of the interaction network among SS genera, offering insights into the intricate relationships spanning the four distinct microbial morphologies. Noteworthy genera such as Candidatus_Competibacter and Nitrosomonas emerged as key nodes within the network, exhibiting prominence in terms of their connectivity. Central nodes including Candidatus_Kuenenia and RBG-13-54-9 displayed extensive interactions with numerous other nodes, underscoring their pivotal roles and contributions to the microbial ecosystem. Conversely, peripheral nodes like OLB14, Saccharimonadales, and Ignavibacterium exhibited fewer connections, suggesting niche-specific roles or less frequent interactions within the network. Candidatus_Kuenenia, SBR1031, and OLB13 formed distinct clusters within the IFAS network, indicative of unique interactions and interdependencies within these specific groups (Figure 4B). Figure 4C elucidated the co-occurrence dynamics among IFAS genera, with genera such as Truepera and Thauera occupying pivotal positions within the network, followed by Candidatus_Kuenenia and Truepera. The central nodes, Candidatus_Kuenenia and Nitrosomonas, within the Biofilm genera played crucial roles, acting as significant nodes within their respective clusters. Candidatus_Brocadia also emerged as a central connector, manifesting essential interactions within the network. While other nodes displayed comparatively fewer connections, they nonetheless played significant roles within their designated clusters, with SM1A02 demonstrating niche-specific or less frequent interactions. In the GS co-occurrence network, data generation was notably absent, potentially due to variances in bioinformatics tools and algorithms leading to biased interpretations of the data.
The robust correlations observed between bacterial genera within SS and IFAS underscore the intricate interactions prevailing within these systems. However, these interactions may not be consistently preserved when these bacteria are cultured in diverse bioreactor environments (Ma et al., 2023). Taxonomic analysis of the co-occurrence network highlighted the prevalence of bacterial genera affiliated with Proteobacteria, Firmicutes, and Bacteroidetes, likely due to the dominance of these phylum among microbial populations (Figures 4B, C). Candidatus_Competibacter, renowned for its role in enhanced biological phosphorus removal processes, exhibited higher abundance in SS compared to Biofilm and IFAS. Notable aerobic ammoxidation bacteria such as Nitrosomonas and the Candidatus_Kuenenia were more pronounced in IFAS, also manifesting presence in Biofilm and AS but to a lesser extent (Welles et al., 2016). Truepera, recognized for its denitrification capabilities, demonstrated greater prevalence in IFAS than in Biofilm. Furthermore, OLB13, a core microbial constituent in anaerobic nitrification processes, exhibited varying abundances across different microbial morphologies, with slightly higher representation in IFAS. Noteworthy interactions, such as the symbiotic relationship between OLB13 and AnAOB as a protective mechanism in harsh environments, further underscored the intricate ecological dynamics at play (Liu et al., 2024).
This study employed machine learning modeling methods to extract key features from extensive microflora data, establish predictive models, and enhance the efficiency of data analysis. The predictive performance of each ML model was evaluated using the metrics of AUC and accuracy (Figure 1). The LR model exhibited an average accuracy of approximately 84%, the SVML model demonstrated an average accuracy of around 86%, the SVMRBF model achieved an accuracy of about 83%. The XGBoost model outperformed all others with the highest accuracy of approximately 94%.
The predictive performance of the models was visually depicted through ROC curves, which reaffirmed the superior efficacy of the XGBoost model (Figure 1B). By plotting the TPR against the FPR at varying thresholds, the ROC curves revealed that all models approached the upper left corner, indicating their excellent classification efficacy across different thresholds. Notably, the XGBoost model exhibited the most outstanding performance, achieving a remarkable 98% AUC. The LR and SVMRBF models trailed slightly behind with 92% AUC, and the SVML model attained 93% AUC. These results decisively favored the XGBoost classification model as the optimal choice.
