- 1State Key Laboratory of Biogeology and Environmental Geology, China University of Geosciences (Wuhan), Wuhan, China
- 2Southern Marine Science and Engineering Guangdong Laboratory (Zhuhai), Zhuhai, China
- 3Institute for Advanced Marine Research, China University of Geosciences, Guangzhou, China
- 4College of Marine Science and Technology, China University of Geosciences (Wuhan), Wuhan, China
Eukaryotic microorganisms were the key components of marine microecosystems and were involved in biogeochemical cycling processes. Although oceanographers have emphasized their importance in open oceans, the current understanding was not comprehensive enough about the assembly processes and co-occurrence network of different fraction eukaryotic microbial communities in the coastal China sea. We used co–occurrence networks and ecological process model to investigate possible ecological interactions in two fractions (small fraction 0.22–3 μm; large fraction 3–200 μm) of eukaryotes throughout different depths and geographical regions of coastal China sea. We found that environmental distance shapes the diversity of the two fractions eukaryotic microorganisms. Dispersal limitation and homogeneous selection processes were the key drivers of eukaryotic microbial community assembly. Co-occurrence network analysis showed that the networks of the 0.2–3 μm eukaryotes more nodes and edges, with more complex interactions between microorganisms, and higher network stability than that of 3–200 μm eukaryotic microorganisms. Temperature, oxygen, salinity, and nutrients play the crucial role in the abundance of two fractions eukaryotic microorganisms. These results crystallize the knowledge of eukaryotic microbes in the coastal China sea, which was essential for addressing the challenges posed by global change.
1 Introduction
Coastal ecosystems assume the dynamic intersection of land, water and atmosphere, and were often subject to disturbances from global climate change and anthropogenic activities (Bonaldo et al., 2023). In addition, coastal environments are inherently more vulnerable than open ocean ecosystems due to their shallower depths and lower water volumes, making them less resistant to environmental changes and anthropogenic damage (Cavalcante et al., 2021). As anthropogenic climate change intensifies, changes in coastal ecosystems were reflected in the rapid response of microbial communities (Dubey et al., 2021; Zhang et al., 2023). Due to the dense distribution of eukaryotic microbial communities in the environment and their high sensitivity to pollutants, their response to environmental changes serves as an indicator for assessing the environmental quality of aquatic ecosystems (Liu et al., 2022). Moreover, eukaryotic microorganisms were involved in driving coastal biogeochemical cycles, mainly in nutrient cycling and pollutant degradation (Wang et al., 2022). Therefore, conducting comprehensive studies of eukaryotic microorganisms contributes to our understanding of microbial ecosystem integrity and functionality. Moreover, it lays the foundation for a deeper understanding and development of predictive models that can conveniently characterize microbial feedback to environmental changes (Mitra et al., 2014).
Ecological networks have shown effectiveness in exploring interspecific symbiotic patterns of environmental microorganisms (Lima–Mendez et al., 2015; Zhou et al., 2021). Previous studies using co–occurrence networks have shown that community stability was primarily driven through microbial interspecific interactions (De Vries et al., 2018; Magurran, 2007). The topological features and complexity of co–occurring networks reflected ecological stability, and stability indicated microbial resistance to environmental climate change and resilience from disturbance (Pärtel et al., 2001; Supp et al., 2015). In addition, the diversity and distribution characteristics of microbial communities were driven by ecological processes, and the investigation of community assembly mechanisms was essential in the field of ecology (Zhou and Ning, 2017; Lin et al., 2024). Within the context of ecological niche theory, stochastic and deterministic processes combined shape the assembly mechanisms of microbial communities. Stochastic processes default to a uniform ecological probability for all species, and stochastic processes such as birth/death, formation/extinction, and migration dominate community construction (Zhou and Zhang, 2008). In contrast, the deterministic process focuses on environmental screening and interspecific interactions (Chesson, 2000). Combined control of stochastic and deterministic processes was the key to shaping marine eukaryotic communities in most of the world oceans (Berdjeb et al., 2018; Mo et al., 2021). Previous work has found that different ecological processes shape the eukaryotic microbial communities in the ocean (Logares et al., 2020) and generate biogeographical differences (Villarino et al., 2022). Such as selection processes that affect community similarity (homogeneous selection) or dissimilarity (heterogeneous selection). The dispersal process affects the homogenization of the community (homogenization diffusion) or increases stochastic changes (diffusion limitation) (Vellend 2010; Stegen et al., 2015). Although there was some understanding of the biogeographic patterns of marine eukaryotic microbial communities, there was still a lack of comprehensive understanding of the ecological processes that drive the aggregation and biogeography of coastal eukaryotic microbial communities, considering environmental heterogeneity, potential diffusion barriers, and geographical factors.
Eukaryotic microbial communities of various sizes tend to differ in species composition and ecological roles, so ecological correlation analysis can be accomplished more specifically based on size classes (Massana and Logares, 2013). Eukaryotic microorganisms of different sizes may represent different lifestyles and assemblages and play different roles in food webs and biogeochemical processes. Smaller eukaryotes may be more efficient at nutrient acquisition and light trapping than larger cells in the same phylum and are more likely to enter aquatic food webs through protist grazing (Stockner, 1988). In addition, size fraction allows for improved discrimination between the smaller and larger microbial eukaryotes and a more detailed analysis of eukaryotes community structure and its response to environmental gradients (Sun et al., 2023). Previous studies mostly analyzed eukaryotic microbial communities through a single filtered sampling, but could not determine the differences between eukaryotic microorganisms of different sizes. In this study, we focused on two fractions to investigate the assembly mechanism and co–occurrence network of eukaryotic microbial, which was crucial to fully recognize the stability of coastal marine ecosystems. We propose to address the following scientific questions: (1) The distribution of coastal eukaryotic microbial diversity in depth and space; (2) The co–occurrence network of eukaryotic microorganisms with two fractions; (3) Factors dominating of eukaryotic microbial community assembly mechanism. Our research findings provide assistance in the biological complexity of global carbon cycling and data support for climate modeling (Guidi et al., 2016).
2 Materials and methods
2.1 Study area and sample collection
Samples were collected from the coastal China sea (112° E–124° E, 21° N–33° N) on a cruise aboard the R/V “Yanping 2” during the summer of 2023. We collected 112 seawater samples from the surface and bottom layers at 30 stations using a conductivity–temperature–depth rosette system (4 stations were collected in the surface layer only). We established sampling stations based on offshore distance and hydrological parameter gradients of seawater. Specifically, there were 11 sampling sites were collected in the Yangtze River Estuary (121° E–124° E, 29° N–33° N), with 11 samples from the surface layer and 9 samples from the bottom layer; and 19 sampling sites were collected in the Pearl River Estuary (112° E–115° E, 21° N–23° N), with 19 samples from the surface layer and 17 samples from the bottom layer. At least 4 L of seawater was sequentially filtered using 47 mm filters of 3 μm to 0.22 μm filters (Millipore), frozen and stored in liquid nitrogen on board the ship, and stored at −80°C until DNA extraction. Nutrient samples were collected from seawater using 100 ml PE bottles, and using copper–cadmium column reduction methods, the indophenol blue method, silico–molybdate complex methods, and phosphormolybdate complex methods for the nitrate, nitrite, ammonium, respectively (Hansen and Koroleff, 1999).
2.2 DNA extraction and sequencing
DNA extraction was performed using the DNeasy PowerWater® kit (Qiagen, Hilden, Germany). DNA was subjected to PCR amplification by targeting a ~380-bp fragment of the 18S rRNA gene variable region V4 using the specific primer set TAReuk454FWD1 (5’-CCAGCASCYGCGGTAATTCC-3’) and TAReukREV3_modified (5’-ACTTTCGTTCTTGATYRATGA-3’) with overhang Illumina adapters (Stoeck et al., 2010; Piredda et al., 2017). The PCR reactions included initial denaturation at 94°C for 5 min, followed by 26 cycles of 30 s at 94°C, 56°C for 30 s, and 72°C for 20 s. At the end of the amplification, the amplicons were subjected to a final 5 min extension at 72°C. Sequencing was performed on an Illumina NovaSeq6000 with two paired end read cycles of 250 bases each (Magigene, China).
Paired-end reads were preprocessed using Trimmomatic software to detect and cut off ambiguous bases(N) (Bolger et al., 2014). It also cut off low quality sequences with average quality score below 20 using sliding window trimming approach. After trimming, paired-end reads were assembled using FLASH software (Reyon et al., 2012). Parameters of assembly were: 10 bp of minimal overlapping, 200 bp of maximum overlapping and 20% of maximum mismatch rate. Sequences were performed further denoising as follows: reads with ambiguous, homologous sequences or below 200 bp were abandoned. Reads with 75% of bases above Q20 were retained using QIIME software (version 1.8.0) (Caporaso et al., 2010). Then, reads with chimera were detected and removed using VSEARCH (Rognes et al., 2016). The singletons have been removed and then the tags were clustered into ASVs at a 99% threshold by QIIME2 (version 2022.2) (Bolyen et al., 2019). After removing chimeric sequences, 18s ASVs were taxonomically assigned with the Protist Ribosomal Reference database [PR2, version 5.0.0] (Guillou et al., 2013). The clean tags were mapped to ASVs representative sequences to obtain the ASVs and species abundance profiles. Afterward, all metazoans were discarded before the downstream analyses. The original sequence has been saved in the NCBI database (BioProject: PRJNA1110384).
