- 1Institute of Mechanical, Process and Energy Engineering, School of Engineering and Physical Sciences, Heriot-Watt University, Edinburgh, United Kingdom
- 2School of Geography and Environmental Sciences, Ulster University, Coleraine, Northern Ireland
- 3Department of Biogeochemistry - Biological Oceanography, GEOMAR Helmholtz Centre for Ocean Research Kiel, Kiel, Germany
- 4Changing Oceans Research Group, School of GeoSciences, University of Edinburgh, Edinburgh, United Kingdom
- 5Institute for Materials Science and Engineering, Clausthal University of Technology, Clausthal-Zellerfeld, Germany
The structural complexity of cold-water corals is threatened by ocean acidification. Increased porosity and thinning in structurally critical parts of the reef framework may lead to rapid physical collapse on an ecosystem scale, reducing their potential for biodiversity support. Understanding the structural-mechanical relationships of reef-forming corals is important to enable the use of in silico mechanical models as predictive tools that allow us to determine risk and timescales of reef collapse. Here, we analyze morphological variations of the branching architecture of the cold-water coral species Lophelia pertusa to advance mechanical in silico models based on their skeletal structure. We identified a critical size of five interbranch lengths that allows using homogenized finite element models to analyze mechanical competence. At smaller length scales, mechanical surrogate models need to explicitly account for the statistical morphological differences in the skeletal structure. We showed large morphological variations between fragments of L. pertusa colonies and branches, as well as dead and live skeletal fragments which are driven by growth and adaptation to environmental stressors, with no clear branching-specific patterns. Future in silico mechanical models should statistically model these variations to be used as monitoring tools for predicting risk of cold-water coral reefs crumbling.
1 Introduction
Cold-water coral (CWC) reefs are important ecosystem engineers, since they support high local biodiversity through the three-dimensionally (3D) complex habitat they make (Roberts et al., 2009, 2006). This structural complexity is at risk from climate-driven shifts, particularly ocean acidification. While live coral can continue to calcify under projected temperature and ocean acidification conditions (Büscher et al., 2022; Hennige et al., 2015), the dead coral skeletal framework (i.e., erected skeleton no longer covered by soft tissue and exposed to seawater) is prone to dissolution either through direct passive chemical dissolution (Hennige et al., 2015) or increasing rates of bioerosion (Büscher et al., 2022). Dissolution of the dead framework is of particular concern, as the majority of CWC habitat is typically dead coral that sits above sediment/rubble (Barnhill et al., 2023; Vad et al., 2017). Ocean acidification induced dissolution may lead to loss of material and increased porosity in structurally critical parts of the dead skeletal framework, which can lead to structural weakening and rapid physical habitat collapse on an ecosystem scale (Hennige et al., 2020; Wolfram et al., 2022), reducing the potential for biodiversity support (Barnhill et al., 2023; Kline et al., 2019).
Wolfram et al. (2022) showed that the mechanical mechanisms explaining the collapse of CWCs due to ocean acidification can be described using mathematical and computational models. There, the coral skeleton was modelled as a multiscale, polycrystalline material and the impact of ocean acidification was incorporated as an increase of porosity and a reduction in skeletal thickness (loss of material). With this model, the authors illustrated how changes due to ocean acidification led to a decrease in the loadbearing capacity of the skeleton using image-based finite element (FE) analysis. These high-fidelity image-based models of coral structures represent a powerful tool to assess the risk of collapse in a future ocean and, potentially, to estimate timepoints that are critical to reef-crumbling based on the time they are exposed to acidified water (Hennige et al., 2020). However, the computational cost of this approach together with the reduced availability of 3D image data of these corals and their reef structures restricts its use to small coral colonies and limited timepoints. The development of fast and efficient in silico models of real reef structures therefore remains essential. In combination with projections of seawater chemistry changes, such models may allow us to investigate timescales of loadbearing capacity changes as well as the impact of these changes on CWC reefs overall.
Existing models of tropical coral reefs use cantilever beam theory to evaluate the mechanical vulnerability of coral colonies (Madin and Connolly, 2006; Storlazzi et al., 2005). These models on tropical corals consider the skeletal structure uniformly throughout the entire coral colony. While the assumption of a uniform skeletal structure may hold for some tropical coral species and growthforms, it fails to account for the morphological complexity, structural heterogeneities, and skeletal density of branching corals (Chamberlain and Graus, 1975; Graus et al., 1977; Vosburgh, 1982), and in particular CWC branching corals like the cosmopolitan scleractinian species Lophelia pertusa, also referred to as Desmophyllum pertusum (Addamo et al., 2016). To model future impacts of ocean acidification on L. pertusa’s skeletal integrity, it is important to understand its material architecture (Figure 1) and the structural-mechanical relationships across length scales. Whilst the mechanical properties at the microscale have been previously studied (Hennige et al., 2020; Pasquini et al., 2015; Wolfram et al., 2022), little is known about the influence of the corallite branching arrangement on the mechanical behavior of coral colonies. The branching architecture and morphological variations of L. pertusa skeletons have important implications not only on their loadbearing capacity but also on their ability to interact with the environment and the organisms they provide shelter for (Caley and St John 1996; Paulay 1997; Cole et al., 2008). Unlike their tropical counterparts, the difficulties in accessing CWC colonies has restricted quantitative analysis of their morphological variations to linear measurements of small coral fragments that have been collected from their environment through ROVs for example, or two-dimensional measurements extracted from video data (Addamo et al., 2015; De Clippele et al., 2018; Gass and Roberts, 2011; Quattrini et al., 2017; Sanna and Freiwald, 2021). Recently, Sanna et al. (2023) demonstrated high structural variations in the shape of dead L. pertusa skeletal fragments collected across the mid-Norwegian continental shelf using X-ray computed tomography (CT). However, their analysis was restricted to volume compactness and surface complexity and did not include the shape and size of individual coral branches. To identify critical branch sizes that, in turn, allow us to formulate appropriate surrogate models to capture the mechanical weakening and structural impacts of ocean acidification on exposed CWCs, an analysis of the 3D structural variations of coral skeletons across scales is needed. More importantly, morphological differences in dead and live skeletons need to be investigated as material loss due to dissolution was observed in skeletons no longer covered by soft tissue (i.e., dead coral) (Hennige et al., 2020).
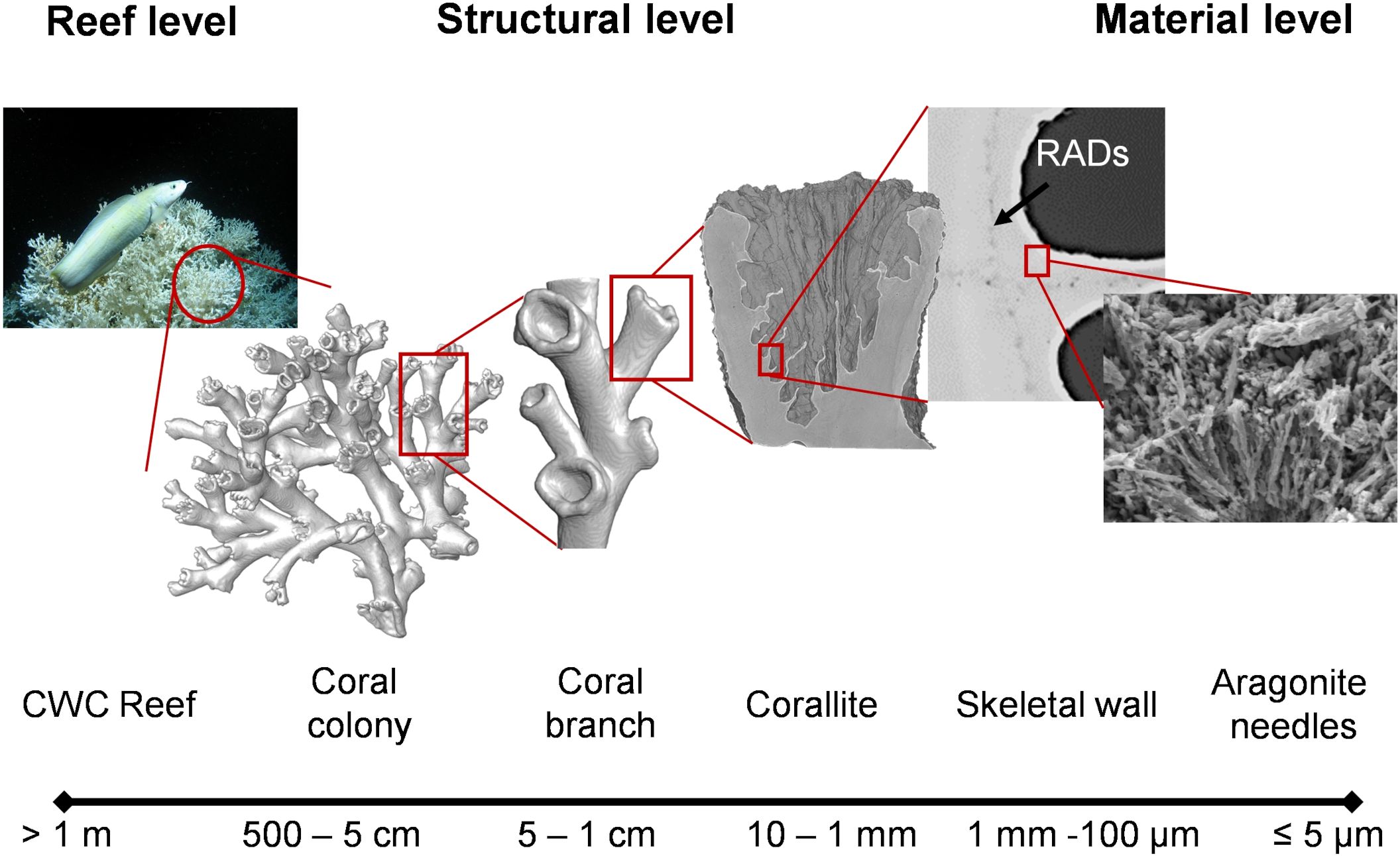
Figure 1. The material architecture of the branching cold-water coral (CWC) L. pertusa ranges from reef framework to aragonite crystal needles. L. pertusa reefs (> 1 m) extend up to 33 m high and several kilometers in diameter (Mortensen et al., 2001; Roberts et al., 2009, 2006). These reefs are formed by coral colonies (< 5 cm) that results from the concurrent growth of multiple coral branches at the structural level (Sanna and Freiwald, 2021), where branches are an assembly of corallites arranged in a fractal-like fashion at the mesoscale (~ 1 cm). The basic structural unit of the corallite’s skeletal wall (> 100 μm) is made of aragonite crystals at the material level (~ 5 μm) that protrude from rapid accretion deposits (RADs) forming thickening bands between centers of calcifications (Von Euw et al., 2017).
A potential path towards upscaling the mechanical behavior from the structural to reef length scale levels (Figure 1) relies on homogenized FE models (Hollister et al., 1994; Dirrenberger et al., 2019). However, homogenization procedures are only applicable in the case of statistically uniform materials. Therefore, they rely on the existence of a representative volume element (RVE), or at least a close approximation of it, whose analysis yields the effective material properties. As such, the effective mechanical properties of coral colonies can be captured as mean values of apparent properties of RVEs of the underlying skeletal branching structure (Harrigan et al., 1988; Pfeiffer et al., 1997). These in silico models require low computational resources and overcome previous assumptions of a uniform homogeneous structure on tropical corals (Madin and Connolly, 2006; Storlazzi et al., 2005). However, whether local effective properties can be defined within L. pertusa colonies to account for the heterogeneity of their skeletons depends on the critical size of such RVE. Here, we hypothesize that a critical size of a RVE for L. pertusa skeletal structures exists, where for coral colonies larger than such critical size, a homogenized FE approach can be used to investigate the risk of CWC reef collapse. Conversely, for coral colonies smaller than such critical size, the underlying skeletal structure needs to be explicitly modelled. For both approaches, it is important to determine the morphological variations of the underlying skeletal structure as either branch density, arrangements, or morphological features of the branches themselves must be represented.
In this study, we investigate the morphological variations of dead and live L. pertusa skeletal fragments to advance in silico mechanical models of their complex architecture. To achieve this aim, we (i) investigate the critical size of L. pertusa skeletal structure that allow us to use a mechanical homogenization approach to investigate crumbling and collapse of whole reef structures; (ii) analyze the morphology of L. pertusa skeletal fragments from coral colonies that were alive when collected, and dead erect coral framework to explain how corals occupy continuous space; and (iii) characterize the branching morphology of L. pertusa skeletons to describe size and shapes of individual corallites.
