- 1Mercator Ocean International, Toulouse, France
- 2Nologin Oceanic Weather Systems, Santiago de Compostela, Spain
- 3Institute of Electrical and Electronics Engineers (IEEE), Paris, France
- 4MetOffice, Exeter, United Kingdom
- 5Laboratoire de Physique des Océans, IFREMER, Brest, France
- 6NVIDIA Corporate, Santa Clara, CA, United States
- 7Nansen Environmental and Remote Sensing Center, Bergen, Norway
- 8Japan Agency for Marine-Earth Science and Technology, Kanagawa, Japan
- 9Bureau of Meteorology, Melbourne, VIC, Australia
- 10European Marine Observation and Data Network, Brussels, Belgium
- 11Ecological Modelling (ECOMOD), Royal Belgian Institutes of Natural Sciences, Brussels, Belgium
- 12Biogeochemistry and Modelling of the Earth System (BgeoSys), Université Libre de Bruxelles, Brussels, Belgium
- 13Center for Ocean-Atmospheric Prediction Studies, Florida State University, Tallahassee, FL, United States
- 14Department of Meteorology, Institute of Geosciences, Federal University of Rio de Janeiro (UFRJ), Rio de Janeiro, Brazil
- 15Centro Euro-Mediterraneo sui Cambiamenti Climatici (CMCC) Foundation - Euro-Mediterranean Center on Climate Change, Bologna, Italy
- 16Data Science and Water Quality, Deltares, Delft, Netherlands
- 17Department of Oceanography, Istituto Nazionale di Oceanografia e di Geofisica Sperimentale, Trieste, Italy
- 18InfraScience Lab, Istituto di Scienza e Tecnologie dell’Informazione “Alessandro Faedo”, Dipartimento di Ingegneria, ICT e Tecnologie per l’Energia e i Trasporti, Consiglio Nazionale delle Ricerche, Pisa, Italy
- 19Institute for Marine and Antarctic Studies, Oceans and Cryosphere, University of Tasmania, Hobart, TAS, Australia
- 20Oceanography Department, Fisheries and Oceans Canada, North Atlantic Fisheries Center, St. John’s, NL, Canada
- 21Department of Oceanography, University of Cape Town, Cape Town, South Africa
- 22Department of Oceanography, Dalhousie University, Halifax, NS, Canada
- 23Oden Institute for Computational Engineering and Sciences, The University of Texas at Austin, Austin, TX, United States
- 24Laboratoire d’Etudes en Géophysique et Océanographie Spatiales (LEGOS), Institut de Recherche pour le Développement, Toulouse, France
- 25National Oceanic and Atmospheric Administration (NOAA), National Centers for Environment Information, Stennis Space Center, Hancock County, MS, United States
- 26Physical Science and Engineering Division, King Abdullah University of Science and Technology (KAUST), Thuwal, Saudi Arabia
- 27Indian National Centre for Ocean Information Services (INCOIS), Telangana, Hyderabad, India
- 28Marine Systems Modelling, National Oceanography Center, Southampton, United Kingdom
- 29Centro Euro-Mediterraneo sui Cambiamenti Climatici (CMCC) Foundation - Euro-Mediterranean Center on Climate Change, Lecce, Italy
- 30Meteorological Research Division, Environment and Climate Change Canada, Québec, QC, Canada
- 31Decade Coordinating Office - Ocean Observing, Intergovernmental Oceanographic Commission - United Nations Educational, Scientific and Cultural Organization (IOC – UNESCO), Paris, France
- 32Physical & Biological Sciences Division, Ocean Sciences Department Institute of Marine Sciences, Institute of Marine Sciences, University of California, Santa Cruz, Santa Cruz, CA, United States
- 33ETT SpA, Genoa, Italy
- 34IBM Research, Dublin, Ireland
- 35Science and Technology Facilities Council, Daresbury Laboratory, Hartree Centre, Daresbury, United Kingdom
- 36First Institute of Oceanography, Ministry of Natural Resources, Qingdao,China
- 37Commonwealth Scientific and Industrial Research Organization (CSIRO) Environment, Castray Esplanade, Hobart, TAS, Australia
- 38Data, Science and Technology, National Oceanography Centre, Southampton, United Kingdom
- 39Institute of Coastal Systems - Analysis and Modeling, Helmholtz-Zentrum Hereon, Geesthacht, Germany
- 40Hakai Institute, Calvert Island, BC, Canada
- 41Egagasini Node, South African Environmental Observation Network (SAEON), Cape Town, South Africa
- 42National Marine Environmental Forecasting Center, Beijing, China
- 43Department of Marine and Coastal Sciences, Rutgers, The State University of New Jersey, New Brunswick, NJ, United States
Predicting the ocean state in a reliable and interoperable way, while ensuring high-quality products, requires forecasting systems that synergistically combine science-based methodologies with advanced technologies for timely, user-oriented solutions. Achieving this objective necessitates the adoption of best practices when implementing ocean forecasting services, resulting in the proper design of system components and the capacity to evolve through different levels of complexity. The vision of OceanPrediction Decade Collaborative Center, endorsed by the UN Decade of Ocean Science for Sustainable Development 2021-2030, is to support this challenge by developing a “predicted ocean based on a shared and coordinated global effort” and by working within a collaborative framework that encompasses worldwide expertise in ocean science and technology. To measure the capacity of ocean forecasting systems, the OceanPrediction Decade Collaborative Center proposes a novel approach based on the definition of an Operational Readiness Level (ORL). This approach is designed to guide and promote the adoption of best practices by qualifying and quantifying the overall operational status. Considering three identified operational categories - production, validation, and data dissemination - the proposed ORL is computed through a cumulative scoring system. This method is determined by fulfilling specific criteria, starting from a given base level and progressively advancing to higher levels. The goal of ORL and the computed scores per operational category is to support ocean forecasters in using and producing ocean data, information, and knowledge. This is achieved through systems that attain progressively higher levels of readiness, accessibility, and interoperability by adopting best practices that will be linked to the future design of standards and tools. This paper discusses examples of the application of this methodology, concluding on the advantages of its adoption as a reference tool to encourage and endorse services in joining common frameworks.
1 Introduction
Ocean forecasting enhances our understanding of the dynamic marine environment, supports sustainable ocean use, and protects lives, livelihoods, and marine ecosystems. It plays a vital role in disaster preparedness and response (Link et al., 2023; Visbeck, 2018; She et al., 2016), helping authorities anticipate and mitigate the impacts of extreme events such as tsunamis (Tsushima and Ohta, 2014; Sugawara, 2021), storm surges (Pérez Gómez et al., 2022; Morim et al., 2023; Chaigneau et al., 2023), marine heatwaves (Hartog et al., 2023; de Boisséson and Balmaseda, 2024; Bonino et al., 2024), oil spill accidents (Cucco et al., 2024; Keramea et al., 2023; Kampouris et al., 2021), etc. It supports maritime safety by providing warnings of hazardous conditions such as storm surges, rough seas, or strong currents, enabling ships to navigate safely and avoid potential dangers (Goksu and Arslan, 2024; Jeuring et al., 2024). Furthermore, it facilitates efficient planning and operations for industries such as offshore energy, shipping, and coastal engineering, optimizing activities like offshore drilling, vessel routing, and coastal infrastructure development (Nezhad et al., 2024; Kim and Lee, 2022; Fennel et al., 2019).
Given the importance of ocean forecasting, the application of best practices is essential for several reasons. Firstly, they promote the reliability of forecasted information, which is crucial for making informed decisions. By adhering to established best practices, forecasters can maintain high standards of data quality, enhancing the credibility and trustworthiness of their forecasts. Additionally, best practices promote consistency and interoperability among different forecasting systems, enabling seamless integration of forecast data into decision-support tools. Ultimately, best practices help ensure that ocean forecasting services meet the evolving user’s needs (Pearlman et al., 2019; Buck et al., 2019; Tanhua et al., 2019; Kourafalou et al., 2015).
Unfortunately, no well-established set of best practices for ocean forecasting activities exists. This often results in non-optimal and non-interoperable systems and in significant difficulties when setting up a new service, especially for scientists and engineers working in environments less experienced. This document addresses these gaps and describes the practices required to improve critical aspects of an ocean forecasting service (through operations, validation, and data dissemination). The Operational Readiness Level (ORL) presented here is designed to guide and promote the adoption of such practices and will serve system developers and users to assess the operational development status of an ocean forecasting system. It will pinpoint gaps that should be addressed to further mature a system. Improving the ORL qualification of a service will be a means to identify and implement best practices and standards in ocean forecasting, enhancing the overall operability of the system.
The ORL here described applies to operational forecasting systems that produce daily or weekly updated predictions for Essential Ocean Variables (EOV) (or even on higher frequency). Future evolution of the ORL concept will additionally consider systems that update regularly ocean reanalysis and climate projections.