2.3 Bioinformatic and statistical methods
All statistical analyses were performed in R software (V4.2.2). Alpha diversity, NMDS (non–metric multidimensional scaling), and ANOSIM (similarity analysis) were implemented using the “vegan” software package (Oksanen et al., 2019). Community distance-decay analyses were implemented using the “vegan” and “geosphere” packages, and the “gbmplus” package with 500 trees was used to implement ABT (aggregated boosted tree) analyses (De’ath, 2007). The βNTI (beta nearest–taxon–index) was implemented using the “iCAMP” function of the picante package (Kembel et al., 2010; Stegen et al., 2015). The fit of the neutral community model was calculated using the “Hmisc” package. Co–occurrence models were constructed based on SparCC and visualized in Gephi (version 0.9). The details of the method were in the supplementary.
3 Results
3.1 The distribution and alpha diversity of eukaryotes
The sampling stations were divided into two regions on the map (Yangtze Eatuary and Pearl River Estuary) in the coastal China sea (Figure 1A). The average surface temperature was 26.66 °C, and the average surface salinity was 31.05 in the surveyed sea region (Figure 1B). In the surface layer, the Yangtze Eatuary has relatively high averages of fluorescence, oxygen and nutrients, while the Pearl River Estuary has relatively high temperature and salinity. At the bottom, the Yangtze Eatuary has higher salinity, silicate and nitrate, while the Pearl River Estuary has relatively high temperature, fluorescence, oxygen, nitrite and ammonium (Supplementary Table S1). Surface fluorescence (p < 0.05) was significantly different in two regions; and bottom temperature, oxygen, silicate, and nitrate (p < 0.05) were significantly different in two regions. Other environmental parameters were not significantly different between the two regions (p > 0.05) (Supplementary Figure S1). The composition of eukaryotic microorganisms varied across stations and depths in the survey area. Overall, the reads of eukaryotes were dominated by Alveolata (63.72%), Stramenopiles (15.85%), and Chlorophyta (14.73%) in the surveyed sea region. In the 0.2–3 μm fractions, the reads of eukaryotes were dominated by Alveolata (64.47%), Stramenopiles (13.90%), and Chlorophyta (14.78%). And in the 3–200 μm fractions, the reads of eukaryotes were dominated by Alveolata (62.86%), Stramenopiles (18.11%), and Chlorophyta (14.68%) (Figure 1C).
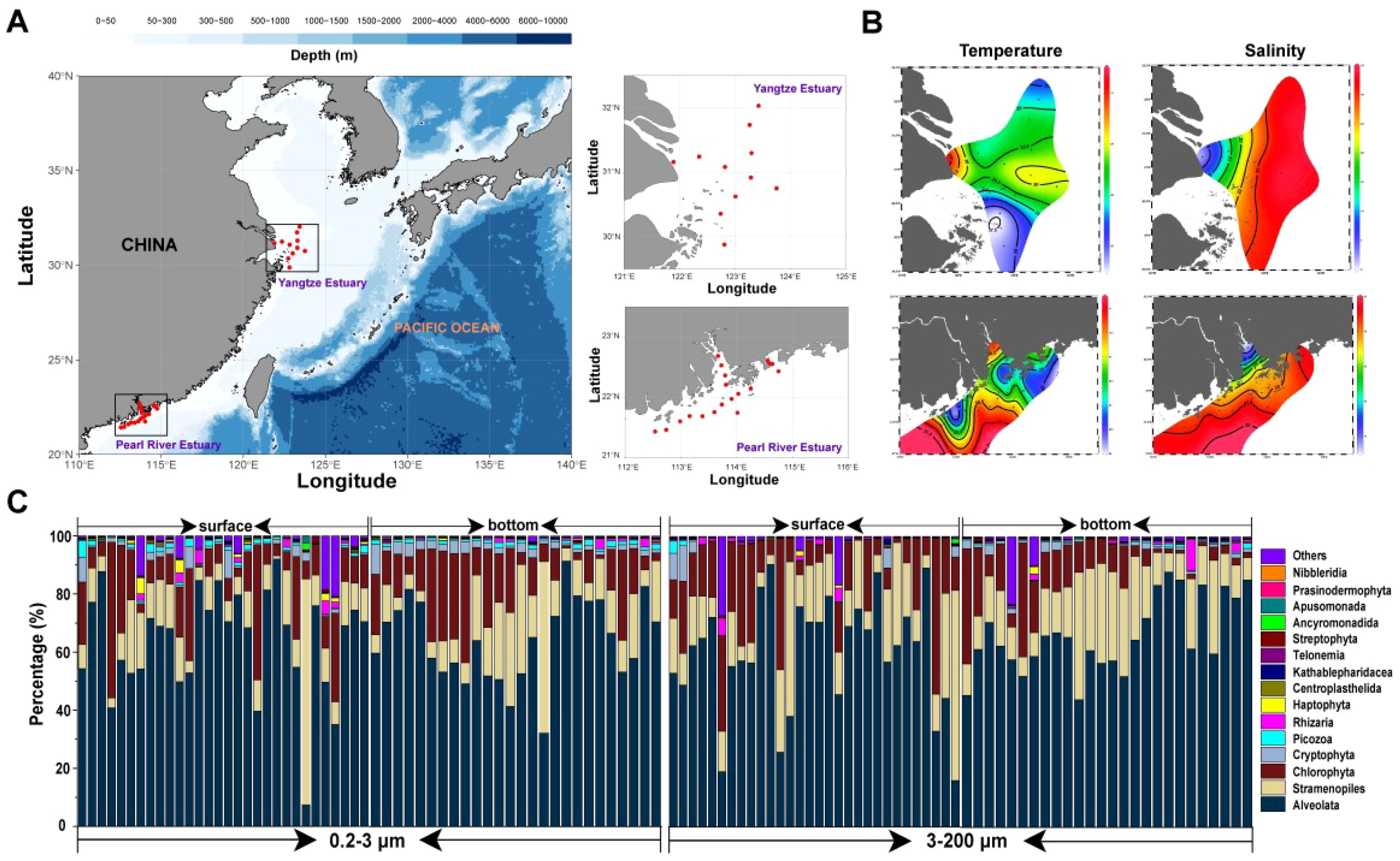
Figure 1. (A) The sampling locations in the coastal China sea; (B) Horizontal distribution of temperature (°C), salinity of surface waters; (C) Composition of eukaryotic microbial communities of two fractions (0.2–3 μm, 3–200 μm).
We further subdivided the three major eukaryotic microbial, the results showed that the composition was different with the two fractions (Supplementary Figure S2). Firstly, in the Alveolata, the 0.2–3 µm eukaryotic microorganisms were mainly Syndiniales (79.35%) and Dinophyceae (15.24%); the 3–200 µm eukaryotic microorganisms were mainly Syndiniales (29.41%) and Dinophyceae (65.88%). Secondly, in the Stramenopiles, eukaryotic microorganisms of 0.2–3 µm were dominated by Bicoecea (41.58%), Sagenista (15.64%) and Opalozoa (10.83%); eukaryotic microorganisms of 3–200 µm were dominated by Mediophyceae (48.12%) and Peronosporomycetes (21.01%). Thirdly, in the Chlorophyta, Mamiellophyceae (74.56%) dominated among eukaryotic microorganisms of 0.2–3 μm; the 3–200 μm eukaryotic microorganisms were mainly Chloropicophyceae (24.49%), Mamiellophyceae (16.54%) and Trebouxiophyceae (16.10%).
Four diversity indexes (Richness, Shannon, Simpson and Pielou) were used to differences between Deukaryotic microbial communities (Figure 2). Richness’ results were not significant (p > 0.05) in the depth and region but differed significantly between the two fractions (p < 0.05). The Shannon and Pielou’s results differed significantly in the depth and size. Simpson’s diversity index only differed significantly between the depth. The diversity indexes of invsimpson, Chao1, ACE showed the same results (Supplementary Figure S3). Thus, we found that the differences between regions were not significant (p > 0.05), there were significant differences between depths (p < 0.05), and the differences between sizes were extremely significant (p < 0.01, except Simpson and invsimpson) (Figure 2; Supplementary Figure S3). Volcano plots showing the enriched between the cell-sizes reached 385, and the depleted reached 226 (Figure 2).