2 Materials and methods
2.1 Cold-water coral specimens
We investigated morphological variations of L. pertusa specimens collected by Büscher et al. (2019) from two Norwegian reef sites (Sula Reef Complex at 64°06.32’N, 8°07.1’E and 303 m depth, and Leksa Reef at 63°36.46’N, 9°22.76’E, 157 m depth and 63°36.43’N, 9°22.45’E, 152 m depth). The offshore Sula Reef location consisted of a relatively stable habitat (e.g., constant temperature, pH, and currents), whereas the inshore Leksa Reef location is subjected to strong tidal and currents, leading to a highly variable environment (Büscher et al., 2019; Büscher et al., 2024). Live coral colony fragments as well as dead erect coral framework fragments were sampled from both sites to provide a better representation of the environmental variability L. pertusa corals are found.
To investigate the critical size of a RVE for CWC skeletal structure, we examined two L. pertusa specimens collected from Rockall Bank (57°54.9’N, 13°52.296’W, unknown depth) and West Shetland (60°43.188’N, 2°55.788’W, unknown depth), which provided a larger representation of the skeletal structure (Table 1).
2.2 Image acquisition and processing
Computed tomography (CT) images from Büscher et al. (2019) of dried coral fragments from Norwegian reefs were acquired with a Toshiba Aquilion 64 clinical CT (120 kV, 600 mA, 0.351 mm in-plane pixel size, 0.5 mm slice thickness, 0.3 mm slice spacing). Images were reconstructed with a voxel size of 0.351x0.351x0.3 mm3. For the large specimens, we performed CT with a Siemens Somatom clinical CT (120 kV 80 mA, 0.6 mm slice thickness, 0.35 mm slice spacing). The CT images had an in-plane pixel size of 0.662 mm for the Rockall Bank and 0.445 mm for the West Shetland specimen.
We used Python 3.8 libraries SimpleITK and scikit-image for image post processing. First, we resampled the images to an isotropic voxel size of 0.351 mm3 for the Norwegian specimens, 0.662 mm3 for the Rockall Bank specimen, and 0.445 mm3 for the West of Shetland specimen. We reduced noise using a recursive Gaussian filter with a filter width of . Thereafter, we segmented coral skeletons and cavities individually. We segmented the coral skeletons using a maximum entropy algorithm and we used a connected component analysis to remove isolated regions that were not attached to the skeleton (Figure 2A, D). We then segmented cavities within the skeleton in three steps. First, we applied a 3D morphological closing filter with a kernel radius of 6 pixels followed by a 3D binary dilation with a kernel radius of two pixels to the segmented coral skeleton to create a coarse mask contour image of the combined coral skeleton and cavities. This mask was then refined using an iterative 3D geodesic active contour (3D-GAC) algorithm (Ohs et al., 2021), which allowed us to identify the external contour of the skeleton (Figure 2B, E). We obtained a binary image containing only the cavities by subtracting the binary coral skeleton from the contour mask image. We labelled individual corallite calices using a hierarchical watershed on the 3D distance map (Figure 2C, F).
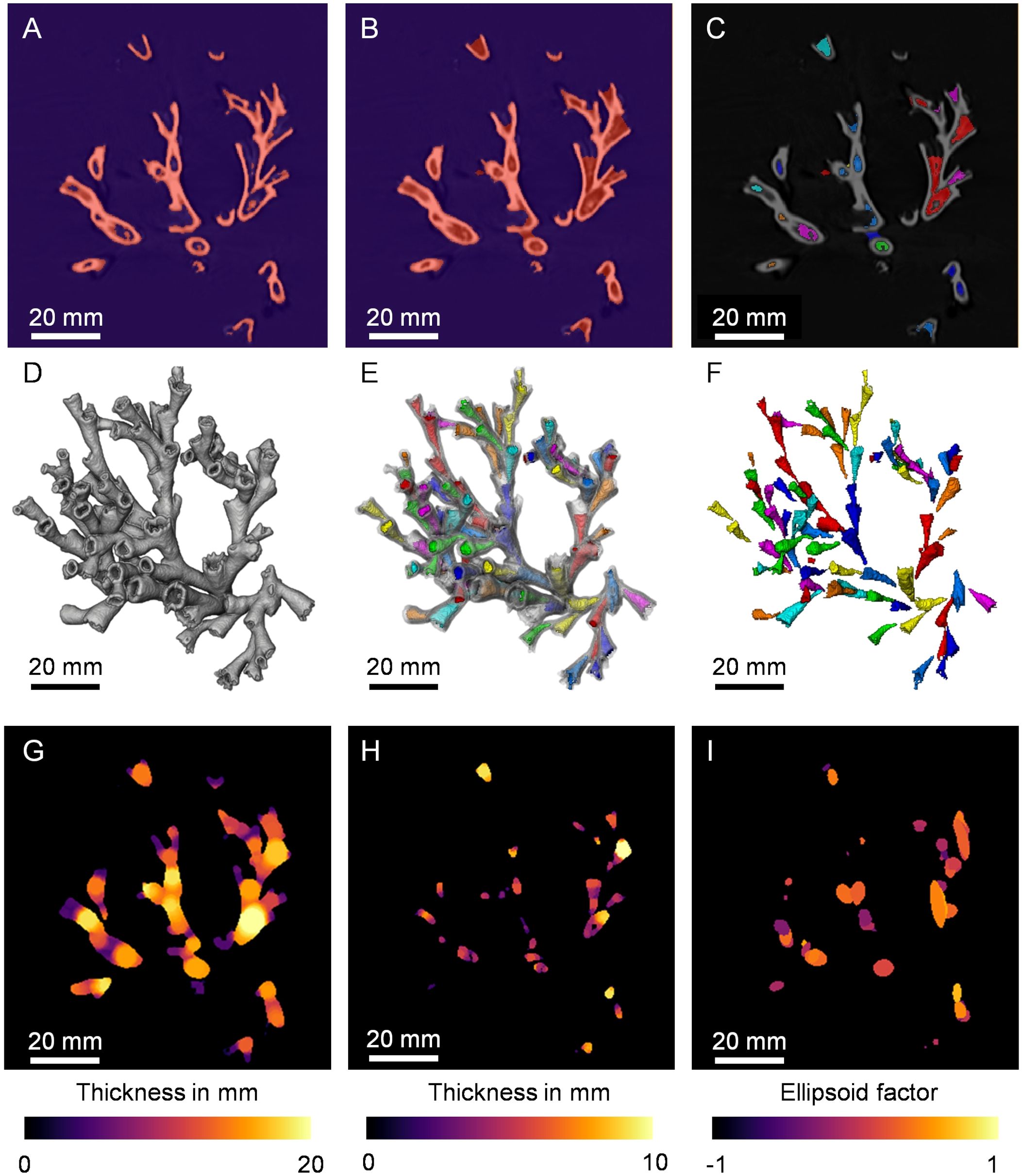
Figure 2. Segmentation of skeleton and cavities for an exemplary CWC specimen of the Leksa Reef. A representative CT cross-section and 3D render of the specimen are shown. (A, D) Coral skeleton was segmented. (B, E) A mask contour image was created by filling the cavities within the skeleton. (C, F) Cavities were segmented subtracting the skeleton from the masked contour and individually labelled. (G, H) The local diameter of the skeleton and branch thickness were computed from the mask contour image and the cavities image. (I) The shape of the coral branches was computed using the ellipsoid factor, where a value of -1 indicates a highly oblate shape and a value of 1 a highly prolate shape.
To quantify the local size of both the coral skeleton and corallites, we computed the 3D thickness maps (Hildebrand et al., 1999) of the contour and cavities mask images, respectively (Figure 2G, H). We assessed the local shape of the coral skeleton by measuring the 3D ellipsoid factor maps (Doube, 2015) on the contour mask image (Figure 2I). Finally, we computed the mean spacing between skeletal branches (i.e., interbranch lengths) from the 3D spacing maps of the contour images. Thickness (Br.Th), spacing (Br.Sp), and ellipsoid factor (Br.Ef) were computed using BoneJ (Doube et al., 2010) plugin in Fiji (Schindelin et al., 2012).
2.3 Critical size of representative volume element of cold-water corals
A RVE can be defined as the smallest volume element of a heterogeneous structure for which a macroscopic constitutive representation is sufficiently accurate to model the mean constitutive response (Drugan and Willis, 1996). Therefore, an appropriate size of a RVE of the skeletal structure should be found that consider: (i) a large enough number of heterogeneities to be statistically representative of their structure; (ii) a size small enough so that it can still be considered as a material point from a macroscopic point of view. The RVE domain shall comply with the Hill condition (Hill, 1963), which states the necessary and sufficient conditions for equivalence between energetically and mechanically defined properties of elastic materials:
This means that the average of the product of the stress and strain tensors (microscale) equals the product of their averages. Similar to trabecular bone (Pahr and Zysset, 2008), we do not strive to find a RVE where (1) is exactly fulfilled but where we can obtain a usable approximation.
Numerical techniques, such as the FE method, can be used to approximate the critical size for a RVE by analyzing the size dependence of the elastic symmetries and properties of the structure (Kanit et al., 2003). These properties can be estimated from the stiffness tensor, S, using a direct mechanics approach through an optimization procedure where the best orthotropic representation of S may be found (van Rietbergen et al., 1995). Here, we approximate the critical size of a RVE for L. pertusa skeletal structures by analyzing the convergence of the orthotropy assumption.
2.3.1 Finite element modelling
We virtually extracted a cuboid volume element with edge lengths of 114.2 mm x 58.9 mm x 114.2 mm in x, y, and z direction, respectively, which corresponded to the largest cuboid fully occupied by the structure, from the CT reconstruction of the Rockall Bank and West of Shetland L. pertusa specimens (Figure 3). From this, we generated 64 cuboids for each specimen with edge lengths varying from 114.5 mm to 34 mm in the x and z direction while keeping the y direction constant. We created FE models by direct conversion of image voxels into isotropic linear hexahedral elements using previously implemented methods (Peña Fernández et al., 2022). Additionally, we generated a second type of model to investigate larger skeleton sizes than the ones physically available for scanning. We mirrored the original cuboid volume element along x, y, and z axis (Figure 3C) which resulted in a 225 mm cubical volume element. From this cuboid, we generated 217 cuboid volume elements and associated FE models with edge lengths varying from 225 mm to 45 mm.
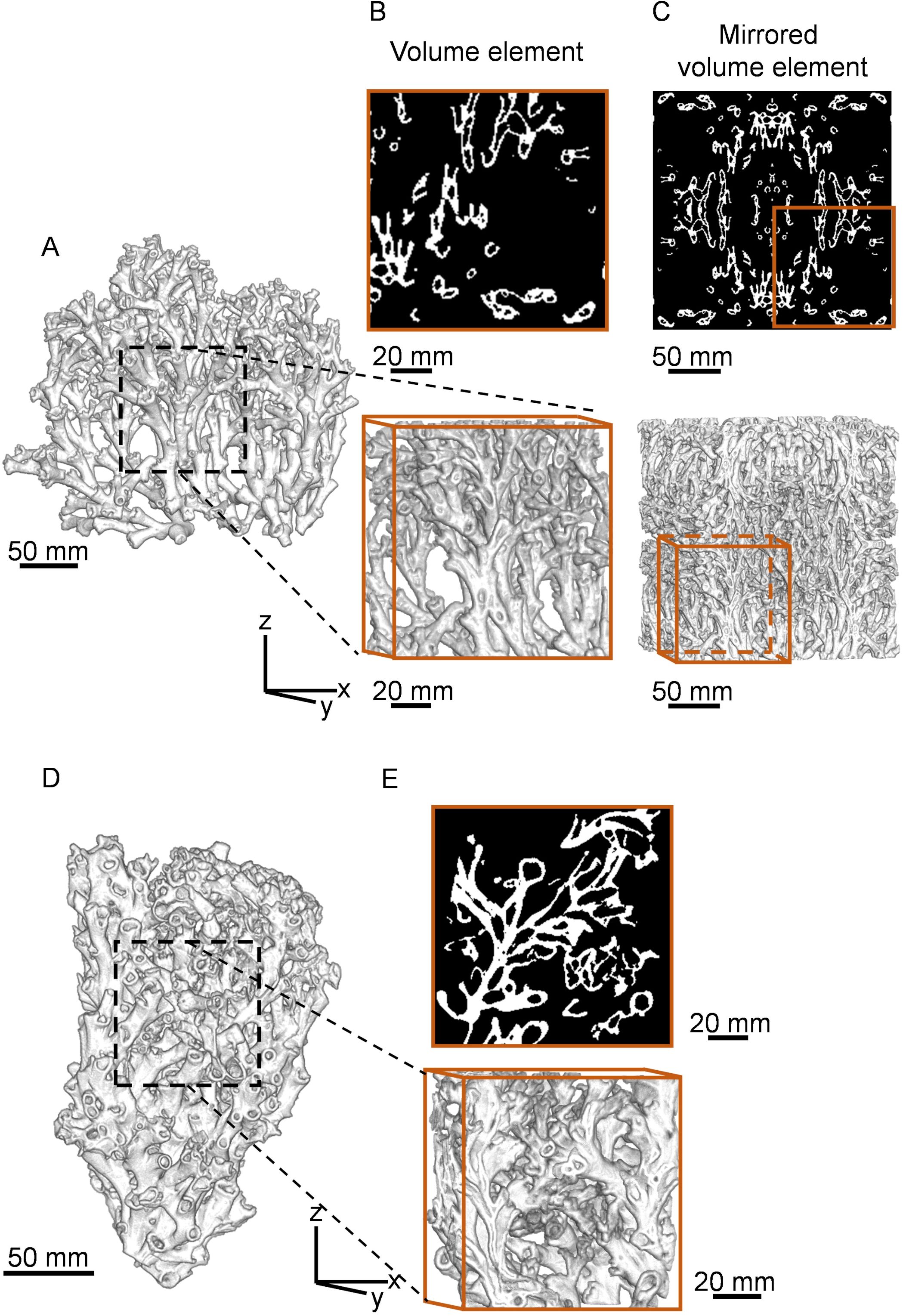
Figure 3. Representative volume element of large cold-water coral specimens from Rockall Bank and West Shetland. 3D renders of (A) Rockall Bank and (D) West Shetland cold-water coral specimens used for finite element analysis. (B), (E) 2D cross-sections (top) and 3D volume elements (bottom) with 114.2 mm edge length in x and z axis and (C) mirrored model with 225 mm edge length.