This paper was developed by The Ocean Forecasting Co-Design Team (OFCT), a group of worldwide experts integrated into the OceanPrediction Decade Collaborative Center (OceanPrediction DCC1). This DCC is a cross-cutting structure, as described in the Decade Implementation Plan (UNESCO-IOC, 2021), to develop collaborative efforts towards “a predicted ocean based on a shared and coordinated global effort in the framework of the UN Ocean Decade”. The United Nations (UN) Decade of Ocean Science for Sustainable Development 2021-2030 (referred to as ‘the Ocean Decade’) was proclaimed by the 72nd Session of the UN General Assembly (UNGA) on the 5th of December 2017. The Decade is being coordinated by UNESCO-IOC to promote transformational, large-scale change to advance urgent action on moving from the ‘ocean we have’ to the ‘ocean we want’. It includes a focus on least-developed countries (LDCs), Small Island Developing States (SIDS), and land-locked developing countries (LLDCs). The Decade will support ocean data, information, and knowledge systems to evolve to a much higher level of readiness, accessibility, and interoperability.
The main objective of the OFCT is to analyze the status of ocean forecasting, identify gaps and ways forward, and design an ocean forecasting architecture, including the present ORL, oriented to promote the adoption of best practices. This is done in collaboration with Decade Programmes. Amongst them, and having participated in this document, it is important to highlight the following ones, that are primarily attached to OceanPrediction DCC: the Ocean Prediction Capacity for the Future (ForeSea2), Ocean Practices for the Decade (OceanPractices3), and the Digital Twins of the Ocean (DITTO4).
2 Desired characteristics of an ocean forecasting service and associated best practices
A useful ocean forecasting service must properly solve the technicalities associated with several key characteristics of the system (Davidson et al., 2019). These are reliability and timeliness of operations; validation; and dissemination of results. This section will describe the best practices associated with these technicalities.
In addressing practices for ocean forecasting, several factors impact the maturity of a practice. These include (Bushnell and Pearlman, 2024) the level of a practice’s documentation, its replicability, the breadth of usage, the endorsement of the practice by an expert team, and sustainability attributes of a practice such as understanding the uncertainties in employing the practice, user feedback mechanisms, and training. Based on an ocean maturity model (Mantovani et al., 2024), practices can be categorized as emerging, documented, good, better, or best. The maturity model describes the attributes of each level and the path toward mature best practices. There are also other guidelines that should be considered for ORL implementation (Pearlman et al., 2021). The goal in defining the ORL is to have all practices used for ocean forecasting as best practices, but there is an evolution to achieve a best practice and it is understood that the term “best practices” used here takes into account that some of the “better practices” can provide significant benefits while they are still evolving to be a best practice. The ORL is a tool that will guide the adoption of best practices and facilitate the creation of practices where gaps are identified. From this perspective, this section offers practices that support key attributes of an effective ocean forecasting system.
2.1 Reliability and timeliness of operations
Users must have confidence in the reliability of the forecasting service (Brassington, 2021), and in the timeliness of the delivery of its results (Le Traon et al., 2019; Sotillo et al., 2020). This is linked to the existence of a robust operational chain, a properly designed and maintained technical environment, mechanisms to secure operations, and fulfillment of user needs and expectations (Alvarez Fanjul et al., 2022). Each of these will be addressed in the following points.
2.1.1 Robust operational chain
The operational chain is a key component to ensure a reliable and timely ocean forecasting service. This chain should verify the existence of all the required forcings and other upstream data, run the model or artificial intelligence in charge of the computations, and archive the output.
The operational chain must be robust. The software should be able to launch the process even if some upstream data is missing (a good example of this is using climatology or persistence for rivers in case real-time data is not available). The integrity of the forcing data files should be checked before their use (e.g., looking at the file size, or checking data integrity through a checksum function). Provision of key forcing and validation data should ideally be available from the data providers via a Service Level Agreement or any other similar mechanism. Additionally, selected results of the ocean forecasting service should be automatically checked, via software, for their physical, and/or chemical, and/or biological consistency (one example is to check that salinity is always higher than zero).
All the main steps of the processing chain should be automatically tracked via a log file, where clear information is provided about these steps of the sequence and, more particularly, about failures in the chain. If this is not achieved, it should at least create a basic log file on each forecasting cycle informing on the start and correct (or incorrect) ending of the procedure.
All the processing chain and software managing the operations should be properly documented. Software and documents should be stored in a repository with a clear versioning policy.
2.1.2 Properly designed and maintained technical environment
The technical environment hosting the forecast service must be properly designed and maintained. Its proper design must ensure that sufficient and reliable computational resources are secured for the operation of the system and that the computers and networks employed are properly protected against cyber-attacks. Hardware used for computations should be in a room/facility that fulfills the required specifications for its proper functioning, or in a cloud system that complies with these requirements (for example, some High-Performance Computing (HPC) systems could require a server room with properly controlled cooling).
To have a robust and well-designed working environment, the software of the operational chain should be executed in a different working environment (production environment) than the one(s) used for testing and/or development.
Backup of results and computing resources is important. A backup storage system should be used to safeguard the operational software availability and to ensure the security of the data resulting from the system. Optimally, the data backup hardware should be located at a different facility or in a cloud environment, reinforcing reliability and disaster recovery. It is also desirable to count on a backup HPC resource (could be a cloud resource), ready to take over the operations in case of a malfunction or unscheduled downtime of the main HPC capability (with codes compiled and access to all input data). Planned downtimes of the nominal HPC facility should be communicated sufficiently early to allow switching to a backup one. Backup system performance should be routinely verified.
2.1.3 Secured operations
The system and its environments (i.e., production, testing and/or development) should be resilient and protected against unexpected events and malfunctions. This can be achieved by combining human intervention with ad-hoc software.
Optimally, the resolution of non-hardware-related problems on the operational chain should be secured when required by human intervention, on any day of the year, not only on working days. If this is not possible, these problems should be solved by human intervention during office hours (typically 8 hours - 5 days per week). A human resources rotation plan should be ready to cover the holiday periods of the people responsible for the system. Similarly, hardware functioning should be monitored on any day of the year, not only on working days, with plans to solve component malfunctions in place (for example, replacement of a defective hard drive) that includes a realistic estimation of resolution times.
The availability of computing resources (e.g., disk space, number of cores) should be checked before launching the operational chain and monitored during operations. If this is not possible, a procedure should be executed routinely to check and ensure the availability of sufficient disk space and networking resources.
Technical staff should be properly trained and must have all the required information for system monitoring and troubleshooting. Documented recovery procedures should be designed and available for each failure mode of the processing chain that has repeatedly occurred in the past (these procedures could be based on actions launched via software or by human intervention). The responsible personnel must get the right information about the functioning of the service; they should be alerted automatically about malfunctions in the operations (either by e-mail or other means of communication) and a monitoring dashboard could be set up to visualize the status of the operational service workflow, alerting the operator in case of failures or problems.
2.1.4 User needs
Users should have detailed and timely information about specific aspects of the operational chain that might affect them, such as the delivery time of products. A Service Level Agreement (SLA) or any other similar mechanism should be available to describe product delivery time, recovery time in case of malfunction/unavailability, Key Performance Indicators (KPI), and other operational properties of the system.
Changes in the operations may impact users. An evolution of the system consisting of major changes in the software or hardware that could affect the results should include a period long enough when operations of older and newer versions are being done in parallel. This will permit the validation of the continuity of performance and facilitate the transition to the updated system. Additionally, a roadmap for future service evolution describing changes in the operational suite that might affect users (for example, improvements in the delivery time) should be available on request.
Incidents that can arise during the execution of the operational chain may significantly affect the resulting products and users need to be informed. For example, when forecasts are generated using forcings and/or observations that are not optimal for the corresponding cycle (for example, in case a climatology is used when no data is available), this situation should be flagged automatically on the log files and, ideally, this information should be present on the product metadata corresponding to the specific forecast cycle.
There should be mechanisms in place for collecting users’ feedback on those aspects related to operations (reliability, timeliness, etc.).
2.2 Validation
Regular validation of the system results with observations is an essential process in ocean forecasting (Hernandez et al., 2015; Alvarez Fanjul et al., 2022). Proper service validation is also at the core during the set-up phase of the systems. Additionally, product assessment should also be used to ensure satisfactory performance over time, and a correct service evolution, and, certainly, it is critical to ensure user confidence in the forecasts (Le Traon et al., 2021).
2.2.1 Set up the system
Validation is critical during the setup phases of the system. Offline system validation should be done during the service’s setup and/or pre-operational phase, covering a period long enough to assess the quality of the solution concerning the main phenomena to be forecasted. Quality control of observational data through quality flags, if provided in origin, should be considered during the offline validation. If no data quality control is provided, a simple ad hoc quality control process should be carried out (i.e., check of values over thresholds, detection of outliers to remove, etc.) to ensure the quality of observational input data.
In the case of downscaled or nested systems, an initial validation of the child model should be performed and compared with the one obtained from the parent model. In the case of operational systems with data assimilation, the quality of the data assimilation should be demonstrated by offline studies comparing outputs with independent observations (non-assimilated observations) and non-assimilated variables. An intercomparison of the validation results obtained from other similar systems covering the same domain should also be performed when possible.
2.2.2 Operational control of the system
Regular validation of system results is critical to ensure that the system performance is satisfactory and that solutions are free from undesired trends or spikes. This validation should be done both in graphical format and using significant statistical numerical analysis. Validation should be executed in real-time, including the last received observations, and it should use all possible available observations.
Some validation processes executed during the system setup phase should also be carried out on a regular basis during the operational phase: this includes a comparison of validation of the system with the one from the parent model (in case of downscaled or nested system), or intercomparison with other similar systems covering the same service domain.