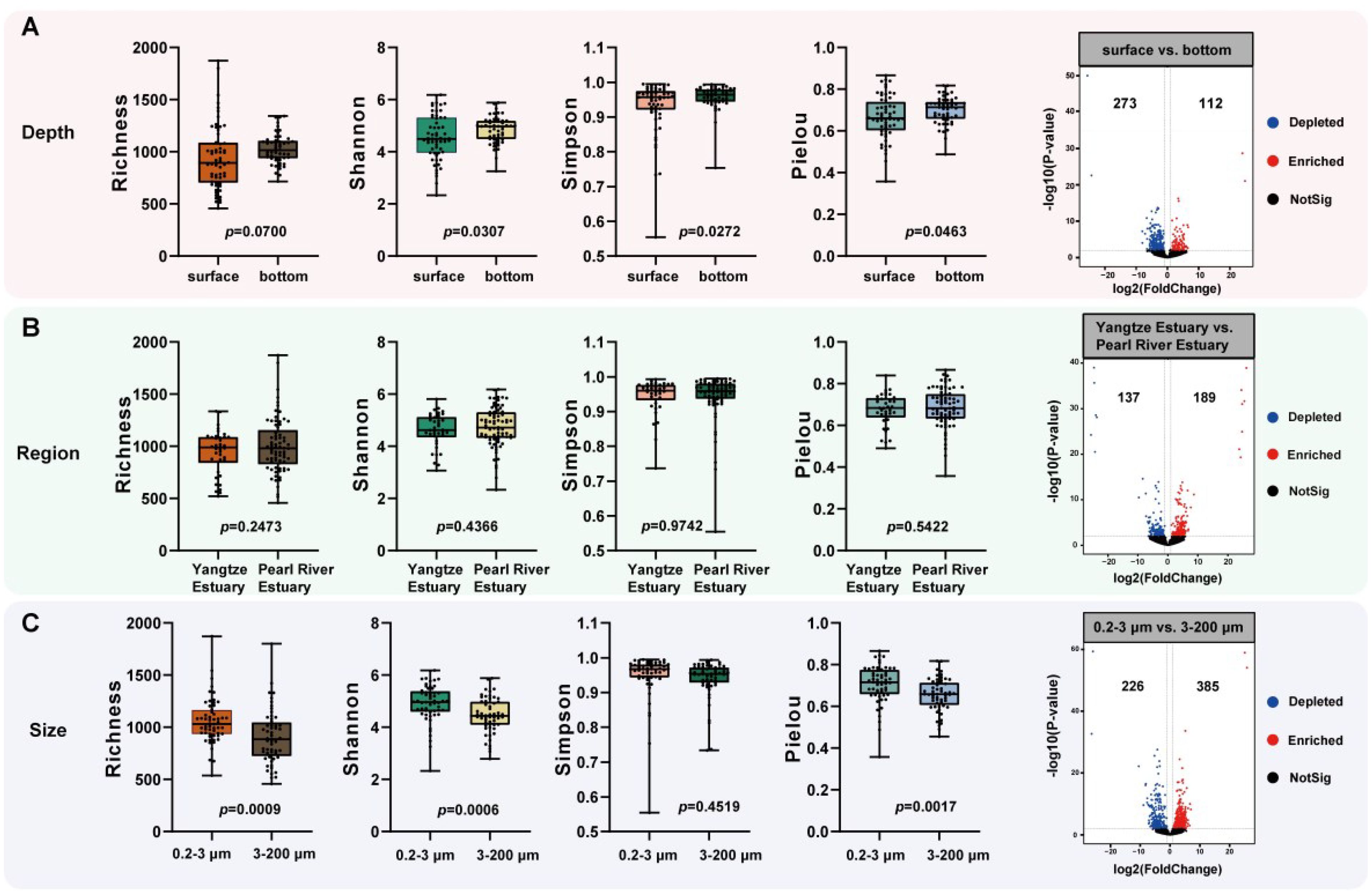
Figure 2. Alpha diversity and Volcano plots of eukaryotic microbial communities between the groups. (A) Depth; (B) Region; (C) Size. The p value indicates the statistical significance.
3.2 Beta diversity and distance decay patterns of eukaryotes
In order to explore the if there was a difference of community composition in the groups, used the NMDS plot and ADONIS analysis based on Bray–Curtis distance (Figure 3; Supplementary Figure S4). Community composition differed significantly in the depth (R = 0.156, p = 0.001), region (R = 0.168, p = 0.001) and size (R = 0.556, p = 0.001). Subsequently, biogeographic analyses helped us in order to explore potential influences on eukaryotic microbial community similarity. The geographical distance results showed significant differences between eukaryotic microorganisms of different depths and sizes (p < 0.001). Eukaryotic microorganisms differed even more significantly at environmental distances, presenting highly significant differential results (p < 0.001) in all three delineation criteria (depth, region and size). In contrast, the Bray–Curtis similarity of eukaryotic microbial communities all showed greater correlation values with environmental distance than their correlation values with geographic distance. This suggests that eukaryotic microbial communities were more influenced by environmental factors.
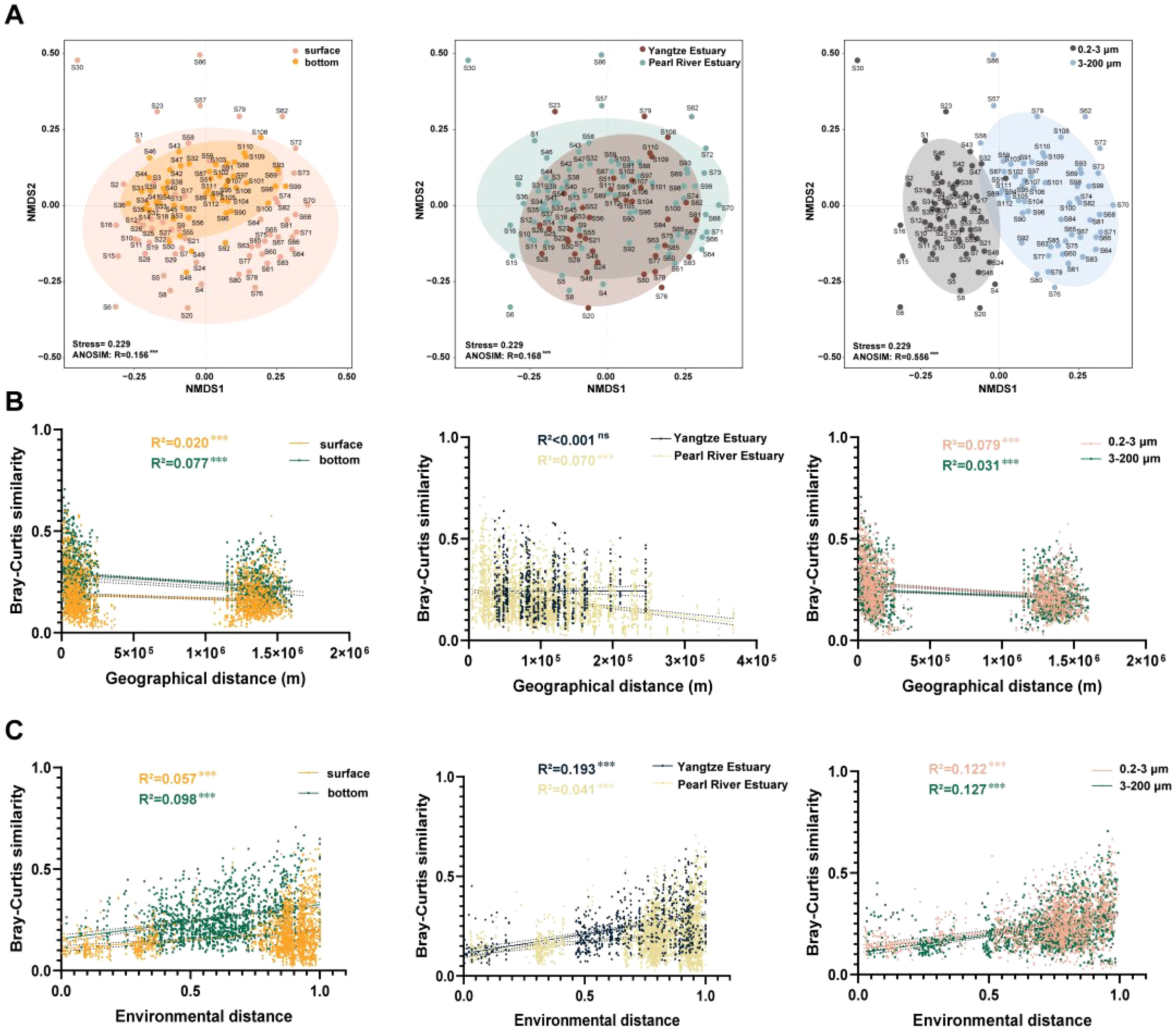
Figure 3. Beta diversity and distance decay patterns of eukaryotes of eukaryotic microbial communities. (A) NMDS and ANOSIM of eukaryotic microbial communities in the groups; (B) Correlation of Bray–Curtis similarity with geographical distance; (C) Correlation of Bray–Curtis similarity with environmental distance. R2 represents the fit to this model, as well as the significance level (***: p < 0.001; ns: no significance). Shaded regions indicate the 95% confidence interval.
3.3 Correlation of different sizes eukaryotic communities with environmental factors
In order to explore the correlation of eukaryotic microbial communities with environmental factors, disentangling the drivers of microbial community similarity and environmental distance decay with the Mantel test. The results revealed the 0.2–3 μm eukaryotic microorganisms showed a significant correlation with salinity, density, and silicate (r > 0.1, p < 0.05), while the 3–200 μm eukaryotic microorganisms showed a significant correlation with depth, temperature, and oxygen (r > 0.3, p < 0.001) (Figure 4A; Supplementary Table S2). The effect of environmental factors on the ordination of eukaryotic abundance in the investigated waters was assessed with the help of ABT. The results showed that the key determinants of the 0.2–3 μm eukaryotic microorganisms’ richness were fluorescence, nitrite, ammonium, latitude and salinity. And fluorescence, oxygen, latitude, density and longitude were playing the key role in the 3–200 μm eukaryotic microorganisms’ richness (Figure 4B).