Moreover, the classification prediction performance of the four models was comprehensively assessed using confusion matrix heat maps (Figures 1C–F). The data in the blocks of the confusion matrix represented the comparison between the predicted results and the actual results in a classification task. The majority of misclassifications were observed between Biofilm and IFAS systems, accounting for approximately 2-3% of all forecasts, whereas fewer erroneous predictions were noted between GS and other morphologies. The difficulty in accurately classifying Biofilm and IFAS could be attributed to their relatively similar community structures (Figure 2). It was evident that the intricate interplay among various microbial morphologies influences the predictive efficacy of ML models concerning operational characteristics. Overall, all models exhibited predictive accuracy, with XGBoost demonstrating superior performance in terms of accuracy, AUC, and minimal classification errors. XGBoost exhibited significant performance advantages due to its incorporation of regularization techniques to prevent overfitting, built-in handling of missing values, efficient tree pruning algorithms, parallel processing capabilities, advanced methods for addressing imbalanced data. These features collectively enhanced model’s efficiency, accuracy, and robustness in handling complex data scenarios, making it a superior choice for many machine learning tasks.
The XGBoost model was employed to conduct feature importance analysis on the OTU count table, derived from summarizing the classification distribution profiles of bacteria at the genus level for each sample (Figure 5). LD-RB-34 emerged as the most influential feature impacting the SS type, as shown in Figure 5A. The norank_f_LD-RB-34 facilitates the anammox process by engaging in the PD process, utilizing nitrate as an electron acceptor, reducing it to nitrite, and providing the essential nitrite for AnAOB (Wu et al., 2023). This nitrite accumulation is facilitated by the synergistic activity of multiple microorganisms, not merely a single genus. Genera such as Candidatus Competibacter and Defluviicoccus, both capable of denitrification, also contribute to the PD process. The combined action of these genera results in efficient nitrite accumulation, which is crucial for subsequent anammox processes (Li et al., 2020). Candidatus_Kerfeldbacteria belongs to the candidate phylum. Candidate phylum represent a group of microorganisms that have not yet been cultured or sufficiently characterized for classification. Although the current study indicates that Candidatus_Kerfeldbacteria is not among the known genera involved in the anammox process, its presence may be indirectly related to the anammox process. Peredibacter is an obligate predatory bacterium that attacks its prey through efficient locomotion, attaches to the outer membrane of prey cells, and preys exclusively on other Gram-negative bacteria via epibiotic predation or by penetrating the periplasmic space of the prey (epigenetic predation) (Davidov and Jurkevitch, 2004). By preying on Gram-negative bacteria involved in the anammox process, Peredibacter can regulate the population dynamics and structure of AnAOB, thereby playing a crucial role in anammox (Ezzedine et al., 2020). Candidatus_Competibacter, a member of the glycogen-accumulating organisms (GAOs), does not directly participate in phosphorus removal, but plays a critical role in the Enhanced Biological Phosphorus Removal (EBPR) wastewater treatment system (McIlroy et al., 2014). As they compete for resources with polyphosphate accumulators organisms (PAOs), the competition between GAOs and PAOs can be optimized by adjusting the operating conditions of EBPR system to enhance its phosphorus removal efficiency (Song et al., 2022).
Figure 5. The importance of the microbial flora in predictive decision-making is ranked based on their characteristics. The XGBoost model was employed to estimate the mean absolute SHAP values for each bacterial population [(A) SS, (B) GS, (C) Biofilm, (D) IFAS] and to generate the corresponding SAP value dot plots.
In the GS system, the predominant bacterial taxa included KF-JG30-C25, Pla4_lineage, and Fimbriimonadaceae. According to previous studies, KF-JG30-C25 is pertinent to the study of soil microbial communities, particularly in stable isotope detection (SIP) experiments, which are employed to identify the Acidobacteria Granulicella sp. The microbial community associated with extracellular polymer substances (EPS) produced by strain WH15 constitutes a group of bacteria potentially significant in soil microbial communities, potentially playing a role in the metabolism and nutrient cycling of soil organic matter (Costa et al., 2020). Notably, Pla4_lineage achieves biological nitrogen removal in anammox by converting ammonia into nitrogen through anammox process, which is similar to other planctomycetes communities, and this process contributes significantly to the global nitrogen cycle, especially in the Marine environment (Wiegand et al., 2020). The Fimbriimonadaceae family showed a significantly correlation with ammonia removal, suggesting that it may contain ammonia-oxidizing bacteria groups or have positive interactions with ammonia-oxidizing bacteria, thereby facilitating the anammox process (de Celis et al., 2020).
