- 1Civil, Infrastructural and Environmental Engineering Department, Khalifa University of Science and Technology, Abu Dhabi, United Arab Emirates
- 2Department of Engineering Mathematics and Internetworking, Dalhousie University, Halifax, NS, Canada
- 3Meteorological Research Division, Environment and Climate Change Canada (ECCC), Dorval, QC, Canada
- 4Meteorological Service of Canada, Environment and Climate Change Canada (ECCC), Dorval, QC, Canada
- 5Fisheries and Oceans Canada, Bedford Institute of Oceanography, Dartmouth, NS, Canada
The Surface Water Ocean Topography (SWOT) mission significantly improves on the capabilities of current nadir altimeters by enabling two-dimensional mapping. Assimilating this advanced data into high-resolution models poses challenges. To address this, Observing System Simulation Experiments (OSSEs) were conducted to evaluate the effects of both simulated and actual SWOT data on the Regional Ice Ocean Prediction System (RIOPS). This study examines the OSSEs’ design, focusing on the simulated observations and assimilation systems used. The validity of the OSSE designs is confirmed by ensuring the deviations between the assimilation system and the Nature Run (NR) align with discrepancies observed between actual oceanic data and OSSE simulations. The study measures the impact of assimilating SWOT and two nadir altimeters by calculating root mean square forecast error for sea surface height (SSH), temperature, and velocities, along with performing wave-number spectra and coherence analyses of SSH errors. The inclusion of SWOT data is found to reduce RMS SSH errors by 16% and RMS velocity errors by 6% in OSSEs. The SSH error spectrum shows that the most notable improvements are for scales associated with the largest errors in the range of 200-400 km, with a 33% reduction compared to traditional data assimilation. Additionally, spectral coherence analysis shows that the limit of constrained scales is reduced from 280 km for conventional observations to 195 km when SWOT is assimilated as well. This study also represents our first attempt at assimilating early-release SWOT data. A set of Observing System (data denial) experiments using early-release SWOT measurements shows similar (but smaller) responses to OSSE experiments in a two nadir-altimeter context. In a six-altimeter constellation setup, a positive impact of SWOT is also noted, but of significantly diminished amplitude. These findings robustly advocate for the integration of SWOT observations into RIOPS and similar ocean analysis and forecasting frameworks.
1 Introduction
Nadir altimeter Sea Level Anomaly (SLA) measurements have significantly enriched our understanding of ocean circulation dynamics. While along-track SLA data can discern wavelengths as small as 50–70 km (Dufau et al., 2016), the global mesoscale resolution remains limited by the spacing (distance between adjacent tracks) and temporal samplings (repeat period) of individual altimeter missions. Multiple altimeters are indispensable for achieving global maps of mesoscale variability. Numerous studies have evaluated the efficacy of altimeter constellations (e.g., Pascual et al., 2006; Dibarboure et al., 2011), affirming that a minimum of three to four altimeters is requisite for reconstructing the global ocean surface topography at a mesoscale resolution. Nevertheless, amalgamating data from multiple altimeter missions falls short of resolving wavelengths smaller than 150–200 km (e.g., Ducet et al., 2000; Le Traon, 2013).
The Surface Water Ocean Topography (SWOT) mission, launched on 16 December 2022 through collaboration among NASA, CNES, the Canadian Space Agency, and the UK Space Agency, promises to expand on the capabilities of existing nadir altimeters. It will facilitate two-dimensional mapping at a significantly enhanced effective resolution, down to wavelengths as small as 20 km (e.g., Fu and Ferrari, 2008; Fu et al., 2009). This advancement will be realized through both a nadir altimeter and a Ka-band Radar Interferometer (KaRIn). With a swath width of 120 km, the spatial coverage will span nearly the entire globe every 21 days. Although SWOT will furnish highly detailed observations along its swaths, it will overlook the evolution of high-frequency signals (with periods less than 21 days). Integrating SWOT data with that from conventional along-track altimeters (e.g., Pujol et al., 2012) and very high-resolution models (with resolutions of a few kilometers) present a significant challenge, necessitating dynamic interpolation.
Observing System Simulation Experiments (OSSEs; Halliwell et al., 2014) is a prevalent approach for assessing the impact of new observations on analysis and forecasting systems. It entails simulating the “true” ocean using a numerical model and subsequently determining instrument sampling and errors using predefined parameters. This method facilitates the evaluation of how future measurements could augment existing analyses and forecasts based on assimilation systems, and guides the design of observation networks to enhance ocean state sampling at specific spatial and temporal scales. Given the potential importance of the SWOT mission, studies of various complexity have begun to assess how to best make use of this new type of observation. Simplified models such as Quasi-Geostrophic and Surface Quasi-Geostrophic models have been employed to assess SWOT observability and estimate critical ocean state features such as vertical velocity fields (e.g., Klein et al., 2009; Qiu et al., 2016). While advantageous due to their conceptual simplicity compared to Primitive Equation (PE) models, these simplified models do not encapsulate the full spectrum of oceanic regimes. Thus, preparing to employ SWOT observations with more complex ocean models is imperative. Recently, Carrier et al. (2016) conducted an OSSE utilizing SWOT observations and a PE model of the Gulf of Mexico, showcasing improved forecast scores and representation of mesoscale features compared to assimilation of data from conventional altimeters. Benkiran et al. (2021) and Tchonang et al. (2021) investigated and analyzed the impact of SWOT observations over the global oceans using a different version of their 1/12th resolution model as “truth” and found significant improvements in SSH and surface current errors. Souopgui et al. (2020) quantified improvements in ocean state estimation through the assimilation of simulated SWOT observations by using a multi-scale 3DVAR approach with an OSSE. This approach effectively enhances skill metrics across spatial scales by initially creating large-scale analyses and incrementally incorporating smaller-scale corrections, with significant improvements in mixed layer depth estimates. Zhou et al. (2024) introduced a novel multi-scale Four-Dimensional Variational Data Assimilation (4DVAR) system to enhance the assimilation of high-resolution SWOT SSH within the Regional Ocean Modeling System (ROMS), demonstrating significant improvements in model accuracy and fine-scale feature representation.
This paper uses an approach similar to that of Benkiran et al. (2021) and extends previous studies in three ways. First, the Nature Run (from which the synthetic observations are taken) is a higher-resolution (1-km horizontal grid) configuration that includes greater energy at small spatial scales than the assimilation model. This additional variability simulates the unresolved scales present in real world. Second, the ocean model used in the OSSE contains external tidal forcing, which also increases the complexity of online filtering required in the assimilation of sea surface height data. Finally, we also confirm the impact of SWOT using Observing System Experiments with early-release measurements over a roughly 2-month period.
The paper’s structure is as follows: Section 2 focuses on the Nature Run description and provides details concerning the production of synthetic observations. Section 3 presents the assimilation system, highlighting changes made to the data assimilation scheme for the OSSEs, followed by the OSSE design. In Section 4, the impacts of the assimilation for the Sea Surface Height (SSH) and Velocity field are evaluated. In Section 5, we focus on the wavenumber analysis of the impacts of the data assimilation by showing the SSH error spectrum and coherence, while Section 6 concentrates on data withholding experiments using early-release SWOT data over the North Atlantic and Arctic Oceans for two months. Section 7 draws conclusions regarding the impacts of the data assimilation experiments and presents future perspectives.
2 Data
2.1 NATL60 nature run simulation
The NATL60 simulation (referred to hereafter as the Nature Run (NR) simulation), based on the NEMO framework (Madec et al., 2019), features a horizontal grid with variable spacing from 1.6 km at 26°N to 0.9 km at 65°N. The grid is tailored to capture the scales of motion targeted by the SWOT altimetric mission. For initial and boundary conditions, the model employs the GLORYS2v3 ocean reanalysis (Masina et al., 2017), incorporating a relaxation zone at the northern boundary to manage sea ice concentration and thickness. Vertically, the model includes 300 levels, with the finest resolution of 1 m in the uppermost layers. Atmospheric conditions are determined using the DFS5.2 dataset (Dussin et al., 2018). Grid structure and bathymetry are aligned with the specifications outlined by Ducousso et al. (2017). To dynamically adjust lateral viscosity and diffusivity according to flow characteristics, a third-order upwind advection scheme is employed for both momentum and tracers. The model undergoes a 6-month spin-up phase followed by a detailed 1-year simulation the period covering from October 1, 2012, to September 30, 2013, the results of which have been referenced in recent publications (Amores et al., 2018; Buckingham et al., 2019; Ajayi et al., 2020). Unlike OSSE simulations (described in Section 3), NATL60 does not include tidal forcing. A more recent simulation by this model (referred to as eNATL60) does include tides but is only available for a shorter period. As a result, we prefer to use NATL60. Figure 1 shows the NATL60 domain and the SSH snapshot on August 1, 2013. The spectral and coherence analyses are conducted in the nested black rectangular region (Gulf Stream) in Section 5.