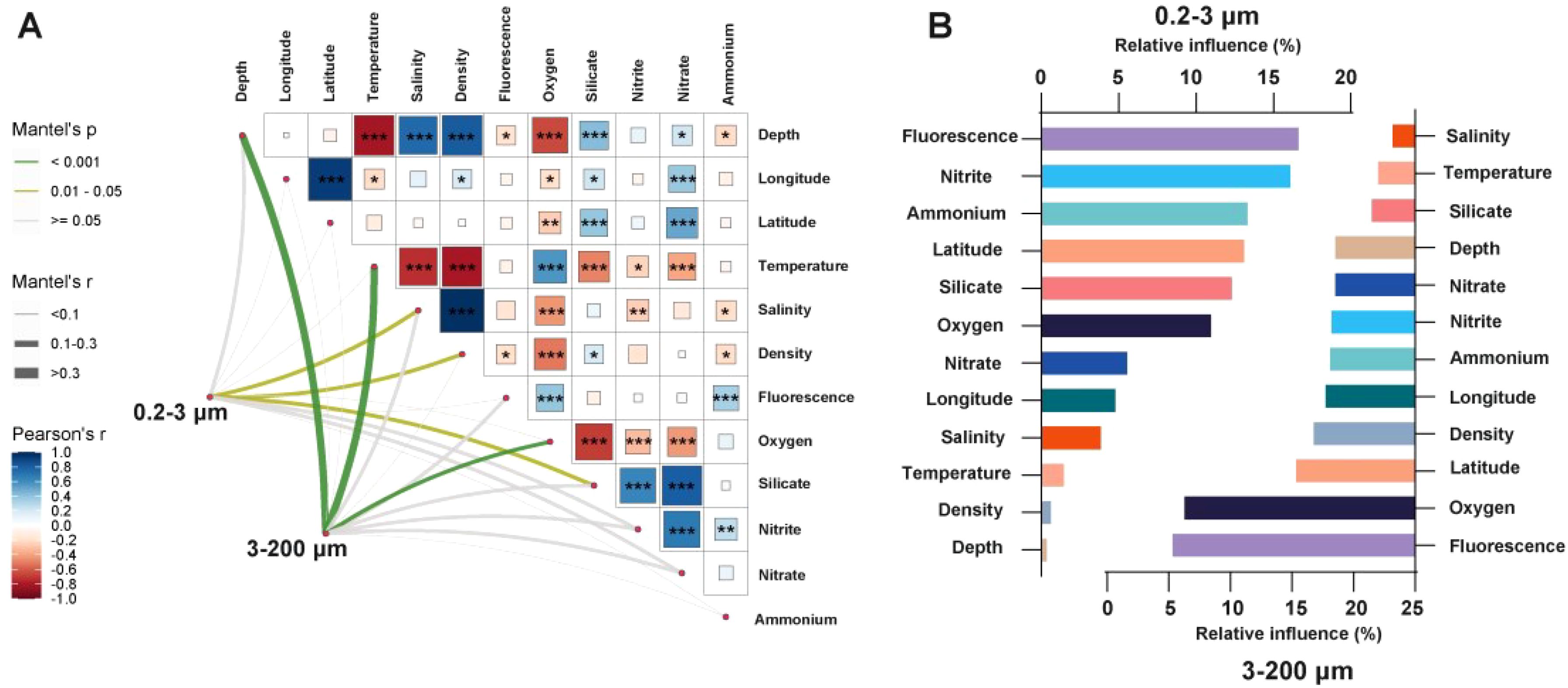
Figure 4. Correlation of eukaryotic microbial communities with environmental factors. (A) The mantel tests for eukaryotic microbial communities and environmental factors for two fractions; (B) ABT analyzed the relative influence of environmental factors on eukaryotes’ richness for two fractions. (***: p < 0.001; **: p < 0.01; *: p < 0.05).
3.4 Co–occurrence network patterns of eukaryotes
We explore the interaction among eukaryotic microorganisms based on co–occurrence networks. The result indicates that the networks in the 0.2–3 μm eukaryotic microorganisms exhibit more nodes and edges than the 3–200 μm eukaryotic microorganisms, suggesting more complex interactions among 0.2–3 μm eukaryotic microorganisms compared to the networks in 3–200 μm eukaryotic microorganisms (Figure 5A). In addition, we compared the network stability of two fractions eukaryotic microorganisms and tested their resistance to environmental interference by randomly removing nodes to test the magnitude of natural connectivity changes (Mo et al., 2021). According to our research results, it was found that after removing the same proportion of nodes, the natural connectivity of 0.2–3 μm eukaryotic microbial networks decreased more significantly, indicating weaker network resistance (slope = −0.127, R2 = 0.88, p < 0.001). When comparing the network stability between two fractions, the natural connectivity of 0.2–3 μm eukaryotic microorganisms was greater than the 3–200 μm eukaryotic microorganisms, indicated that the 0.2–3 μm eukaryotic microbial communities had stronger network stability (Figure 5B). Our results indicate that the 0.2–3 μm eukaryotic microorganisms have the wider niche width compared to the 3–200 μm eukaryotic microorganisms (Supplementary Figure S5), indicating that they could adapt to the wide range of environmental niches compared to the 3–200 μm eukaryotic microorganisms.
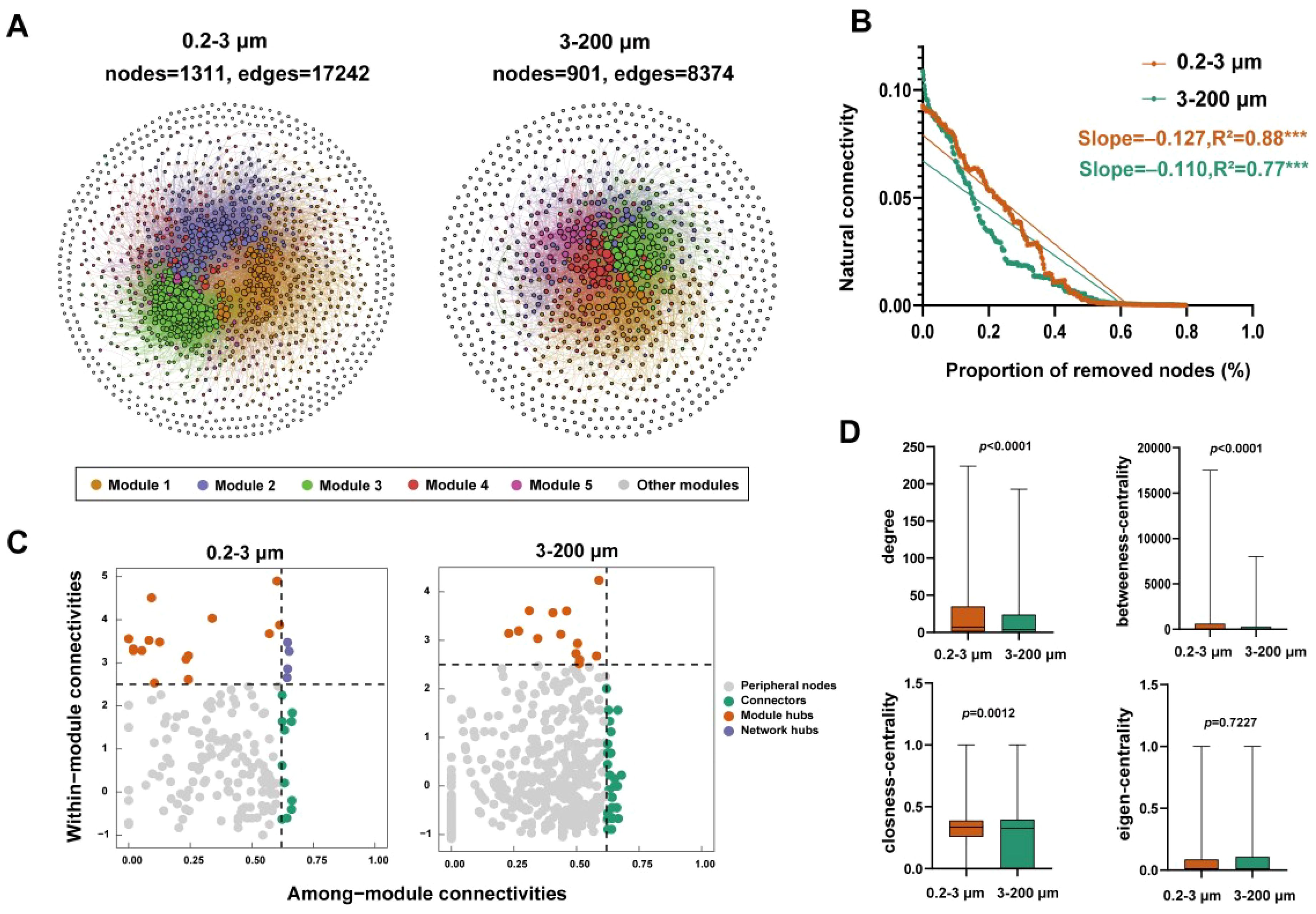
Figure 5. Co–occurrence network patterns of eukaryotic microbial communities. (A) co–occurrence networks of the two fractions; (B) Robustness analysis eukaryotic microbial communities; (C) Analysis of the Zi and Pi; (D) Network properties of the two fractions. R2 represents the fit to this model. Asterisks in B indicate the statistical significance (***: p < 0.001).