All models were analyzed using kinematic uniform boundary conditions, where six independent load cases (three uniform longitudinal compressive strains and three uniform shear strains) were applied (Pahr and Zysset, 2008). The tissue material is assumed to be isotropic with a Young’s modulus of and Poisson’s ratio of for all models (Wolfram et al., 2022). The apparent stiffness tensor, S, of each model was derived from the FE analysis via the apparent stresses and strains as in (Pahr and Zysset, 2008).
2.3.2 Determination of orthotropic assumption
We calculated the orientation of the closest orthotropic stiffness tensor by minimizing the objective function defined by:
Where represents the nonorthotropic terms of the stiffness tensor and the orthotropic terms of the transformed stiffness tensor (van Rietbergen et al., 1995). The orientation of the transformed stiffness tensor was obtained through a series of rotations, as defined by the Euler angles, about the coordinate axes x, y, and z. The optimization approach then yielded to the best possible orthotropic representation of the stiffness tensor, . We defined an orthotropic approximation of by setting the nonorthotropic components to zero. The accuracy of the orthotropic assumption was quantified using the error of the orthotropic approximation (Pahr and Zysset, 2008), defined as:
We analyzed the convergence of the error with respect to the size of the volume elements expressed as edge length of the volume element and number of interbranch lengths, i.e., edge length over the mean spacing between skeletal branches, Br.Sp. Both and were checked to confirm they were positive definite.
2.4 Morphology of L. pertusa colony fragments
We introduce six shape variables to quantify the morphology of the L. pertusa skeletal fragments at the colony level. We calculated these based on the segmented coral skeleton images (Figures 2A, D) in Python 3.8.5. We quantified shape through the sphericity and sparsity (capturing volume compactness) and the surface area to volume ratio and fractal dimension (capturing surface complexity) as:
i. Sphericity () is an invariant measurement of the compactness of an object’s volume. is defined as the ratio between the surface area of a sphere with the same skeletal volume () as the coral colony and the surface area of the coral skeleton (SA).
ii. Sparsity (S) is an invariant measurement of the degree to which there is space between different regions of the coral structure. S is defined as the ratio between the volume of an ellipsoid () fitting the coral colony and the skeletal volume () of the coral.
iii. Surface area to volume ratio () refers to the amount of surface area (SA) per unit volume of the skeletal volume () of the coral colony.
iv. Fractal dimension () captures how the surface of the coral skeletal structure fills space, and it is an estimate of the spatial complexity. is computed as the slope of the number of boxes at a size s that contains part of the coral skeletal structure () and the size of the boxes (s).
2.5 Morphology of L. pertusa skeletal branches
To quantify morphological variations of the coral specimens at the branch level (i.e., size and shape of the individual and/or group of corallites), we first performed a skeletonization (Kruszyński et al., 2007) of the mask image contour (Figures 3B, E) via a 3D thinning algorithm and we converted the skeletonized image into a graph object (Supplementary Figure S1) using the NetworkX package (Hagberg et al., 2008).
Initially, we assigned a 3D spatial coordinate to each node based on the image coordinates and we then inspected the resulting graphs and manually selected the root (i.e., base) of each skeleton based on the morphology, from where a newly oriented graph was created via a depth-first-search algorithm (Cormen et al., 2001). We added the mean coral branch thickness (Br.Th), length (Br.Len), area (Br.Ar), volume (Br.Vol), taper rate (Br.Tr), and ellipsoid factor (Br.Ef) as nodal attributes to account for the size and shape of each individual branch.
We then introduced four topological descriptors that represent morphological features of the branching coral structure (Khalil et al., 2022). These descriptors associate a function to a given coral skeleton whose independent variable is either the path, , or radial, r, distance from the skeletal root (Supplementary Figure S1D).
i. Branching pattern () quantifies the skeletal complexity of the coral specimens and the distribution of the branches. is related to skeletal growth and spatial arrangement and it can be defined as a function of the radial distance from the root, r, as:
Where represents the cardinality of each set and the radial distance of i-th node, , to the root.
ii. Terminal branch index () counts the number of end points that can be reached from a given node. quantifies the hierarchical branching growth of CWC skeletons and it is defined as a function of the path distance, from the root along the branches as:
Where represents the path distance from the i-th node to the root.
iii. Tortuosity () is defined as the ratio of the path distance, , by the Euclidean distance, , between a node and the root. is related to the branch growth mechanism, and it is defined as a function of the radial distance from the root, r, as:
Where represents the Euclidean distance from the i-th node to the root.
iv. Volume distribution () gives a measure of how the volumetric mass of the branches are distributed relative to the root. For a given coral skeleton, we consider all its nodes as cloud points in 3D space, , each of them carrying a weight equal to the volume of the branch, . This volume affects the space around them such that each node contributes to a field, , which is normalized to have length and which is of the form:
By superimposition, the node configuration of the skeleton gives rise to a vector field,V, whose magnitude is used as , and it is defined as a function of the radial distance to the root, as:
To reduce the dimensionality of the proposed descriptors as well as the coral branch attributes (i.e., Br.Th, Br.Len, Br.Ar, Br.Vol, Br.Tr and Br.Ef) we combined those measurements into a vector, , by considering the area under the curve defined by each descriptor, as:
Where x is the normalized path, , or radial, , distance from the skeletal root. The corresponding vector for each coral skeleton, , is:
This vectorization allows us to optimize classification of the data (Khalil et al., 2022).
2.6 Analysis of morphological parameters
We compared morphological differences between dead and live skeletal fragments as well as Sula and Leksa locations at both colony and branch level. For each specimen a distribution of branch morphological parameter was obtained and statistical information calculated. Thereafter, statistical analysis was based on the median values of such parameters.
Statistical analyses of morphological parameters coral skeletons were conducted in RStudio (Version 1.1.456). We used quantile–quantile plots and Shapiro–Wilk post-hoc tests to test normal distribution of data. If normality was given, we compared groups using Student’s t-tests. Where data were non-normal, we used Wilcoxon rank sum tests. We assumed a significance level of p = 0.05.
We used principal component analysis (PCA) to visualize patterns of morphological variations at the colony and branch level. We standardized variables with a mean of zero and unit variance to reduce the influence of variable scale on the projection. We used score plots to visualize the projection of each coral specimen onto the span of the two first principal components and how each group relates to each other. We used confidence ellipsoids around the main points of dead/live classes to illustrate data points lying within the multivariate distributions. A 95% confidence level defined the size of the ellipsoids, whereas the shape was determined by the covariance matrix. Finally, we investigated the relationships between the first two principal components and the original morphological variables using loading plots.
3 Results
3.1 Critical size of representative volume element L. pertusa
The error of the orthotropic approximation of the stiffness tensor decreases with increasing specimen size (Figure 4A). The error decreased significantly after 6 cm edge length and converged to less than 3% at ~9 cm edge length. Considering that the mean Br.Sp was 2.57 cm for the Rockall Bank coral specimen and 1.75 cm for the West Shetland specimen, the error converged at four to five Br.Sp (Figure 4B). The size of the volume element influenced the Young’s and shear modulus but had minimal influence in the Poisson’s ratio (Supplementary Figure S2). Overall, the Rockall Bank specimen displayed lower elastic and shear modulus compared to the West Shetland specimen as a result of its lower skeletal volume fraction, (Supplementary Figure S3). The underlaying structure had little influence on the error of the orthotropic approximation, with observed for ranging between 5% to 20%, and a wide range of branching and terminal nodes (Supplementary Figure S3). Similar convergence was observed for the mirrored models ( at ~9 cm edge length, ~4 Br.Sp). The imposed orthotropic structure in those models resulted in and lower standard deviation for extracted volume elements >13 cm. Therefore, a critical size of ~13 cm (five to seven Br.Sp), should provide sufficiently averaged continuum quantities, thus, allowing for a mechanical homogenization approach for L. pertusa skeletal structures.
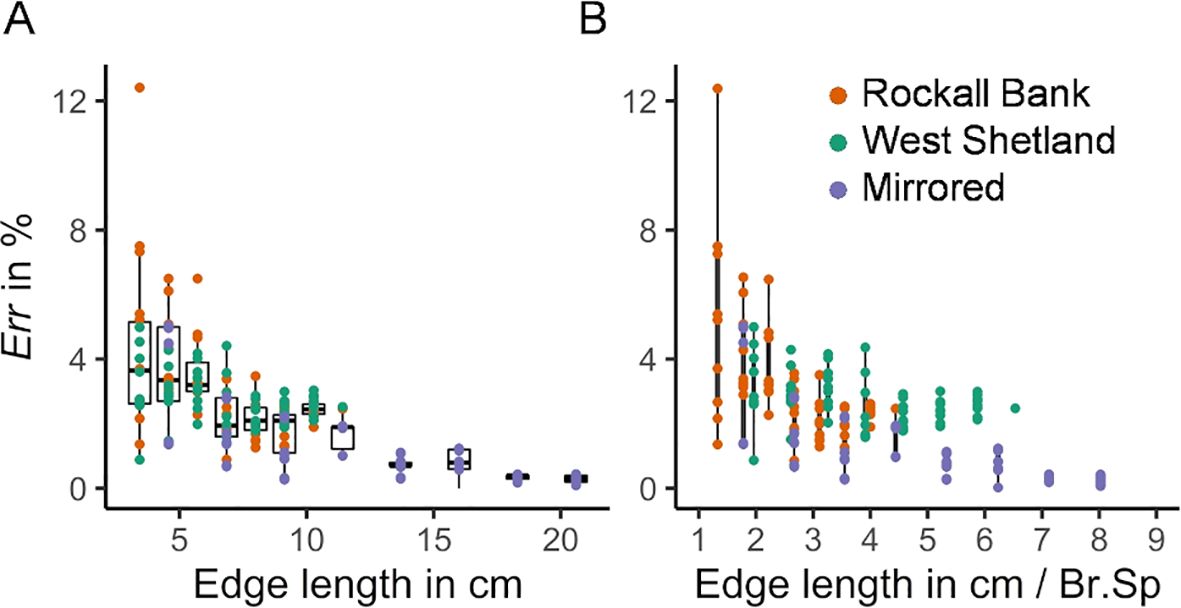
Figure 4. Error of orthotropic approximation. Boxplots of the errors for the analyzed volume elements of the Rockall Bank, West Shetland and mirrored models as a function of the (A) edge length of the cuboid volume elements and (B) number of interbranch spacings, i.e., edge length over mean branch spacing (Br.Sp).
3.2 Morphology of L. pertusa colony fragments
Live coral fragments exhibited significantly greater SA: Vol (Figure 5A), while dead fragments displayed a less compact structure (i.e., lower sphericity and sparsity) (Figures 5C, D). Both sphericity and sparsity were significantly larger for live Sula fragments (Figures 5C, D). The complexity of the dead coral framework was demonstrated through the higher fractal dimension (Figure 5B). Live coral specimens showed larger variability of the shape parameters and greater differences between specimens from Leksa and Sula reef.