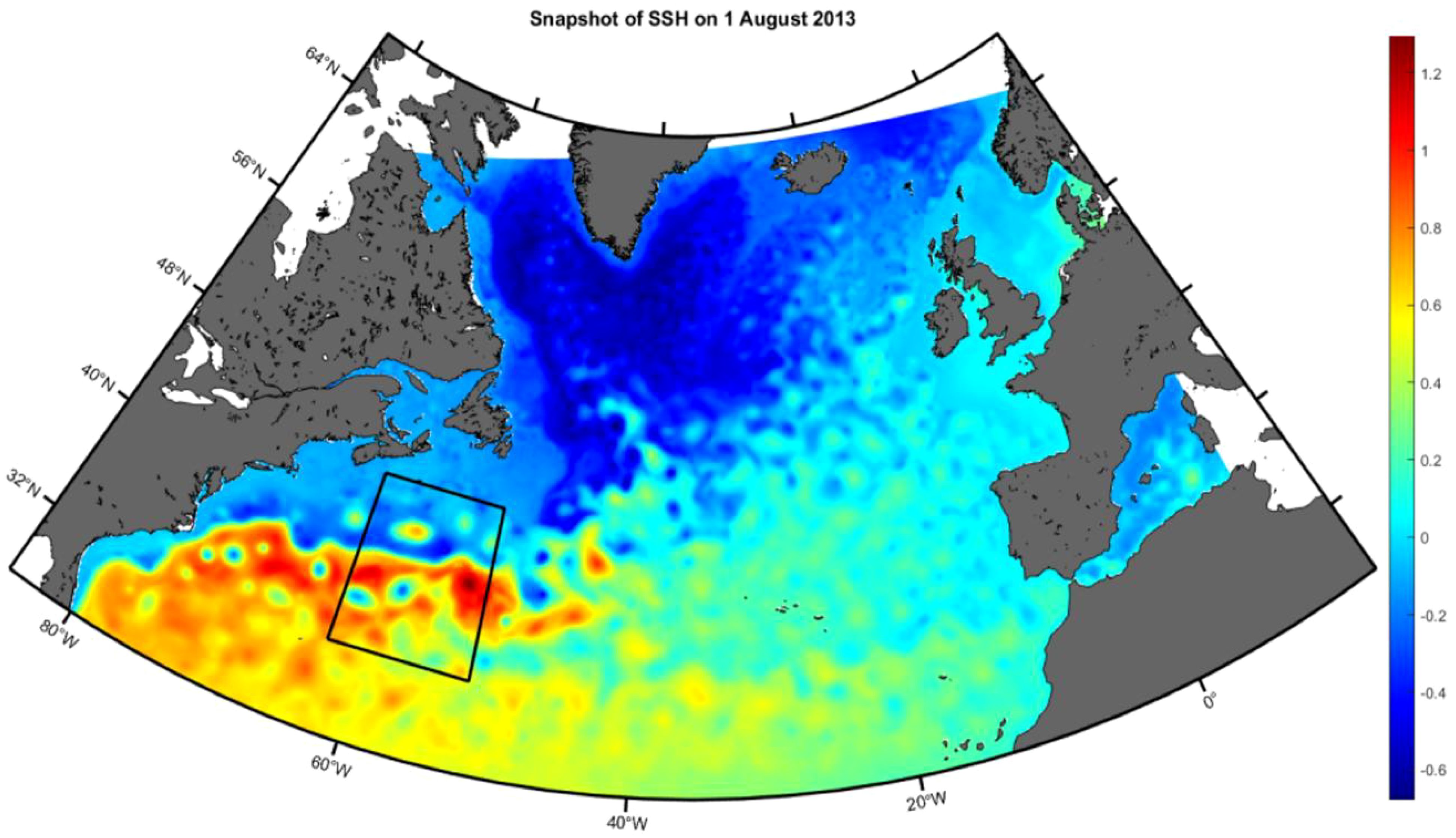
Figure 1. NATL60 domain and the daily-mean SSH (m) snapshot for August 1, 2013. The black nested rectangle delineates the dynamic Gulf Stream region, with a side length of 1000 kilometers and centered at coordinates (-57.5, 38.5).
2.2 Generation of synthetic observations
To accurately assess the impact of assimilating SWOT data, we need to generate synthetic observations for both the conventional observing network and the estimated measurements from SWOT. Since the Nature Run (NR) is a model simulation that doesn’t exactly match the real ocean state, producing these synthetic observations ensures consistency between the observations and the NR. This approach allows us to evaluate the impact of assimilating SWOT data without the confounding effects arising from discrepancies between the NR and real-world observations. It is also important to add Gaussian noise to the synthetic observations to simulate observational error. Details concerning both these datasets and noise characteristics are provided below.
2.2.1 Conventional observations
Synthetic observations are produced for conventional observations currently assimilated in RIOPS (as described in Section 3). These observations include nadir altimetry, gridded Sea Surface Temperature (SST), and vertical profiles of temperature and salinity. Synthetic observations were extracted from the NATL60 simulation, and these observations were collected over a period of 12 months (from October 1, 2012 to September 30, 2013) which includes the period covered by the OSSEs. The daily-mean SST was directly extracted from the NR over the full grid to simulate the Level-4 SST product usually assimilated in RIOPS. A random error of 0.5°C was applied to the SST. This value corresponds roughly to the nominal error of Level-4 SST analyses (as used in the operational version of RIOPS). Errors applied to the nadir altimeters are described in Section 2.2.2 below.
The temperature and salinity (T/S) profiles were extracted at the same points and dates as the real in situ profiles observed as found in the CORA4.1 database provided by the Coriolis and CMEMS in situ data center (Cabanes et al., 2013). Observations include profiles from the Argo Array, field campaigns, gliders and moorings. 3D daily mean temperature and salinity fields from the NR were used to simulate this in situ data as instantaneous outputs were not available. This is expected to lead to a somewhat reduced variance of synthetic profile observations as compared to the real world for coastal observations (e.g. gliders), although this is not expected to impact the sensitivity of the system to the assimilation of wide-swath altimetry. To simulate instrument error, we applied a random error with a standard deviation of 0.05°C for temperature and 0.01 psu for salinity. We did not explicitly include representativeness error because it is implicitly accounted for by using a high-resolution Nature Run (NR) to generate the synthetic observations.
2.2.2 Simulated nadir data
The along-track nadir pseudo-observations datasets contain noise-free SSH data, which is the direct interpolation of the hourly model SSH onto the nadir track. And the SSH data with simulated noise is obtained using the “SWOT simulator” (Esteban-Fernandez et al., 2017). As explained in the SWOT simulator reference manual, the simulated noise for the nadir altimeter follows a spectrum of error consistent with global estimates from the Jason-2 altimeter. The along-track point spacing is 7 km for Jason-2 and Cryosat-2, 7 km for the SWOT nadir observations.
2.2.3 Simulated SWOT data
Surface Water Ocean Topography provides global SLA observations under a 120 km wide-swath with a middle gap of 20 km. In this study, we considered the SWOT data as two-dimensional fields under the swath with a regular along-track and across-track resolution of 7 km. The pseudo-SWOT observations were simulated from hourly outputs of the NR using the “SWOT Simulator” developed at the Jet Propulsion Laboratory (Gaultier et al., 2016), which is used to generate observations with the expected SWOT sampling and error budget. The along-track and cross-track point spacing is 7 km for the SWOT KaRIn observations. The SWOT simulator models the most significant errors that are expected to affect the SWOT data, i.e., the KaRIn noise, roll errors, phase errors, baseline dilation errors, and timing errors. It produces random realizations of uncorrelated noise and correlated errors following the spectral descriptions of the SWOT error budget document (Esteban-Fernandez et al., 2017).
In our experiments, we only used the KaRin noise for two reasons: (i) the simulator models the worst expected case and (ii) the observation distribution centers are planning to filter the data from most of these errors. Consequently, as the final error budget is still uncertain and as this was our first effort to assimilate such data in a North Atlantic model, we preferred to use a more optimistic error budget. The same simulator was used to simulate the nadir data of SWOT.
2.2.4 Real SWOT data
Section 6 presents data withholding experiments performed using early-release SWOT observations. The observations used were the AVISO v0.3 Level 3 product made available in December 2023. The observations used cover the period 2023-09-06 to 2023-11-22. KaRIn measurements are provided with 2-km spacing both along and cross track. As this is higher resolution than the RIOPS model grid it is necessary to decimate the observations. As a result the observations are averaged using a 9-pt stencil to provide one point every 6 km (AVISO/DUACS, 2024). This approach provides a straightforward means to decimate the data without any a priori knowledge about relative errors of different pixels.
3 Ocean assimilation system and OSSE setup
3.1 Ocean assimilation system
The System d’Assimilation Mercator version 2 (SAM2), a multivariate, reduced-order Extended Kalman Filter, plays a crucial role in constraining oceanic fields toward observations and reducing forecast error. This scheme is specifically deployed for the assimilation of sea level anomaly (SLA), sea surface temperature (SST), and in situ data related to temperature and salinity profiles, as detailed by Wong et al. (2020). For a thorough understanding of SAM2, one can refer to the extensive descriptions by Lellouche et al. (2013) and Lellouche et al. (2018), including its specialized adaptations for RIOPS outlined in Smith et al. (2016, 2021, 2024). Below is a concise overview of the pertinent aspects.
The background error of the model is specified through static multivariate fields derived from sub-monthly anomalies recorded over a decade of hindcasts. RIOPS analyses are produced using a 7-day assimilation period, distributing analysis increments uniformly via an Incremental Analysis Updating method (IAU) as described by Bloom et al. (1996) and Benkiran and Greiner (2008). A multi-scale technique adjusts temperature and salinity fields by implementing large-scale increments from a 3DVar analysis based on average innovations over the last four cycles. The Mean Dynamic Topography (MDT) used in the observation operator for SLA is a hybrid variant discussed in Lellouche et al. (2018), merging the CNES-CLS13 MDT (Rio et al., 2014) with average innovations from ocean reanalysis (Smith et al., 2024).
Furthermore, an online sliding-window harmonic analysis excludes tidal effects within the SLA observation operator, accommodating non-stationary tides influenced by seasonal sea ice, a method detailed in Smith et al. (2021). Additionally, the inverse barometer effect is removed to account for the local model’s atmospheric pressure responses. SLA observations include those typically assimilated within operational frameworks, specifically from satellites like Cryosat2, Jason3, Saral/Altika, and Sentinel 3a/3b.
Regarding SST, ECCC’s gridded Level-4 analyses are utilized (Brasnett and Colan, 2016). A 3DVar ice analysis produced on a 5-km grid is used to constrain sea ice concentration (Buehner et al., 2013, 2016). The ice analysis is blended with the ocean analysis using the Rescaled Forecast Tendencies method from Smith et al. (2016) to modify the ten ice thickness categories based on total ice concentration increments.