The results of the validations must be supervised by the system manager regularly. A qualitative check of the validation results should be performed by a human operator (e.g., typically once a week). Tendencies or spikes should be reported to the operational and development teams even if they only turn out to be random fluctuations.
Skill scores corresponding to the different forecast horizons should be computed regularly.
Very advanced systems could include additional characteristics, such as forcings validation with relevant data in each forecast cycle and/or in delayed mode to support the understanding of the impact of its errors in the ocean forecast, or additional quality control of the observations entering the validation (done by the forecasting service), verifying and/or improving the quality control done at the original distribution center.
2.2.3 System evolution
Evolution of systems (consisting of major changes in the system’s software or hardware that could affect the system results) should include a re-computing of offline validation for a period long enough to evaluate properly the dynamics of the predicted variables.
In case a reprocessing of the observations produces changes in their values or in their quality control, the system should be accordingly re-validated against the updated set of observations (for some observational services this is done typically every 6 months or every year).
All the validation software should be properly documented and stored in a repository with a clear versioning policy.
2.2.4 User confidence
Users of ocean forecasting services need to have confidence in the reliability of the forecasts (Hernandez et al., 2018). Validation instills this confidence by demonstrating that the forecasting models have been rigorously tested and validated against observational data (Byrne et al., 2023; Lorente et al., 2019). Validation helps mitigate risks associated with relying on inaccurate forecasts, thus enabling better-informed decisions that can prevent potential hazards or losses.
Ideally, the users must have access to validations from all existing observational sources (both in graphical format and using statistical analysis) corresponding to the whole period of operations, from the start of operations to the last real-time data received. Evolution of the system (major changes in the system’s software or hardware that could affect the system results) should include an updated validation. Additionally, it is desirable that the user can obtain, under request, information about the validation results carried out during the setup or pre-operational phase.
To serve specific users and purposes, tailored uncertainty information for users and/or process-oriented validation (for example, eddy/mesoscale activity) should be provided and updated either on each forecast cycle and/or in delayed mode. The evolution of systems should include reassessment of tailored uncertainty estimations and/or process-oriented validation.
A roadmap for future service evolution describing potential changes in the validation should be available to users on request.
2.3 Product dissemination and system interoperability
System results must be easily accessible by authorized users. The ability to integrate them with other systems and data sources enhances the usefulness of the forecasting service. Interoperability facilitates data exchange and enables users to incorporate ocean forecasts into their existing workflows and decision-support tools (Snowden et al., 2019). To properly deliver the forecast, the data resulting from the system must be organized as a “product” that must be carefully designed, counting with efficient distribution mechanisms, and properly implemented from the technical point of view.
2.3.1 Product design
The results of the system must be served in the form of a well-defined “product”. This product should contain the results of the forecasting service, as well as all the required associated metadata. Metadata should contain updated information on the quality of the dataset or a link to where this information is available. Product metadata should identify unequivocally a product and its system version. This can be done, for example, via a Digital Object Identifier (DOI). Good metadata serves the user but also increases the level of confidence that the supplier (the manager of the forecasting service) has in their data being used appropriately, in the origin of data being acknowledged, and in its efforts being recognized.
Data contained in the product should be stored in a well-described data format, so the users can use them easily.
If allowed by upstream data providers, forcings and/or observations, as used and processed by the forecasting system, should be distributed along with the results (for example, heat fluxes derived from bulk formulations).
2.3.2 Distribution mechanisms
Ideally, distribution mechanisms should be in place to allow users to access all the products in the catalogue as produced by the forecasting service, starting from the day of its release until the last executed cycle. This could be done, for example, via FTP (File Transfer Protocol) or through a specific API (Application Programming Interface). Numerical data should be distributed to users using internationally agreed data standard formats. Online tools should be available to explore in graphical format (for example via plots of time series or 2D fields in a web page) all the data in the product catalogue.
On very advanced services, an analysis of the fulfillment of FAIR (Findable, Accessible, Interoperable, and Reusable) principles (Wilkinson et al., 2016; Tanhua et al., 2019; Schultes et al., 2020) should be available, as well as a plan to improve the situation for those that are not satisfied. This analysis could be done via a FAIR implementation profile (Schultes et al., 2020).
2.3.3 Technical environment
The limits of the network bandwidth and the internet server used for system product distribution should be checked through load tests regularly. If needed, load balancing should be implemented (load balancing here refers to the technical capacity for distributing the incoming traffic from users’ requests across several dedicated servers to guarantee good performance).
A mechanism for tracking the number of users and their associated available information (i.e., the country where they reside) should be available and executed regularly to get a better understanding of the impact of the system products.
2.3.4 User support
A product catalogue and a user’s guide should be available and maintained.
Ideally, for a very advanced service, a help desk could operate 24/7 (i.e., 24 hours, every day of the week) solving user problems and providing answers to questions. Optionally, a help desk that provides a 24/7 service could be based on a two-level scheme: Initially (service level 1), the user is served by a chatbot or a similar automatic mechanism. If the user is not getting a satisfactory reply on this first level, it is offered the option of speaking to a human operator (service level 2), on 8/5 support (i.e., 8 hours a day - 5 days of the week). Nevertheless, for most of the services, a help desk operating on 8/5 will be sufficient to provide user support.
A mechanism that allows users to register on the system, compatible with FAIR principles, should be available. This mechanism should be designed to provide additional information to system developers about the use of the products and can be used as a contact point for notifications. Registered users should be notified of changes in the system that could affect them (e.g., changes in the data format) with sufficient time in advance.
A co-design mechanism should be in place, ensuring that the products evolve to fulfill users’ needs. These could be identified and documented, for example, through surveys. One example could consist of the improvement of a service product by providing higher frequency datasets, moving from daily to hourly means, if this is a major user request. A user feedback mechanism for comments and recommendations is also desirable for designing product catalogue evolution.
Documentation describing the evolution in time of a system and its products should be available. A roadmap for future service evolution describing changes in the dissemination tools should be available to users on request. Documentation for training in the use of the system products should be available.
3 Operational readiness level
3.1 Definition
The Operational Readiness Level (ORL) for ocean forecasting is a new tool to promote the adoption and implementation of the practices as described in the previous section. Some of these practices refer to an ideal situation, corresponding to a “perfect service”, that is rarely achieved. The ORL breaks down these concepts into small advances or steps towards the described optimal solutions, facilitating the tracking of successive improvements that could lead to a progressively better service.
The ORL serves as a tool for system developers to assess the operational status of an ocean forecasting system. Improving the ORL qualification of a service is a means to implement best practices in ocean forecasting, improving the system.
The ORL comprises three independent digits designed to certify the operational status of an ocean forecasting system (Figure 1). These reflect the three key attributes described in the previous section. Each digit ranges from 0 (minimum) to 5 (maximum), with decimal numbers being allowed. These digits correspond to distinct aspects related to operationality:
● The First Digit reflects the reliability of the service, focusing on production aspects rather than product quality.
● The Second Digit monitors the level of validation for the service.
● The Third Digit assesses the various degrees of product dissemination achievable by the system.
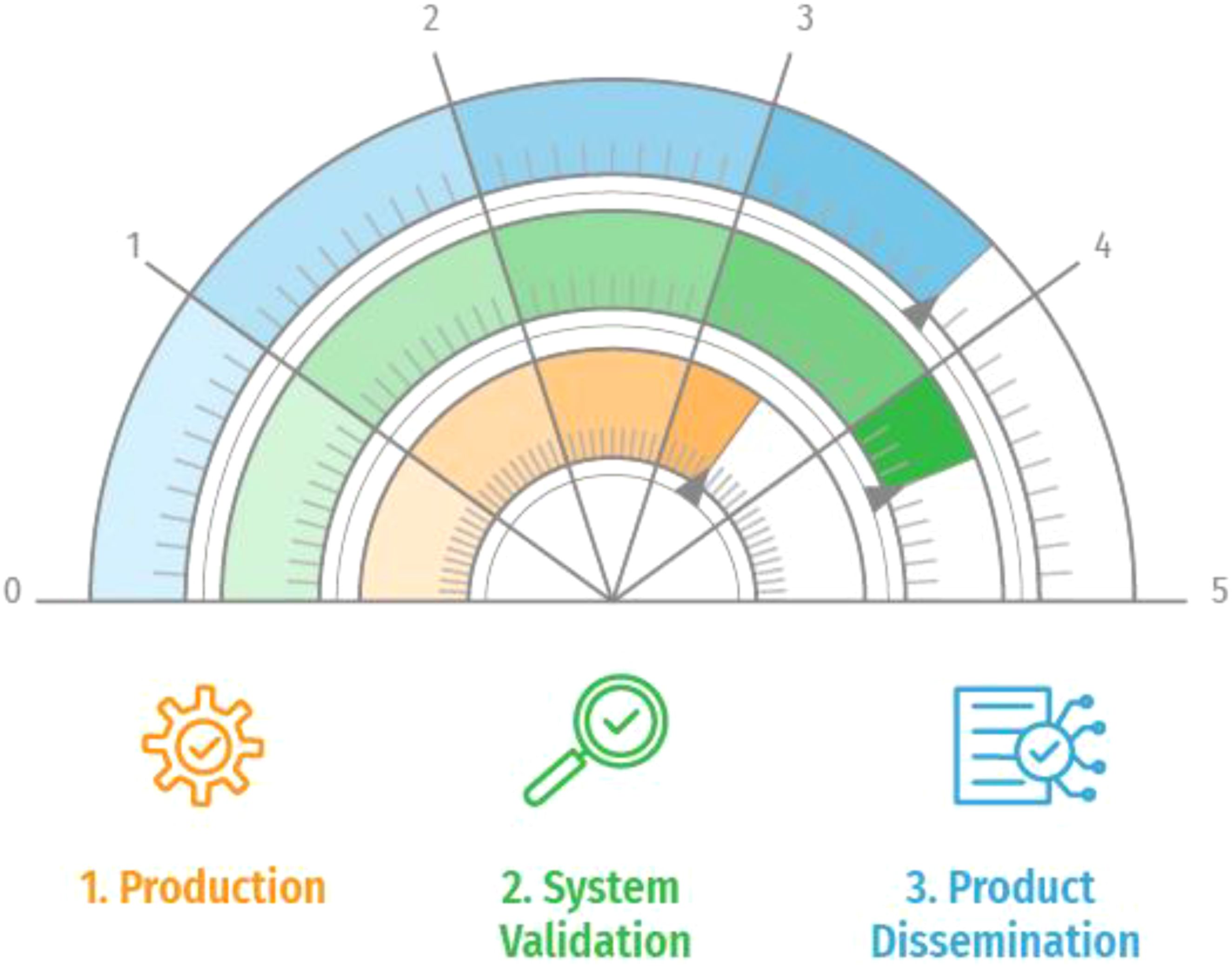
Figure 1. The OceanPrediction DCC operational readiness level: the first digit “Production” reflects the reliability of the service, focusing on operational aspects rather than product quality; the second digit “System Validation” monitors the level of validation of the service; the third digit “Product Dissemination” assesses the various degrees of product dissemination achievable by the system.