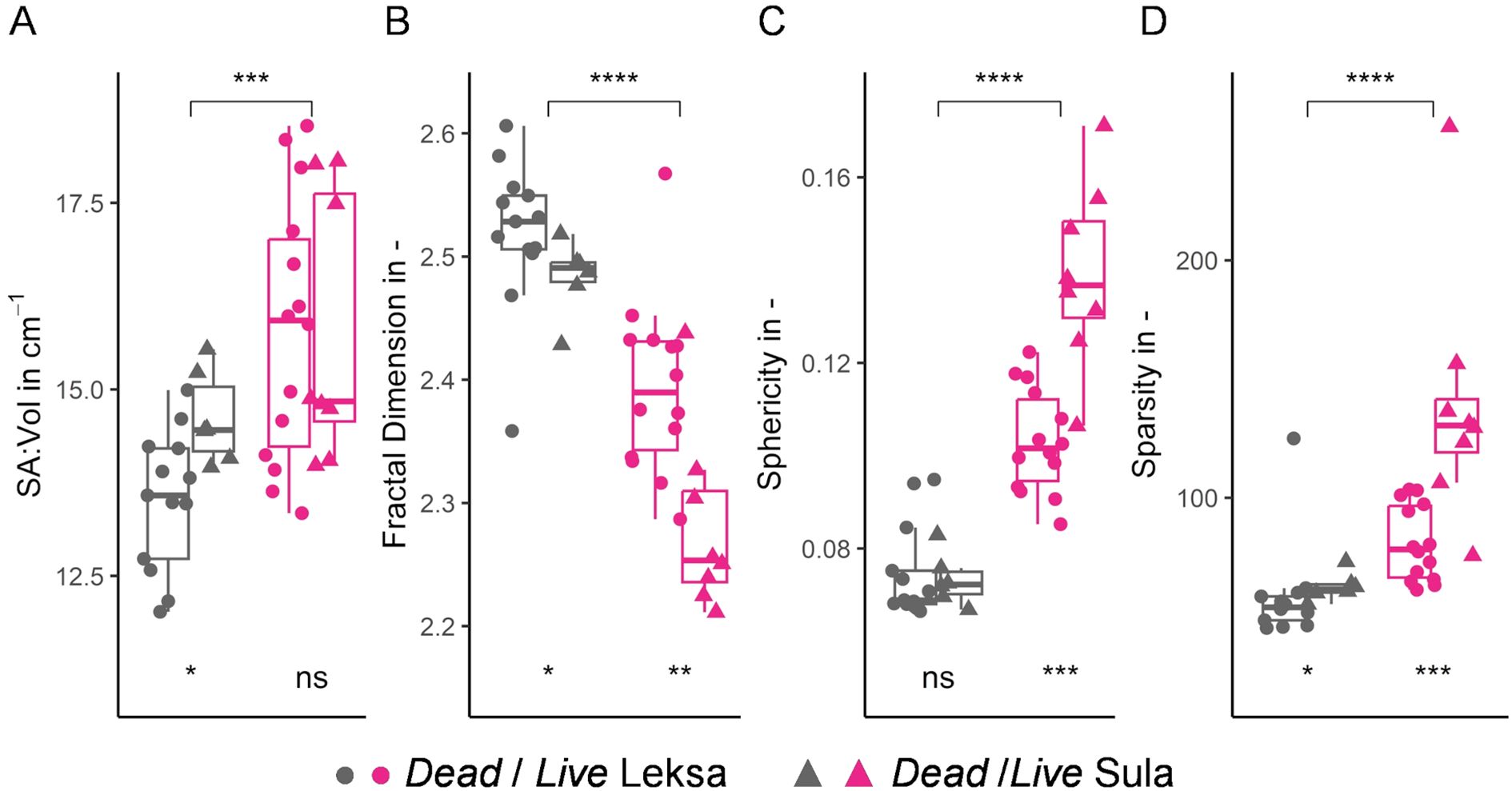
Figure 5. Morphological parameters of L. pertusa coral colonies. Boxplots of (A) surface area to volume ratio (SA: Vol), (B) fractal dimension, (C) sphericity and (D) sparsity for dead and live coral fragments are shown in grey and pink colors, respectively. ‘●’ and ‘▲’ symbols correspond to specimens from Leksa and Sula reef, respectively. Significance levels between dead and live fragments are indicated at the top of each boxplot and those between Leksa and Sula at the bottom. ns: non-significant, *p < 0.05, **p < 0.01, ***p < 0.001, ****p < 0.0001.
3.3 Morphology of L. pertusa skeletal branches
Live corals contained significantly larger branches compared to the dead coral fragments (Figure 6B), yet median branch areas and volume were not significantly different (Figures 6A, D). Dead coral framework branches were significantly thicker than those from live specimens (Figure 6E). The larger values of the ellipsoid factor and taper rate for live corals indicate a more prolate shape and wider opening of their branches (Figures 6C, F). Median branch length and thickness of live corals from Leksa reef were significantly lower than those from Sula reef (Figures 6B, E). Overall, skeletal branch morphology was highly variable within each specimen (Figure 7; Supplementary Tables S1-S3), as demonstrated when pooling all analyzed branches (Supplementary Figure S4).
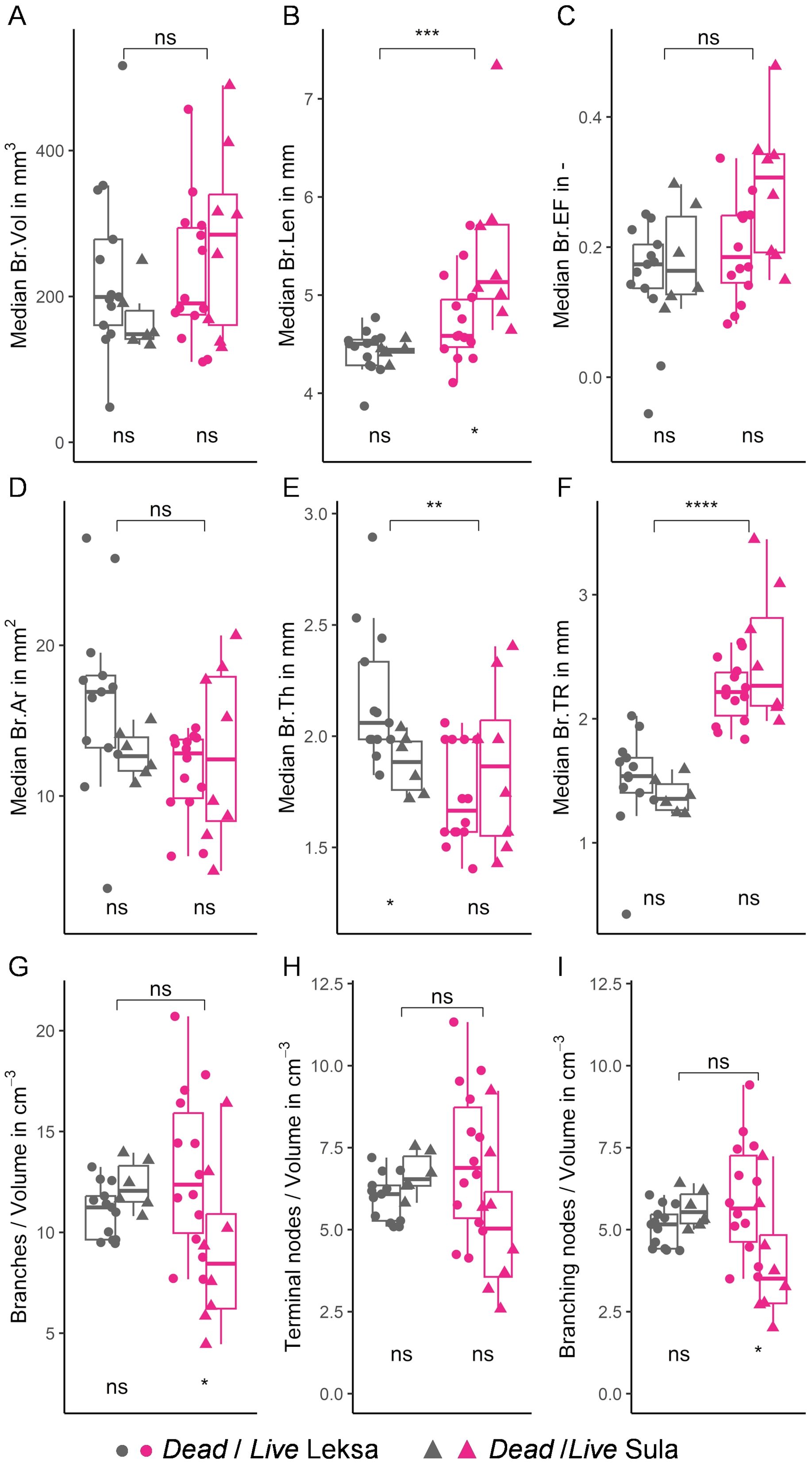
Figure 6. Morphological parameters of L. pertusa coral branches. Boxplots of (A) median branch volume (Br.Vol), (B) area (Br.Ar), (C) length (Br.Len), (D) thickness (Br.Th), (E) ellipsoid factor (Br.Ef), (F) taper rate (Br.Tr), (G) number of branches per unit volume, (H) terminal nodes per unit volume, and (I) branching nodes per unit volume for dead and live coral fragments are shown in grey and pink colors, respectively. ‘●’ and ‘▲’ symbols correspond to specimensfrom Leksa and Sula reef, respectively. Significance levels between dead and live fragments are indicated at the top of each boxplot and those between Leksa and Sula at the bottom. ns: non-significant, *p < 0.05, **p < 0.01, ***p < 0.001, ****p < 0.0001.
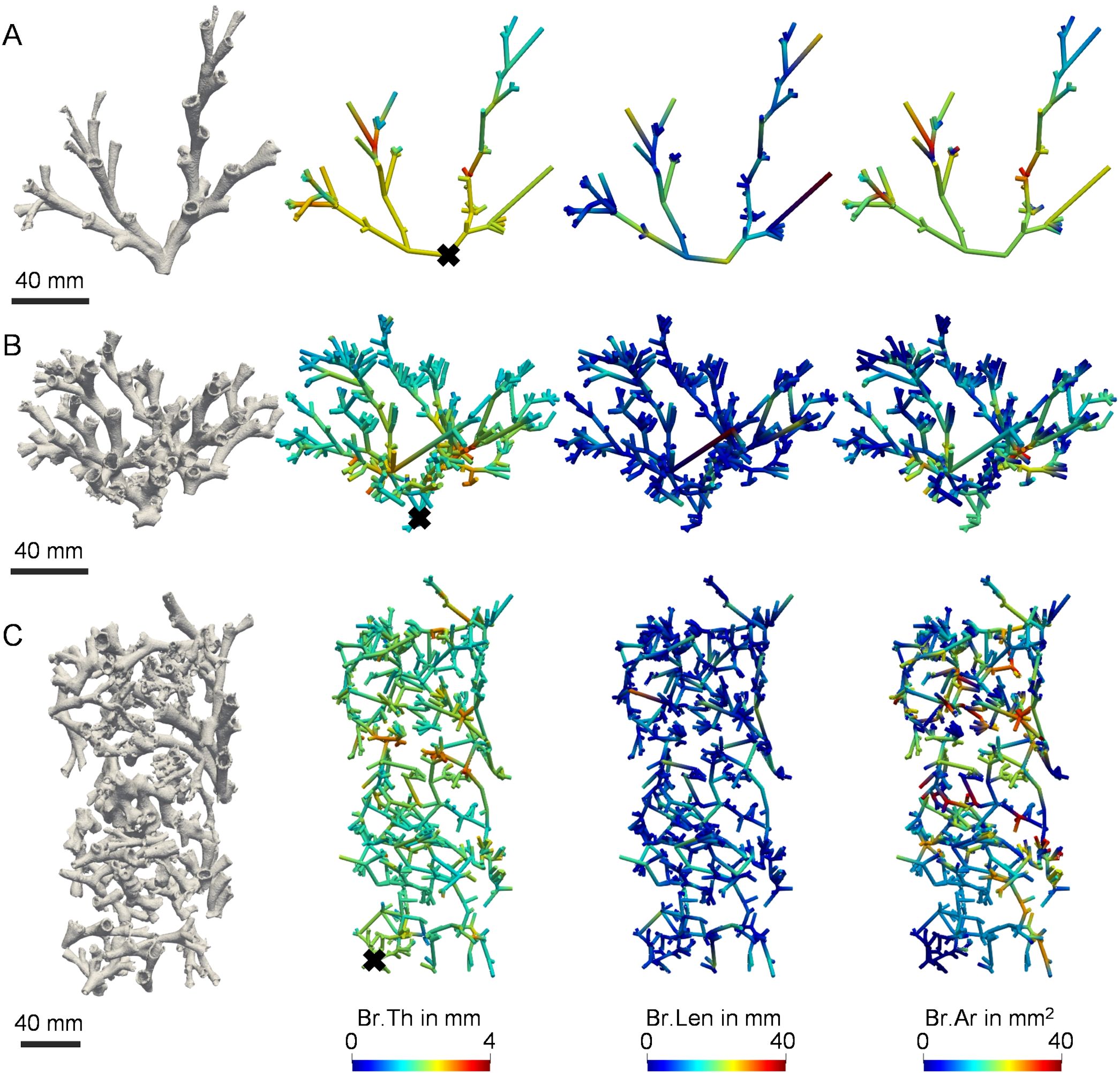
Figure 7. Local morphometry of L. pertusa skeletal branches. 3D graphical representation of branch thickness (Br.Th), length (Br.Len), and area (Br.Ar) of the individual branches for three representative L. pertusa specimens with increasing levels of complexity; (A) Live coral fragment from Leksa reef, (B) Live coral fragment from Sula reef, and (C) dead coral fragment from Sula reef. Please note the fusion of multiple coral origins in the dead coral fragment (C). ‘×’ symbols correspond to the defined skeletal root (base).