3.2 OSSE setup
In an operational context an MDT field is removed from model SSH to provide the model equivalent of SLA observations. As noted above, the MDT field is based on a combination of observations together with mean model innovations. As a result, its use introduces additional errors to the assimilation of SLA which are accounted for through use of an MDT error field with values of up to 20 cm (Lellouche et al., 2018; Smith et al., 2024). Since synthetic observations for the OSSEs are taken from a model, there is no need for an MDT and SSH can be assimilated directly. We have nonetheless kept the MDT error field unchanged to maintain consistency with operational settings and because it also accounts for representative error due to unresolved features. This approach may underrepresent somewhat the errors associated with assimilating SWOT. An instrumental error of 3 cm is used for SWOT data. While this is somewhat higher than estimates of KaRIn error for SWOT, it provides a conservative value that allows for incomplete filtering of other observations errors (e.g. roll, phase). This is also similar to the error value used for most nadir altimeters. We feel that this improves the ‘fairness’ of the comparison with the impacts found for OSSEs using nadir altimeters (i.e. we avoid over-fitting to SWOT due to an overly small and possibly unrealistic error).
As a daily-mean SST is assimilated, the SST observation operator is changed to use a daily mean as well, instead of the nocturnal SST normally used. Note also that since the domain of RIOPS is larger than that for NATL60, for regions outside the NATL60 domain no observations are assimilated. As a result, the blending with the 3DVar ice analysis is not used. Additionally, the 3DVar bias correction for temperature and salinity profile observations is not used as it has a long spin-up time (on the order of a year) and thus would not have time to adjust over the limited OSSE period. Finally, RIOPS usually uses fields from the Global Ice Ocean Prediction to specify open boundary conditions. However, these were not available for this period. As a result, open boundary conditions were produced using fields from the GLORYS12 reanalysis (Lellouche et al., 2018). Initial conditions were also obtained from GLORYS12 fields using a bi-linear interpolation of temperature, salinity and velocities from the 0.08° resolution grid upon which the GLORYS12 fields are disseminated.
Starting from the simulated data obtained from the NR, three OSSEs were carried out using a different NEMO configuration but the same spatial resolution of 1/12° (~7 km). An additional experiment was performed, called the Free Run (FR), in which no observations are assimilated. This simulation is used to assess the relative performance of the assimilative experiments. To assess the impact of SWOT data, it was compared with assimilation of conventional altimeter data from two nadir altimeters Jason-2 and Cryosat-2. The data assimilated in the different OSSEs are detailed in Table 1. The OSSE0 (FREE) is a free run with no data assimilation. OSSE1(STD) is the standard data assimilation of conventional observations including Cryosat-2, Jason-2, T&S profiles and SST. OSSE2 (SWOT) includes the assimilation of data from SWOT, together with T&S profiles and SST. Finally, OSSE3 (SWOT+NADIR) assimilates data from SWOT in addition to conventional data (Cryosat-2, Jason-2, T&S profiles and SST).
The simulations start from a free model state on October 3, 2012. A 12-month simulation (until 25 September 2013) was carried out with assimilation of SSH, temperature and salinity as presented in Table 1. This period was determined by the availability of the NATL60 data.
3.3 Validation of OSSE method
When setting up an OSSE framework it is important to verify that the impact of assimilating the synthetic observations is similar to that obtained from real observations. Therefore, as an initial step a three-month experiment is performed whereby the standard set of real conventional observations are assimilated (i.e. equivalent to OSSE1 but using real observations). The reduction in innovations statistics for this run as compared to the free run (OSSE0) is then assessed together with differences in innovations statistics between OSSE1 and OSSE0. A similar sensitivity is found for innovations of SLA, SST and temperature and salinity profiles. For example, root-mean-squared (RMS) SLA innovations for Jason 2 were found to decrease from 13.2 cm to 9.4 cm (29% reduction) using real observations and from 13.4 cm to 8.8 cm (34%) using synthetic observations.
4 Impact of assimilating synthetic observations on model fields
4.1 SSH impacts
The objective of this section is to investigate the impacts of assimilating SSH data from SWOT versus two nadir altimeters. Over a one-year period, the RMS difference of SSH between the Nature Run (NR) and the Observing System Simulation Experiments (OSSEs) is calculated and displayed in Figure 2. This figure highlights significant variability in highly dynamic areas such as the Gulf Stream (GS). The impact of assimilating conventional observations (as compared to a free run) can be clearly seen when comparing OSSE1 to OSSE0, with a nearly 40% improvement in domain averaged RMSE (values of 11.38cm and 7.95 cm respectively for OSSE0 and OSSE1). When SWOT is assimilated in place of conventional nadir altimetry (OSSE2) a notable improvement in the Gulf Stream can be seen with a domain-averaged decrease in RMS differences down to a value of 7.08 cm (11% improvement). Among the OSSEs, OSSE3 (assimilating all observations) exhibits the most accurate performance, with the lowest domain-averaged RMSE value of 6.81 cm (14% improvement). Generally, the RMS errors indicate that discrepancies predominantly occur over the GS across all OSSE scenarios. As a reference, the domain-averaged RMS of the Mean Dynamic Topography (MDT) and the RMS of the Sea Level Anomaly (SLA) for OSSE0 are 22 cm and 9.2 cm, respectively, both of which are greater than the RMSE values for OSSE1-3.
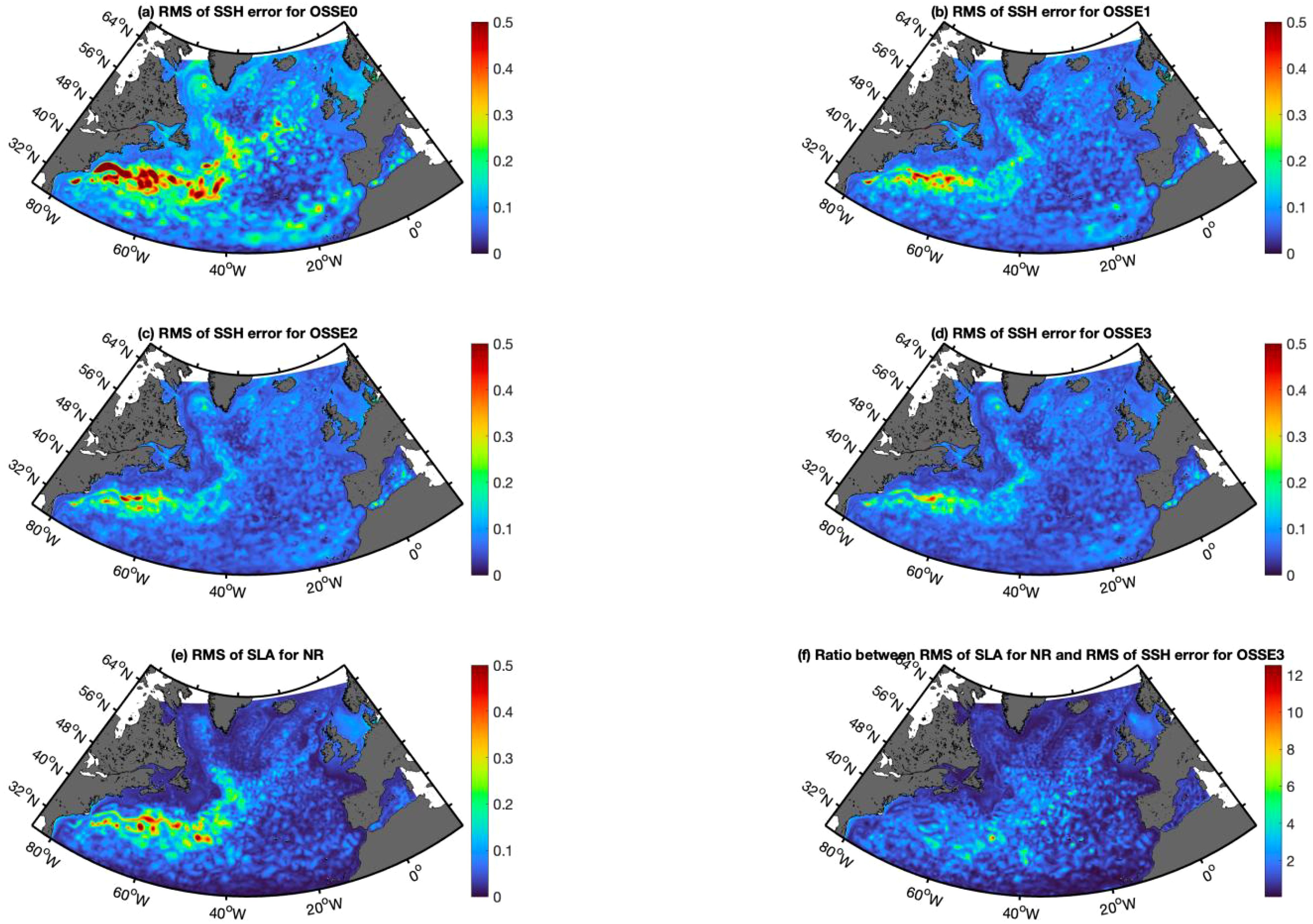
Figure 2. RMS of SSH error comparing the NR and OSSEs: (A) OSSE0 (FREE), (B) OSSE1 (STD), (C) OSSE2 (SWOT), (D) OSSE3(SWOT+NADIR). (E) RMS of the SLA for NR (Unit:m) and (F) the Ratio between RMS of SLA for NR and RMS of SSH error for OSSE3.