3.2 Computing the operational readiness level
The centers responsible for operating a service will calculate the ORL for their respective systems. The results will only be public if the center responsible for the system decides so.
The process of computing the Operational Readiness Level of a service is summarized in Figure 2, and a practical example is presented in Figure 3. It consists of a ladder where the advances are achieved by fulfilling the criteria (C) as expressed in each of the categories - production (P), validation (V), and dissemination (D) (PC, VC, and DC, respectively represented in Figure 2). The list of criteria per each category is fully shown in Figures 4–9. The computation of each digit’s value is done following a two-step process:
● First Step: computation of the Base Level. The base level is defined as the point where all the criteria below are fulfilled. For example, to reach Base Level 2 (intermediate) as shown in Figure 2 for Production (first digit of the ORL), all the criteria under PC-0 and PC-1 must be fulfilled. The number of points to be added to the digit on this first step corresponds to the achieved base level (for example, 2 points for Base Level 2).
● Second Step: additional points from higher increment criteria. Once the base level is determined, the score can still be increased by adding points corresponding to the fulfilled criteria of the two adjacent superior levels. For example, if the Base Level for Production is 1 (Basic) because there is a criterium on PC-1 that is not fulfilled, the score may still be increased by adding the points corresponding to the criteria fulfilled in PC-1 and PC-2, as represented in Figure 3.
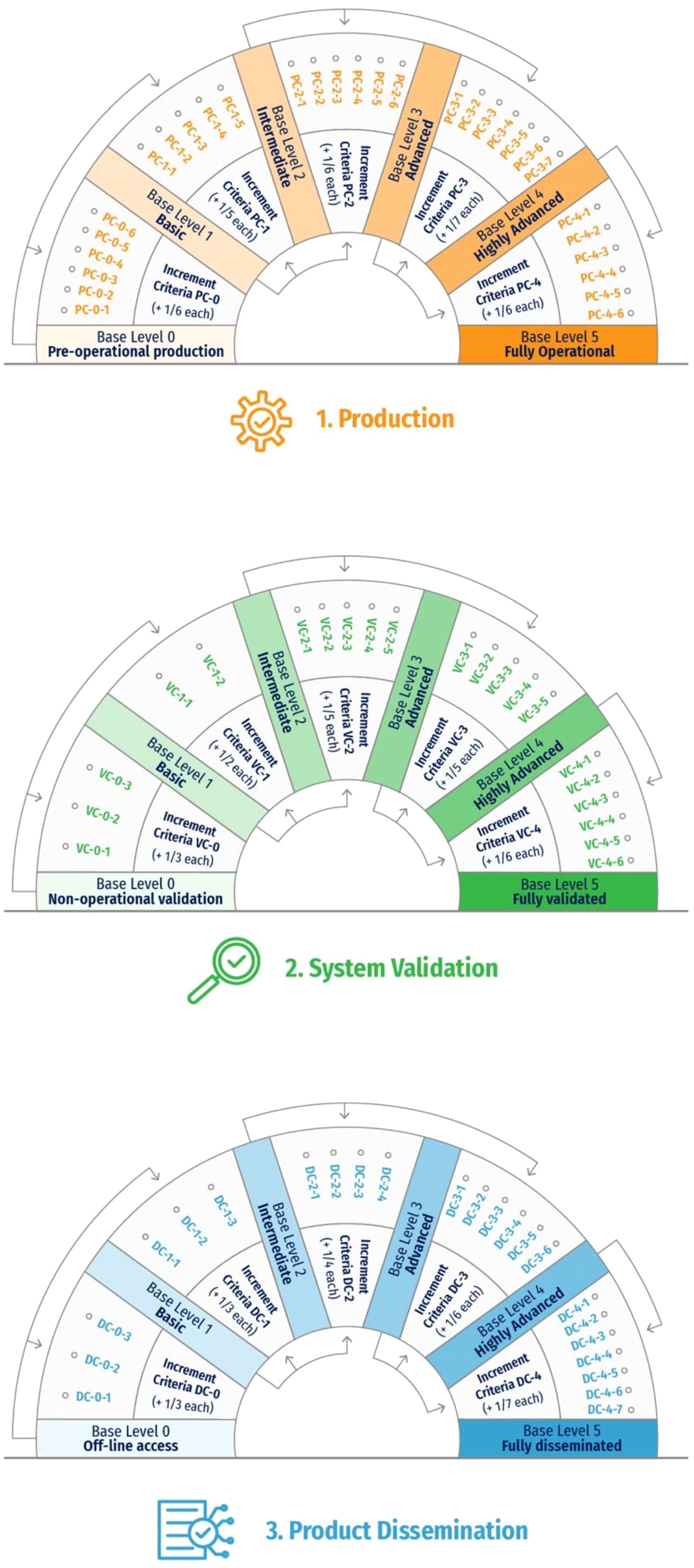
Figure 2. Workflow for the calculation of ORL digits for Production (top), System Validation (middle), and Product Dissemination (bottom).
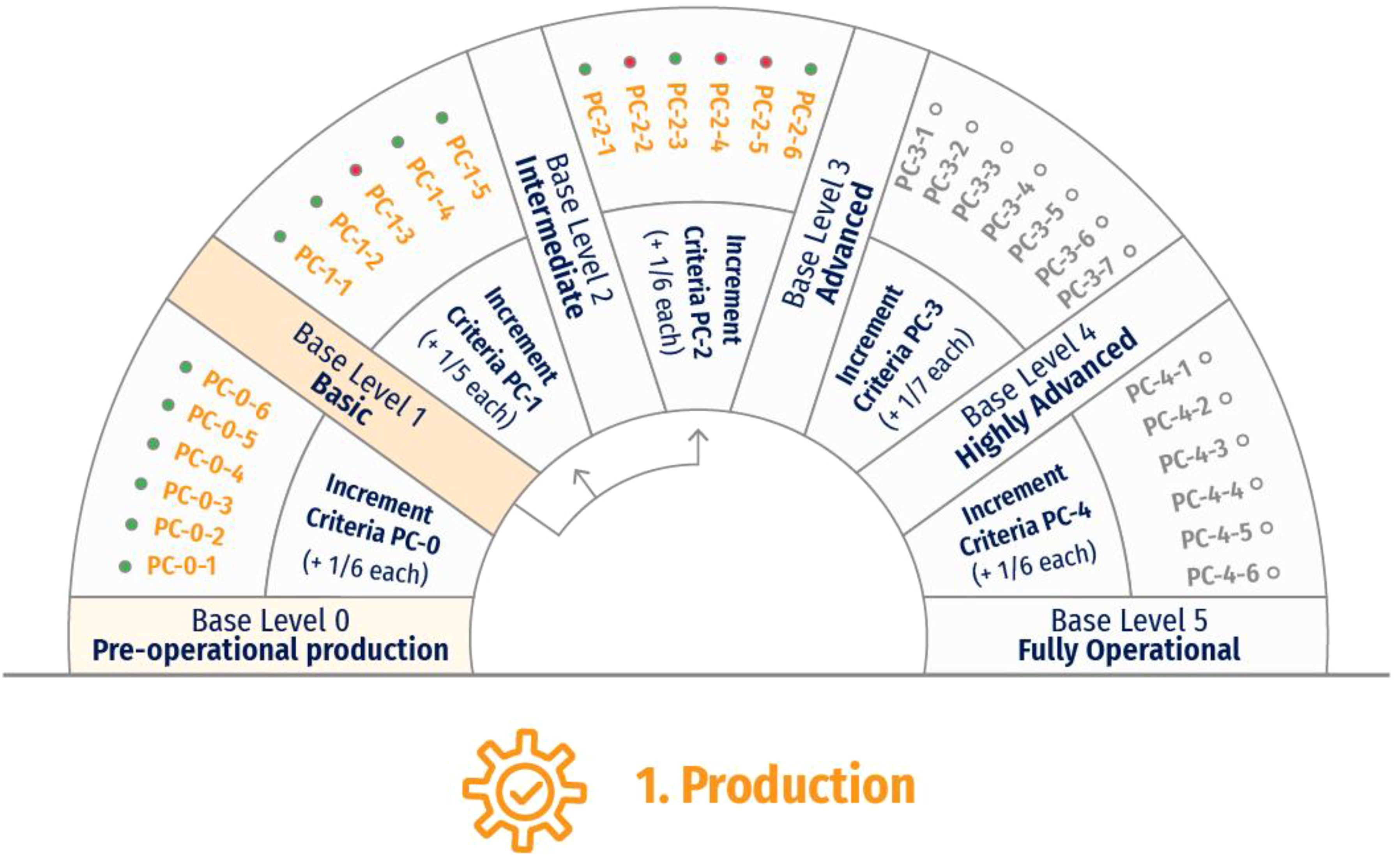
Figure 3. Example of ORL computation methodology. In this case, the Base Level is 1 (resulting from a missing criterium in PC-1, represented with a red dot). The resulting score for this index is given by summing fulfilled criteria’s scores (green dots) and resulting in 1 + (4*(1/5)) + (3*(1/6)) = 2.3. Therefore, the system can be catalogued as “Intermediate” in terms of Production, since the index is larger than 2 (although the label is less significant than the figure and should be used only for communication purposes).