Unclassified Brocadiaceae, classified as a genus of AnAOB, emerges as the predominant genus exerting the most substantial influence on the Biofilm type. Notably, fluctuations in the abundance of Unclassified Brocadiaceae have been used as an indicator of anaerobic ammonium oxidation reactor stability in previous studies (Deng et al., 2023). Thus, although the specific classification of these microorganisms remains pending, their presence and variations play a pivotal role in monitoring and assessing the operational efficiency of anammox systems (Yang et al., 2021). In the biofilm ecosystem, Thiobacillus orchestrates energy production via sulfide oxidation, fostering the growth and metabolism of diverse microbial communities (Lopez-Fernandez et al., 2023). Moreover, the uncultured OM190 group within the Planctomycetes phylum is significant in the nitrogen cycle, particularly associated with genes involved in nitrogen oxide reductase, nitrogenase, and hydroxylamine reductase pathways (Ludington et al., 2017). Additionally, bacteria such as Thauera, part of the Betaproteobacteria class, are gram-negative bacteria actively engaged in denitrification processes, catalyzing nitrate reduction to nitrogen and enhancing nitrogen cycle dynamics (Rujakom et al., 2023).
In the context of IFAS type prediction, BSV26 emerges as a resilient microorganism exhibiting robust environmental adaptability, crucial for sustained proliferation and maintenance within the IFAS ecosystem. Its presence significantly impacts the system’s stability and metabolic functionality (Bai et al., 2022). Moreover, the involvement of CCM19a in phosphorus removal processes underscores its crucial role in environmental remediation strategies (Stokholm-Bjerregaard et al., 2017). The Unclassified_Comamonadaceae genus, a member of the Proteobacteria phylum, is a dominant bacterium genus integral to denitrification processes. It collaborates with diverse microbial cohorts within the system, contributing to hydrogen autotrophic denitrification processes that are critical for sewage treatment and nitrogen elimination (Lu et al., 2024). Furthermore, Flavobacterium bacteria, characterized as gram-negative microbes, are prominently associated with biofilm formation, organic matter decomposition, and significantly contribute to the carbon cycle within aquatic ecosystems (Kolton et al., 2016).
In addition to genus level taxonomic data for bacterial groups, this study utilized machine learning models to identify environmental factors that significantly influence microbial morphology (Figure 6A). The findings derived from the XGBoost classification model highlighted the critical role of DO in shaping microbial community dynamics (Figure 6B), the x-axis represented the feature importance of each feature, which means the degree of contribution of each feature to the model prediction results. AnAOB exhibited heightened sensitivity to variations in DO levels due to their metabolic functions in hypoxic environments. Elevated concentrations of DO were shown to potentially hinder AnAOB activity and viability. Experimental observations indicated that a properly increase in DO concentration (e.g., from 0.4 to 0.6 mg/L) could stimulate AnAOB growth without detrimental effects. This phenomenon may be attributed to increased nitrite availability at higher DO levels, thereby facilitating the proliferation of AnAOB (Zhao et al., 2023). AOB and NOB, functioning aerobically to oxidize ammonia and nitrite, were notably influenced by DO levels. Suboptimal DO conditions could impede the growth and activities of these bacteria. Therefore, properly DO management represents a key modulatory factor for optimizing the Anammox process, enhancing biological nitrogen removal efficiency, and mitigating competition from AOB and NOB for substrates (Duan et al., 2024).