To provide context in terms of the amplitude of the errors, it is useful to compare these errors to the amplitude of the variability in the NR itself. Figure 2E presents the RMS of the SLA for NR. We can clearly see that while the error in OSSE0 is much larger than the RMS of the SLA in the NR, for the OSSEs with data assimilation (OSSE1-3) the errors appear similar to, or smaller than that for the RMS SLA of the NR. Figure 2F displays the ratio between the RMS of the SLA for NR and the RMS of the SSH error for OSSE3 (domain-averaged value of 1.1). For most of the Gulf Stream and downstream regions, the ratio is bigger than 1, indicating that the errors are smaller than the variability of the NR.
Figures 3A, B visually demonstrate the effects of data assimilation from SWOT and nadir altimeters on the RMS of SSH errors. Specifically, Figure 3A is dominated by blue hues, particularly over the Gulf Stream and its northern extensions, illustrating significant reductions in RMS errors for OSSE3 compared to OSSE1 (with a domain-averaged difference of 1.14 cm). This indicates that incorporating SWOT data markedly improves accuracy in these dynamically complex regions. There are also some small isolated red areas spread throughout the domain indicating slightly higher errors in OSSE3 relative to OSSE1. But these are likely just noise. Additionally, Figure 3B compares the RMS of SSH errors between OSSE3 and OSSE2, revealing that nadir altimetry has a less significant impact when SWOT data is assimilated (domain-averaged difference of 0.27 cm). Together, these figures underscore the benefits of assimilating SWOT data in improving the precision of SSH predictions, especially in highly dynamic oceanic environments.
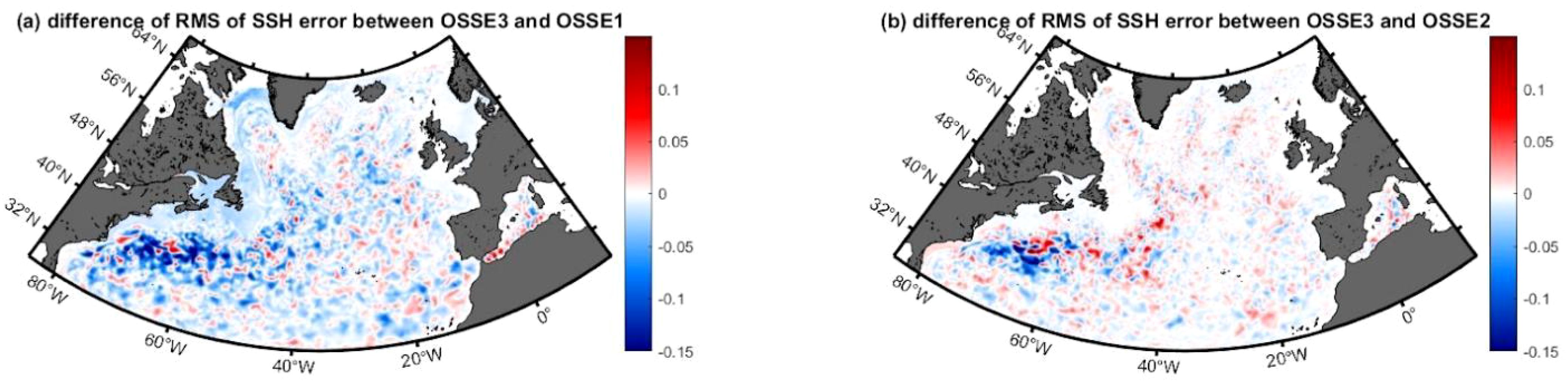
Figure 3. (A) shows the difference of the RMS of SSH error between OSSE3 and OSSE1, (B) difference of the RMS of SSH error between OSSE3 and OSSE2 (Unit:m).
In an operational context it is not possible to compare errors over a full field, rather errors are often assessed in terms of differences with observations. As a result, the “domain-averaged” errors reflect the particular sampling of the observations used. For reference in Section 6 when early-release SWOT data are assimilated in an operational context, it is useful to compare full field statistics provided here with those obtained using observational sampling. As compared to synthetic observations for Cryosat2 only, we obtain RMS differences of 14.33 cm, 8.66 cm, 7.56 cm and 7.34 cm for OSSE0-3 respectively. These values imply an improvement of RMS error for OSSE2 and OSSE3 (as compared to OSSE1) of 13% and 15% respectively. These values are similar to those obtained using the full SSH field noted above (i.e. 11% and 14% respectively). In consequence, we conclude that Cryosat2 sampling provides a similar assessment of the domain-averaged error, allowing a comparison of results between OSSEs and OSEs.
4.2 Impacts on velocity
This section analyzes the impact of SWOT data on representing the upper ocean currents (at 15 meters depth). Considering the significance of ocean currents, it is intriguing to examine how their accuracy improves with the assimilation of SWOT data. The RMSE over 1 year for the amplitude of velocity at 15 m depth (referred to hereafter simply as velocity) between the NR and the OSSE3 is calculated and displayed in Figure 4A. In all the simulations, the highest errors are concentrated around the Gulf Stream and its extension into the North Atlantic, where the dynamical complexity is most pronounced. The domain-averaged RMS velocity errors show that OSSE3 achieves the best accuracy, with an error of 11.1 cm/s. When compared to OSSE1, which has an error of 11.8 cm/s, OSSE3 demonstrates a 6.2% improvement. Finally, compared to OSSE2, which has an error of 11.7 cm/s, OSSE3 shows a 4.9% improvement. These results highlight how OSSE3, with more comprehensive assimilation of SWOT data, enhances the accuracy of upper ocean current representations compared to the other OSSE simulations. Figure 4B shows the difference of the RMS of velocity error between OSSE3 and OSSE0 with a domain-average number of -0.91 cm/s. Additionally, for reference, the RMS of velocity for the Nature Run is shown in Figure 4C with a domain-average number of 17.68 cm/s.
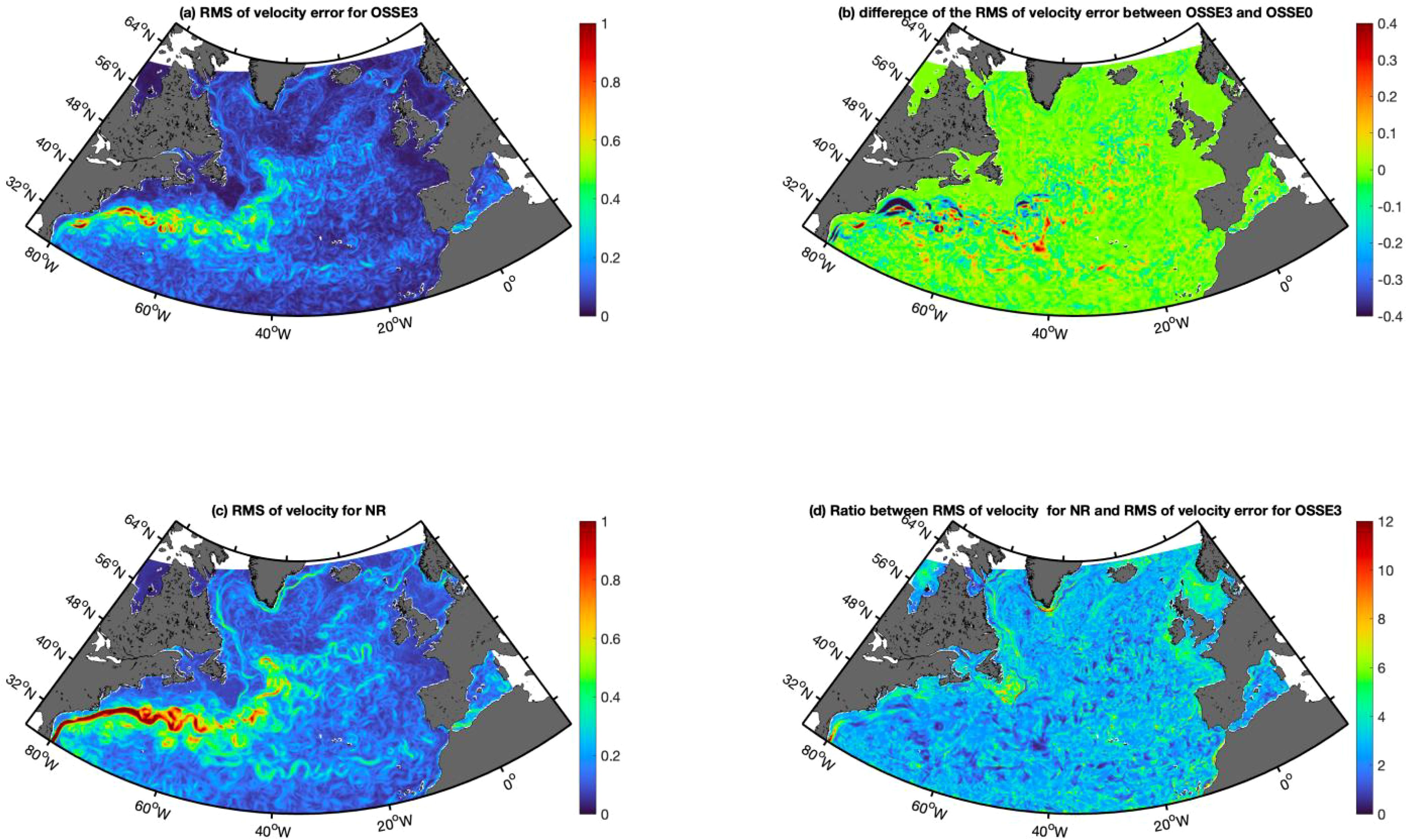
Figure 4. (A) RMS error in the velocity magnitude (15-m depth) comparing the NR and OSSE3. (B) Difference in RMS velocity error between OSSE3 and OSSE0. (C) RMS velocity for the NR (unit: m/s). (D) Ratio of RMS velocity for NR to RMS velocity error for OSSE3.
Figure 4D shows the ratio between the RMS of velocity for NR and the RMS of velocity error for OSSE3. We can see that the RMS of velocity error is less than the RMS of velocity for NR generally with a domain-average number of 1.97. This demonstrates that there is a relatively good accuracy of the velocity field in OSSE3, as the errors are significantly smaller than the actual RMS of velocity for NR, enhancing our confidence in the overall model performance.