Skeletonization of the coral structure demonstrated no significant differences in the number of branches and nodes per unit of skeletal volume between live and dead skeletal fragments (Figures 6G-I). Live fragments from Leksa reef had significantly more branches and branching nodes per unit of skeletal volume compared to those specimens from Sula Reef, however, the number of terminal nodes per unit of skeletal volume was not significantly different.
3.4 Topological descriptors
decreased with increasing radial distance (Figure 8A). Such a decrease was faster for the dead fragments. This illustrates that for the live specimens, newer polyps appear further from the initial base. Similarly, the distribution of (Figure 8B) showed a faster decrease for live coral fragments, that is, the path to reach the end points of the structure is more direct than for the dead fragments. The higher complexity of the skeletal structure of dead fragments results in increased (Figure 8C), as well as a larger (Figure 8D), which decreased for the more distant branches, as a consequence of the dense packing of the structure (Figure 5).
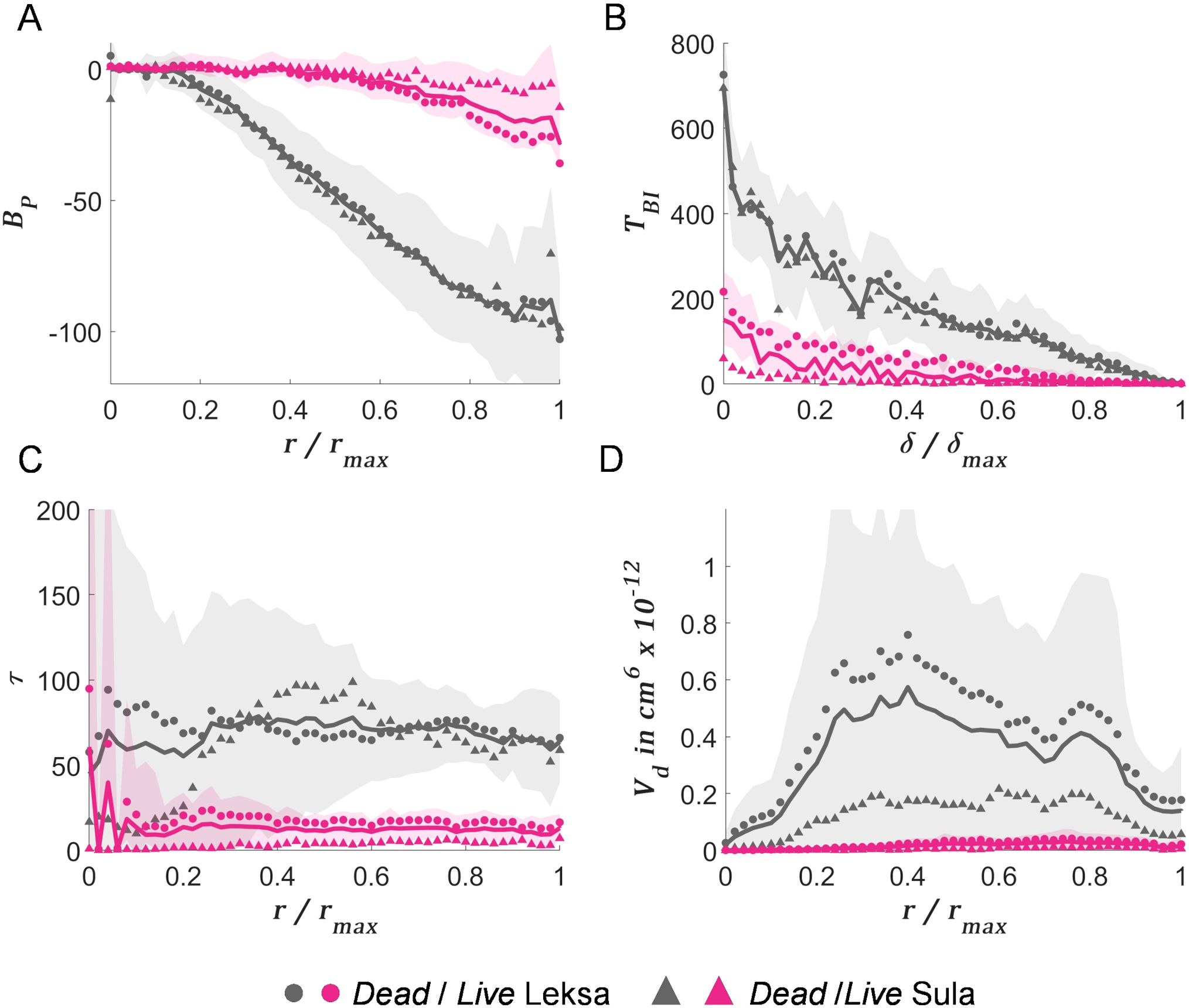
Figure 8. Topological descriptors for L. pertusa fragments. (A) Branching pattern (), (B) terminal branch index (), (C) tortuosity (), and (D) volume distribution () as a function of the radial, , or path distance, , from the skeletal root. Solid lines indicate the mean values, while shaded areas show the mean ± standard deviation for dead and live coral fragments in grey and pink colors, respectively. ‘●’ and ‘▲’ symbols correspond to mean values for specimens from Leksa and Sula reef, respectively.
3.5 Principal component analysis
At the colony fragment length scale, the first principal component (PC1) of the PCA explained 47.3% of variation (Figures 9A, B). The projection of live coral fragments from Leksa reef in the score plots was largely aligned in the direction of PC1 (Figure 9A), which was primarily contributed by the branching descriptors (i.e., number of branches, branching nodes, and terminal nodes per unit of volume) (Figure 9B). The second principal component (PC2) explained 34.5% of variation, with a major contribution of the fractal dimension and sparsity. A clear positive correlation between the branching parameters, as well as a negative correlation between fractal dimension and sparsity, sphericity and SA: Vol. SA: Vol had the lowest contribution on both PCs. At the skeletal branch level, PC1 explained 76.1% of the variation, whereas the PC2 explained only 11.1% of the variation (Figures 9C, D). and Br.Tr were positively correlated and largely aligned with PC1, while showing a negative correlation with Br.Th, Br.Len, Br.Ar, and . The confidence ellipsoids demonstrate that dead specimens showed the lowest variance of the morphometric parameters at the colony fragment level (Figure 9A), but highest variance at the skeletal branch level (Figure 9C).
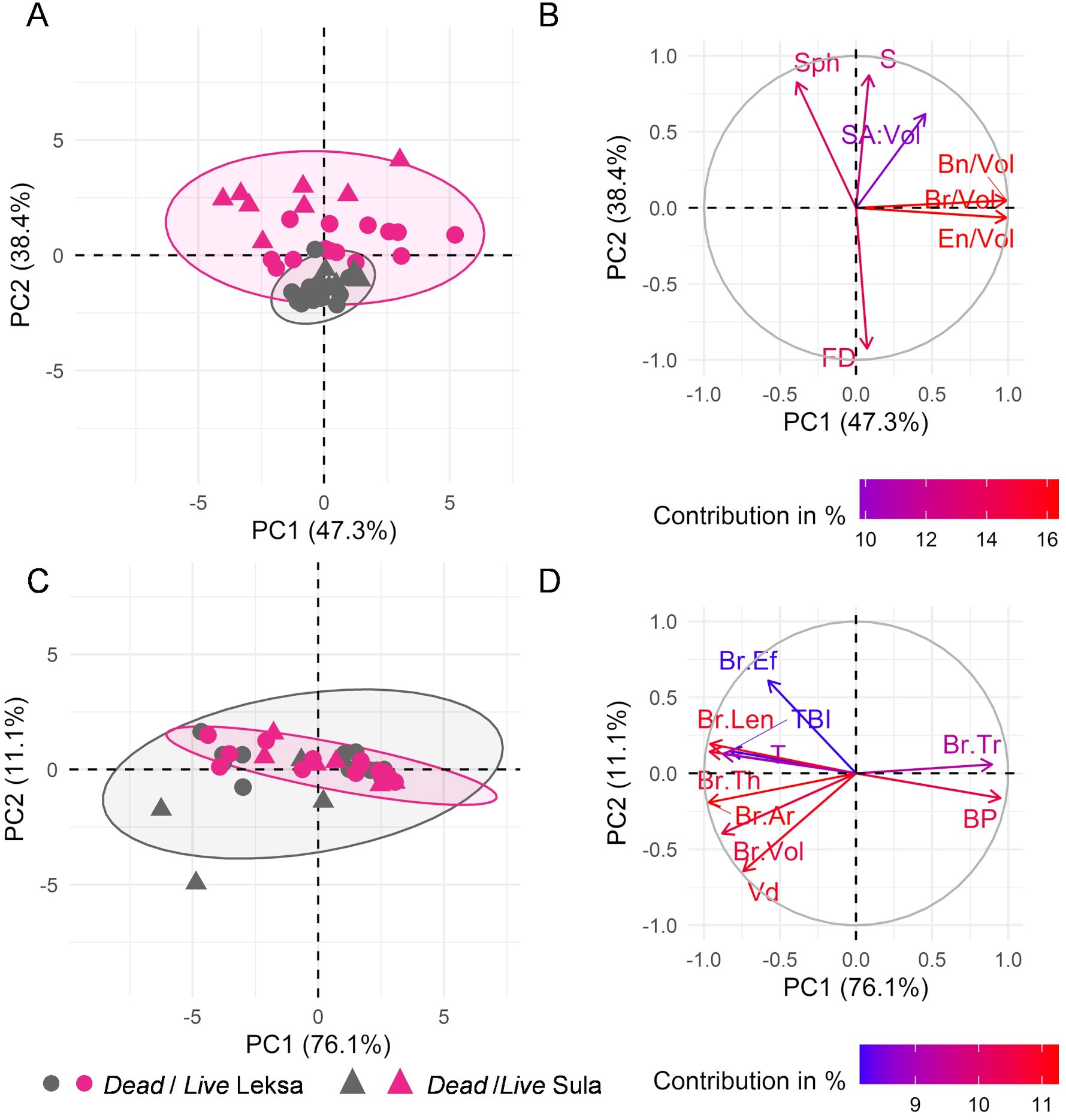
Figure 9. Principal component analysis. (A, C) Score and (B, D) loading plots resulting from PCA for the morphological analysis at (A, B) colony and (C, D) skeletal branch level. Points in (A, C) represent the projection of the analyzed dead and live coral fragments in grey and pink color, respectively. ‘●’ and ‘▲’ symbols in (A, C) correspond to specimens from Leksa and Sula reef, respectively. Confidence ellipsoids centered at the mean value of dead/live labels are also shown. Arrows in (B, D) indicate variable loadings. , sphericity; , sparsity; , surface area to volume ratio; , fractal dimension; , number of branches; , branching nodes; , ending nodes; , branching pattern; , terminal branch index; , volume distribution; , tortuosity; , branch volume; , branch area; , branch length; , branch thickness; , branch ellipsoid factor; : branch taper rate.