Figure 6. The performance of ML modeling utilizes environmental condition data to determine the significance of each feature. (A) Classification prediction accuracy and AUC of four ML models. (B) Estimation of the mean absolute SHAP value for each environmental factor using the XGBoost model.
Temperature and pH emerged as pivotal determinants closely linked to microbial community predictions within the ML framework. To date, more than 20 anammox species have been discovered, each exhibiting varying adaptabilities to temperature (Lin et al., 2018; Nsenga Kumwimba et al., 2020). Temperature fluctuations modulate the metabolic pathways of AnAOB, with optimal performance typically observed within the range of 35°C to 40°C (Tomaszewski et al., 2017b). Temperature alterations can induce shifts in AnAOB metabolic pathways, thereby influencing nitrogen removal efficacy. The anammox process is also affected by short-term disturbances in DO under two different temperature regimes (Niederdorfer et al., 2021). Furthermore, AnAOB exhibited heightened sensitivity to pH variations. The optimal pH range for AnAOB is typically between 6.7 and 8.3, with high and low pH values exerting varying degrees of inhibitory effects on the anammox community. Precise pH control within a narrow band (7.2 to 7.6) is advocated to maintain consistent anammox performance and prevent substrate inhibition (Zhao et al., 2023). Temperature and pH fluctuations not only individually impact the anammox process but also create synergistic effects on parameters such as free ammonia and free nitrite content. Some studies have shown that the interaction effect of temperature and pH has an important effect on anammox activity. For example, a central combinatorial design experiment found that higher pH levels result in increased anammox activity at low temperatures (Daverey et al., 2015). Tomaszewski also indicated that pH has a more significant effect on anammox activity at lower temperatures (Tomaszewski et al., 2017a). Additionally, fluctuations in temperature and pH not only individually impact the anammox process but also create synergistic effects on parameters such as free ammonia and free nitrite content (Daverey et al., 2015). Therefore, enhancing anammox activity by adjusting temperature and pH can promote synergistic interactions with microorganisms and optimize substrate utilization, thereby improving nitrogen removal efficiency in water treatment applications.
In this study, bioinformatics and ML methods were used to analyze the microbial communities of four different forms of anammox systems. The results of bioinformatics analysis showed that the composition of microbial communities in the IFAS system had great diversity and complexity, and there were complex interactions and associations among different bacterial genera. In machine learning modeling, in particular the XGBoost model, feature importance analysis was performed to identify the most influential bacterial genera and environmental factors that influenced the classification results of various microbial morphology. The results demonstrated that changes in DO, temperature, and pH are the crucial factors influencing microbial community composition across various forms and altering anammox activity. Overall, these findings enhanced the understanding of the differences in the four different forms of bacterial communities during anammox, and also provided an important theoretical basis for improving wastewater treatment processes. Although four microbial morphologies have been analyzed using bioinformatics and ML methods in this study, there are few existing experimental studies and a lack of long-term experimental validation in actual wastewater treatment systems. Long-term operational experiments applied to real wastewater treatment systems in the future would help to validate the findings of this study and provide strong support for more efficient and stable wastewater treatment.
The original contributions presented in the study are included in the article/Supplementary Material. Further inquiries can be directed to the corresponding authors.
SZ: Writing – review & editing, Writing – original draft, Software, Methodology, Investigation, Data curation, Conceptualization. WZ: Writing – review & editing, Writing – original draft, Software, Methodology, Investigation, Data curation, Conceptualization. YH: Writing – review & editing, Supervision, Methodology, Conceptualization. TZ: Writing – review & editing, Supervision, Methodology, Conceptualization. ZJ: Writing – review & editing, Supervision, Methodology. MZ: Writing – review & editing, Supervision, Methodology. NW: Writing – review & editing, Supervision, Methodology.
The author(s) declare financial support was received for the research, authorship, and/or publication of this article. This research was financially supported by the National Natural Science Foundation of China (22276135).
This research was financially supported by the National Natural Science Foundation of China (22276135).
The authors declare that the research was conducted in the absence of any commercial or financial relationships that could be construed as a potential conflict of interest.