The position of a node (ASV) within the network can be determined by the “within module connectivity” (Zi) and “among–module connectivity” (Pi). There were 14 module hubs in the 0.2–3 μm eukaryotic microorganisms and 4 network hubs. The 3–200 μm eukaryotic microorganisms had 13 module hubs (Figure 5C). Additionally, the network topological characteristic parameters results shown that the 0.2–3 μm eukaryotic microorganisms had the relatively higher degree, betweenness-centrality, and closeness-centrality. While the 3–200 μm eukaryotic microorganisms had the higher eigen-centrality (Figure 5D).
3.5 Assembly mechanisms of eukaryotic microbial communities
The assembly process of eukaryotic microbial communities is realized by the NCM. In general, the NCM demonstrated a good fit for both the two fractions eukaryotic microbial community, underscoring the undeniable influence of stochastic processes in shaping the assembly process of the eukaryotic microbial community (Figure 6A). The values of the migration rate (m) of the 3–200 μm eukaryotic microorganisms were lower than those in the 0.2–3 μm eukaryotic microorganisms, reflecting the higher dispersal capability of the 3–200 μm eukaryotic microorganisms. To future investigate the relative contribution of assembly mechanism processes, we compared the βNTI of the eukaryotic microbial community (Figure 6B). The results showed that the majority of the eukaryotic microbial community the βNTI value were βNTI < −2 and |βNTI| < 2. This indicates that dispersal limitation and homogeneous selection processes contribute significantly to the construction of eukaryotic microbial communities. And the βMNTD (beta mean nearest taxon distance) value of the 0.2–3 μm eukaryotic microorganisms was relatively high (Supplementary Figure S6). We used iCAMP framework to Analyze the relative importance of community construction process (Figure 6C). It was found that the 0.2–3 μm eukaryotic microbial communities were composed of dispersal limitation, homogeneous selection, and heterogeneous selection processes, while the 3–200 μm eukaryotic microbial communities were mainly composed of dispersal limitation and homogeneous selection processes.
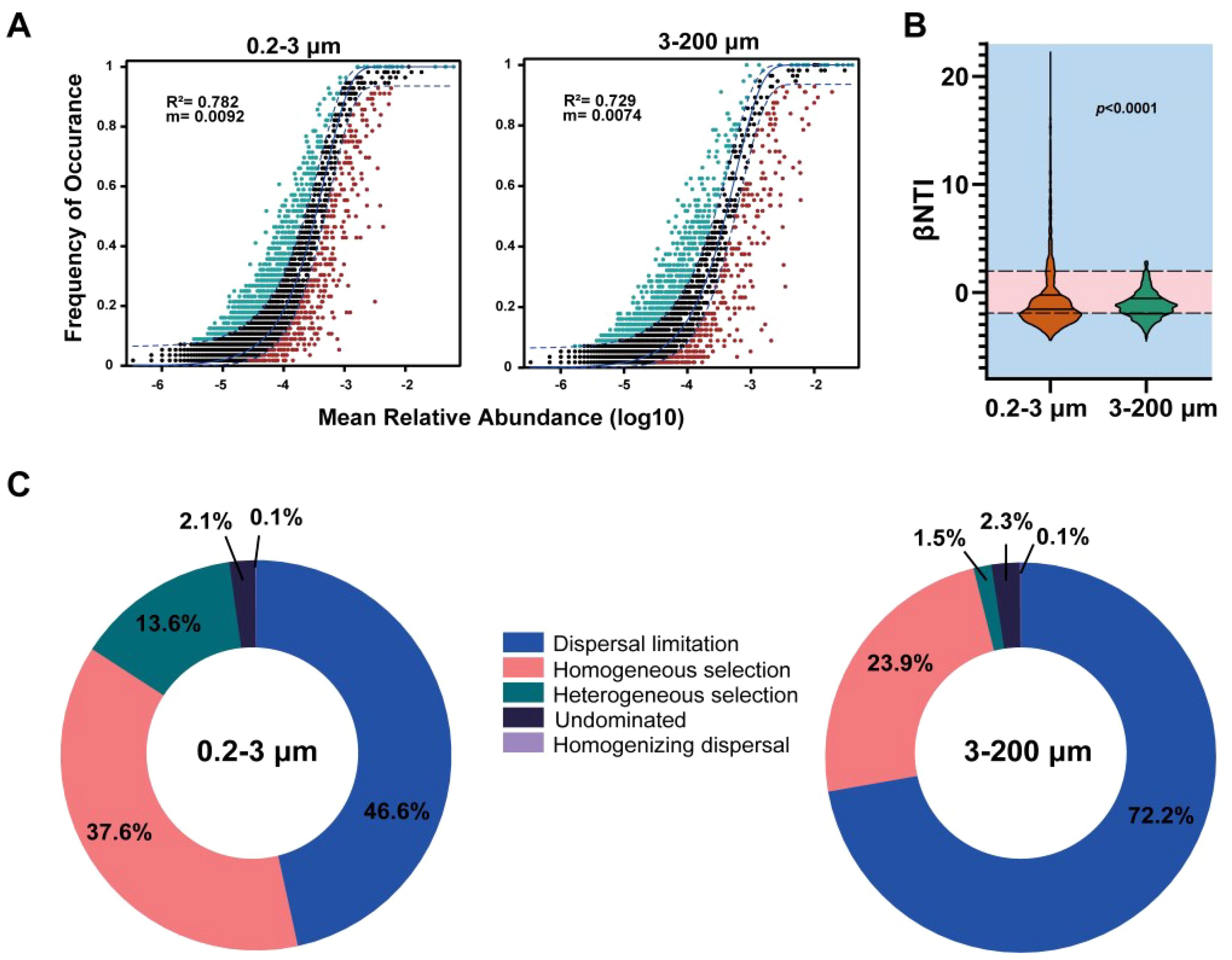
Figure 6. The assembly mechanism processes of eukaryotic microbial communities. (A) Fit of the NCM of the two fractions; (B) The βNTI of the two fractions. (C) The relative importance of community construction process. The p value indicates the statistical significance.
4 Discussion
4.1 Composition of eukaryotic microbial communities is different in two fractions
In this study, we explored the composition of the eukaryote’s community at 30 stations in the coastal China sea (Figure 1). The proportion of two fractions eukaryotic communities was different at each station. Overall, Alveolate (63.72%) accounts for a significant proportion. Previous studies have shown that, the Alveolates as one of the main eukaryotic lineages, which includes various nutritional types of eukaryotes and had widely distributed in various habitat environments (Patterson, 1999; Leander and Keeling, 2003). It mainly includes two highly representative branches in marine ecosystems, ciliates and dinoflagellates. Some species in Alveolates were important primary producers (for example, about half of the flagellates can fix carbon dioxide through photosynthesis), and some dinoflagellates were the cause of toxic algal blooms (Guillou et al., 2008). Similarly, the Malaspina expedition report suggests that Alveolates were the dominant protist groups across the world’s oceans (Pernice et al., 2016).
Community diversity was an important factor in shaping ecosystems and maintaining their functioning (Naeem et al., 2012; Duffy et al., 2017), and the heterogeneity within habitats was a driver of community diversity (Curd et al., 2018; Torsvik et al., 2002). Cell-size was an important ecological parameter of microorganisms, which affects various aspects such as intracellular material content, growth rate, extracellular material exchange, ecological niche (Chien et al., 2012; Young, 2006). Alpha diversity results showed that differences between eukaryotic microbial volume cell-sizes were more significant (p < 0.001, except for Simpson) than region and depth in the surveyed area (Figure 2). The findings emphasize the non-negligible nature of cell-size in shaping microbial community structure.
Our results also show that eukaryotic microbial composition is different at two fractions (Supplementary Figure S2). A global survey of microorganisms across ecosystems suggests that among protist taxa, Alveolata have the highest proportion in the oceans (Xiong et al., 2021). Our results showed that dinoflagellate (62.91%) had a higher percentage of the 3–200 μm eukaryotic microorganisms in Alveolata (Supplementary Figure S2). Studies have shown that the presence of dinoflagellates and associated cysts in coastal areas was an indicator for tracking anthropogenic eutrophication processes (Liu et al., 2023). Dinoflagellates dynamics were sensitive to water temperature, with most dinoflagellates growing at an optimal temperature of 20°C (Kumar et al., 2020). Our results also showed a strong correlation between the 3–200 μm eukaryotic microorganisms and temperature (Figure 4). We infer that the high abundance of dinoflagellate in the investigated area may be related to eutrophication and warm water temperatures.