As shown, the differences among the OSSEs are shown in Figure 5. OSSE3 exhibits the most accurate performance, with OSSE2 performing marginally better than OSSE1, which can be clearly detected in Figure 5. Here, Figure 5A illustrates the reduction in RMS of velocity error between OSSE3 and OSSE1, indicating that assimilating SWOT data decreases the error compared to scenarios without SWOT data assimilation. Moreover, Figure 5B compares the RMS of SSH error between OSSE3 and OSSE2. This reveals that incorporating data from both altimeters modestly enhances the predictive accuracy. The domain-average of Figure 5A is -0.73 cm/s and -0.56 cm/s for Figure 5B.
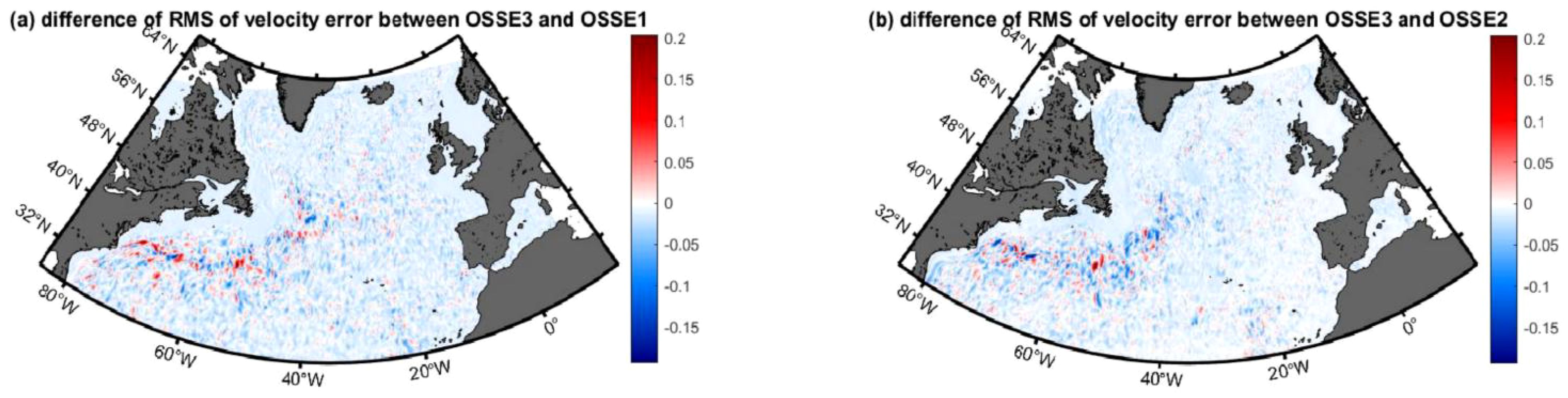
Figure 5. (A) shows the difference of the RMS of velocity error between OSSE3 and OSSE1, (B) difference of the RMS of velocity error between OSSE3 and OSSE2(unit: m/s).
Figure 6 shows the temporal evolution of velocity error variance at a 15-meter depth over the whole year. OSSE0 (dashed black line), which is the free run, shows the highest RMS of SSH error, starting above 200 cm²/s² and gradually decreasing but remaining above 160 cm²/s². All the OSSE1–OSSE3 simulations exhibit similar seasonal variations. OSSE1 (dashed red line) starts with an RMS of SSH error around 180 cm²/s², dropping sharply in the first few weeks, then fluctuating between 120 and 150 cm²/s². OSSE2 (dashed blue line) follows a similar trend to OSSE1 but generally has a lower RMS of SSH error, ranging between approximately 110 and 140 cm²/s². OSSE3 (dashed green line) shows the lowest RMS of SSH error overall, starting around 160 cm²/s² and steadily decreasing, often falling below 100 cm²/s² towards the end of the observation period. The temporal evolution of velocity error variance clearly demonstrates that OSSE3 consistently maintains the lowest error variance, as expected, because it assimilates all available data, including both SWOT and nadir altimeters. While OSSE2 (SWOT-only) shows lower error than OSSE1 (using two nadir altimeters), indicating that if we had to choose between two nadir altimeters and one SWOT, we should choose SWOT. Thus, OSSE3 remains the best scenario overall because it assimilates all data sources.
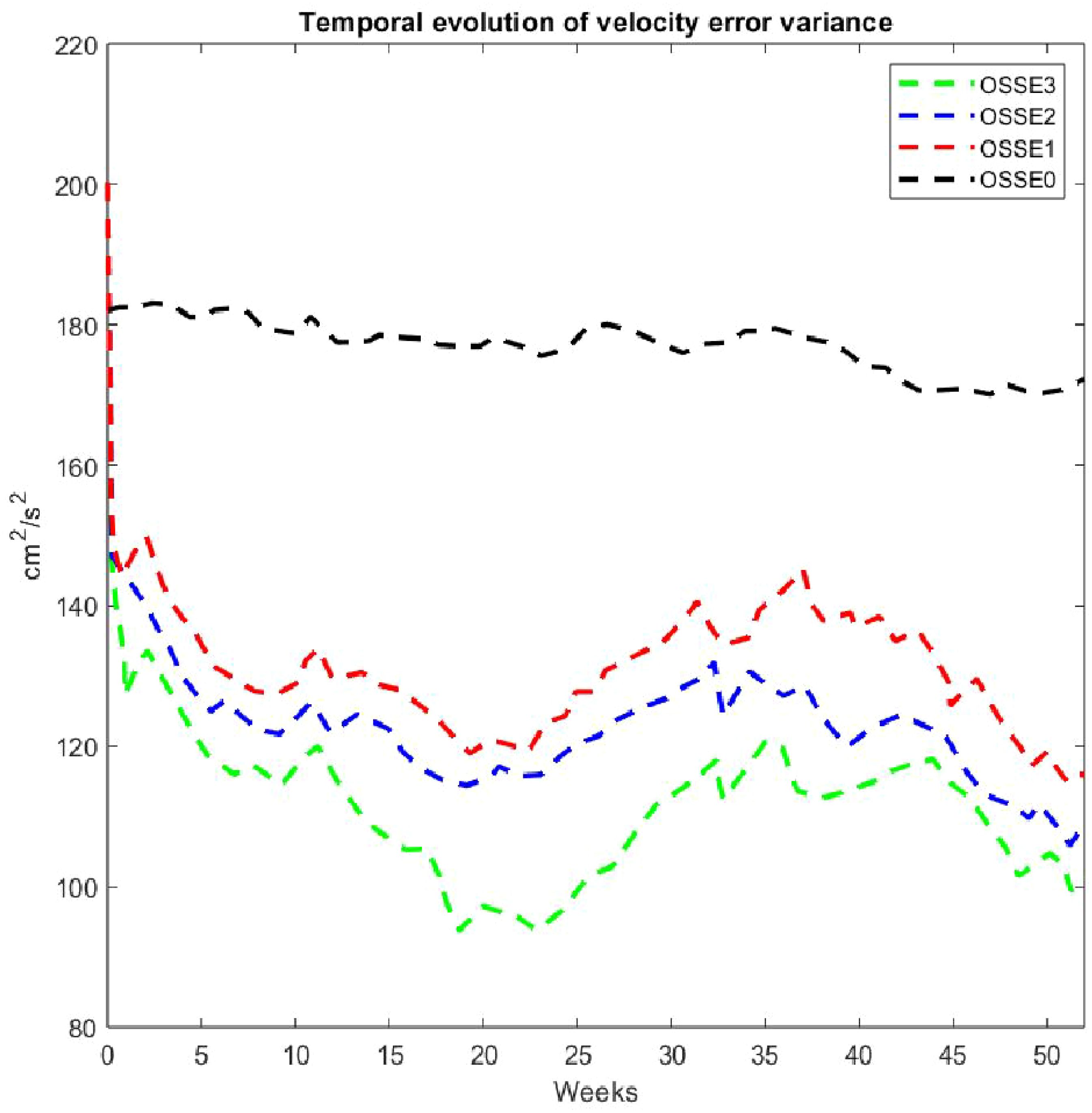
Figure 6. Temporal evolution of velocity error variance (at 15-meter depth) over the Gulf Region. Dashed black is RMS of SSH error for OSSE0 (free run), dashed red is RMS of SSH error for OSSE1, dashed blue is RMS of SSH error for OSSE2, and dashed green is RMS of SSH error for OSSE3.
It is also interesting to note that when the data assimilation is first activated the increments are quite large and despite the IAU a shock occurs in all experiments that results in a net degradation in velocity statistics for the first cycle. Following this initial shock the velocity improves quite quickly over the first few cycles. This improvement is mostly related to the path of the Gulf Stream, and to second order, to the constraint of the data assimilation system on mesoscale features. This analysis is only possible due to the OSSE framework that allows a comparison of full-field velocities. Further analysis regarding this initial shock is underway.
5 Spectral analysis and coherence
In this section, we compute and analyze the wavenumber Power Spectral Density (PSD) and the spatial and temporal coherence for each OSSE simulations in comparison with the Nature Run (NR), specifically over the Gulf Stream, where significant discrepancies are observed. We utilize the wavenumber PSD and spectral coherence to assess the spatial structure of SSH forecast errors in this region. The wavenumber spectra, as detailed by Dufau et al. (2016), are derived from daily zonal SSH error fields spanning the period from October 1, 2012, to September 30, 2013, using a Fast Fourier Transform (FFT). To minimize spectral leakage, a Hanning window was applied to the data. Subsequently, the spectra were averaged both meridionally and temporally. Additionally, to evaluate how the spatial scales of SSH signals are resolved in the different OSSEs, the spectral coherence is calculated, following the methodology of Thomson and Emery (2014). This spectral coherence quantifies the correlation between two signals as a function of wavelength, as described by Ubelmann et al. (2015). Within this study, the spectral coherence between the SSH signals of the OSSEs and the NR is represented as ‘Coh’ and is defined as follows:
where CSD represents the cross-spectral density, SD represents the spectral density and j refers to the j-th OSSE experiment (Equation 1). Both SSH error spectrum and coherence are calculated in the box as shown in Figure 1.