All claims expressed in this article are solely those of the authors and do not necessarily represent those of their affiliated organizations, or those of the publisher, the editors and the reviewers. Any product that may be evaluated in this article, or claim that may be made by its manufacturer, is not guaranteed or endorsed by the publisher.
The Supplementary Material for this article can be found online at: https://www.frontiersin.org/articles/10.3389/fmars.2024.1458853/full#supplementary-material
Bai L., Liu X., Hua K., Tian L., Wang C., Jiang H. (2022). Microbial processing of autochthonous organic matter controls the biodegradation of 17α-ethinylestradiol in lake sediments under anoxic conditions. Environ. pollut. 296, 118760. doi: 10.1016/j.envpol.2021.118760
Cai W., Lesnik K. L., Wade M. J., Heidrich E. S., Wang Y., Liu H. (2019). Incorporating microbial community data with machine learning techniques to predict feed substrates in microbial fuel cells. Biosens Bioelectron 133, 64–71. doi: 10.1016/j.bios.2019.03.021
Costa O. Y. A., Pijl A., Kuramae E. E. (2020). Dynamics of active potential bacterial and fungal interactions in the assimilation of acidobacterial EPS in soil. Soil Biol. Biochem. 148, 107916. doi: 10.1016/j.soilbio.2020.107916
Daverey A., Chei P. C., Dutta K., Lin J. G. (2015). Statistical analysis to evaluate the effects of temperature and pH on anammox activity. Int. Biodeterioration Biodegradation 102, 89–93. doi: 10.1016/j.ibiod.2015.03.006
Davidov Y., Jurkevitch E. (2004). Diversity and evolution of Bdellovibrio-and-like organisms (BALOs), reclassification of Bacteriovorax starrii as Peredibacter starrii gen. nov., comb. nov., and description of the Bacteriovorax-Peredibacter clade as Bacteriovoracaceae fam. nov. Int. J. Syst. Evol. Microbiol. 54, 1439–1452. doi: 10.1099/ijs.0.02978-0
de Celis M., Belda I., Ortiz-Álvarez R., Arregui L., Marquina D., Serrano S., et al. (2020). Tuning up microbiome analysis to monitor WWTPs’ biological reactors functioning. Sci. Rep. 10, 4079. doi: 10.1038/s41598-020-61092-1
Deng J., Xiao X., Li Y.-Y., Liu J. (2023). Low-carbon nitrogen removal from power plants circulating cooling water and municipal wastewater by partial denitrification-anammox. Bioresource Technol. 380, 129071. doi: 10.1016/j.biortech.2023.129071
Duan C., Zhang Q., Li J., Feng W., Zhang L., Peng Y. (2024). Partial nitrification response to dissolved oxygen variation and aerobic starvation: Kinetics and microbial community analyses. Chem. Eng. J. 481, 148621. doi: 10.1016/j.cej.2024.148621
Edgar R. C. (2010). Search and clustering orders of magnitude faster than BLAST. Bioinformatics 26, 2460–2461. doi: 10.1093/bioinformatics/btq461
Ezzedine J. A., Chardon C., Jacquet S. (2020). New 16S rRNA primers to uncover Bdellovibrio and like organisms diversity and abundance. J. Microbiological Methods 175, 105996. doi: 10.1016/j.mimet.2020.105996
Kolton M., Erlacher A., Berg G., Cytryn E. (2016). “The flavobacterium genus in the plant holobiont: ecological, physiological, and applicative insights,” in Microbial Models: From Environmental to Industrial Sustainability. Ed. Castro-Sowinski S. (Springer Singapore, Singapore), 189–207.