Note that the relevant outcome of the process is a set of numerical values corresponding to the ORL digits. If a label is desired for communication purposes, the one to be applied corresponds to the number resulting from the application of the two steps as described above, not just the computation of the Base Level resulting from the first one. For example, if one of the criteria of PC-1 is not fulfilled (see Figure 3, with a red dot), a system has a Base Level of 1 for Production, but if after adding all the points corresponding to the Second Step the final score is 2.3, the system could be described as “Intermediate” in terms of Production.
This way of computing the ORL promotes that all the steps along the ladder are fulfilled, but, at the same time, it allows some flexibility to increase the ORL in case advanced features corresponding to two adjacent higher levels are available, also encouraging the adoption of best practices corresponding to higher levels of the increment criteria. Additionally, this methodology prevents high scores when one of the very initial conditions on the ladder is not fulfilled.
4 Discussion
4.1 Accuracy of ocean forecasting services
This paper has described best practices for operating, validating, and disseminating the results of an ocean forecasting service. Based on these, an ORL has been created to promote its adoption. The application of this ORL will guide the forecasting community toward more robust, timely, resilient, user-friendly, validated, and interoperable services. Nevertheless, this is not enough to guarantee accurate services.
While accuracy is often viewed as an objective measure, its interpretation is inherently relative, and shaped by various factors and considerations. Accuracy in ocean forecasting is a relative concept, and what is considered accurate can vary based on users’ needs. This was clearly shown in Ciliberti et al. (2023), where users and developers showed a large discrepancy in the evaluation of the accuracy of ocean forecasting services. This work demonstrated a very different perception of the concept depending on the person asked. While end-users are usually quite satisfied with the systems, experts are generally more critical. These different perceptions are linked to several factors. For example, a port pilot could be satisfied by knowing if wave heights will or will not be over a given threshold, but their decision-making is not affected if the waves have one value or another over that threshold, because operations will be cancelled independently of how much the variable is exceeding the threshold. In summary, a system could be accurate enough for a particular application, but not for a different one.
It is also impossible to define accuracy in absolute terms. For example, a system with a given root mean square error that is operated in an open ocean region dominated by mesoscale and sub-mesoscale baroclinic circulation could be considered accurate, but a similar system running on a region dominated by tides and having the same error figures could be in contrast considered inaccurate because on these areas the solutions are harmonic, easier to characterize, and less prone to large errors.
On top of that, accuracy is mostly related to all the complex factors related to the numerical modeling: choice of a numerical model (that depends on the temporal and spatial scale and on the EOV to be solved), quality of bathymetry, setup of the system, abundancy of quality-controlled observations, input data treatment, nesting technique, etc.
All the previous considerations imply that establishing best practices for the improvement of accuracy is a task that depends on many factors linked to the “art” of numerical modeling, on the EOV to be solved, and on the expected application of the forecasting system. Therefore, is a problem different in nature to others explored in this paper (operations, validation, and dissemination). Since the criteria related to accuracy improvement are also model-dependent, and therefore complex and cumbersome in application, including them on the new tool would jeopardize its simplicity and usefulness, and in consequence, we have excluded them. For a user-oriented evaluation of the accuracy of a service, we suggest following an approach like the one in Ciliberti et al. (2023).
4.2 Connection with observations in the framework of the decade
The value of an Ocean Forecasting platform is heavily dependent on the data that is available to it. Difficulty in finding or accessing data, or latency issues, will affect the ability of the system to provide timely forecasts, and it will impact the user experience of the user interacting with the platform. Achieving ease of access to the necessary data, and ensuring a low latency, requires that the data, from the time of measurement through to the time of ingest to the platform be FAIR and that it be adequately described by metadata that is fit for purpose. Therefore, an effective ocean data value chain requires three fundamental components as core and foundational activities: Ocean Observing, Ocean Data Sharing, and Ocean Forecasting.
The Ocean Decade presents a unique opportunity to cultivate these essential components coherently, laying the groundwork for robust advancements in addressing the ten Ocean Decade Challenges. Within this framework, the Decade Coordination Offices (DCO) of the Ocean Observing5, and the Ocean Data Sharing6, together with the OceanPrediction DCC are actively engaging with these aspects. These collaborative bodies, interconnected and working in tandem, serve as the backbone for various Decade activities, encompassing thematic and geographical dimensions. Their concerted efforts not only address the challenges of the Decade but also foster the development of the Ocean data value chain worldwide, extending its implementation beyond just the more technically advanced regions.
This paper articulates the collective commitment of the Ocean Observing DCO, the Ocean Data Sharing DCO, and the OceanPrediction DCC to collaborate to enhance our global capacity to develop robust ocean digital ecosystems that are actively used for decision-making for sustainable ocean management. To achieve this objective, we advocate for the collaborative development of architectural designs for key elements within the value chain related to Ocean Observing, Ocean Data Sharing, and Ocean Forecasting.
These architectures will encompass shared data standards and employ well-identified tools. Accompanied by best practice recommendations, they will serve as guidelines to foster the development of observation and forecasting services, with a specific emphasis on less developed countries. For example, an ORL index for ocean observations is needed and will be developed to check if data is ready for ingestion and use in an ocean forecasting platform. While less descriptive metadata may be fit for purpose for simple analysis – indicated by a lower level ORL score, ocean forecasting systems that perform complex analysis with low latency will require higher levels of readiness and therefore more detailed metadata – as would be indicated by a higher ORL score.
The overarching goal is to present straightforward and easily implementable recommendations/designs, all of which must receive endorsement from the Ocean Decade. If feasible, additional endorsements from relevant bodies will be sought to expand the scope of adoption. Other DCCs and DCOs, such as those focused on best practices or coastal resilience, will play a vital role in disseminating these insights.
This comprehensive approach is anticipated to significantly diminish the existing gaps, stimulate the creation of new services in developing countries, and facilitate interoperability and integration into Digital Twins, fostering collaboration even among the most developed regions.
Finally, the importance of ocean forecasting services in the design of observing services must be highlighted. The global ocean observing system of today was designed to answer the questions that we had about the ocean yesterday. The global ocean observing system of tomorrow, discussed today, will need to be designed so that ocean forecasting systems and their users will get the information they need to understand and mitigate climate change, biodiversity loss, etc. Ocean forecasting platforms and their end-users therefore have a key role in clarifying for the ocean-observing community what data is important, in what priority, and to what degree of resolution, accuracy, and confidence level. This feedback loop must be actively considered and built into the digital ecosystem, of which the ocean forecasting platform is the most visible part.
4.3 Applying the ORL to a real-world case
During the development of the methodology for computing the ORL, the concept was tested with several system operators worldwide. This process led to numerous improvements and clarifications in the formulation of the questions that make up the ORL. In this section, we present the results of one such exercise, using the IBI-MFC (Iberia-Biscay-Irish Monitoring and Forecasting Center, Sotillo et al., 2021a), a component of the Copernicus Marine Service7, as an example.