Figure 7 illustrates the SSH error spectrum over the Gulf Stream for the OSSE simulations. All OSSEs exhibit a peak in the error spectrum around the 300 km wavelength. Note that while the specific wavelength at which this peak occurs is sensitive to details of the PSD calculation (e.g. resolution in wavenumber space), a peak value in the range of 200-400 km is consistent with the errors being dominated by mesoscale variability which dominates the SSH variance in the Gulf Stream. As compared to the free run (OSSE0), the assimilation of conventional observations provides an improvement for all scales greater than about 200 km, with a 45% of the peak value. As compared to conventional altimetry (OSSE1), assimilation of SWOT observations provides an additional benefit across these same scales. Furthermore, when SWOT is assimilated together with conventional altimetry (as would be the case if SWOT were to be added to the operational system), the errors are further reduced, with peak values reduced by 33% (OSSE3 compared to OSSE1). However, for wavelengths less than 200 km, it is difficult to discern the impact of the OSSEs on the error spectrum as the errors are dominated by large mesoscale variability. To shed more light on the impact on smaller scales we now assess the spectral coherence.
Figure 8 shows the spectral coherence between the NR and OSSE simulations. Here we consider a value of 0.5 as the threshold for acceptable performance that determines the limit of constrained variability (Ubelmann et al., 2015). First, we can see clearly that the free run (OSSE0) with no data assimilation contains uncorrelated variability with respect to the NR (i.e. coherence values well below 0.5 at all scales). The assimilation of conventional observations (OSSE1) shows high values of coherence demonstrating that the data assimilation system is able to constrain variability, but only for scales above 280 km. When conventional nadir altimetry is replaced by SWOT (OSSE2), the limit of constrained scales is extended to a scale of about 230 km. The synergy of SWOT and nadir together is highlighted by the further benefit seen in OSSE3, with the limit of constrained scales extended to 195 km. While the precise values obtained for the limit of constrained scales is somewhat sensitive to the threshold used, the sensitivity found in the different OSSEs is quite robust down to a threshold of about 0.4. Below this value all OSSEs start to be affected by a strong drop in coherence for scales below about 180 km. This sharp transition is likely due the combined impact of limitations in the data assimilation system and the long (21 day) repeat time of SWOT overpasses.
While these results suggest that assimilation of SWOT observations should help to constrain smaller scales than through assimilation of conventional observations alone, it is not clear if it is due to the inclusion of a wide-swath altimeter (i.e. SWOT), or simply due to an increase in the number of observations assimilated. This question is investigated further in Section 6 using early-release SWOT observations in both a 2 nadir altimeter and 6-nadir altimeter framework.
6 Assimilation of the early-release SWOT data
Following the successful launch of the SWOT satellite mission on 16 December 2022 and a calibration and validation phase, the satellite was put in its nominal science orbit starting on 21 July 2023. An early-release SWOT Level-3 dataset (AVISO/DUACS, 2024) covering the period from 6 September 2023 to 21 November 2023 was made available in December 2023. This version is based on beta pre-validated Level-2 data from NASA/CNES with some known issues from the ground segment. It also has limitations due to the use of the first generation of Level-3 algorithms (See Dibarboure et al., 2024 for a detailed discussion). It nonetheless provides an opportunity to assess whether the impacts found in the OSSEs are robust and can also be detected in this short sample of real-world SWOT data. A Level-3 dataset is required for evaluation in RIOPS as this is what is done for other nadir altimetry data.
In this section we present a series of Observing System Experiments (OSEs) to assess the impact of assimilating early-release SWOT observations with different configurations of altimeters. As shown in Table 2, OSE1 uses the standard operational configuration with six nadir altimeters. OSE2 is designed to be comparable to OSSE1 whereby only two nadir altimeters were available. OSE3 assimilates SWOT together with two altimeters (i.e. equivalent to OSSE3). Finally, OSE4 adds SWOT to the current constellation of 6 altimeters used in operations. Comparison of OSE3 to OSE2 demonstrates the impact of SWOT in the context of a two nadir altimeter constellation, as used for the OSSEs, whereas comparison of OSE4 to OSE1 provides the expected impact of adding SWOT to the current operational RIOPS system. All OSE experiments are initialized from a RIOPS operational analysis on 6 September, 2023 and run until 22 November, 2023 (11 7-day analysis cycles) using the standard operational configuration. An important difference in the OSEs as compared to OSSEs, is that the early-release SWOT observations cover the entire RIOPS domain, whereas the NATL60 simulation used for the NR only covered the North Atlantic Ocean. As a result, the impact of assimilating SWOT can now be assessed over a broader region including the North Atlantic, Arctic and North Pacific Oceans.
In Section 4, it was shown that the assimilation of SWOT in addition to conventional altimetry with two nadir altimeters (OSSE3 compared to OSSE1) provided an improvement of 14% in the RMS errors of SSH as compared to the experiment assimilating conventional observations only. First, we would like to assess whether this improvement is found using the early-release SWOT observations. The differences in RMS innovation statistics for SLA for the different OSEs are presented in Figure 9. Comparison of OSE3 to OSE2 (equivalent to comparison of OSSE3 to OSSE1) shows a significant reduction in innovations throughout the model domain, consistent with the OSSE results presented in Section 4. While a domain-averaged reduction in RMS of 6.2% is found, this is somewhat smaller than found for OSSEs. This may be due to a combination of larger errors in early-release SWOT data and the assessment of impacts over the full domain. Nonetheless, this notable reduction in error suggests that approximations made as part of the OSSE setup were appropriate and that results from the OSSEs may be transferable to real-world applications. Additionally, reductions outside the domain of NATL60 can now be seen as well, with smaller innovations in the Greenland-Iceland-Norwegian Seas, the Beaufort Sea and in the Pacific Ocean sector. Note that there are several small isolated areas of degradation in the highly-energetic Gulf Stream region. These are not statistically significant and may simply be spurious errors due to the short period of the assessment.
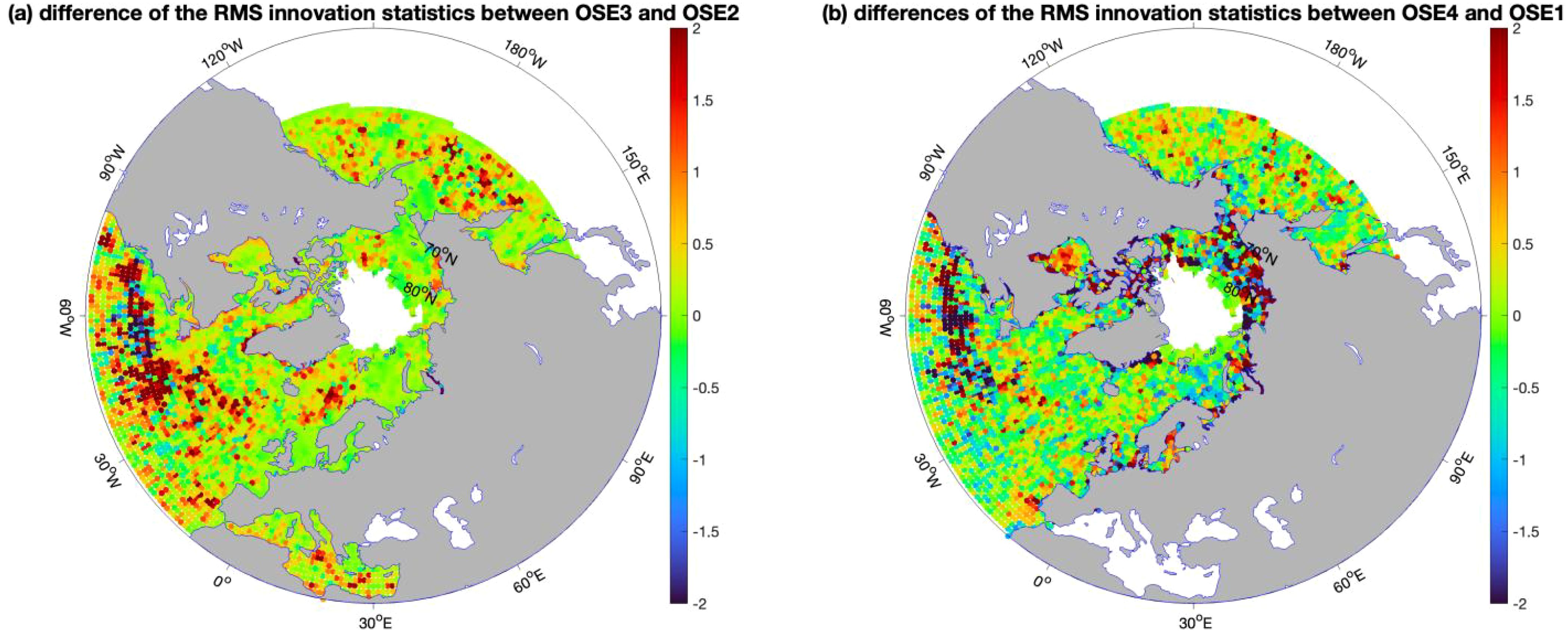
Figure 9. Difference in RMS innovations statistics for experiments assimilating early-release SWOT observations. (A) Shows the difference of the RMS innovation statistics between OSE3 and OSE2. (B) Shows the differences of the RMS innovation statistics between OSE4 and OSE1.