Kuczynski J., Stombaugh J., Walters W. A., González A., Caporaso J. G., Knight R. (2011). Using QIIME to analyze 16S rRNA gene sequences from microbial communities. Curr. Protoc. Bioinf. 10, 10.17.11–10.17.20. doi: 10.1002/0471250953.bi1007s36
Lackner S., Gilbert E. M., Vlaeminck S. E., Joss A., Horn H., van Loosdrecht M. C. (2014). Full-scale partial nitritation/anammox experiences–an application survey. Water Res. 55, 292–303. doi: 10.1016/j.watres.2014.02.032
Laureni M., Falås P., Robin O., Wick A., Weissbrodt D. G., Nielsen J. L., et al. (2016). Mainstream partial nitritation and anammox: long-term process stability and effluent quality at low temperatures. Water Res. 101, 628–639. doi: 10.1016/j.watres.2016.05.005
Laureni M., Weissbrodt D. G., Szivák I., Robin O., Nielsen J. L., Morgenroth E., et al. (2015). Activity and growth of anammox biomass on aerobically pre-treated municipal wastewater. Water Res. 80, 325–336. doi: 10.1016/j.watres.2015.04.026
Lawson C. E., Wu S., Bhattacharjee A. S., Hamilton J. J., McMahon K. D., Goel R., et al. (2017). Metabolic network analysis reveals microbial community interactions in anammox granules. Nat. Commun. 8, 15416. doi: 10.1038/ncomms15416
Li G., Yu Y., Li X., Jia H., Ma X., Opoku P. A. (2024). Research progress of anaerobic ammonium oxidation (Anammox) process based on integrated fixed-film activated sludge (IFAS). Environ. Microbiol. Rep. 16, e13235. doi: 10.1111/1758-2229.13235
Li J., Lou J., Lv J. (2020). The effect of sulfate on nitrite-denitrifying anaerobic methane oxidation (nitrite-DAMO) process. Sci. Total Environ. 731, 139160. doi: 10.1016/j.scitotenv.2020.139160
Li J., Peng Y., Zhang L., Liu J., Wang X., Gao R., et al. (2019). Quantify the contribution of anammox for enhanced nitrogen removal through metagenomic analysis and mass balance in an anoxic moving bed biofilm reactor. Water Res. 160, 178–187. doi: 10.1016/j.watres.2019.05.070
Lin X., Wang Y., Ma X., Yan Y., Wu M., Bond P. L., et al. (2018). Evidence of differential adaptation to decreased temperature by anammox bacteria. Environ. Microbiol. 20, 3514–3528. doi: 10.1111/1462-2920.14306
Liu F., Xu H., Shen Y., Li F., Yang B. (2024). Rapid start-up strategy and microbial population evolution of anaerobic ammonia oxidation biofilm process for low-strength wastewater treatment. Bioresource Technol. 394, 130201. doi: 10.1016/j.biortech.2023.130201
Lopez-Fernandez M., Westmeijer G., Turner S., Broman E., Ståhle M., Bertilsson S., et al. (2023). Thiobacillus as a key player for biofilm formation in oligotrophic groundwaters of the Fennoscandian Shield. NPJ Biofilms Microbiomes 9, 41. doi: 10.1038/s41522-023-00408-1
Lotti T., Kleerebezem R., Hu Z., Kartal B., de Kreuk M. K., van Erp Taalman Kip C., et al. (2015). Pilot-scale evaluation of anammox-based mainstream nitrogen removal from municipal wastewater. Environ. Technol. 36, 1167–1177. doi: 10.1080/09593330.2014.982722
Lu T., Zheng Q., Huang A., Chen J., Liu X., Qin Y. (2024). Investigation of denitrification to Anammox phase transformation performance of Up-Flow anaerobic sludge blanket reactor. Bioresource Technol. 394, 130190. doi: 10.1016/j.biortech.2023.130190
Ludington W. B., Seher T. D., Applegate O., Li X., Kliegman J. I., Langelier C. R., et al. (2017). Assessing biosynthetic potential of agricultural groundwater through metagenomic sequencing: A diverse anammox community dominates nitrate-rich groundwater. PLoS One 12, e0174930. doi: 10.1371/journal.pone.0174930