The analyzed system was the IBI Ocean Physics (IBI-PHY) Analysis and Forecasting System (Amo-Baladron et al., 2023), which, together with the Biogeochemical and Wave components, is part of the IBI-MFC. The IBI-PHY system provides near real-time information on the physical ocean state in the Northeast Atlantic and the Western Mediterranean basins at the horizontal resolution of 1/36° and 50 vertical layers. The system provides forecasts with a horizon of 5 days (extended up to 10 days from Nov 2024), and weekly analyses; a second “definitive” analysis is performed two weeks after to benefit from the best observational coverage and lateral open boundary conditions provided by the Global Ocean Analysis and Forecasting System (Le Galloudec et al., 2023). Operational assessment of product quality is performed through the NARVAL tool (Lorente et al., 2019) and with new Python-based tools for the calculation of more metrics and Estimated Accuracy Numbers (EAN, Ciliberti et al., 2024): that are then delivered to the Copernicus Marine Product Quality Dashboard (Sotillo et al., 2021b). All the IBI-MFC operational production is performed in the supercomputer Finisterrae-3 (at the Centro de Supercomputación de Galicia). IBI-PHY operational datasets are then uploaded (in standard NetCDF or Zarr formats) to the Copernicus Marine Data Store for their dissemination to end-users through the three main interfaces offered by the Copernicus Marine Service
The computation of ORL digits for this IBI-PHY forecast system is performed using the steps described in Section 3. The main conclusions for each estimated digit are discussed in the following:
4.3.1 Production (final score 4.7)
The IBI-PHY system achieves a high ORL digit for production thanks to its reliable production capacity and robust operational suites. Its modularity guarantees adequate control of each processing step – from upstream data download and access to monitoring of parallel execution of the core model, to optimized post-processing for the transformation of model results to NetCDF CF-compliant (CF stays for Climate Forecast convention8) products and final delivery to the Copernicus Marine Data Store for further dissemination. The operational chain is constantly monitored to solve automatically, or through human intervention, any potential failure that can compromise the timely delivery of final products (and the Service Level Agreement compliance). The computational resources needed are guaranteed during the whole lifecycle of the chain and works are performed under a controlled environment. Expert technical staff is dedicated daily to operating the service (mainly for troubleshooting and support to users through a Service Desk component), and a plan for human resources management (outside normal working time and holidays included). The IBI-MFC Operational Team designs and maintains updated technical documentation both for users (e.g., Product User Manual, delivered through the Copernicus Marine catalog) and for internal purposes (describing operational chain functionalities, processes, etc). Currently, the IBI-PHY production unit does not account for any other HPC backup resources that could be operated in case of an extended unscheduled downtime of the nominal one.
4.3.2 Validation (final score 4.4)
The IBI-PHY products are characterized by an advanced scientific assessment, based on a multi-observations/multi-models/multi-parameters approach. For each planned release, including new service evolutions, the IBI Development Team performs a pre-operational model qualification of selected EOV to assess accuracy, and capacity in reproducing seasonally the main oceanographic features in the IBI region, of the new proposed numerical solution. Metrics are then analyzed in the Quality Information Document (delivered through the Copernicus Marine catalog) or made available to registered users through the NARVAL (North Atlantic Regional VALidation, Lorente et al., 2019) application. Once in operations, delayed model validation is performed monthly to assess analysis and forecast datasets (using for this aim satellite sea surface temperature, sea level anomaly, and in situ temperature and salinity observations provided by mooring and Argo floats): resulting EANs are then delivered to the Copernicus Marine Product Quality Dashboard9. Also, a daily online validation of the operational forecast cycles is performed. Furthermore, the IBI-PHY solution is intercompared with its parent model – the Global Ocean forecasting system (Le Galloudec et al., 2023) – as well as with other available model solutions in the overlapping area, such as the Mediterranean forecasting system (Clementi et al., 2021). Currently, assessment of the IBI-PHY operational product does not include calculation of tailored metrics, uncertainties, and process-oriented validation, nor update of metrics in case new observational data are included in the product catalog in near real-time.
4.3.3 Product Dissemination (final score 4.8)
The IBI-PHY NRT datasets, once produced, are delivered to the Copernicus Marine Data Store, which is in charge of implementing a set of advanced interfaces for data access and download as well as operational visualization of EOV through an interactive mapping capability. A very high score is then guaranteed by the consolidated service, which also offers user support through a dedicated local Service Desk. The IBI-MFC Team delivers and discusses system and service evolution plans with the Copernicus Marine Technical Coordination, ensuring a smooth transition to new versions, communication with users, and proper upgrade of technical interfaces for data access and interoperability. The Marine Data Store technical infrastructure establishes functionalities for optimal data access, while the Copernicus Marine Service is in charge of tracking the number of users that access and use the IBI-PHY operational products, producing relevant statistics, shared with the IBI-MFC for addressing, if needed, the future evolution of product catalog. KPIs are produced by the IBI-MFC operational team and currently, the service does not offer a 24/7 Service Desk (Copernicus Marine Service proposes as a baseline an 8/5 human-supported service on working days), even if it implements 2 levels of support (i.e., Level 1 through chatbot and Level 2 for direct contact of Service Desk Operator and IBI-PHY Technical Experts).
5 Conclusions and ways forward
This paper introduces a set of Best Practices designed to enhance the operational aspects of ocean forecasting services, as well as to better validate and disseminate their products. Additionally, it introduces a novel concept: the Operational Readiness Level (ORL), which will serve as a tool to encourage adopting these Practices.
Adopting the ORL will have the following advantages:
● A mechanism for users and developers to understand the state of an operational forecast system.
● A way to guide, stimulate, and track services development progression for an individual system, but also collectively within a region or the world.
● Promote the adoption of tools, data standards, and Best Practices. System developers can assess where improvements to their systems are needed to progress up the readiness ladder.
● A mechanism to encourage and endorse services to join common frameworks. The ORL can serve to establish operational thresholds for common framework managers to permit the integration of new systems (i.e., into Digital Twins).
● A mechanism for system managers to inform users of a justified level of trust when applying its results to management and policy.
It is worth mentioning that the presented description of many of the best practices could benefit from a more detailed description. We propose that the Expert Team on Operational Ocean Forecast Systems (ETOOFS10), in close collaboration with OceanPrediction DCC, the Ocean Practices Programme, ForeSea, and others, actively work to refine these definitions by providing greater detail and specificity. Once fully detailed, these best practices will be incorporated into a new GOOS/ETOOFS document, complementing the existing ETOOFS guide (Alvarez-Fanjul et al., 2022).
In line with this strategy, ETOOFS, in collaboration with OceanPrediction DCC, will develop an online tool to evaluate ORL for existing ocean forecasting services. This tool will help identify which best practices are yet to be implemented at a given service, thus guiding its development priorities. The institutions responsible for operating a service will assess the ORL for their respective systems, with the results made public only if the institution decides to do so. Additionally, if requested by the relevant institutions, ETOOFS will provide certification for the computed ORL, indicating “ETOOFS operationally ready” status upon achieving certain scores.
Data availability statement
The original contributions presented in the study are included in the article/supplementary material. Further inquiries can be directed to the corresponding author.
Author contributions
EA: Conceptualization, Investigation, Methodology, Resources, Supervision, Validation, Writing – original draft, Writing – review & editing. SAC: Investigation, Methodology, Validation, Visualization, Writing – review & editing, Writing – original draft. JP: Methodology, Validation, Writing – review & editing, Investigation, Writing – original draft. KW: Project administration, Writing – review & editing, Resources. PB: Writing – review & editing, Funding acquisition, Resources, Validation. FA: Writing – review & editing. AA: Writing – review & editing. KA: Writing – review & editing. RA: Validation, Writing – review & editing. MB: Writing – review & editing. LB: Validation, Writing – review & editing. SB: Writing – review & editing. GB: Writing – review & editing. JC: Methodology, Validation, Writing – review & editing. AC: Writing – review & editing. ECh: Writing – review & editing. SCi: Writing – review & editing. MC: Writing – review & editing. ECl: Validation, Writing – review & editing. LC: Writing – review & editing. GC (21st Author): Writing – review & editing. GC (22nd Author): Writing – review & editing. SCo: Writing – review & editing. FD: Writing – review & editing. MD: Validation, Writing – review & editing. YD: Validation, Writing – review & editing. RD: Writing – review & editing. GE: Writing – review & editing. GF: Validation, Writing – review & editing. KF: Writing – review & editing. DF: Writing – review & editing. OL: Writing – review & editing. XH: Writing – review & editing. JL: Writing – review & editing. PHe: Writing – review & editing. FH: Writing – review & editing. PHo: Writing – review & editing. IH: Writing – review & editing. SuJ: Writing – review & editing. SiJ: Writing – review & editing. PL: Validation, Writing – review & editing. SL: Writing – review & editing. MM (43rd Author): Writing – review & editing. MM (44th Author): Writing – review & editing. PM: Writing – review & editing. TM: Methodology, Validation, Writing – review & editing. AMe: Writing – review & editing. YM: Writing – review & editing. AMo: Writing – review & editing. AN: Writing – review & editing. FO: Writing – review & editing. AP: Writing – review & editing. FQ: Writing – review & editing. HR: Writing – review & editing. JR: Validation, Writing – review & editing. SS: Writing – review & editing. AS: Writing – review & editing. JSi: Writing – review & editing. MS: Validation, Writing – review & editing. JSt: Writing – review & editing. CT: Writing – review & editing. PT: Writing – review & editing. MT: Methodology, Validation, Writing – review & editing. JVa: Writing – review & editing. JVe: Validation, Writing – review & editing. KV: Writing – review & editing. LW: Writing – review & editing. JW: Writing – review & editing. AZ: Validation, Writing – review & editing. RZ: Writing – review & editing.
Funding
The author(s) declare financial support was received for the research, authorship, and/or publication of this article. This paper was prepared under OceanPrediction DCC funding, but most of the authors have contributed on a voluntary basis. AC was supported by the BELSPO FedTwin Project Recap (Prf-2019-008).