4 Discussion
4.1 Critical size for Lophelia pertusa skeletons
We performed a preliminary investigation on the critical size of L. pertusa skeletal structure that allows us to use a mechanical homogenization approach to study the mechanical vulnerability of CWCs to ocean acidification. We built upon concepts developed for porous structures such as trabecular bone, where similar challenges are present (e.g., trabecular thickness and connectivity depending on age/disease and their influence on bone fracture). We showed that the orthotropic approximation of the stiffness tensor for the coral skeletal structure converges at ~13 cm edge length, which reflects the RVE size for the estimation of macroscopic properties of L. pertusa skeletons at the structural level. This critical size corresponds to five to seven times the mean spacing between skeletal branches (i.e., interbranch length) and over 20 times the mean thickness of the branches. Thus, averaging the mechanical properties over less than five interbranch lengths does not provide sufficient continuum quantities, and the homogenization approach must be replaced by statistical models of the underlaying structure. Similar results are seen in other material architectures, such as trabecular bone, in which average mechanical properties are sufficient when considering volume elements over five intertrabecular lengths (Harrigan et al., 1988) or carbon reinforce polymers, where RVEs larger than 15-16 times the heterogeneity size (i.e., fiber thickness) fulfil the Hill criteria (Khisaeva and Ostoja-Starzewski, 2006; Trias et al., 2006). Here, we limited our investigation to two specimens from different locations due to the unavailability of 3D data on large coral colonies. While this low number of specimens is likely insufficient to provide a representation of the elastic properties of L. pertusa skeletal structure across many locations, it illustrates the variability which can be found in skeletal structure from specimens from relatively close sites. Indeed, we observe that the lower skeletal density of the Rockall Bank specimen led to lower elastic and shear modulus compared to the West Shetland specimen, yet the error of the orthotropic assumption was not dependent on such skeletal density or the branch distribution (Supplementary Figure S3). While larger number of specimens will be needed to derive the relationships between the effective elastic properties at the macroscale and underlying structure, our convergence study demonstrates that the determined critical sizes are robust and applicable to other coral skeletal fragments. In addition to this, we demonstrate the applicability of the methodology so that our results can be easily confirmed as larger samples become accessible.
4.2 Morphological variability of L. pertusa skeletal structure
The structural complexity of L. pertusa reefs makes it difficult to standardize the sampling, which resulted in a large range of specimen sizes and aspect ratios (Table 1; Supplementary Figure S5), with dead coral fragments bigger than live ones. For this reason, a comparison of size morphometric variables of the colonies, such as volume or area, is not legitimate. Therefore, we used four shape variables to describe how L. pertusa colonies occupy the space. Variations in volume compactness capture a gradient from dead to live coral specimens (Figure 9). This is indicative of the open branching structure of live colonies, which is optimized for food particle capture, opposite to the dense structure of the dead framework resulting from the packing and fusion of several branches as they thicken when aging. Moreover, the ability for building reef frameworks increases for colonies with high compactness (Rasser and Riegl, 2002). However, compactness constrained surface complexity, as previously shown by Zawada et al. (2019b). Thus, live colonies with higher levels of compactness tended to be smooth (i.e., higher SA: Vol and lower fractal dimension). The increased SA: Vol of live colonies also implies increased exposure to the environment. Indeed, variation in surface complexity relates to competition and resource use (Zawada et al., 2019a), where colonies with higher structural complexity have less access to those resources (e.g., nutrients), but can have more polyps packed within a given space (Wangpraseurt et al., 2012). From a mechanical perspective, the highly packed structure of the dead framework serves to support living colonies by sustaining external loads.
When comparing these shape variables between specimens from Leksa and Sula Reef we observed higher surface complexity (i.e., fractal dimension) in dead and live fragments from Leksa Reef, which agrees with data from Sanna et al. (2023) in dead skeletal framework of L. pertusa. However, in contrast to Sanna et al. (2023), we obtained higher compactness values (i.e., sphericity and sparsity) in colony fragments from Sula Reef. These differences were especially significant for live coral fragments (Figure 6), and may be driven by current flows (Sanna et al., 2023). This would also explain the higher diversity pattern observed by Mortensen and Fossâ (2006) in mid-Norwegian inshore reefs (including Leksa) compared to reefs at Sula, as increased surface complexity and reduced compactness creates niches for other associated organisms (Zawada et al., 2019b).
We quantified the morphology of L. pertusa skeletal branches based on a skeletonization of 3D CT images, as first proposed by Kruszyński et al. (2007) for the tropical scleractinian coral Madracis mirabilis. While the authors focused on branch diameters and spacings, which are controlled by a combination of hydrodynamics and genetics (Sebens et al., 1997), we extended the analysis to other parameters that may influence the load-bearing capacity of L. pertusa reefs, such as wall thickness, branch length, cross-sectional area, and volume. Some of these parameters (e.g., thickness and length) have only been quantified using linear measurements from small coral fragments (Gass and Roberts, 2011; Sanna and Freiwald, 2021), consequently, restricting the analysis to a small number of corallites. However, L. pertusa colonies are made up of hundreds to thousands of corallites. Thus, the method we present here provides an efficient tool to assess intraspecific morphological variations in coral skeletal branches. In line with Gass and Roberts (2011) and Sanna and Freiwald (2021), we showed a large variation in size and shape for the analyzed branches (Supplementary Figure S4). These local architectural variations both within the same coral fragment and in between fragments may reflect an adaptive response of L. pertusa to environmental stressors, where the size and shape of branches adapt to minimize mechanical stresses on the coral skeletons while optimizing food capture. Live fragments from the Sula Reef Complex displayed significantly shorter branches, yet more branches per unit of volume. This confirms findings from Büscher et al. (2019) who hypothesized such difference may reflect the more stable environment in this offshore location, which allows L. pertusa skeletal branches to grow longer without risk of fracture. An aspect that was unresolved here was the growth time before death of the dead skeletal material, and hence time for the older polyps to radially thicken while alive. Interestingly, dead skeletal fragments from Leksa reef displayed significantly higher median thickness compared to fragments from Sula reef, which may be due to higher bioerosion rates found in the Sula reef (Büscher et al., 2019).
An additional unknown variable that remains a challenge for live coral research is the ability to store and transfer nutrients within and between individual coral polyps (Georgoulas et al., 2023). We assume that energetic reserves are maintained within each coral polyp unit – while this is assured if connective tissue is lost between polyps, where connective tissue is retained, are energetic reserves partly mobile? To compound this challenging point further, as coral branches come into contact, there is fusion of tissue and skeletal material (Figure 8C) – complete with regards to individual and closely genetically related (sibling) colonies (Hennige et al., 2014). From a mechanical perspective, the fusion of skeletal branches likely contributes to the stiffness of the coral structure. Indeed, other studies have shown that increased connectivity of network-like structures such as fibrous networks or trabecular bone led to higher elastic modulus (Davoodi Kermani et al., 2021; Maquer et al., 2015). While our methodological approach does not allow us to determine the occurrence of such fusions, we evaluated the density of branching nodes as a surrogate of connectivity (Figure 6I). Those branching nodes account not only for the fused branches but also the “split” of branches as they grow.
The measured mean taper rate (i.e., difference in diameter between top and bottom of the corallite) and areas aligns well with previous studies (Farber et al., 2016; Gass and Roberts, 2011; Sanna and Freiwald, 2021), highlighting that our CT image-based approach is able to capture slight variations of branch morphology. However, we reported thicker wall values, which may be explained by differences in the employed methodology, where we consider the mean thickness of the entire branch based on the CT data as opposite to the linear measurement of the thinner wall at the top of the corallites in Gass and Roberts, 2011 and Sanna and Freiwald, 2021. Accurate measurements of the wall thickness in CWC skeletons is important as ocean acidification induces dissolution of the skeletal wall material, decreasing the load bearing capacity of the entire structure (Hennige et al., 2020, 2015; Wolfram et al., 2022).
In addition to the skeletal branch size and shape, we analyzed four topological descriptors that describe the branching characteristics of L. pertusa structures and their distribution in the 3D space. These descriptors confirmed the higher complexity of the dead framework, as seen in the increased tortuosity and volume distribution, which may be a consequence of dense packing of several colony fragments. Although the increased volume distributions (i.e., higher mass density) may represent an advantage of the dead framework to support living colonies under mechanical stressors, the bare dead coral skeleton, which lacks protection by organic tissue or defense mechanisms (Beuck et al., 2010), is more vulnerable to dissolution (Hennige et al., 2020) and bioerosion (Büscher et al., 2019; Wisshak et al., 2012), and consequently, mechanical damage (Vad et al., 2017). Therefore, degradation of skeletal branches in the dead framework may compromise the stability of the entire colony, leading to a rapid collapse and, consequently, loss of biodiversity support (Hennige et al., 2020). The environmental conditions of the analyzed location are reflected in these descriptors, where lower complexity (i.e., branching pattern, tortuosity) is seen for live fragments from the Sula Reef complex (relatively stable currents) compared to fragments from Leksa. Similarly, the higher volume distribution of dead fragments in Leksa reflect the higher compactness of the inshore corals to withstand stronger currents.
Our principal component analysis did not point to clear branching pattern-specific differences in branch morphology between dead and live skeletal fragments (Figure 9). This suggests that we cannot simply skip morphological features when developing in silico mechanical models. Nevertheless, reduced morphological variation was observed for live coral specimens compared to dead framework at the skeletal branch level.
4.3 Towards reef-scale modelling of cold-water corals
The investigation of timescales for reef crumbling relies on the development of computational tools that are able to provide accurate and efficient predictions of the mechanical behavior based on the time L. pertusa coral reefs are exposed to acidified waters (Hennige et al., 2020; Wolfram et al., 2022). These tools are currently non-existent, partially due to the lack of information on the structure-function relationships of corals at the structural level (Figure 1). Here, we showed that homogenized FE models of coral colonies may be used to estimate the risk of crumbling of coral reefs when these surpass the identified critical size of five interbranch lengths. Similarly to previous studies in other multiscale structures such as bone (Cowin, 1986, 1985; Pahr and Zysset, 2009), the stiffness and strength of the individual finite elements can account for the skeletal volume fraction and architectural information (Figure 10A). This approach outperforms current models that considered a uniform homogeneous coral skeletal structure (Madin and Connolly, 2006; Storlazzi et al., 2005), thus, missing the influence of L. pertusa branching structure and composition (i.e., live or dead skeleton) on the mechanical response. However, CWC reefs comprise hundreds to thousands of colonies of varying sizes and shapes which are mostly related to variations in the substrate and local hydrodynamics (De Clippele et al., 2018), meaning they are subjected to diverse loading conditions. As the size of some of those colonies may be smaller than the critical size we determined (e.g. on Tisler Reef (Norway) almost 60% of colonies have sizes smaller than 30 cm (De Clippele et al., 2018)), homogenized FE models would be unsuitable and must be replaced by explicit models of the branching structure of L. pertusa skeletons.
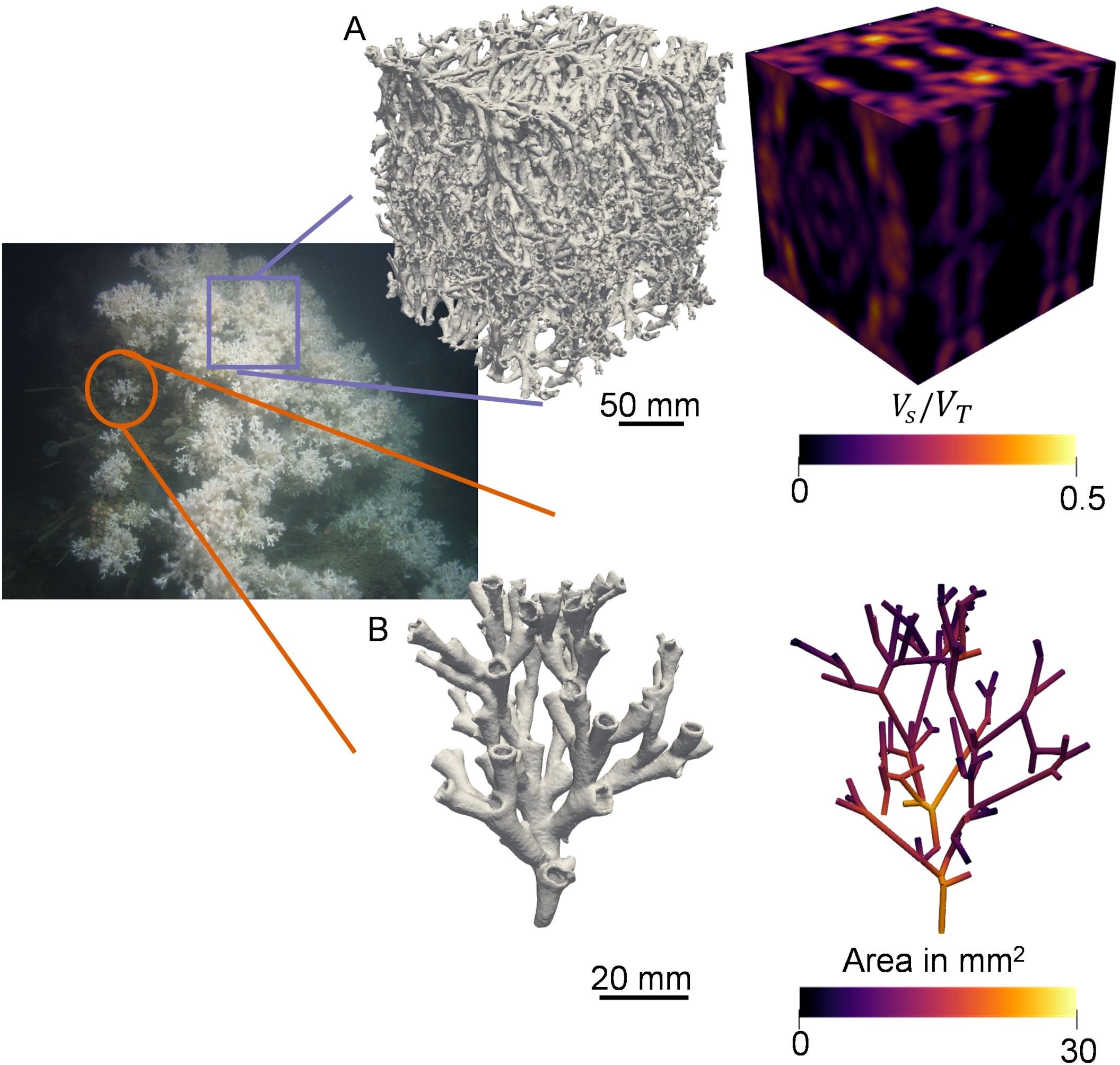
Figure 10. Realms of in silico models for L. pertusa skeletal structure. (A) Large coral colonies (> 13 cm, 5 interbranch lengths or 800 branches) may be modelled using homogenized finite element models based on density (i.e., skeletal volume fraction, ) and/or fabric information. (B) The morphology of the skeletal branches needs to be considered modelling smaller coral colonies, using, for example, nonlinear beams.