Lundberg S. M., Erion G., Chen H., DeGrave A., Prutkin J. M., Nair B., et al. (2020). From local explanations to global understanding with explainable AI for trees. Nat. Mach. Intell. 2, 56–67. doi: 10.1038/s42256-019-0138-9
Ma Y., Rui D., Dong H., Zhang X., Ye L. (2023). Large-scale comparative analysis reveals different bacterial community structures in full- and lab-scale wastewater treatment bioreactors. Water Research. 242, 120222. doi: 10.1016/j.watres.2023.120222
McIlroy S. J., Albertsen M., Andresen E. K., Saunders A. M., Kristiansen R., Stokholm-Bjerregaard M., et al. (2014). ‘Candidatus Competibacter’-lineage genomes retrieved from metagenomes reveal functional metabolic diversity. ISME J. 8, 613–624. doi: 10.1038/ismej.2013.162
Niederdorfer R., Hausherr D., Palomo A., Wei J., Magyar P., Smets B. F., et al. (2021). Temperature modulates stress response in mainstream anammox reactors. Commun. Biol. 4, 23. doi: 10.1038/s42003-020-01534-8
Nsenga Kumwimba M., Lotti T., Şenel E., Li X., Suanon F. (2020). Anammox-based processes: How far have we come and what work remains? A review by bibliometric analysis. Chemosphere 238, 124627. doi: 10.1016/j.chemosphere.2019.124627
Okabe S., Shafdar A. A., Kobayashi K., Zhang L., Oshiki M. (2021). Glycogen metabolism of the anammox bacterium “Candidatus Brocadia sinica. ISME J. 15, 1287–1301. doi: 10.1038/s41396-020-00850-5
Rahimi S., Modin O., Mijakovic I. (2020). Technologies for biological removal and recovery of nitrogen from wastewater. Biotechnol. Advances: Int. Rev. J. 43-), 43.
Rujakom S., Kamei T., Kazama F. (2023). Thauera sp. in hydrogen-based denitrification: effects of plentiful bicarbonate supplementation on powerful nitrite reducer. Sustainability 15, 277.
Sayers E. W., Bolton E. E., Brister J. R., Canese K., Chan J., Comeau D. C., et al. (2022). Database resources of the national center for biotechnology information. Nucleic Acids Res. 50, D20–d26. doi: 10.1093/nar/gkab1112
Song X., Yu D., Qiu Y., Qiu C., Xu L., Zhao J., et al. (2022). Unexpected phosphorous removal in a Candidatus_Competibacter and Defluviicoccus dominated reactor. Bioresource Technol. 345, 126540. doi: 10.1016/j.biortech.2021.126540
Stokholm-Bjerregaard M., McIlroy S. J., Nierychlo M., Karst S. M., Albertsen M., Nielsen P. H. (2017). A critical assessment of the microorganisms proposed to be important to enhanced biological phosphorus removal in full-scale wastewater treatment systems. Front. Microbiol. 8. doi: 10.3389/fmicb.2017.00718
Sun Y., Wang H., Wu G., Guan Y. (2018). Nitrogen removal and nitrous oxide emission from a step-feeding multiple anoxic and aerobic process. Environ. Technol. 39, 814–823. doi: 10.1080/09593330.2017.1311947
Tomaszewski M., Cema G., Ziembinska-Buczynska A. (2017a). Significance of pH control in anammox process performance at low temperature. Chemosphere 185, 439–444. doi: 10.1016/j.chemosphere.2017.07.034
Tomaszewski M., Cema G., Ziembińska-Buczyńska A. (2017b). Influence of temperature and pH on the anammox process: A review and meta-analysis. Chemosphere 182, 203–214. doi: 10.1016/j.chemosphere.2017.05.003
Welles L., Lopez-Vazquez C. M., Hooijmans C. M., van Loosdrecht M. C. M., Brdjanovic D. (2016). Prevalence of 'Candidatus Accumulibacter phosphatis' type II under phosphate limiting conditions. AMB Express 6, 44. doi: 10.1186/s13568-016-0214-z
Wiegand S., Jogler M., Boedeker C., Pinto D., Vollmers J., Rivas-Marín E., et al. (2020). Cultivation and functional characterization of 79 planctomycetes uncovers their unique biology. Nat. Microbiol. 5, 126–140. doi: 10.1038/s41564-019-0588-1