4.2 Environmental factors shape eukaryotic microbial communities
The distribution and metabolic capacity of eukaryotic microorganisms were largely influenced by environmental factors (Thompson et al., 2017). The results of this study show that eukaryotic microorganisms were more affected by environmental distance than geographical distance (Figure 3). Eukaryotic biodiversity in oceans around the world was influenced by a combination of physicochemical environmental factors (Kirkham et al., 2013; Jing et al., 2018). So, we used statistical methods (Mantel test and ABT analysis) to revealed the main environmental factors that shaped the structure of eukaryotic microbial communities (Figure 4). The 0.2–3 μm eukaryotic microorganisms (0.2–3 μm) showed a significant correlation with Salinity, Density, and Silicate (r > 0.1, p < 0.05), while the 3–200 μm eukaryotic microorganisms showed a significant correlation with depth, temperature, and oxygen (r > 0.3, p < 0.001). Temperature directly impacts microbial growth, metabolism, and reproduction by affecting all levels of the biological hierarchy and it has been widely reported that temperature was a crucial environmental factor that determines the global geographical distribution of oceanic microbes (Brown et al., 2004; Shu and Huang, 2022). Changes in temperature could directly affect photosynthesis in a subset of eukaryotic microorganisms and also increased the rate of chemical reactions in eukaryotic microorganisms by accelerating the dissolution of mineral elements (Konrad–Schmolke et al., 2018). We found that the 3–200 μm eukaryotic microbial communities would be more affected by temperature (Figure 4A). The results of the study will help predict trends in the response of eukaryotic microbial communities in the context of global warming.
In specialized environments, microbial communities’ structural was changed in order to adapted the environmental survival. Previous studies were shown that depth limits planktonic intracellular enzyme activity, metabolism, and energy utilization-related capabilities, implying that planktonic communities need to adapted the bathypelagic zones or become less biologically active, leading to a reduction in alpha diversity (Daniel and Danson, 2013). In addition, oxygen concentration directly screens eukaryotic microorganisms that were better suited for survival or indirectly affects eukaryotic cells by creating unique biogeochemical ecological niches (Fenchel, 2002; Wang et al., 2020). Salinity has been identified as a major determinant of many microecosystems, even surpassing the importance of temperature in some environments (Lozupone and Knight, 2007). In contrast, the analyses of functional traits of species in the marine environment suggest that temperature and light have a stronger effect on microorganisms than nutrients or salinity (Raes et al., 2011; Sunagawa et al., 2015). Our results suggest that eukaryotes with different cell-sizes were affected by their environment to varying degrees. The 0.2–3 μm eukaryotic microorganisms were more affected by salinity, while the 3–200 μm eukaryotic microorganisms were more concerned within temperature and oxygen. Fluorescence was more closely controlled by richness of eukaryotes at two fractions. In conclusion, the variations of environmental conditions were affected eukaryotic microbial communities of different cell-sizes.
4.3 Unique assembly process of eukaryotes in the coastal China sea
The patterns of microorganism co–occurrence was regarded as a significant factor influencing community formation (Yu et al., 2023). In the co–occurrence network, positive correlations indicate cooperative relationships between species, while negative correlations indicate competitive relationships (Zhang et al., 2018). Our results show that there was more positive correlation between networks at the two fractions, indicating more reciprocal cooperation among species (Supplementary Figure S7). And the 0.2–3 μm eukaryotic microbial networks were more complex and have better stability (Figure 5). Furthermore, the modular structure was observed in networks, suggesting that co–occurring eukaryotic microorganisms employ various strategies to utilize the available resources in their environment, thereby avoiding direct competition (Macalady et al., 2008). This adaptability was advantageous for these eukaryotes in the natural environments. What’s more, it has been demonstrated that key taxa play an integral role in maintaining the structure of the network, and their absence will lead to instability or even disintegration of the network (Banerjee et al., 2018). In this study, the numbers of keystone taxa varied across two fractions, indicating differences in the essential taxa required to maintain network stability within different fractions. Additionally, the node-level topological characteristic parameters results shown that the 0.2–3 μm eukaryotic microorganisms had the relatively higher degree, betweenness–centrality, and closeness-centrality. While the 3–200 μm eukaryotic microorganisms had the higher eigen–centrality (Figure 5D). It has been found that the decrease in network stability is accompanied by an increase in node connectivity (Fan et al., 2018), centrality (Jordan, 2009), and network complexity (Gravel et al., 2016).
In this study, the construction of eukaryotic microbial communities required the combined contribution of stochastic and deterministic processes. This was mainly reflected in the important contributions of diffusion limitation and homogeneous selection processes to the construction of eukaryotes (Figure 6). The NCM fits well with the R2 values (R2 = 0.782 for 0.2–3 μm and R2 = 0.729 for 3–200 μm) to the eukaryotes (Figure 6A). The NCM also estimated the migration rate (m) of the community, reflecting the role of random processes in community construction (Zhang et al., 2022). In ecosystems with weaker environmental heterogeneity or resource competition, random assembly mechanisms may overturn deterministic processes (Sun et al., 2021; Yi et al., 2022). On the contrary, deterministic processes dominate (Logares et al., 2018). It was widely recognized that the balance between assembly processes can be explained by geographical scales and environmental gradients (Hanson et al., 2012). Furthermore, it was shown that temperature was the main factor mediating the equilibrium of community assembly processes (He et al., 2021). Our research findings indicate that the extent to which assembly processes shape the eukaryotes was largely determined by environmental changes in the coastal China sea.
5 Conclusion
Taken together, the differences in the diversity of eukaryotic microorganisms in the coastal China sea were mainly reflected in cell-size. The 0.2–3 μm eukaryotic microorganisms possess higher diversity and network complexity. Compared to geographical distance, environmental distance has the more significant impact on eukaryotic microorganisms. Temperature and oxygen determine the growth of larger eukaryotic microorganisms, while 0.2–3 μm eukaryotic microorganisms are more in need of proper salinity. Temperature and oxygen determine the growth of larger eukaryotic microorganisms, while 0.2–3 μm eukaryotic microorganisms were more in need of proper salinity and silicate. Fluorescence was important for richness in eukaryotic microorganisms of two fractions. In addition, dispersal limitation and homogeneous selection processes were major drivers in shaped eukaryotic microbial community structure. Our research findings provide new insights into the microbial ecological community and network structure in the coastal China sea.
Data availability statement
The datasets presented in this study can be found in online repositories. The names of the repository/repositories and accession number(s) can be found in the article/Supplementary Material.
Author contributions
ZC: Data curation, Writing – original draft, Writing – review & editing. TG: Formal analysis, Investigation, Writing – review & editing. JS: Conceptualization, Funding acquisition, Supervision, Writing – review & editing.
Funding
The author(s) declare financial support was received for the research, authorship, and/or publication of this article. This research was financially supported the National Key Research and Development Program of China (No. 2022YFC3105301); and also acknowledges support by Projects of Southern Marine Science and Engineering Guangdong Laboratory (Zhuhai) (SML2023SP218); the National Natural Science Foundation of China (41876134); the Changjiang Scholar Program of Chinese Ministry of Education (T2014253) to Jun Sun; and State Key Laboratory of Biogeology and Environmental Geology, China University of Geosciences (No. GKZ22Y656). And the project was supported by the “CUG Scholar” Scientific Research Funds at China University of Geosciences (Wuhan) (Project No.2020087). The project was supported by the Fundamental Research Funds for the Central Universities, China University of Geosciences (Wuhan) (No.CUG240631).
Acknowledgments
The authors were very thankful to the crew of the R/V “Yanping 2” implementing the open research cruise supported. We thanked Shuqun Song, Jinyu Yang and Zhijie Tan who provided the nutrient data and CTD data. We appreciate Xiamen University’s assistance with the cruise. We also appreciate the assistance provided by laboratory members Wei Teng and Yang Lu during the sample collection process.
Conflict of interest
The authors declare that the research was conducted in the absence of any commercial or financial relationships that could be construed as a potential conflict of interest.
Publisher’s note
All claims expressed in this article are solely those of the authors and do not necessarily represent those of their affiliated organizations, or those of the publisher, the editors and the reviewers. Any product that may be evaluated in this article, or claim that may be made by its manufacturer, is not guaranteed or endorsed by the publisher.