The 3D graph representation we presented here may serve to define in silico mechanical models of these smaller colonies consisting of nonlinear beams (Figure 10B). Beam FE models have demonstrated to predict the mechanical properties of complex structures in an accurate and computational efficient manner (Pothuaud et al., 2004; Stauber et al., 2004). Since at small length scales L. pertusa skeletal structure is not random, the properties of each beam (i.e., skeletal branch) may account for the statistical information of the morphological analysis we introduce here. Future analyses would need to validate the use of such specimen-specific beam FE models against image-based FE models to confirm its applicability for predictions of the risk of crumbling of L. pertusa skeletal structures.
The expected reduced computational cost of the models we identified here can ease the translation from the mechanical response at the structural level to entire reefs, facilitating in silico models at the reef scale to investigate the mechanical vulnerability of CWC reefs to ocean acidification. Most importantly, we could model the increased dissolution in the dead framework observed from increasing aragonite concentration (Hennige et al., 2020) as a decrease in the skeletal volume fraction in our homogenized models or a reduced branch thickness in our beam models.
Our study points to some important gaps in knowledge. Although we provide a detailed morphological evaluation of a large number of L. pertusa skeletal fragments, these only represent a fraction of the colony from two unique sites in Norway. While these two locations represent different environmental conditions, future analysis should focus on the characterization of morphological variations of skeletal fragments collected from other regions to better understand the impact of local environmental stressors on L. pertusa structure. To date, monitoring reef corals largely relies on 2D measurements of colony size or colony cover (De Clippele et al., 2018; Vad et al., 2017), thus lacking high-resolution volumetric information. To investigate the risk of crumbling of larger colonies we need to investigate new techniques that allow us to infer 3D volumetric information from planar data (House et al., 2018). The most crucial gap is potentially the lack of information on time estimates for morphological changes due to exposure to ocean acidification and consequent increasing rates of bioerosion (Büscher et al., 2022). This will require extension of previous mesocosm experiments (Hennige et al., 2020) to different acidification conditions over long time-scales and complementary in situ measurements of seawater chemistry to establish an exposure trajectory of aragonite concentrations and the relationships with ocean acidification induced dissolution. This data would allow us to use the proposed surrogate models as predictive tools to investigate timescales of loadbearing capacity changes as well as the impact of those changes based on the time CWCs are exposed to acidified waters, and if combined with models detailing where corals of the future may be (Cordes et al., 2023), we can also start to assess the habitat quality of these reefs into the future.
5 Conclusion
We here investigated the critical size of a representative volume element of L. pertusa skeletons based on morphological variations to evaluate mechanical surrogate models of their skeletal structure. We proposed a size limit of five interbranch lengths that allows the determination of the type of model to be used based on the characteristic material architecture of coral skeletons. Our morphological analyses point to large variations between L. pertusa skeletal fragments and branches, as well as dead and live skeletal structures which are driven by growth and adaptation to environmental stressors. Spatially large colonies may be modelled using homogenized FE models by averaging the mechanical properties over five interbranch lengths, whereas small colonies may be modelled using specimen-specific beam-like FE models. Both approaches may allow us to efficiently scale up the analysis to entire reef systems to investigate reef crumbling due to the time they are exposed to acidified waters. Ultimately, this will support future conservation and management efforts by indicating which marine ecosystems are at greatest risk, when they will be at risk, and how much of an impact this will have on the biodiversity they support.
Data availability statement
The original contributions presented in the study are included in the article/Supplementary Material. Further inquiries can be directed to the corresponding authors.
Author contributions
MPF: Conceptualization, Data curation, Formal analysis, Investigation, Methodology, Validation, Visualization, Writing – original draft, Writing – review & editing. JW: Methodology, Writing – review & editing. JB: Data curation, Investigation, Writing – review & editing. JMR: Funding acquisition, Writing – review & editing. SH: Conceptualization, Funding acquisition, Project administration, Resources, Supervision, Writing – review & editing. UW: Conceptualization, Funding acquisition, Project administration, Resources, Supervision, Writing – review & editing.
Funding
The author(s) declare financial support was received for the research, authorship, and/or publication of this article. This work was supported by a Leverhulme Trust Research Project Grant to UW (RPG-2020-215) and an Independent Research Fellowships to SH (NE/K009028/1, NE/K009028/2). Norwegian coral samples were collected as part of the German coordinated BMBF (Federal Ministry of Education and Research)-funded project BIOACID II (FKZ 03F0655A). This paper is a contribution to the European Union’s Horizon 2020 research and innovation program under grant agreement no. 678760 (ATLAS) and no. 818123 (iAtlantic), and the UKRI GCRF One Ocean Hub (NE/S008950/1). It reflects the authors’ views, and the European Union is not responsible for any use that may be made of the information it contains.
Acknowledgments
The authors acknowledge Dr Jürgen Titschack for providing the CT datasets. We would also like to thank Dr Carola Daniel for her assistance during CT imaging at the University of Edinburgh and captains and crews of research cruises POS455 (2013) and POS473 (2014) with RV POSEIDON during which Norwegian samples were collected.
Conflict of interest
The authors declare that the research was conducted in the absence of any commercial or financial relationships that could be construed as a potential conflict of interest.
The author(s) declared that they were an editorial board member of Frontiers, at the time of submission. This had no impact on the peer review process and the final decision.
Publisher’s note
All claims expressed in this article are solely those of the authors and do not necessarily represent those of their affiliated organizations, or those of the publisher, the editors and the reviewers. Any product that may be evaluated in this article, or claim that may be made by its manufacturer, is not guaranteed or endorsed by the publisher.
Supplementary material
The Supplementary Material for this article can be found online at: https://www.frontiersin.org/articles/10.3389/fmars.2024.1456505/full#supplementary-material
References
Addamo A. M., Martínez-Baraldés I., Vertino A., López-González P. J., Taviani M., Machordom A. (2015). Morphological polymorphism of Desmophyllum dianthus (Anthozoa: Hexacorallia) over a wide ecological and biogeographic range: Stability in deep habitats? Zool Anz 259, 113–130. doi: 10.1016/j.jcz.2015.10.004
Addamo A. M., Vertino A., Stolarski J., García-Jiménez R., Taviani M., Machordom A. (2016). Merging scleractinian genera: The overwhelming genetic similarity between solitary Desmophyllum and colonial Lophelia. BMC Evol. Biol. 16, 108. doi: 10.1186/s12862-016-0654-8
Barnhill K. A., Roberts J. M., Myers-Smith I., Williams M., Dexter K. G., Ryan C., et al. (2023). Incorporating dead material in ecosystem assessments and projections. Nat. Clim Chang 13, 113–115. doi: 10.1038/s41558-022-01565-5
Beuck L., Freiwald A., Taviani M. (2010). Spatiotemporal bioerosion patterns in deep-water scleractinians from off Santa Maria di Leuca (Apulia, Ionian Sea). Deep Res. Part II Top. Stud. Oceanogr 57, 458–470. doi: 10.1016/j.dsr2.2009.08.019
Büscher J. V., Form A. U., Wisshak M., Kiko R., Riebesell U. (2022). Cold-water coral ecosystems under future ocean change: Live coral performance vs. framework dissolution and bioerosion. Limnol Oceanogr. 67, 2497–2515. doi: 10.1002/lno.12217
Büscher J. V., Juva K., Flögel S., Wisshak M., Rüggeberg A., Riebesell U., et al. (2024). Water mass characteristics and hydrodynamics at an inshore versus an offshore mid-Norwegian cold-water coral reef habitat. Front. Mar. Sci. 11, 1363542. doi: 10.3389/fmars.2024.1363542
Büscher J. V., Wisshak M., Form A. U., Titschack J., Nachtigall K., Riebesell U. (2019). In situ growth and bioerosion rates of Lophelia pertusa in a Norwegian fjord and open shelf cold-water coral habitat. PeerJ 2019, 1–36. doi: 10.7717/peerj.7586
Caley M. J., St John J. (1996). Refuge Availability Structures Assemblages of Tropical Reef Fishes. Journal of Animal Ecology 65(4), 414–428. doi: 10.2307/5777
Chamberlain J., Graus R. R. R. (1975). Water flow and hydromechanical adaptations of branched reef corals. Bull. Mar. Sci. 25, 112–125.
Cole A. J., Pratchett M. S., Jones G. P. (2008). Diversity and functional importance of coral-feeding fishes on tropical coral reefs. Fish and Fisheries 9, 286–307. doi: 10.1111/j.1467-2979.2008.00290.x
Cordes E. E., Mienis F., Gasbarro R., Davies A., Baco A. R., Bernardino A. F., et al. (2023). “A global view of the cold-water coral reefs of the world BT - cold-water coral reefs of the world,”. Eds. Cordes E., Mienis F. (Cham: Springer International Publishing) 19, 1–30. doi: 10.1007/978-3-031-40897-7_1
Cormen T. H., Leiserson C. E., Rivest R. L., Stein C. (2001). Introduction to algorithms. 2nd ed. (Cambridge, MA: MIT Press and McGraw-Hill).
Cowin S. C. (1985). The relationship between the elasticity tensor and the fabric tensor. Mech. Mater 4, 137–147. doi: 10.1016/0167-6636(85)90012-2
Cowin S. C. (1986). Fabric dependence of an anisotropic strength criterion. Mech. Mater 5, 251–260. doi: 10.1016/0167-6636(86)90022-0
Davoodi Kermani I., Schmitter M., Eichinger J. F., Aydin R. C., Cyron C. J. (2021). Computational study of the geometric properties governing the linear mechanical behavior of fiber networks. Comput. Mater Sci. 199, 110711. doi: 10.1016/j.commatsci.2021.110711
De Clippele L. H., Huvenne V. A. I., Orejas C., Lundälv T., Fox A., Hennige S. J., et al. (2018). The effect of local hydrodynamics on the spatial extent and morphology of cold-water coral habitats at Tisler Reef, Norway. Coral Reefs 37, 253–266. doi: 10.1007/s00338-017-1653-y
Dirrenberger J., Forest S., Jeulin D. (2019). Computational Homogenization of Architectured Materials. In: Estrin Y., Bréchet Y., Dunlop J., Fratzl P. (eds) Architectured Materials in Nature and Engineering. Springer Series in Materials Science, vol 282. Springer, Cham, Switzerland. doi: 10.1007/978-3-030-11942-3_4
Doube M. (2015). The ellipsoid factor for quantification of rods, plates, and intermediate forms in 3D geometries. Front. Endocrinol. (Lausanne) 6. doi: 10.3389/fendo.2015.00015
Doube M., Klosowski M. M., Arganda-Carreras I., Cordelières F. P., Dougherty R. P., Jackson J. S., et al. (2010). BoneJ: Free and extensible bone image analysis in ImageJ. Bone 47, 1076–1079. doi: 10.1016/j.bone.2010.08.023
Drugan W. J., Willis J. R. (1996). A micromechanics-based nonlocal constitutive equation and estimates of representative volume element size for elastic composites. J. Mech. Phys. Solids 44, 497–524. doi: 10.1016/0022-5096(96)00007-5
Farber C., Titschack J., Hanna Lydia Schönberg C., Ehrig K., Boos K., Baum D., et al. (2016). Long-term macrobioerosion in the Mediterranean Sea assessed by micro-computed tomography. Biogeosciences 13, 3461–3474. doi: 10.5194/bg-13-3461-2016
Gass S. E., Roberts J. M. (2011). Growth and branching patterns of Lophelia pertusa (Scleractinia) from the North Sea. J. Mar. Biol. Assoc. United Kingdom 91, 831–835. doi: 10.1017/S002531541000055X
Georgoulas K., Hennige S., Lee Y. C. (2023). Smoothed particle hydrodynamics for modelling cold-water coral habitats in changing oceans. J. Sea Res. 192, 102358. doi: 10.1016/j.seares.2023.102358
Graus R. R. Jr, Chamberlain J. A., Boker A. M. M. (1977). Structural modification of corals in relation to waves and currents. Stud. Geol 4, 135–153.