Wu L., Ning D., Zhang B., Li Y., Zhang P., Shan X., et al. (2019). Global diversity and biogeography of bacterial communities in wastewater treatment plants. Nat. Microbiol. 4, 1183–1195. doi: 10.1038/s41564-019-0426-5
Wu P., Chen J., Garlapati V. K., Zhang X., Wani Victor Jenario F., Li X., et al. (2022). Novel insights into Anammox-based processes: A critical review. Chem. Eng. J. 444, 136534. doi: 10.1016/j.cej.2022.136534
Wu X., Chen Y., Liu H., Ma J., Dang H. (2023). Characteristics of NO2–N accumulation in partial denitrification during granular sludge formation. Biochem. Eng. J. 194, 108817. doi: 10.1016/j.bej.2023.108817
Yang X.-R., Li H., Su J.-Q., Zhou G.-W. (2021). Anammox bacteria are potentially involved in anaerobic ammonium oxidation coupled to iron(III) reduction in the wastewater treatment system. Front. Microbiol. 12. doi: 10.3389/fmicb.2021.717249
Yin X., Wen J., Zhang Y., Zhang X., Zhao J. (2022). Long-term performance of nitrogen removal and microbial analysis in an anammox MBBR reactor with internal circulation to provide low concentration DO. Toxics 10. doi: 10.3390/toxics10110640
Yue H., Zhang Y., He Y., Wei G., Shu D. (2019). Keystone taxa regulate microbial assemblage patterns and functional traits of different microbial aggregates in simultaneous anammox and denitrification (SAD) systems. Bioresource Technol. 290, 121778. doi: 10.1016/j.biortech.2019.121778
Zhao X., Yu D., Zhang J., Miao Y., Ma G., Li J., et al. (2023). Enhancing nitrite production rate made anammox bacteria have a competitive advantage over nitrite oxidizing bacteria in mainstream anammox system. Water Environ. Res. 95, e10878. doi: 10.1002/wer.10878
Keywords: anammox, bacterial community, sludge morphology, machine learning, 16S rRNA gene
Citation: Zhou S, Zhu W, He Y, Zhang T, Jiang Z, Zeng M and Wu N (2024) A comprehensive analysis of microbial community differences in four morphologies of mainstream anaerobic ammonia oxidation systems using big-data mining and machine learning. Front. Mar. Sci. 11:1458853. doi: 10.3389/fmars.2024.1458853
Received: 03 July 2024; Accepted: 14 August 2024;
Published: 04 September 2024.
Edited by:
Shuping Wang, Chinese Research Academy of Environmental Sciences, ChinaReviewed by:
Zhibin Wang, Shandong University, ChinaCopyright © 2024 Zhou, Zhu, He, Zhang, Jiang, Zeng and Wu. This is an open-access article distributed under the terms of the Creative Commons Attribution License (CC BY). The use, distribution or reproduction in other forums is permitted, provided the original author(s) and the copyright owner(s) are credited and that the original publication in this journal is cited, in accordance with accepted academic practice. No use, distribution or reproduction is permitted which does not comply with these terms.
*Correspondence: Tianxu Zhang, MTMxMTAwMTA3OTZAMTYzLmNvbQ==; Ming Zeng, bWluZy56ZW5nQHR1c3QuZWR1LmNu
Disclaimer: All claims expressed in this article are solely those of the authors and do not necessarily represent those of their affiliated organizations, or those of the publisher, the editors and the reviewers. Any product that may be evaluated in this article or claim that may be made by its manufacturer is not guaranteed or endorsed by the publisher.
Research integrity at Frontiers
Learn more about the work of our research integrity team to safeguard the quality of each article we publish.