Supplementary material
The Supplementary Material for this article can be found online at: https://www.frontiersin.org/articles/10.3389/fmars.2024.1444870/full#supplementary-material
References
Banerjee S., Schlaeppi K., van der Heijden M. G. (2018). Keystone taxa as drivers of microbiome structure and functioning. Nat. Rev. Microbiol. 16, 567–576. doi: 10.1038/s41579–018–0024–1
Berdjeb L., Parada A., Needham D. M., Fuhrman J. A. (2018). Short-term dynamics and interactions of marine protist communities during the spring-summer transition. ISME. J. 12, 1907–1917. doi: 10.1038/s41396-018-0097-x
Bolger A. M., Lohse M., Usadel B. (2014). Trimmomatic: a flexible trimmer for illumina sequence data. Bioinformatics 30, 2114–2120. doi: 10.1093/bioinformatics/btu170
Bolyen E., Rideout J. R., Dillon M. R., Bokulich N. A., Abnet C. C., Al–Ghalith G. A., et al. (2019). Reproducible, interactive, scalable and extensible microbiome data science using QIIME 2. Nat. Biotechnol. 37, 852–857. doi: 10.1038/s41587–019–0209–9
Bonaldo D., Sanchez–Arcilla A., Samaras A. G., Snoussi M. (2023). Editorial: Climate change impacts on Mediterranean coastal and transitional areas: assessment, projection, and adaptation. Front. Mar. Sci. 10. doi: 10.3389/fmars.2023.1341637
Brown J. H., Gillooly J. F., Allen A. P., Savage V. M., West G. B. (2004). Toward a metabolic theory ecology. Ecology 85, 1771–1789. doi: 10.1890/03–9000
Caporaso J. G., Kuczynski J., Stombaugh J., Bittinger K., Bushman F. D., Costello E. K., et al. (2010). QIIME allows analysis of high-throughput community sequencing data. Nat. Methods 7, 335–336. doi: 10.1038/nmeth.f.303
Cavalcante G. H., Vieira F., Abouleish M., Atabay S., Campos E., Bento R. (2021). Environmental aspects of semi–closed lagoons in the Sharjah coastline during spring/neap tides, southern Arabian/Persian Gulf coast. Reg. Stud. Mar. Sci. 46, 101896. doi: 10.1016/j.rsma.2021.101896
Chesson P. (2000). Mechanisms of maintenance of species diversity. Annu. Rev. Ecol. Syst. 31, 343–366. doi: 10.1146/annurev.ecolsys.31.1.343
Chien A. C., Hill N., Levin P. (2012). Cell size control in bacteria. Curr. Biol. 22, R340–R349. doi: 10.1016/j.cub.2012.02.032
Curd E. E., Martiny J. B. H., Li H., Smith T. B. (2018). Bacterial diversity is positively correlated with soil heterogeneity. Ecosphere 9, e02079. doi: 10.1002/ecs2.2079
Daniel R. M., Danson M. J. (2013). Temperature and the catalytic activity of enzymes: A fresh understanding. FEBS Lett. 587, 2738–2743. doi: 10.1016/j.febslet.2013.06.027
De’ath G. (2007). Boosted trees for ecological modeling and prediction. Ecology 88, 243–251. doi: 10.1890/0012–9658(2007)88[243:BTFEMA]2.0.CO;2
De Vries F. T., Griffiths R. I., Bailey M., Craig H., Girlanda M., Gweon H. S., et al. (2018). Soil bacterial networks are less stable under drought than fungal networks. Nat. Commun. 9, 3033. doi: 10.1038/s41467–018–05516–7
Dubey M., Hadadi N., Pelet S., Carraro N., Johnson D. R., van der Meer J. R. (2021). Environmental connectivity controls diversity in soil microbial communities. Commun. Biol. 4, 492. doi: 10.1038/s42003–021–02023–2
Duffy J. E., Godwin C. M., Cardinale B. J. (2017). Biodiversity effects in the wild are common and as strong as key drivers of productivity. Nature 549, 261–264. doi: 10.1038/nature23886
Fan K., Weisenhorn P., Gilbert J. A., Chu H. (2018). Wheat rhizosphere harbors a less complex and more stable microbial co–occurrence pattern than bulk soil. Soil Biol. Biochem. 125, 251–260. doi: 10.1016/j.soilbio.2018.07.022
Fenchel T. (2002). Microbial behavior in a heterogeneous world. Science 296, 1068–1071. doi: 10.1126/science.1070118
Gravel D., Massol F., Leibold M. A. (2016). Stability and complexity in model meta–ecosystems. Nat. Commun. 7, 12457. doi: 10.1038/ncomms12457
Guidi L., Chaffron S., Bittner L., Eveillard D., Larhlimi A., Roux S., et al. (2016). Plankton networks driving carbon export in the oligotrophic ocean. Nature 532, 465–470. doi: 10.1038/nature16942
Guillou L., Bachar D., Audic S., Bass D., Berney C., Bittner L. (2013). The Protist Ribosomal Reference database (PR2): a catalog of unicellular eukaryote small sub-unit rRNA sequences with curated taxonomy. Nucleic Acids Res. 41, D597–D604. doi: 10.1093/nar/gks1160
Guillou L., Viprey M., Chambouvet A., Welsh R. M., Kirkham A. R., Massana R., et al. (2008). Widespread occurrence and genetic diversity of marine parasitoids belonging to Syndiniales (Alveolata). Environ. Microbiol. 10, 3349 3365. doi: 10.1111/j.1462–2920.2008.01731.x
Hanson C. A., Fuhrman J. A., Horner–Devine M. C., Martiny J. B. H. (2012). Beyond biogeographic patterns: processes shaping the microbial landscape. Nat. Rev. Microbiol. 10, 497–506. doi: 10.1038/nrmicro2795
He Q., Wang S., Hou W. G., Feng K., Li F. R., Hai W. M., et al. (2021). Temperature and microbial interactions drive the deterministic assembly processes in sediments of hot springs. Sci. Total. Environ. 772, 145465. doi: 10.1016/j.scitotenv.2021.145465
Jing H. M., Zhang Y., Li Y. D., Zhu W. D., Liu H. B. (2018). Spatial variability of picoeukaryotic communities in the Mariana trench. Sci. Rep. 8. doi: 10.1038/s41598–018–33790–4
Jordan F. (2009). Keystone species and food webs. Philos. Trans. R. Soc B–Biol. Sci. 364, 1733–1741. doi: 10.1098/rstb.2008.0335
Kembel S. W., Cowan P. D., Helmus M. R., Cornwell W. K., Morlon H., Ackerly D. D., et al. (2010). Picante: R tools for integrating phylogenies and ecology. Bioinformatics 26, 1463–1464. doi: 10.1093/bioinformatics/btq166
Kirkham A. R., Lepère C., Jardillier L. E., Not F., Bouman H., Mead A., et al. (2013). A global perspective on marine photosynthetic picoeukaryote community structure. ISME. J. 7, 922–936. doi: 10.1038/ismej.2012.166
Konrad–Schmolke M., Halama R., Wirth R., Thomen A., Klitscher N., Morales L., et al. (2018). Mineral dissolution and reprecipitation mediated by an amorphous phase. Nat. Commun. 9, 1637. doi: 10.1038/s41467–018–03944–z
Kumar P. S., Kumaraswami M., Ezhilarasan P., Rao G. D., Sivasankar R., Rao V. R., et al. (2020). Blooming of Gonyaulax polygramma along the southeastern Arabian Sea: Influence of upwelling dynamics and anthropogenic activities. Mar. pollut. Bull. 151, 110817. doi: 10.1016/j.marpolbul.2019.110817
Leander B. S., Keeling P. J. (2003). Morphostasis in alveolate evolution. Trends Ecol. Evol. 18, 395–402. doi: 10.1016/S0169–5347(03)00152–6
Lima–Mendez G., Faust K., Henry N., Decelle J., Colin S., Carcillo F., et al. (2015). Determinants of community structure in the global plankton interactome. Science 348, 1262073. doi: 10.1126/science.1262073
Lin G. M., Gao D. Z., Yang P., Liu S. T., Sun D. Y., Lin X. B. (2024). Linking microbial-driven key processes with carbon and nitrogen cycling in estuarine, coastal, and the nearshore areas. Front. Microbiol. 15. doi: 10.3389/fmicb.2024.1382148
Liu X. H., Liu Y. Y., Chai Z. Y., Hu Z. X., Tang Y. Z. (2023). A combined approach detected novel species diversity and distribution of dinoflagellate cysts in the Yellow Sea, China. Mar. pollut. Bull. 187, 114567. doi: 10.1016/j.marpolbul.2022.114567
Liu Y. P., Pan B. Z., Zhu X. Z., Zhao X. H., Sun H., He H. R., et al. (2022). Patterns of microbial communities and their relationships with water quality in a large–scale water transfer system. J. Environ. Manage. 319, 115678. doi: 10.1016/j.jenvman.2022.115678
Logares R., Deutschmann I. M., Junger P. C., Giner C. R., Krabberod A. K., Schmidt T. S. B., et al. (2020). Disentangling the mechanisms shaping the surface ocean microbiota. Microbiome 8, 55. doi: 10.1186/s40168-020-00827-8
Logares R., Tesson S. V. M., Canbäck B., Pontarp M., Hedlund K., Rengefors K. (2018). Contrasting prevalence of selection and drift in the community structuring of bacteria and microbial eukaryotes. Environ. Microbiol. 20, 2231–2240. doi: 10.1111/1462–2920.14265
Lozupone C. A., Knight R. (2007). Global patterns in bacterial diversity. Proc. Natl. Acad. Sci. 104, 11436–11440. doi: 10.1073/pnas.0611525104
Macalady J. L., Dattagupta S., Schaperdoth I., Jones D. S., Druschel G. K., Eastman D. (2008). Niche differentiation among sulfur–oxidizing bacterial populations in cave waters. ISME. J. 2, 590–601. doi: 10.1038/ismej.2008.25
Magurran A. E. (2007). Species abundance distributions over time. Ecol. Lett. 10, 347–354. doi: 10.1111/j.1461–0248.2007.01024.x
Massana R., Logares R. (2013). Eukaryotic versus prokaryotic marine picoplankton ecology: Marine ecology of picoeukaryotes and prokaryotes. Environ. Microbiol. 15, 1254–1261. doi: 10.1111/1462-2920.12043
Mitra A., Flynn K. J., Burkholder J. M., Berge T., Calbet A., Raven J. A., et al. (2014). The role of mixotrophic protists in the biological carbon pump. Biogeosciences 11, 995–1005. doi: 10.5194/bgd–10–13535–2013
Mo Y. Y., Peng F., Gao X. F., Xiao P., Logares R., Jeppesen E., et al. (2021). Low shifts in salinity determined assembly processes and network stability of microeukaryotic plankton communities in a subtropical urban reservoir. Microbiome 9, 128. doi: 10.1186/s40168–021–01079–w
Naeem S., Duffy J. E., Zavaleta E. (2012). The functions of biological diversity in an age of extinction. Science 336, 1401–1406. doi: 10.1126/science.1215855
Oksanen J., Blanchet F. G., Friendly M., Kindt R., Legendre P., McGlinn D., et al. (2019). vegan: community ecology package. Available online at: http://CRAN.Rproject.org/package=vegan. (Accessed 20 July 2024).