Acknowledgments
This document was prepared thanks to the framework provided by the Ocean Decade. The participation of several Decade programmes, such as Foresea, Ocean practices, DITTO, and others has been critical for the elaboration of the ORL criteria. Support for the evolution and application of ORL will be provided by GOOS-ETOOFS.
Conflict of interest
Authors SC, RA, MS, JVa were employed by company Nologin Oceanic Weather Systems. Author KA was employed by NVIDIA Corporate. Author AN was employed by ETT SpA. Author FO was employed by company IBM Research.
The remaining authors declare that the research was conducted in the absence of any commercial or financial relationships that could be construed as a potential conflict of interest.
The author(s) declared that they were an editorial board member of Frontiers, at the time of submission. This had no impact on the peer review process and the final decision.
Publisher’s note
All claims expressed in this article are solely those of the authors and do not necessarily represent those of their affiliated organizations, or those of the publisher, the editors and the reviewers. Any product that may be evaluated in this article, or claim that may be made by its manufacturer, is not guaranteed or endorsed by the publisher.
Footnotes
- ^ https://www.unoceanprediction.org/en
- ^ https://oceandecade.org/actions/foresea-the-ocean-prediction-capacity-of-the-future/
- ^ https://oceandecade.org/actions/ocean-best-practices-for-the-decade/
- ^ https://ditto-oceandecade.org/
- ^ https://oceandecade.org/actions/decade-coordination-office-for-ocean-observing/
- ^ https://oceandatasharing-dco.org/
- ^ https://marine.copernicus.eu/
- ^ https://cfconventions.org/
- ^ https://pqd.mercator-ocean.fr/
- ^ https://goosocean.org/who-we-are/expert-team-on-operational-ocean-forecast-systems-etoofs/
References
Alvarez-Fanjul E., Ciliberti S., Bahurel P. (2022). Implementing Operational Ocean Monitoring and Forecasting Systems (IOC-UNESCO, GOOS-275), 16. doi: 10.48670/ETOOFS
Amo-Baladron A., Reffray G., Levier B., Escudier R., Gutknecht E., Aznar R., et al. (2023). Product User Manual for Atlantic-Iberian Biscay Irish-Ocean Physics Analysis and Forecast Product IBI_ANALYSISFORECAST_PHY_005_001. doi: 10.48670/moi-00027
Bonino G., Galimberti G., Masina S., McAdam R., Clementi E. (2024). Machine learning methods to predict sea surface temperature and marine heatwave occurrence: a case study of the Mediterranean Sea. Oc. Sci. 20, 417–432. doi: 10.5194/os-20-417-2024
Brassington G. B. (2021). “System design for operational ocean forecasting,” in Operational Oceanography in the 21st Century, Chapter 18. Eds. Schiller A., Brassington G. B. (Springer Science+Business Media B.V. 2011) 03, 327-328. doi: 10.1007/978-94-007-0332-2_18
Buck J. J. H., Bainbridge S. J., Burger E. F., Kraberg A. C., Casari M., Casey K. S., et al. (2019). Ocean data product integration through innovation - the next level of data interoperability. Front. Mar. Sci. 6. doi: 10.3389/fmars.2019.00032
Bushnell M., Pearlman J. (2024). Ocean best practices system endorsement: guidance for the ocean community, version 2024-03-20. OBPS, 8pp. doi: 10.25607/OBP-1983
Byrne D., Polton J., O’Dea E., Williams J. (2023). Using the COAsT Python package to develop a standardised validation workflow for ocean physics models. GMD 16, 13, 3749–3764. doi: 10.5194/gmd-16-3749-2023
Chaigneau A. A., Law-Chune S., Melet A., Voldoire A., Reffray G., Aouf L. (2023). Impact of sea level changes on future wave conditions along the coasts of western Europe. Ocean Sci. 19, 1123–1143. doi: 10.5194/os-19-1123-2023
Ciliberti S. A., Alonso A., Amo-Baladron A., Castrillo L., Garcia M., Escudier R., et al. (2024). Progresses in assessing the quality of the Copernicus Marine near real time Northeast Atlantic and Shelf Seas models application, EGU General Assembly 2024, Vienna, Austria, 14-19 Apr 2024, EGU24-10942. doi: 10.5194/egusphere-egu24-10942
Ciliberti S. A., Alvarez Fanjul E., Pearlman J., Wilmer-Becker K., Bahurel P., Ardhuin F., et al. (2023). “Evaluation of operational ocean forecasting systems from the perspective of the users and the experts,” in 7th edition of the Copernicus Ocean State Report (OSR7). Eds. von Schuckmann K., Moreira L., Le Traon P.-Y., Grégoire M., Marcos M., Staneva J., et al (Copernicus Publications, State Planet), 1–osr7, 2. doi: 10.5194/sp-1-osr7-2-2023
Clementi E., Aydogdu A., Goglio A. C., Pistoia J., Escudier R., Drudi M., et al. (2021). Mediterranean Sea Physical Analysis and Forecast (CMEMS MED-Currents, EAS6 system) (Version 1) [Data set] (Copernicus Monitoring Environment Marine Service (CMEMS). doi: 10.25423/CMCC/MEDSEA_ANALYSISFORECAST_PHY_006_013_EAS8
Cucco A., Simeone S., Quattrocchi G., Sorgente R., Pes A., Satta A., et al. (2024). Operational oceanography in ports and coastal areas, applications for the management of pollution events. J. Mar. Sci. Eng. 12, 380. doi: 10.3390/jmse12030380
Davidson F., Alvera-Azcárate A., Barth A., Brassington G. B., Chassignet E. P., Clementi E., et al. (2019). Synergies in operational oceanography: the intrinsic need for sustained ocean observations. Front. Mar. Sci. 6. doi: 10.3389/fmars.2019.00450
de Boisséson E., Balmaseda M. A. (2024). Predictability of marine heatwaves: assessment based on the ECMWF seasonal forecast system. Oc. Sci. 20, 265–278. doi: 10.5194/os-20-265-2024
Fennel K., Gehlen M., Brasseur P., Brown C. W., Ciavatta S., Cossarini G., et al. (2019). Advancing marine biogeochemical and ecosystem reanalyses and forecasts as tools for monitoring and managing ecosystem health. Front. Mar. Sci. 6. doi: 10.3389/fmars.2019.00089
Goksu S., Arslan O. (2024). Evaluation of dynamic risk factors for ship operations. AJMOA, 1–27. doi: 10.1080/18366503.2024.2340170
Hartog J. R., Spillman C. M., Smith G., Hobday A. J. (2023). Forecasts of marine heatwaves for marine industries: Reducing risk, building resilience and enhancing management responses. Deep-Sea Res. Pt. II 209, 105276. doi: 10.1016/j.dsr2.2023.105276
Hernandez F., Blockley E., Brassington G. B., Davidson F., Divakaran P., Drévillon M., et al. (2015). Recent progress in performance evaluations and near real-time assessment of operational ocean products. J. Oper. Oceanogr. 8, S2, s221–s238. doi: 10.1080/1755876X.2015.1050282
Hernandez F., Smith G., Baetens K., Cossarini G., Garcia-Hermosa I., Drévillon M., et al. (2018). “Measuring performances, skill and accuracy in operational oceanography: New challenges and approaches,” in New Frontiers in Operational Oceanography. Eds. Chassignet E., Pascual A., Tintoré J., Verron J. (GODAE OceanView), 759–796. doi: 10.17125/gov2018.ch29
Jeuring J., Samuelsen E. M., Lamers M., Muller M., Åge Hjøllo B., Bertino L., et al. (2024). Map-based ensemble forecasts for maritime operations: an interactive usability assessment with decision scenarios. WCAS 16, 235–256. doi: 10.1175/WCAS-D-23-0076.1
Kampouris K., Vervatis V., Karagiorgos J., Sofianos S. (2021). Oil spill model uncertainty quantification using an atmospheric ensemble. Oc. Sci. 17, 919–934. doi: 10.5194/os-17-919-2021
Keramea P., Kokkos N., Zodiatis G., Sylaios G. (2023). Modes of operation and forcing in oil spill modeling: state-of-art, deficiencies and challenges. J. Mar. Sci. Eng. 11, 1165. doi: 10.3390/jmse11061165
Kim T., Lee W.-D. (2022). Review on applications of machine learning in coastal and ocean engineering. JOET 36, 194–210. doi: 10.26748/KSOE.2022.007
Kourafalou V. H., De Mey P., Le Henaff M., Charria G., Edwards C. A., He R., et al. (2015). Coastal Ocean Forecasting: system integration and evaluation. J. Oper. Oceanogr. 8, S1, s127–s146. doi: 10.1080/1755876X.2015.1022336
Le Galloudec, Law Chune S., Nouel L., Fernandez E., Derval C., Tressol M., et al. (2023). Product User Manual for Global Ocean Physical Analysis and Forecasting Product GLOBAL_ANALYSISFORECAST_PHY_001_024. doi: 10.48670/moi-00016