Hagberg A., Swart P S., Chult D. (2008). Exploring Network Structure, Dynamics, and Function using NetworkX. Proceedings of the Python in Science Conference.
Harrigan T. P., Jasty M., Mann R. W., Harris W. H. (1988). Limitations of the continuum assumption in cancellous bone. J. Biomech 21, 269–275. doi: 10.1016/0021-9290(88)90257-6
Hennige S. J., Morrison C. L., Form A. U., Büscher J., Kamenos N. A., Roberts J. M. (2014). Self-recognition in corals facilitates deep-sea habitat engineering. Sci. Rep. 4, 6782. doi: 10.1038/srep06782
Hennige S. J., Wicks L. C., Kamenos N. A., Perna G., Findlay H. S., Roberts J. M. (2015). Hidden impacts of ocean acidification to live and dead coral framework. Proc. R Soc. B Biol. Sci. 282, 28220150990. doi: 10.1098/rspb.2015.0990
Hennige S. J., Wolfram U., Wickes L., Murray F., Roberts J. M., Kamenos N. A., et al. (2020). Crumbling reefs and cold-water coral habitat loss in a future ocean: evidence of “Coralporosis” as an indicator of habitat integrity. Front. Mar. Sci. 7. doi: 10.3389/fmars.2020.00668
Hildebrand T., Laib A., Müller R., Dequeker J., Rüegsegger P., Ller R. M., et al. (1999). Direct three-dimensional morphometric analysis of human cancellous bone: microstructural data from spine, femur, iliac crest, and calcaneus. J. Bone Miner Res. 14, 1167–1174. doi: 10.1359/jbmr.1999.14.7.1167
Hill R. (1963). Elastic properties of reinforced solids: Some theoretical principles. J. Mech. Phys. Solids 11, 357–372. doi: 10.1016/0022-5096(63)90036-X
Hollister S. J., Brennan J. M., Kikuchi N. (1994). A homogenization sampling procedure for calculating trabecular bone effective stiffness and tissue level stress. J. Biomech 27, 433–444. doi: 10.1016/0021-9290(94)90019-1
House J. E., Brambilla V., Bidaut L. M., Christie A. P., Pizarro O., Madin J. S., et al. (2018). Moving to 3D: Relationships between coral planar area, surface area and volume. PeerJ 6, e4280. doi: 10.7717/peerj.4280
Kanit T., Forest S., Galliet I., Mounoury V., Jeulin D. (2003). Determination of the size of the representative volume element for random composites: statistical and numerical approach. Int. J. Solids Struct. 40, 3647–3679. doi: 10.1016/S0020-7683(03)00143-4
Khalil R., Kallel S., Farhat A., Dlotko P. (2022). Topological Sholl descriptors for neuronal clustering and classification. PloS Comput. Biol. 18, e1010229. doi: 10.1371/journal.pcbi.1010229
Khisaeva Z. F., Ostoja-Starzewski M. (2006). On the size of RVE in finite elasticity of random composites. J. Elast 85, 153–173. doi: 10.1007/s10659-006-9076-y
Kline D. I., Teneva L., Okamoto D. K., Schneider K., Caldeira K., Miard T., et al. (2019). Living coral tissue slows skeletal dissolution related to ocean acidification. Nat. Ecol. Evol. 3, 1438–1444. doi: 10.1038/s41559-019-0988-x
Kruszyński K. J., Kaandorp J. A., van Liere R. (2007). A computational method for quantifying morphological variation in scleractinian corals. Coral Reefs 26, 831–840. doi: 10.1007/s00338-007-0270-6
Madin J. S., Connolly S. R. (2006). Ecological consequences of major hydrodynamic disturbances on coral reefs. Nature 444, 477–480. doi: 10.1038/nature05328
Maquer G., Musy S. N., Wandel J., Gross T., Zysset P. K. (2015). Bone volume fraction and fabric anisotropy are better determinants of trabecular bone stiffness than other morphological variables. J. Bone Miner Res. 30, 1000–1008. doi: 10.1002/jbmr.2437
Mortensen P. B., Fossâ J. H. (2006). Species diversity and spatial distribution of invertebrates on deep-water Lophelia reefs in Norway, Vol. 1868. 1849–1868.
Mortensen P. B., Hovland T., Fosså J. H., Furevik D. M. (2001). Distribution, abundance and size of Lophelia pertusa coral reefs in mid-Norway in relation to seabed characteristics. J. Mar. Biol. Assoc. United Kingdom 81 (4), 581–597. doi: 10.1017/S002531540100426X
Ohs N., Collins C. J., Tourolle D. C., Atkins P. R., Schroeder B. J., Blauth M., et al. (2021). Automated segmentation of fractured distal radii by 3D geodesic active contouring of in vivo HR-pQCT images. Bone 147, 115930. doi: 10.1016/j.bone.2021.115930
Pahr D. H., Zysset P. K. (2008). Influence of boundary conditions on computed apparent elastic properties of cancellous bone. Biomech Model. Mechanobiol 7, 463–476. doi: 10.1007/s10237-007-0109-7
Pahr D. H., Zysset P. K. (2009). A comparison of enhanced continuum FE with micro FE models of human vertebral bodies. J. Biomech 42, 455–462. doi: 10.1016/j.jbiomech.2008.11.028
Paulay G. (1997). Diversity and distribution of reef organisms. in Birkeland C. E., editor. Life and death of coral reefs. (New York, New York, USA: Chapman & Hall), 298–353.
Pasquini L., Molinari A., Fantazzini P., Dauphen Y., Cuif J. P., Levy O., et al. (2015). Isotropic microscale mechanical properties of coral skeletons. J. R Soc. Interface 12, 1–9. doi: 10.1098/rsif.2015.0168
Peña Fernández M., Sasso S. J., McPhee S., Black C., Kanczler J., Tozzi G., et al. (2022). Nonlinear micro finite element models based on digital volume correlation measurements predict early microdamage in newly formed bone. J. Mech. Behav. BioMed. Mater 132, 105303. doi: 10.1016/j.jmbbm.2022.105303
Pfeiffer F., Rammerstorfer F. G., Salençon J. (1997). Continuum micromechanics (Vienna: Springer Vienna). doi: 10.1007/978-3-7091-2662-2
Pothuaud L., van Rietbergen B., Charlot C., Ozhinsky E., Majumdar S. (2004). A new computational efficient approach for trabecular bone analysis using beam models generated with skeletonized graph technique. Comput. Methods Biomech BioMed. Engin 7, 205–213. doi: 10.1080/10255840412331285943
Quattrini A. M., Gómez C. E., Cordes E. E. (2017). Environmental filtering and neutral processes shape octocoral community assembly in the deep sea. Oecologia 183, 221–236. doi: 10.1007/s00442-016-3765-4
Rasser M. W., Riegl B. (2002). Holocene coral reef rubble and its binding agents. Coral Reefs 21, 57–72. doi: 10.1007/s00338-001-0206-5
Roberts J. M., Wheeler A. J., Freiwald A. (2006). Reefs of the deep: The biology and geology of cold-water coral ecosystems. Sci. (80- ) 312, 543–547. doi: 10.1126/science.1119861
Roberts J. M., Wheeler A., Freiwald A., Cairns S. (2009). Cold-water corals: the biology and geology of deep-sea coral habitats (Cambridge: Cambridge University Press). doi: 10.1017/CBO9780511581588
Sanna G., Büscher J. V., Freiwald A. (2023). Cold-water coral framework architecture is selectively shaped by bottom current flow. Coral Reefs 42, 483–495. doi: 10.1007/s00338-023-02361-z
Sanna G., Freiwald A. (2021). Deciphering the composite morphological diversity of Lophelia pertusa, a cosmopolitan deep-water ecosystem engineer. Ecosphere 12 (11), e03802. doi: 10.1002/ecs2.3802
Schindelin J., Arganda-Carreras I., Frise E., Kaynig V., Longair M., Pietzsch T., et al. (2012). Fiji: an open-source platform for biological-image analysis. Nat. Meth 9, 676–682. doi: 10.1038/nmeth.2019
Sebens K. P., Witting J., Helmuth B. (1997). Effects of water flow and branch spacing on particle capture by the reef coral Madracis mirabilis (Duchassaing and Michelotti). J. Exp. Mar. Bio Ecol. 211, 1–28. doi: 10.1016/S0022-0981(96)02636-6
Stauber M., Huber M., van Lenthe G. H., Boyd S. K., Müller R. (2004). A finite element beam-model for efficient simulation of large-scale porous structures. Comput. Methods Biomech BioMed. Engin 7, 9–16. doi: 10.1080/10255840410001656408
Storlazzi C. D., Brown E. K., Field M. E., Rodgers K., Jokiel P. L. (2005). A model for wave control on coral breakage and species distribution in the Hawaiian Islands. Coral Reefs 24, 43–55. doi: 10.1007/s00338-004-0430-x
Trias D., Costa J., Turon A., Hurtado J. E. (2006). Determination of the critical size of a statistical representative volume element (SRVE) for carbon reinforced polymers. Acta Mater 54, 3471–3484. doi: 10.1016/j.actamat.2006.03.042
Vad J., Orejas C., Moreno-Navas J., Findlay H. S., Roberts J. M. (2017). Assessing the living and dead proportions of cold-water coral colonies: Implications for deep-water Marine Protected Area monitoring in a changing ocean. PeerJ 2017, 1–20. doi: 10.7717/peerj.3705
van Rietbergen B., Weinans H., Huiskes R., Odgaard A. (1995). A new method to determine trabecular bone elastic properties and loading using micromechanical finite-element models. J. Biomech 28, 69–81. doi: 10.1016/0021-9290(95)80008-5
Von Euw S., Zhang Q., Manichev V., Murali N., Gross J., Feldman L. C., et al. (2017). Biological control of aragonite formation in stony corals. Sci. (80- ) 356, 933–938. doi: 10.1126/science.aam6371
Vosburgh F. (1982). Acropora reticulata : structure, mechanics and ecology of a reef coral. Proc. R Soc. London Ser. B Biol. Sci. 214, 481–499. doi: 10.1098/rspb.1982.0023
Wangpraseurt D., Larkum A. W. D., Ralph P. J., Kühl M. (2012). Light gradients and optical microniches in coral tissues. Front. Microbiol. 3. doi: 10.3389/fmicb.2012.00316
Wisshak M., Schönberg C. H. L., Form A., Freiwald A. (2012). Ocean acidification accelerates reef bioerosion. PloS One 7, e45124. doi: 10.1371/journal.pone.0045124
Wolfram U., Peña Fernández M., McPhee S., Smith E., Beck R. J., Shephard J. D., et al. (2022). Multiscale mechanical consequences of ocean acidification for cold-water corals. Sci. Rep. 12, 1–17. doi: 10.1038/s41598-022-11266-w
Zawada Kyle J. A., Dornelas M., Madin J. S. (2019b). Quantifying coral morphology. Coral Reefs 38, 1281–1292. doi: 10.1007/s00338-019-01842-4
Keywords: cold-water corals, Lophelia pertusa, ocean acidification, mechanical modelling, 3D morphology
Citation: Peña Fernández M, Williams J, Büscher JV, Roberts JM, Hennige SJ and Wolfram U (2025) Morphological analysis of cold-water coral skeletons for evaluating in silico mechanical models of reef-scale crumbling. Front. Mar. Sci. 11:1456505. doi: 10.3389/fmars.2024.1456505
Received: 28 June 2024; Accepted: 16 December 2024;
Published: 21 January 2025.
Edited by:
Manuel Maldonado, Spanish National Research Council (CSIC), SpainReviewed by:
Jaap Kaandorp, University of Amsterdam, NetherlandsFiorella Prada, Rutgers, The State University of New Jersey, United States
Copyright © 2025 Peña Fernández, Williams, Büscher, Roberts, Hennige and Wolfram. This is an open-access article distributed under the terms of the Creative Commons Attribution License (CC BY). The use, distribution or reproduction in other forums is permitted, provided the original author(s) and the copyright owner(s) are credited and that the original publication in this journal is cited, in accordance with accepted academic practice. No use, distribution or reproduction is permitted which does not comply with these terms.
*Correspondence: Marta Peña Fernández, bS5wZW5hX2Zlcm5hbmRlekBody5hYy51aw==; Uwe Wolfram, dXdlLndvbGZyYW1AdHUtY2xhdXN0aGFsLmRl