Next, we would like to assess the impact of assimilating SWOT observations in the present operational context using the full constellation of six nadir altimeters. Comparison of RMS SSH innovations for OSE3 to those for OSE2 shows that a less prominent impact of SWOT is found (Figure 9B). Reductions in RMS innovations are still present over many of the same areas but with a reduced amplitude, providing a domain-averaged reduction of RMS SSH innovations of only 2.0%. Moreover, some areas of degradation can be seen in the Gulf Stream extension. As noted above, these may simply be noise due to the short period of assessment or due to use of the early-release SWOT data. Longer experiments using updated processing would be beneficial to investigate this further. Nonetheless, assimilation of the early-release SWOT data provides generally consistent results in the presence of a 2 nadir altimeter constellation and suggests that a small positive improvement may also be found even with 6 nadir altimeters.
7 Conclusions and summary
In this study, we investigate the potential benefits of assimilating wide-swath satellite altimetry from SWOT into an operational ice-ocean prediction system for the North Atlantic Ocean using both synthetic and early-release SWOT data. In particular, we extend previous SWOT OSSEs by incorporating tides into a model configuration, and by using a higher-resolution 1-km resolution Nature Run, NATL60, to capture smaller-scale features and increase overall variance in SSH and velocities. Early-release SWOT observations are also assessed in a set of relatively short 3-month long data withholding experiments.
Our OSSE results confirm earlier findings (e.g. Tchonang et al., 2021), showing improvements in RMS errors in SSH and velocity with the assimilation of SWOT data by 14% and 6% respectively. The SSH error spectra indicate that improvements are most notable around peak errors in the range of 200-400 km, with error reductions of roughly 33% over these scales, compared to the simulations with data assimilation of the traditional data. Additionally, spectral coherence analysis reveals an increase in the limit of constrained scales. In particular, the limit of constrained scale using conventional data only is found to be 280 km. When SWOT is assimilated in place of 2 nadir altimeters this limit is extended by 50km down to a scale of 230 km. Finally, when SWOT is assimilated together with conventional observations (including 2 nadir altimeters) a limit of constrained scales of 195 km is obtained (an improvement of 85 km over conventional data only).
The SWOT mission was anticipated to significantly enhance the resolution of features with wavelengths below 200 km, which nadir altimeters struggle to represent accurately (e.g., Le Traon et al., 2017). Here, the impact of SWOT is found to reduce errors at the predominant wavelength around 300 km and increase the limit of constrained scales. However, a significant impact at smaller wavelengths is not found. This may be due to the manner in which the data assimilation system is configured (e.g. the spectral properties of background error), the relatively long (21 day) repeat coverage of SWOT swaths and the fact that the spectral properties of the region of study are dominated by (larger) mesoscale features. Moreover, it is not clear if the improvements seen when SWOT data are assimilated are due to the nature of wide-swath altimetry itself or simply due to an increase in the overall number of observations.
Early-release SWOT data assimilation also shows positive impacts in OSE experiments, yielding RMS improvements in SSH innovations of 6% across the domain when combined with two nadir altimeters. However, when evaluated alongside six nadir altimeters, the impact of SWOT is significantly reduced (only 2% improvement) but remains mostly positive. This suggests that the improvements found for the OSSEs are due in part to the number of observations rather than the specific use of wide-swath altimetry. Moreover, the smaller impact of SWOT together even in the 2 nadir altimeters context, compared to the impact found in OSSEs in a similar framework may be related to the presence of larger errors in the early-release SWOT data (e.g. the geophysical errors, like wet tropospheric delay correction error or sea state bias). As the OSSE framework was validated with a companion OSE we do not believe the differences are due to assumptions made in the OSSE framework.
Nonetheless, the OSE results presented here confirm that the assimilation of wide-swath altimetry is possible with existing data assimilation systems and can provide tangible improvements. While the short evaluation period makes it challenging to ascertain the statistical significance of these results, they nonetheless affirm the beneficial outcomes observed during OSSEs. This suggests that the positive impacts on near-surface velocity observed in OSSEs may also be present (albeit of a reduced amplitude) when assimilating real observations, holding significant potential for various users. These results highlight the potential benefits of assimilating SWOT observations in RIOPS and similar ocean analysis and forecasting systems. It is noteworthy that early-release data from an experimental mission employing a radically new observing principle can demonstrate improvements at all. This is achieved using an assimilation system not specifically tuned to detect the positive impacts of SWOT, which provides infrequent 2D snapshots of sea level and reveals details not captured by other altimeters except on rare occasions when orbits coincide. Therefore, a deeper analysis of the impact of real SWOT data, using longer time series in future studies, is necessary to further validate these findings. The operational version of RIOPS currently applies errors to SLA observations to account for uncertainty in the MDT as well as representativeness error on the shelf. Future studies aimed at reducing these errors, based on error characterization using real SWOT data, could enhance the impact of SWOT assimilation in coastal areas even further.
Data availability statement
The raw data supporting the conclusions of this article will be made available by the authors, without undue reservation.
Author contributions
GL: Formal analysis, Methodology, Validation, Writing – original draft, Writing – review & editing. GS: Investigation, Project administration, Supervision, Writing – review & editing. A-AG: Validation, Writing – review & editing. CH: Writing – review & editing. WP: Funding acquisition, Project administration, Supervision, Writing – review & editing. MS: Writing – review & editing.
Funding
The author(s) declare financial support was received for the research, authorship, and/or publication of this article. Funding is from Canadian Space Agency (CSA) and new Dalhousie University - led initiative, ‘Transforming Climate Action, TCA’.
Acknowledgments
We would like to thank CSA for financing this project. We would also like to thank D. Surcel Colan for her support. M. Benkiran provided useful suggestions with regards to the setup of the OSSE framework. We would also like to thank colleagues at Mercator Ocean International for their assistance and support of the SAM2 code. SLA and profile data used in this study was provided by the EU Copernicus Marine Environmental Monitoring Service. Argo profile data were collected and made freely available by the International Argo Program and the national programs that contribute to it. (https://argo.ucsd.edu, https://www.ocean-ops.org). The Argo Program is part of the Global Ocean Observing System. The SWOT_L3_LR_SSH product, derived from the L2 SWOT KaRIn low rate ocean data products (NASA/JPL and CNES), is produced and made freely available by AVISO and DUACS teams as part of the DESMOS Science Team project”.
Conflict of interest
The authors declare that the research was conducted in the absence of any commercial or financial relationships that could be construed as a potential conflict of interest.
Publisher’s note
All claims expressed in this article are solely those of the authors and do not necessarily represent those of their affiliated organizations, or those of the publisher, the editors and the reviewers. Any product that may be evaluated in this article, or claim that may be made by its manufacturer, is not guaranteed or endorsed by the publisher.
References
Ajayi A., Le Sommer J., Chassignet E., Molines J., Xu X., Albert A., et al. (2020). Spatial and temporal variability of the North Atlantic eddy field from two kilometric-resolution ocean models. J. Geophysical Research: Oceans 125. doi: 10.1029/2019jc015827
Amores A., Jordà G., Arsouze T., Le Sommer J. (2018). Up to what extent can we characterize ocean eddies using present-day gridded altimetric products. J. Geophysical Research: Oceans 123, 7220–7236. doi: 10.1029/2018JC014140
AVISO/DUACS (2024). The SWOT_L3_LR_SSH product, derived from the L2 SWOT KaRIn low rate ocean data products (NASA/JPL and CNES), is produced and made freely available by AVISO and DUACS teams as part of the DESMOS Science Team project”. SWOT Level-3 KaRIn Low Rate SSH Expert (v1.0) [Data set] (CNES). doi: 10.24400/527896/A01-2023.018
Benkiran M., Greiner E. (2008). Impact of the incremental analysis updates on a real-time system of the North Atlantic Ocean. J. Atmospheric Oceanic Technol. 25, 2055–2073. doi: 10.1175/1520-0493(1996
Benkiran M., Ruggiero G., Greiner E., Le Traon P.-Y., Rémy E., Lellouche J. M., et al. (2021). Assessing the impact of the assimilation of SWOT observations in a global high-resolution analysis and forecasting system. Part 1: method. Front. Mar. Sci. 8. doi: 10.3389/fmars.2021.691955
Bloom S. C., Takacs L. L., Da Silva A. M., Ledvina D. (1996). Data assimilation using incremental analysis updates. Monthly Weather Rev. 124, 1256–1271. doi: 10.1175/1520-0493(1996)1242.0.CO;2
Brasnett B., Colan D. S. (2016). Assimilating retrievals of sea surface temperature from VIIRS and AMSR2. J. Atmospheric Oceanic Technol. 33, 361–375. doi: 10.1175/JTECH-D-15-0093.1
Buckingham C. E., Lucas N. S., Belcher S. E., Rippeth T. P., Grant A. L. M., Lesommer J., et al. (2019). The contribution of surface and submesoscale processes to turbulence in the open ocean surface boundary layer. J. Adv. Modeling Earth Syst. 11, 4066–4094. doi: 10.1029/2019MS001801
Buehner M., Caya A., Carrieres T., Pogson L. (2016). Assimilation of SSMIS and ASCAT data and the replacement of highly uncertain estimates in the Environment Canada Regional Ice Prediction System. Q. J. R. Meteorological Soc. 142, 562–573. doi: 10.1002/qj.2408
Buehner M., Caya A., Pogson L., Carrieres T., Pestieau P. (2013). A new environment Canada regional ice analysis system. Atmosphere-Ocean 51, 18–34. doi: 10.1080/07055900.2012.747171
Cabanes C., Grouazel A., von Schuckmann K., Hamon M., Turpin V., Coatanoan C., et al. (2013). The CORA dataset: validation and diagnostics of in-situ ocean temperature and salinity measurements. Ocean Sci. 9, 1–18. doi: 10.5194/os-9-1-2013
Carrier L., Matthew J., Ngodock H. E., Smith S. R., Souopgui I., Bartels B. (2016). Examining the potential impact of SWOT Observations in an Ocean Analysis–Forecasting System. Monthly Weather Rev. 144, 3767–3782. doi: 10.1175/MWR-D-15-0361.1
Dibarboure G., Anadon C., Briol F., Cadier E., Chevrier R., Delepoulle A., et al (2024). Blending 2D topography images from SWOT into the altimeter constellation with the Level-3 multi-mission DUACS system. EGUsphere 2024, 1–64.