Le Traon P. Y., Abadie V., Ali A., Aouf L., Ardhuin F., Artioli Y., et al. (2021). The Copernicus Marine Service from 2015 to 2021: six years of achievements. Special Issue Mercator Océan J. 57. doi: 10.48670/moi-cafr-n813
Le Traon P.-Y., Reppucci A., Alvarez Fanjul E., Aouf L., Behrens A., Belmonte M., et al. (2019). From observation to information and users: the Copernicus marine service perspective. Front. Mar. Sci. 6. doi: 10.3389/fmars.2019.00234
Link J. S., Thur S., Matlock G., Grasso M. (2023) Why we need weather forecast analogues for marine ecosystems. ICES J. Mar. Sci. 80, 2087–2098. doi: 10.1093/icesjms/fsad143
Lorente P., Sotillo M. G., Amo-Baladron A., Aznar R., Levier B., Aouf L., et al. (2019). “The NARVAL software toolbox in support of ocean models skill assessment at regional and coastal scales,” in Computational Science – ICCS 2019. LNCS. Ed. Rodrigues J., et al (Springer, Cham), 11539. doi: 10.1007/978-3-030-22747-0_25
Mantovani C., Pearlman J., Rubio A., Przeslawski R., Bushnell M., Simpson P., et al. (2024). An ocean practices maturity model: from good to best practices. Front. Mar. Sci. 11, 1415374. doi: 10.3389/fmars.2024.1415374
Morim J., Wahl T., Vitousek S., Santamaria-Aguilar S., Young I., Hemer M. (2023). Understanding uncertainties in contemporary and future extreme wave events for broad-scale impact and adaptation planning. Sci. Adv. 9, eade3170. doi: 10.1126/sciadv.ade3170
PubMed Abstract | PubMed Abstract | Crossref Full Text | Google Scholar
Nezhad M. M., Neshat M., Sylaios G., Astiaso Garcia D. (2024). Marine energy digitalization digital twin’s approaches. Renew. Sustain. Energy Rev. 191, 114065. doi: 10.1016/j.rser.2023.114065
Pearlman J., Bushnell M., Coppola L., Karstensen J., Buttigieg P. L., Pearlman F., et al. (2019). Evolving and sustaining ocean best practices and standards for the next decade. Front. Mar. Sci. 6. doi: 10.3389/fmars.2019.00277
Pearlman J., Buttigieg P. L., Bushnell M., Delgado C., Hermes J., Heslop E., et al. (2021). Evolving and sustaining ocean best practices to enable interoperability in the UN decade of ocean science for sustainable development. Front. Mar. Sci. 8. doi: 10.3389/fmars.2021.619685
Pérez Gómez B., Vilibić I., Šepić J., Međugorac I., Ličer M., Testut L., et al. (2022). Coastal sea level monitoring in the Mediterranean and Black seas. Ocean Sci. 18, 997–1053. doi: 10.5194/os-18-997-2022
Schultes E., Magagna B., Hettne K. M., Pergl R., Suchánek M., Kuhn T. (2020). “Reusable FAIR implementation profiles as accelerators of FAIR convergence,” in Advances in Conceptual Modeling. ER 2020. LNCS. Eds. Grossmann G., Ram S. (Springer, Cham), 12584. doi: 10.1007/978-3-030-65847-2_13
She J., Allen I., Buch E., Crise A., Johannessen J. A., Le Traon P.-Y., et al. (2016). Developing European operational oceanography for Blue Growth, climate change adaptation and mitigation, and ecosystem-based management. Oc. Sci. 12, 953–976. doi: 10.5194/os-12-953-2016
Snowden D., Tsontos V. M., Handegard N. O., Zarate M., O’Brien K., Casey K. S., et al. (2019). Data interoperability between elements of the global ocean observing system. Front. Mar. Sci. 6. doi: 10.3389/fmars.2019.00442
Sotillo M. G., Cailleau S., Aznar R., Aouf L., Barrera E., Dabrowski T., et al. (2021a). “The operational CMEMS IBI-MFC service today: review of major achievements along the Copernicus-1 service phase, (2015-2021),” in 9th EuroGOOS International Conference, Shom; Ifremer, Brest, France, May 2021. 321–328. Available at: https://hal.science/hal-03336265v2/document
Sotillo M. G., Cerralbo P., Lorente P., Grifoll M., Espino M., Sanchez-Arcilla A., et al. (2020). Coastal ocean forecasting in Spanish ports: the SAMOA operational service. J. Oper. Oceanogr. 13, 1, 37–1, 54. doi: 10.1080/1755876X.2019.1606765
Sotillo M. G., Garcia-Hermosa I., Drevillon M., Regnier C., Szcypta C., Hernandez F., et al. (2021b). Copernicus marine service product quality assessment. Special Issue Mercator Océan J. 57. doi: 10.48670/moi-cafr-n813
Sugawara D. (2021). Numerical modeling of tsunami: advances and future challenges after the 2011 Tohoku earthquake and tsunami. Earth Sci. Rev. 214, 103498. doi: 10.1016/j.earscirev.2020.103498
Tanhua T., Pouliquen S., Hausman J., O’Brien K., Bricher P., de Bruin T., et al. (2019). Ocean FAIR data services. Front. Mar. Sci. 6. doi: 10.3389/fmars.2019.00440
Tsushima H., Ohta Y. (2014). Review on near-field tsunami forecasting from offshore tsunami data and onshore GNSS data for tsunami early warning. J. Disaster Res. 9, 3. doi: 10.20965/jdr.2014.p0339
UNESCO-IOC. (2021). The United Nations decade of ocean science for sustainable development, (2021-2030): implementation plan. IOC/2021/ODS/20. Available online at: https://oceandecade.org/publications/ocean-decade-implementation-plan/ (Accessed May 25, 2024).
Visbeck M. (2018). Ocean science research is key for a sustainable future. Nat. Commun. 9, 690. doi: 10.1038/s41467-018-03158-3
PubMed Abstract | PubMed Abstract | Crossref Full Text | Google Scholar
Wilkinson M. D., Dumontier M., Aalbersberg I. J. J., Appleton G., Axton M., Baak A., et al. (2016). The FAIR Guiding Principles for scientific data management and stewardship. Sci. Data 3, 160018. doi: 10.1038/sdata.2016.18
PubMed Abstract | PubMed Abstract | Crossref Full Text | Google Scholar
Keywords: operational oceanography, ocean predictions, ocean observations, best practices, standards, data sharing, interoperability, digital twins
Citation: Alvarez Fanjul E, Ciliberti S, Pearlman J, Wilmer-Becker K, Bahurel P, Ardhuin F, Arnaud A, Azizzadenesheli K, Aznar R, Bell M, Bertino L, Behera S, Brassington G, Calewaert JB, Capet A, Chassignet E, Ciavatta S, Cirano M, Clementi E, Cornacchia L, Cossarini G, Coro G, Corney S, Davidson F, Drevillon M, Drillet Y, Dussurget R, El Serafy G, Fearon G, Fennel K, Ford D, Le Galloudec O, Huang X, Lellouche JM, Heimbach P, Hernandez F, Hogan P, Hoteit I, Joseph S, Josey S, Le Traon P-Y, Libralato S, Mancini M, Martin M, Matte P, McConnell T, Melet A, Miyazawa Y, Moore AM, Novellino A, O’Donncha F, Porter A, Qiao F, Regan H, Robert-Jones J, Sanikommu S, Schiller A, Siddorn J, Sotillo MG, Staneva J, Thomas-Courcoux C, Thupaki P, Tonani M, Garcia Valdecasas JM, Veitch J, von Schuckmann K, Wan L, Wilkin J, Zhong A and Zufic R (2024) Promoting best practices in ocean forecasting through an Operational Readiness Level. Front. Mar. Sci. 11:1443284. doi: 10.3389/fmars.2024.1443284
Received: 03 June 2024; Accepted: 17 October 2024;
Published: 18 November 2024.
Edited by:
Toste Tanhua, Helmholtz Association of German Research Centres (HZ), GermanyReviewed by:
Rebecca Cowley, Oceans and Atmosphere (CSIRO), AustraliaLongjiang Mu, Laoshan Laboratory, China
Qi Tang, Université de Neuchâtel, Switzerland
Copyright © 2024 Alvarez Fanjul, Ciliberti, Pearlman, Wilmer-Becker, Bahurel, Ardhuin, Arnaud, Azizzadenesheli, Aznar, Bell, Bertino, Behera, Brassington, Calewaert, Capet, Chassignet, Ciavatta, Cirano, Clementi, Cornacchia, Cossarini, Coro, Corney, Davidson, Drevillon, Drillet, Dussurget, El Serafy, Fearon, Fennel, Ford, Le Galloudec, Huang, Lellouche, Heimbach, Hernandez, Hogan, Hoteit, Joseph, Josey, Le Traon, Libralato, Mancini, Martin, Matte, McConnell, Melet, Miyazawa, Moore, Novellino, O’Donncha, Porter, Qiao, Regan, Robert-Jones, Sanikommu, Schiller, Siddorn, Sotillo, Staneva, Thomas-Courcoux, Thupaki, Tonani, Garcia Valdecasas, Veitch, von Schuckmann, Wan, Wilkin, Zhong and Zufic. This is an open-access article distributed under the terms of the Creative Commons Attribution License (CC BY). The use, distribution or reproduction in other forums is permitted, provided the original author(s) and the copyright owner(s) are credited and that the original publication in this journal is cited, in accordance with accepted academic practice. No use, distribution or reproduction is permitted which does not comply with these terms.
*Correspondence: E. Alvarez Fanjul, ZWFsdmFyZXpAbWVyY2F0b3Itb2NlYW4uZnI=