Pärtel M., Moora M., Zobel M. (2001). Variation in species richness within and between calcareous (alvar) grassland stands: the role of core and satellite species. Plant Ecol. 157, 205–213. doi: 10.1023/A:1013938624716
Pernice M. C., Giner C. R., Logares R., Perera–Bel J., Acinas S. G., Duarte C. M., et al. (2016). Large variability of bathypelagic microbial eukaryotic communities across the world’s oceans. ISME. J. 10, 945–958. doi: 10.1038/ismej.2015.170
Piredda R., Tomasino M. P., D’Erchia A. M., Manzari C., Pesole G., Montresor M., et al. (2017). Diversity and temporal patterns of planktonic protist assemblages at a Mediterranean Long Term Ecological Research site. FEMS Microbiol. Ecol. 93, fiw200. doi: 10.1093/femsec/fiw200
Raes J., Letunic I., Yamada T., Jensen L. J., Bork P. (2011). Toward molecular trait–based ecology through integration of biogeochemical, geographical and metagenomic data. Mol. Syst. Biol. 7, 473. doi: 10.1038/msb.2011.6
Reyon D., Tsai S. Q., Khayter C., Foden J. A., Sander J. D., Joung J. K. (2012). FLASH assembly of TALENs for high throughput genome editing. Nat. Biotechnol. 30, 460–465. doi: 10.1038/nbt.2170
Rognes T., Flouri T., Nichols B., Quince C., Mahe F. (2016). VSEARCH: a versatile open source tool for metagenomics. PeerJ 4. doi: 10.7717/peerj.2584
Shu W. S., Huang L. N. (2022). Microbial diversity in extreme environments. Nat. Rev. Microbiol. 20, 219–235. doi: 10.1038/s41579–021–00648–y
Stegen J. C., Lin X. J., Fredrickson J. K., Konopka A. E. (2015). Estimating and mapping ecological processes influencing microbial community assembly. Front. Microbiol. 6, 370. doi: 10.3389/fmicb.2015.00370
Stockner J. G. (1988). Phototrophic picoplankton: an overview from marine and freshwater ecosystems. Limnol. Oceanogr. 33, 765–775. doi: 10.4319/lo.1988.33.4part2.0765
Stoeck T., Bass D., Nebel M., Christen R., Jones M. D. M., Breiner H. W., et al. (2010). Multiple marker parallel tag environmental DNA sequencing reveals a highly complex eukaryotic community in marine anoxic water. Mol. Ecol. 19, 21–31. doi: 10.1111/j.1365-294X.2009.04480.x
Sun H., Pan B. Z., He H. R., Zhao G. N., Hou Y. M., Zhu P. H. (2021). Assembly processes and co–occurrence relationships in the bacterioplankton communities of a large river system. Ecol. Indic. 126, 107643. doi: 10.1016/j.ecolind.2021.107643
Sun P., Wang Y., Zhang Y. F., Logares R., Cheng P., Xu D. P., et al. (2023). From the sunlit to the aphotic zone: Assembly mechanisms and co-occurrence patterns of protistan-bacterial microbiotas in the Western Pacific Ocean. mSystems 8, e00013–e00023. doi: 10.1128/msystems.00013-23
Sunagawa S., Coelho L. P., Chaffron S., Kultima J. R., Labadie K., Salazar G., et al. (2015). Structure and function of the global ocean microbiome. Science 348, 1261359. doi: 10.1126/science.1261359
Supp S. R., Koons D. N., Ernest S. K. M. (2015). Using life history trade–offs to understand core–transient structuring of a small mammal community. Ecosphere 6, 187. doi: 10.1890/ES15–00239.1
Thompson L. R., Williams G. J., Haroon M. F., Shibl A., Larsen P., Shorenstein J., et al. (2017). Metagenomic covariation along densely sampled environmental gradients in the Red Sea. ISME. J. 11, 138–151. doi: 10.1038/ismej.2016.99
Torsvik V., Ovreås L., Thingstad T. F. (2002). Prokaryotic diversity—-magnitude, dynamics, and controlling factors. Science 296, 1064–1066. doi: 10.1126/science.1071698
Vellend M. (2010). Conceptual synthesis in community ecology. Q Rev. Biol. 85, 183–206. doi: 10.1086/652373
Villarino E., Watson J. R., Chust G., Woodill A. J., Klempay B., Jonsson B., et al. (2022). Global beta diversity patterns of microbial communities in the surface and deep ocean. Glob. Ecol. Biogeogr. 31, 2323–2336. doi: 10.1111/geb.13572
Wang Y. P., Li G. H., Shi F., Dong J., Gentekaki E., Zou S. B., et al. (2020). Taxonomic diversity of pico–/nanoeukaryotes is related to dissolved oxygen and productivity, but functional composition is shaped by limiting nutrients in eutrophic coastal oceans. Front. Microbiol. 11. doi: 10.3389/fmicb.2020.601037
Wang L. F., Wang Y. T., Li Y., Wang L. Q., Zhu J. X., Zhang W. L., et al. (2022). Effect of water chemistry on nitrogen transformation, dissolved organic matter composition and microbial community structure in hyporheic zone sediment columns. Environ. Res. 215, 114246. doi: 10.1016/j.envres.2022.114246
Xiong W., Jousset A., Li R., Delgado–Baquerizo M., Bahram M., Logares R., et al. (2021). A global overview of the trophic structure within microbiomes across ecosystems. Environ. Int. 151, 106438. doi: 10.1016/j.envint.2021.106438
Yi M. L., Fang Y., Hu G. P., Liu S. F., Ni J. R., Liu T. (2022). Distinct community assembly processes underlie significant spatiotemporal dynamics of abundant and rare bacterioplankton in the Yangtze River. Front. Environ. Sci. Eng. 16, 79. doi: 10.1007/s11783–021–1513–4
Young K. D. (2006). The selective value of bacterial shape. Microbiol. Mol. Biol. Rev. 70, 660–703. doi: 10.1128/MMBR.00001–06
Yu B. B., Xie G. J., Shen Z., Shao K. Q., Tang X. M. (2023). Spatiotemporal variations, assembly processes, and co–occurrence patterns of particle–attached and free–living bacteria in a large drinking water reservoir in China. Front. Microbiol. 13. doi: 10.3389/fmicb.2022.1056147
Zhang T., Xu S., Yan R. M., Wang R. Y., Gao Y. X., Kong M. (2022). Similar geographic patterns but distinct assembly processes of abundant and rare bacterioplankton communities in river networks of the Taihu Basin. Water Res. 211, 118057. doi: 10.1016/j.watres.2022.118057
Zhang X. L., Yao C., Zhang B. S., Gong J., Wang G. Y., Zhao J. M., et al. (2023). Dynamics of benthic nitrate reduction pathways and associated microbial communities responding to the development of seasonal deoxygenation in a coastal mariculture zone. Environ. Sci. Technol. 57, 15014–15025. doi: 10.1021/acs.est.3c03994
Zhang B. G., Zhang J., Liu Y., Shi P., Wei G. H. (2018). Co–occurrence patterns of soybean rhizosphere microbiome at a continental scale. Soil Biol. Biochem. 118, 178–186. doi: 10.1016/j.soilbio.2017.12.011
Zhou J., Ning D. (2017). Stochastic community assembly: Does it matter in microbial ecology? Microbiol. Mol. Biol. Rev. 81, e00002–e00017. doi: 10.1128/MMBR.00002–17
Zhou Y. Q., Sun B. Y., Xie B. H., Feng K., Zhang Z. J., Zhang Z., et al. (2021). Warming reshaped the microbial hierarchical interactions. Glob. Change Biol. 27, 6331–6347. doi: 10.1111/gcb.15891
Keywords: eukaryotic microorganisms, coastal environments, assembly mechanism, network stability, biodiversity
Citation: Chen Z, Gu T and Sun J (2024) Dispersed limitation and homogeneous selection drive the eukaryotic microbial community assembly and network stability in the coastal China sea. Front. Mar. Sci. 11:1444870. doi: 10.3389/fmars.2024.1444870
Received: 07 June 2024; Accepted: 19 August 2024;
Published: 03 September 2024.
Edited by:
Xianbiao Lin, Ocean University of China, ChinaCopyright © 2024 Chen, Gu and Sun. This is an open-access article distributed under the terms of the Creative Commons Attribution License (CC BY). The use, distribution or reproduction in other forums is permitted, provided the original author(s) and the copyright owner(s) are credited and that the original publication in this journal is cited, in accordance with accepted academic practice. No use, distribution or reproduction is permitted which does not comply with these terms.
*Correspondence: Jun Sun, cGh5dG9wbGFua3RvbkAxNjMuY29t