Dibarboure G., Pujol M.-I., Briol F., Le Traon P. Y., Larnicol G., Picot N., et al. (2011). Jason-2 in DUACS: updated system description, first tandem results and impact on processing and products. Mar. Geodesy 34, 2011. doi: 10.1080/01490419.2011.584826
Ducet N., Le Traon P. Y., Reverdin G. (2000). Global high-resolution mapping of ocean circulation from the combination of T/P and ERS-1/2. J. Geophysical Res. 105, 477. doi: 10.1029/2000JC900063
Ducousso N., Sommer J. L., Molines J.-M., Bell M. (2017). Impact of the symmetric instability of the computational kind at mesoscale- and submesoscale-permitting resolutions. Ocean Model. 120, 18–26. doi: 10.1016/j.ocemod.2017.10.006
Dufau C., Orsztynowicz M., Dibarboure G., Morrow R., Le Traon P. Y. (2016). Mesoscale resolution capability of altimetry: present and future. J. Geophysical Research: Oceans 121, 4910–4927. doi: 10.1002/2015JC010904
Dussin R., Barnier B., Brodeau L., Molines J.-M. (2018). The making of the DRAKKAR forcing set DFS5. doi: 10.5281/zenodo.1209243
Esteban-Fernandez D., Fu L.-L., Pollard B., Vaze P. (2017). SWOT project mission performance and error budget JPL D-79084, Caltech. Technical Report, Vol. 117.
Fu L.-L., Alsdorf D., Rodriguez E., Morrow R., Mognard N., Lambin J., et al. (2009). “The SWOT (surface water and ocean topography) mission: spaceborne radar interferometry for oceanographic and hydrological applications,” in Proceedings of the OCEANOBS’09 Conference (Venice-Lido).
Fu L.-L., Ferrari R. (2008). Observing oceanic submesoscale processes from space. Eos Trans. Am. Geophysical Union 89, 488. doi: 10.1029/2008eo480003
Gaultier L., Ubelmann C., Fu L. L. (2016). The challenge of using future SWOT data for oceanic field reconstruction. J. Atmospheric Oceanic Technol. 33, 119–126. doi: 10.1175/JTECH-D-15-0160.1
Halliwell G. R., Srinivasan A., Kourafalou V., Yang H., Willey D., Le Hénaff M., et al. (2014). Rigorous evaluation of a fraternal twin ocean OSSE system for the open Gulf of Mexico. J. Atmospheric Oceanic Technol. 31, 105–130. doi: 10.1175/JTECH-D-13-00011.1
Klein P., Isern-Fontanet J., Lapeyre G., Roullet G., Danioux E., Chapron B., et al. (2009). Diagnosis of vertical velocities in the upper ocean from high resolution sea surface height. Geophysical Res. Lett. 36, 359. doi: 10.1029/2009GL038359
Lellouche J. M., Greiner E., Le Galloudec O., Garric G., Regnier C., Drevillon M., et al. (2018). Recent updates to the Copernicus Marine Service global ocean monitoring and forecasting real-time 1/12° high-resolution system. Ocean Sci. 14, 1093–1126. doi: 10.5194/os-14-1093-2018
Lellouche J. M., Le Galloudec O., Drévillon M., Régnier C., Greiner E., Garric G., et al. (2013). Evaluation of global monitoring and forecasting systems at Mercator Océan. Ocean Sci. 9, 57. doi: 10.5194/os-9-57-2013
Le Traon P. Y. (2013). From satellite altimetry to Argo and operational oceanography: three revolutions in oceanography. Ocean Sci. 9, 901–915. doi: 10.5194/os-9-901-2013
Le Traon P. Y., Dibarboure G., Jacobs G., Martin M., Remy E., Schiller A. (2017). “Use of satellite altimetry for operational oceanography,” in Satellite Altimetry Over Oceans and Land Surfaces. Eds. Stammer, Cazenave (CRC Press, Boca Raton, FL).
Madec G., Bourdallé-Badie R., Chanut J., Clementi E., Coward A., Ethé C., et al. (2019). “NEMO ocean engine,” in Notes du Pôle de modélisation de l’Institut Pierre-Simon Laplace (IPSL) (v4.0, Number 27) (Zenodo, Paris, France). doi: 10.5281/zenodo.3878122
Masina S., Storto A., Ferry N., Valdivieso M., Haines K., Balmaseda M., et al. (2017). An ensemble of eddy-permitting global ocean reanalyses from the MyOcean project. Climate Dynamics 49, 813–841. doi: 10.1007/s00382-015-2728-5
Pascual A., Faugère Y., Larnicol G., Le Traon P. Y. (2006). Improved description of the ocean mesoscale variability by combining four satellite altimeters. Geophysical Res. Lett. 33. doi: 10.1029/2005GL024633
Pujol M. I., Dibarboure G., Le Traon P.-Y., Klein P. (2012). Using high-resolution altimetry to observe mesoscale signals. J. Atmospheric Oceanic Technol. 29, 1409–1416. doi: 10.1175/jtech-d-12-00032.1
Qiu B., Shuiming C., Klein P., Ubelmann C., Fu L. L., Hideharu S. (2016). Reconstructability of three-dimensional upper-ocean circulation from SWOT sea surface height measurements. J. Phys. Oceanography 46, 947–963. doi: 10.1175/JPO-D-15-0188.1
Rio M.-H., Mulet S., Picot N. (2014). Beyond GOCE for the ocean circulation estimate: Synergetic use of altimetry, gravimetry, and in situ data provides new insight into geostrophic and Ekman currents. Geophys. Res. Lett. 41, 8918–8925. doi: 10.1002/2014GL061773
Smith G. C., Hébert-Pinard C., Gauthier A. A., Roy F., Peterson K. A., Veillard P., et al. (2024). Impact of assimilation of absolute dynamic topography on Arctic Ocean circulation. Front. Mar. Sci. 11, 1390781. doi: 10.3389/fmars.2024.1390781
Smith G. C., Liu Y., Benkiran M., Chikhar K., Surcel Colan D., Gauthier A. A., et al. (2021). The Regional Ice Ocean Prediction System v2: a pan-Canadian ocean analysis system using an online tidal harmonic analysis. Geoscientific Model. Dev. 14, 1445–1467. doi: 10.5194/gmd-14-1445-2021
Smith G. C., Roy F., Reszka M., Surcel Colan D., He Z., Deacu D., et al. (2016). Sea ice forecast verification in the Canadian global ice ocean prediction system. Q. J. R. Meteorological Soc. 142, 659–671. doi: 10.1002/qj.2555
Souopgui I., D’Addezio J. M., Rowley C. D., Smith S. R., Jacobs G. A., Helber R. W., et al. (2020). Multi-scale assimilation of simulated SWOT observations. Ocean Model. 154, 101683. doi: 10.1016/j.ocemod.2020.101683
Tchonang B. C., Benkiran M., Le Traon P.-Y., Jan van Gennip S., Lellouche J. M., Ruggiero G. (2021). Assessing the impact of the assimilation of SWOT observations in a global high-resolution analysis and forecasting system – part 2: results. Front. Mar. Sci. 8. doi: 10.3389/fmars.2021.687414
Thomson R. E., Emery W. J. (2014). “Chapter 5–Time series analysis methods,” in Data Analysis Methods in Physical Oceanography, 3rd Edn, eds Thomson R. E., Emery W. J. (Boston, MA: Elsevier), 425–591. doi: 10.1016/B978-0-12-387782-6.00005-3
Ubelmann C., Klein P., Fu L. L. (2015). Dynamic interpolation of sea surface height and potential applications for future high-resolution altimetry mapping. J. Atmos. Ocean. Technol. 32, 177–184. doi: 10.1175/JTECH-D-14-00152.1
Wong A. P., Wijffels S. E., Riser S. C., Pouliquen S., Hosoda S., Roemmich D., et al. (2020). Argo data 1999–2019: two million temperature-salinity profiles and subsurface velocity observations from a global array of profiling floats. Front. Mar. Sci. 7. doi: 10.3389/fmars.2020.00700
Keywords: SWOT, North Atlantic modeling, data assimilation, OSSE experiments, ocean dynamic
Citation: Liu G, Smith GC, Gauthier A-A, Hébert-Pinard C, Perrie W and Shehhi MRA (2024) Assimilation of synthetic and real SWOT observations for the North Atlantic Ocean and Canadian east coast using the regional ice ocean prediction system. Front. Mar. Sci. 11:1456205. doi: 10.3389/fmars.2024.1456205
Received: 28 June 2024; Accepted: 10 October 2024;
Published: 12 November 2024.
Edited by:
Peter R. Oke, Oceans and Atmosphere (CSIRO), AustraliaReviewed by:
David Griffin, Commonwealth Scientific and Industrial Research Organisation (CSIRO), AustraliaDaniel James Lea, Met Office, United Kingdom
Santha Akella, National Oceanic and Atmospheric Administration (NOAA), United States
Copyright © 2024 Liu, Smith, Gauthier, Hébert-Pinard, Perrie and Shehhi. This is an open-access article distributed under the terms of the Creative Commons Attribution License (CC BY). The use, distribution or reproduction in other forums is permitted, provided the original author(s) and the copyright owner(s) are credited and that the original publication in this journal is cited, in accordance with accepted academic practice. No use, distribution or reproduction is permitted which does not comply with these terms.
*Correspondence: Guoqiang Liu, Z29xYW5nbEBnbWFpbC5jb20=